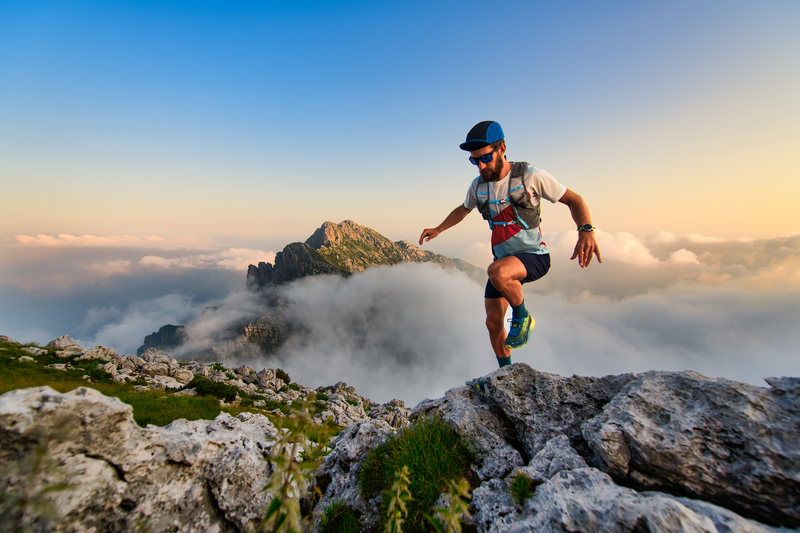
95% of researchers rate our articles as excellent or good
Learn more about the work of our research integrity team to safeguard the quality of each article we publish.
Find out more
ORIGINAL RESEARCH article
Front. Phys. , 18 November 2024
Sec. Social Physics
Volume 12 - 2024 | https://doi.org/10.3389/fphy.2024.1484589
This study proposes a novel perspective to calibrate the conditional value at risk (CoVaR) of countries based on the monotone composite quantile regression neural network (MCQRNN). MCQRNN can fix the “quantile crossing” problem, which is more robust in CoVaR estimating. In addition, we extend the MCQRNN method with quantile-on-quantile (QQ), which can avoid the bias in quantile regression. Building on the estimation results, we construct a systemic risk spillover network across countries in the Asia–Pacific region by considering the suffering and overflow effects. A comparison among MCQRNN, QRNN, and MCQRNN-QQ indicates the significance of monotone composite quantiles in modeling CoVaR. Additionally, the network analysis of composite risk spillovers illustrates the advantages of MCQRNN-QQ-CoVaR compared with QRNN-CoVaR. Moreover, the average composite systemic suffering index and the average composite systemic overflow index are introduced as country-specific measures that enable identifying systemically relevant countries during extreme events.
The Sino–U.S. trade war and the COVID-19 epidemic have caused huge fluctuations in Asia–Pacific stock markets. Compared with other economic organizations, the Asia–Pacific Economic Cooperation (APEC) organization provides a diversified financial markets environment, including developed and developing countries. In addition, APEC’s organizational structure and cooperation mechanism are more flexible, which means member countries cooperate while maintaining autonomy. Moreover, the economic structure of APEC countries is highly complementary; for example, resource-rich countries tend to trade closely with countries with developed manufacturing industries. By establishing interconnectivity, APEC encourages deeper cooperation in infrastructure, trade, and investment among countries in the region. According to statistical data, APEC members account for more than 40% of global trade. Within the region, trade among members is higher than trade with non-members. Despite the large volume of intra-APEC trade, APEC trade relations may depend more on bilateral relationships of large countries such as China and the United States than other economic organizations such as the European Union. This means that the trade closeness of APEC is greatly affected by the policy changes of large countries. Consequently, in the stage of Sino–U.S. trade friction, the trade cooperation of member countries will undergo great changes.
In the COVID-19 phase, the economic conditions of China and the United States will directly affect the risk level of the organization’s members. Therefore, the research on systemic financial risks among APEC member countries in this paper is helpful for a deeper analysis of the risk contagion mechanisms between different economies and could provide a supplement to existing literature. Growing uncertainty results in countries facing cross-border risk shocks, making the issue of systemic risk a renewed focus of research by academics and regulators. Systemic risk caused by the bankruptcy of systemically important economies is primarily the failure of the financial system. From the aspect of international markets, when an important node is damaged by a shock, other markets may also be affected and could eventually be contagious to the entire financial system.
To measure the systemic risk, studies in recent years have begun to focus on the risk contagion or spillover effect [1]. The former is mainly from a theoretical modeling perspective [2–6]. Additionally, more focus on empirical measurement provides compositions for the “edge” of the financial network. For instance, Engle (2002) constructs the GARCH-DCC method to capture the risk spillover among market indexes [7]. Rodriguez (2007) measures the interdependence among East Asian stock via switching-parameter copulas [8]. Billio et al. (2012) prompt the empirical framework of a risk spillover network based on Granger causality [9]. Diebold and Yılmaz (2014) involve variance decomposition in risk spillover and analyze the vulnerability by financial networks [10]. Baruník and Křehlík (2018) study the risk spillover from aspects of heterogeneous frequency responses to shock [11].
The risk modeling system of this paper is an addition to the conditional value at risk (
This paper seeks to expand the research perspective on systemic financial risk by examining the composite risk spillover effects among financial markets to avoid possible errors in the setting of quantiles. We construct the composite risk spillover measure based on the multi-quantile
Some studies focus on this problem. Acharya et al. (2017) assessed the expected loss below one quantile in dealing with the problem of quantile crossing [27]. Catania and Luati (2023) used a semiparametric model to satisfy the condition of non-crossing quantiles [28]. By investigating the
This paper proposes a quantile-on-quantile regression to examine the two-sided quantile in CoVaR estimation. The effects of systemic risk are analyzed by three-dimensional surface plots in empirical research. We extend the quantile regression in the systemic risk approach with a monotone composite quantile regression neural network, which can not only be suitable for solving the nonlinear issue but also optimize the “quantile crossing” problem. Moreover, we introduce the composite systemic suffering indicator, the composite systemic overflow indicator, and the total composite overflow indicator as three country-specific measures to identify systemically relevant countries in the Asia–Pacific region during extreme events.
This article makes three main contributions. First, we estimate the systemic financial risk through a new perspective that adopts 3D surface plots. Second, multi-quantiles are adopted in the model to capture the multi-state characteristics of risk. Third, MCQRNN is used to relieve the quantile crossing problem.
The remainder of the paper will be organized as follows: Section 2 will (i) introduce the multi-quantile
A quantile regression model based on a linear regression equation estimates parameters for
where
The difference between
However, the loss function in the quantile regression is not differentiable everywhere. It limits the use of artificial neural networks’ regular algorithms (ANNs) in
In addition, when the capacity of the neural network is large, it is prone to over-fitting problems. Choosing a modest neural network structure and hyperparameters is an effective approach often used in machine learning. In a single hidden layer network, the most important hyperparameter is the number of hidden nodes
where
The calibration details of
Step 1: Estimation of value at risk with
Because it is challenging to select common macro-state variables for all indexes, the linear quantile regression of value at risk is no longer suitable for measuring the tail risk of stock markets. Hence,
where
Step 2: Estimation of
First, the
Assume the
It should be noted that, in distinction to Keilbar and Wang, this paper estimates
Step 3: Calculation of composite risk spillover effects
To examine the margin impact of index
where the derivative of the transfer function is set as
where the
To reflect the sensitivity of average-level composite risk spillover to the two-sided quantiles, we decompose the
The partial spillover indicators are used to reflect the average impact of the two-sided risk state on the spillover from market
The non-linear characteristics of inter-market risk spillover can be analyzed by comparing the two types of partial spillover indices under different quantiles, and the mutation quantile of spillover can be captured. In addition, to reflect the extreme risk spillover, the
Last but not least, similar to the partial spillover indicators, four partial
The quantile crossing problem in the estimation of
For each market, the total number of “quantile crossing” problems can be accumulated by a monotonicity test. Because the
Step 4: Network analysis for composite systemic risk
The average measures of composite systemic risk will be gained in the final step. First, because the risk spillover of market index
Second, the composite systemic overflow indicator
To reveal the trend of the spillover effect, the total composite overflow indicator
The total overflow indicator can be regarded as the weighted sum of
Lastly, the adjusted adjacency matrix is defined as Equation 16.
The adjusted adjacency matrix accounts are the risk spillover indicators. Systemic spillover effects are thus determined by the marginal effects of the
We select the stock market indices of
As shown in Table 2, the total number of observations is 9,396 because some markets have missing samples due to the holidays or other factors. The average weekly return of each market is all positive, which indicates that the indices prices of Asia–Pacific stock markets at the end of 2021 are higher than they were in 2011. It is worth noting that the minimum value of all samples is −20.13%, which is the weekly return of the Chile index in the fourth week of March 2020. The maximum value occurred in the next week, which is 15.81% in Japan. The World Health Organization recognized the COVID-19 outbreak as a global pandemic on 11 March 2020. After 2 weeks of declines, most global stock markets rebounded sharply in the last week of March. Except for the stock markets of Mexico, mainland China, and Hong Kong, all the financial markets have a kurtosis of 3 or more in their return distributions. The return curves show a sharp peak pattern, which indicates that the outliers are more dispersed. In addition, the skewness of all the markets in the sample is negative, indicating that the return series of each market is left skewness; that is, the probability of negative extreme value is higher than positive. The augmented Dickey–Fuller (ADF) value of each market return is negative and less than the test critical value at the 1% significant level, rejecting the null hypothesis of a unit root. All Jarque–Bera (JB) statistics are significant at the 1% level, which rejects the null hypothesis of Gaussian distribution for the market returns.
The
The
Figure 3. Multi-quantile CAViaR diagrams of the United States and China. (A) The United States of America and (B) mainland China.
According to the count method described in Equation 11, the number of cross-quantile occurrences in each market is shown in Table 3. Because 18 pairs of adjacent quantiles and 522 periods in each market are compared, the first column of the table shows that the quantile crossing is a common problem in the estimation of
Figure 4. MCQRNN-QQ-CoVaR 3D-surface plots for other market indexes. (A) Australia, (B) Canada, (C) Chile, (D) Hong Kong, (E) Indonesia, (F) Japan, (G) Malaysia, (H) Mexico, (I) New Zealand, (J) Philippines, (K) Korea, (L) Russia, (M) Singapore, (N) Tai Wan, (O) Thailand, and (P) Vietnam.
We select China and the United States as outstanding examples. Figure 5 represents the level of
Figure 5. QQ-CoVaR surface and partial CoVaR curve of China and the United States. (A) QRNN-QQ-CoVaR of US, (B) MCQRNN-QQ-CoVaR of US, (C)
By comparing the
The reasons for the differences between China and the United States are market structure, investor base, and risk distribution. The American market is the largest and most diversified in the world, with companies representing a wide array of industries and sectors. This diversification helps to mitigate stand-alone risks associated with individual companies, sectors, or events. Additionally, American institutional investors like mutual funds, pension funds, and hedge funds play a significant role. These institutions usually employ sophisticated risk management strategies, including diversification and hedging, which further diminish sensitivity to stand-alone risks. Furthermore, the US market offers a wide array of financial instruments, such as options, futures, and swaps, that allow for the hedging of specific risks. This availability of hedging tools enables market participants to isolate and manage stand-alone risks effectively. Because the
Considering that
According to Equation 8, after the estimation of
As shown in Figure 6, each cell of the matrix represents a risk spillover correlation between the two markets. Where the color is darker, the level of risk spillover represented by the cell is higher. The cells with relatively dark colors in the graph are, respectively, the risk spillovers of HK →CN, CA →US, and US →CA. The mutual risk spillover between the United States and Canada can be explained by their geographical location, economic connections, and political policies. Canada is adjacent to the United States, and the two countries have a very close political relationship and an active trade association. Similar to the US and CA, the geographical location and economic connections between CN and HK are quite tight. However, the risk spillover level of HK →CN is the highest, while that of CN →HK is much lower. It is obvious that the high level of risk spillover effect from Hong Kong to the mainland China is due to the economic linkage between the two countries and the effect of Shanghai-Hong Kong Stock Connect program. In contrast, the economic policy of the Chinese system is different from that of western systems. The financial institutions in mainland China are not aggressive in investing. Moreover, the trade from mainland China to Hong Kong concentrates on domestic goods, which are at low prices. Those goods are why the risk spillover from CN to HK is relatively low. Therefore, the Hong Kong stock market is more mature and less susceptible to shocks.
In the following, we present a two-way weighted network to analyze systemic risks with a clearer visual structure. First, the adjacency matrix needs to be read via the NetworkX package in Python. Figure 7 shows a network map using the mean value of the samples. The arrow indicates the direction of the risk spillover. Both the size of the arrow and the width of the line segment indicate the intensity of risk spillover. Note that the width of the line segment states the level of the spillover of the larger one in the two-way relationship, in which the thinner one is covered. Therefore, the level of the risk spillover can only be judged based on the arrows in the comparison of two-way relationships. As can be seen, the most prominent line segment in this map is from HK to CN because of the Shanghai-Hong Kong Stock Connect and Shenzhen-Hong Kong Stock Connect programs. The level of risk spillover between the United States and Canada is also high, but the two-way relationship is symmetrical with almost equal size arrows. Similar two-way relationships also exist between TW and KP, JP and KP, ID and PH, and AU and CA. Such two-way relationships can be explained by the frequent trade interactions. It implies that stock markets are not only barometers of the economy but also effective reflections of the economic trades and global value chains through risk spillovers among financial markets.
Figure 8 is a frequency histogram of the risk spillover relationships. Four colors represent four algorithms adopted in calculating the risk spillover. The intensity of risk spillovers can be seen to exhibit a right-skewed spike with a thick tail. This indicates that while most risk spillovers are at low levels, the risk in extreme conditions is substantially outside the average range. In addition, the distribution of the red line is relatively flat, which means the risk spillover may be overestimated by
A 3D-mesh surface is also employed to illustrate the one-way spillover from Hong Kong to mainland China, which is the most significant correlation in the Asia–Pacific region. As can be seen from the result from
Figure 9. Risk spillover from HK to CN and partial indicators. (A)
To compare the overflow dynamic throughout the sample period, the time series diagram is drawn in Figure 10. Four lines in various colors represent the overall overflow levels of the two algorithms at the extreme level or the average level, based on the computational method of
Compared with the composite overflow indicators calculated by the multi-quantile algorithm (in green and gray), the peak of overflow levels in the extreme condition represented by the red and blue lines are relatively higher because the peaks of overflow levels at the multi-quantile are flattened by averaging. Moreover, although spillover instability under extreme quantile conditions is reduced, the fluctuations of overflow calculated by the
Although the fluctuations of each line are different, there are four significant periods with high overall composite overflow levels. The first period is from May 2012 to September 2013, which corresponds to the EU debt crisis and the US stock market crash. The second period is in the second half of 2015 before the Chinese stock market crash occurred. The third period begins in 2018, which corresponds to the Sino-American trade war. The last fluctuant period is from March 2020 to February 2021, which is caused by the outbreak of COVID-19.
In this part, we analyze the average overflow of each market to the systemic
The suffering indicators
Although Figure 11 shows differences in index calculations under the four algorithms, the comprehensive index is smaller than that calculated by a single sub-site. Whether under single or multi-component sites, the exponents obtained by the
To improve the traditional paradigm of risk spillovers among financial markets, this paper has calculated a multi-quantile
First, by visualizing the partial
Second, the overestimation of spillover may occur when calculated by
Third, the stock market in mainland China is highly exposed to the risk spillovers from the Hong Kong stock market. In addition to the short geographical distance between them, another reason for this relatively asymmetric risk spillover may be that investors in mainland China are more concerned about the opposite, but investors in Hong Kong are more independent and have more complete information. In addition, the mutual spillovers between the United States and Canada are also significant, which may be due to the special geographic relationship between the United States and Canada as well as tight trade cooperation and economic dependency between two markets. Cross-market comparisons show that the model supports the traditional view that Hong Kong, Canada, United States, and Singapore are more important markets in the Asia–Pacific region. In contrast, the Chinese mainland and Japanese markets received the most spillovers during the sample period.
This paper studies the systemic risk and risk spillover under multiple quantiles, providing a reference for stock investment and risk regulation in the Asia–Pacific market. This method can not only be applied to the study of inter-institutional risk spillover but can also be helpful in capturing the nonlinear characteristics of individuals’ systemic risk. However, this paper still has some shortcomings. Limited by the time and space complexity of the algorithms, it is impossible to use the rolling window to estimate and calculate the daily samples. Therefore, the out-of-sample prediction effect of the model cannot be investigated. Further study is needed to improve the efficiency of the model and expand the sample.
The original contributions presented in the study are included in the article/Supplementary Material; further inquiries can be directed to the corresponding author.
CR: conceptualization, investigation, methodology, software, supervision, writing–original draft, and writing–review and editing. ZZ: conceptualization, formal analysis, investigation, resources, and writing–original draft. DZ: formal analysis, investigation, resources, and writing–original draft.
The author(s) declare that financial support was received for the research, authorship, and/or publication of this article. This research is funded by the National Nature Science Foundation of China (NSFC), grant number 72173018; the Humanity and Social Science Research Project of Anhui Educational Committee, grant number 2024AH052470; and the High-level Talent Research Initiation Project at Anhui Business College, grant number 2024KYQD05.
The authors declare that the research was conducted in the absence of any commercial or financial relationships that could be construed as a potential conflict of interest.
All claims expressed in this article are solely those of the authors and do not necessarily represent those of their affiliated organizations, or those of the publisher, the editors, and the reviewers. Any product that may be evaluated in this article, or claim that may be made by its manufacturer, is not guaranteed or endorsed by the publisher.
The Supplementary Material for this article can be found online at: https://www.frontiersin.org/articles/10.3389/fphy.2024.1484589/full#supplementary-material
1“Higher risk but less loss” means the greater the risk, the smaller the loss because of the quantile crossing.
2
3
4
5MQ-CAViaR charts for other stock markets are shown in Supplementary Appendix Figure SA1.
6Supplementary Appendix Figure SA2 presents the QRNN-QQ-CoVaR 3D-surface plots for other market indexes.
7Supplementary Appendix Figure SA3 represents the stand-alone
8In Section 3.4, we calculate the average values for
1. Allen F, Carletti E. What is systemic risk? J Money, Credit Banking (2013) 45:121–7. doi:10.1111/jmcb.12038
3. Acemoglu D, Carvalho VM, Ozdaglar A, Tahbaz-Salehi A. The network origins of aggregate fluctuations. Econometrica (2012) 80:1977–2016. doi:10.3982/ECTA9623
4. Acemoglu D, Ozdaglar A, Tahbaz-Salehi A. Systemic risk and stability in financial networks. Am Econ Rev (2015) 105:564–608. doi:10.1257/aer.20130456
5. Elliott M, Golub B, Jackson MO. Financial networks and contagion. Am Econ Rev (2014) 104:3115–53. doi:10.1257/aer.104.10.3115
6. Greenwood R, Landier A, Thesmar D. Vulnerable banks. J Financial Econ (2015) 115:471–85. doi:10.1016/j.jfineco.2014.11.006
7. Engle R. Dynamic conditional correlation: a simple class of multivariate generalized autoregressive conditional heteroskedasticity models. J Business and Econ Stat (2002) 20:339–50. doi:10.1198/073500102288618487
8. Rodriguez JC. Measuring financial contagion: a Copula approach. J Empirical Finance (2007) 14:401–23. doi:10.1016/j.jempfin.2006.07.002
9. Billio M, Getmansky M, Andrew WL, Pelizzon L. Econometric measures of connectedness and systemic risk in the finance and insurance sectors. J Financial Econ (2012) 104:535–59. doi:10.1016/j.jfineco.2011.12.010
10. Diebold FX, Yilmaz K. On the network topology of variance decompositions: measuring the connectedness of financial firms. J Econom (2014) 182:119–34. doi:10.1016/j.jeconom.2014.04.012
11. Baruník J, Křehlík T. Measuring the frequency dynamics of financial connectedness and systemic risk. J Financial Econom (2018) 16:271–96. doi:10.1093/jjfinec/nby001
13. Hautsch N, Schaumburg J, Schienle M. Financial network systemic risk contributions. Rev Finance (2015) 19:685–738. doi:10.1093/rof/rfu010
14. Fan Y, Härdle WK, Wang W, Zhu L. Single-index-based CoVaR with very high-dimensional covariates. J Business and Econ Stat (2018) 36:212–26. doi:10.1080/07350015.2016.1180990 (Accessed July 17, 2024).
15. Härdle WK, Wang W, Yu L. TENET: tail-Event driven NETwork risk. J Econom (2016) 192:499–513. doi:10.1016/j.jeconom.2016.02.013
16. Keilbar G, Wang W. Modelling systemic risk using neural network quantile regression. Empir Econ (2022) 62:93–118. doi:10.1007/s00181-021-02035-1
17. Naeem MA, Karim S, Tiwari AK. Quantifying systemic risk in US industries using neural network quantile regression. Res Int Business Finance (2022) 61:101648. doi:10.1016/j.ribaf.2022.101648
18. Anwer Z, Khan A, Naeem MA, Tiwari AK. Modelling systemic risk of energy and non-energy commodity markets during the COVID-19 pandemic. Ann Oper Res (2022) 1–35. doi:10.1007/s10479-022-04879-x
19. Xiao C, Xu X, Lei Y, Zhang K, Liu S, Zhou F. Counterfactual graph learning for anomaly detection on attributed networks. IEEE Trans Knowledge Data Eng (2023) 35:10540–53. doi:10.1109/TKDE.2023.3250523
20. Li H-J, Feng Y, Xia C, Cao J. Overlapping graph clustering in attributed networks via generalized cluster potential game. ACM Trans Knowl Discov Data (2023) 18(27):1–26. doi:10.1145/3597436
21. Bekiros S, Shahzad SJH, Arreola-Hernandez J, Ur Rehman M. Directional predictability and time-varying spillovers between stock markets and economic cycles. Econ Model (2018) 69:301–12. doi:10.1016/j.econmod.2017.10.003
22. Dong Z, Li Y, Zhuang X, Wang J. Impacts of COVID-19 on global stock sectors: evidence from time-varying connectedness and asymmetric nexus analysis. The North Am J Econ Finance (2022) 62:101753. doi:10.1016/j.najef.2022.101753
23. Ren Y, Tan A, Zhu H, Zhao W. Does economic policy uncertainty drive nonlinear risk spillover in the commodity futures market? Int Rev Financial Anal (2022) 81:102084. doi:10.1016/j.irfa.2022.102084
24. El Adlouni S, Baldé I. Bayesian non-crossing quantile regression for regularly varying distributions. J Stat Comput Simulation (2019) 89:884–98. doi:10.1080/00949655.2019.1573899
25. Huang Y. Restoration of monotonicity respecting in dynamic regression. J Am Stat Assoc (2017) 112:613–22. doi:10.1080/01621459.2016.1149070
26. Allen F, Carletti E. What is systemic risk? J Money, Credit Banking (2013) 45:121–7. doi:10.1111/jmcb.12038
27. Acharya V, Pedersen L, Philippon T, Richardson M. Measuring systemic risk. The Rev Financial Stud (2017) 30:2–47. doi:10.1093/rfs/hhw088
28. Catania L, Luati A. Semiparametric modeling of multiple quantiles. J Econom (2023) 237:105365. doi:10.1016/j.jeconom.2022.11.002
29. Li Y, Luo J, Jiang Y. Policy uncertainty spillovers and financial risk contagion in the Asia-Pacific network. Pacific-Basin Finance J (2021) 67:101554. doi:10.1016/j.pacfin.2021.101554
30. Lee K-J, Lu S-L, Shih Y. Contagion effect of natural disaster and financial crisis events on international stock markets. J Risk Financial Management (2018) 11:16. doi:10.3390/jrfm11020016
31. Pavlova I, de Boyrie ME. Carry trades and sovereign CDS spreads: evidence from asia-pacific markets. J Futures Markets (2015) 35:1067–87. doi:10.1002/fut.21694
33. Taylor JW. A quantile regression neural network approach to estimating the conditional density of multiperiod returns. J Forecast (2000) 19:299–311. doi:10.1002/1099-131X(200007)19:4<299::AID-FOR775>3.0.CO;2-V
34. Cannon AJ. Non-crossing nonlinear regression quantiles by monotone composite quantile regression neural network, with application to rainfall extremes. Stoch Environ Res Risk Assess (2018) 32:3207–25. doi:10.1007/s00477-018-1573-6
35. Chen X, Shen X. Sieve extremum estimates for weakly dependent data. Econometrica (1998) 66:289–314. doi:10.2307/2998559
36. Chen X, White H. Improved rates and asymptotic normality for nonparametric neural network estimators. IEEE Trans Inf Theor (1999) 45:682–91. doi:10.1109/18.749011
37. Huber PJ. Robust statistics. In: Robust statistics. John Wiley and Sons, Ltd (2024) 297–305. doi:10.1002/9780470434697.ch13
38. Bishop CM. The multi-layer perceptron. In: CM Bishop, Editor. Neural networks for pattern recognition. Oxford University Press (2024) 0. doi:10.1093/oso/9780198538493.003.0004
39. Cannon AJ. Quantile regression neural networks: implementation in R and application to precipitation downscaling. Comput and Geosciences (2011) 37:1277–84. doi:10.1016/j.cageo.2010.07.005
40. Cannon AJ. Non-crossing nonlinear regression quantiles by monotone composite quantile regression neural network, with application to rainfall extremes. Stoch Environ Res Risk Assess (2018) 32:3207–25. doi:10.1007/s00477-018-1573-6
41. Engle RF, Manganelli S. CAViaR: conditional autoregressive value at risk by regression quantiles. J Business and Econ Stat (2004) 22:367–81. doi:10.1198/073500104000000370
42. Buczyński M, Chlebus M. Is CAViaR model really so good in Value at Risk forecasting? Evidence from evaluation of a quality of Value-at-Risk forecasts obtained based on the: GARCH(1,1), GARCH-t(1,1), GARCH-st(1,1), QML-GARCH(1,1), CAViaR and the historical simulation models depending on the stability of financial markets. Faculty of Economic Sciences, University of Warsaw (2017). Available from: https://econpapers.repec.org/paper/warwpaper/2017-29.htm (Accessed August 2, 2024).
43. Mullen KM, Ardia D, Gil DL, Windover D, Cline J. DEoptim: an R package for global optimization by differential evolution. J Stat Softw (2011) 40:1–26. doi:10.18637/jss.v040.i06
44. Härdle WK, Wang W, Yu L. TENET: tail-Event driven NETwork risk. J Econom (2016) 192:499–513. doi:10.1016/j.jeconom.2016.02.013
Keywords: multiple quantile risk spillover, MCQRNN, QQ-CoVaR, systemic financial risk, quantile crossing
Citation: Ren C, Zhu Z and Zhou D (2024) Multi-quantile systemic financial risk based on a monotone composite quantile regression neural network. Front. Phys. 12:1484589. doi: 10.3389/fphy.2024.1484589
Received: 24 August 2024; Accepted: 09 October 2024;
Published: 18 November 2024.
Edited by:
Hui-Jia Li, Nankai University, ChinaReviewed by:
Peican Zhu, Northwestern Polytechnical University, ChinaCopyright © 2024 Ren, Zhu and Zhou. This is an open-access article distributed under the terms of the Creative Commons Attribution License (CC BY). The use, distribution or reproduction in other forums is permitted, provided the original author(s) and the copyright owner(s) are credited and that the original publication in this journal is cited, in accordance with accepted academic practice. No use, distribution or reproduction is permitted which does not comply with these terms.
*Correspondence: Chao Ren, Y3JlbjEyMDJAaG90bWFpbC5jb20=
†ORCID ID: Ziyan Zhu, orcid.org/ 0000-0002-2658-9867; Donghai Zhou, orcid.org/ 0000-0001-9160-8713
Disclaimer: All claims expressed in this article are solely those of the authors and do not necessarily represent those of their affiliated organizations, or those of the publisher, the editors and the reviewers. Any product that may be evaluated in this article or claim that may be made by its manufacturer is not guaranteed or endorsed by the publisher.
Research integrity at Frontiers
Learn more about the work of our research integrity team to safeguard the quality of each article we publish.