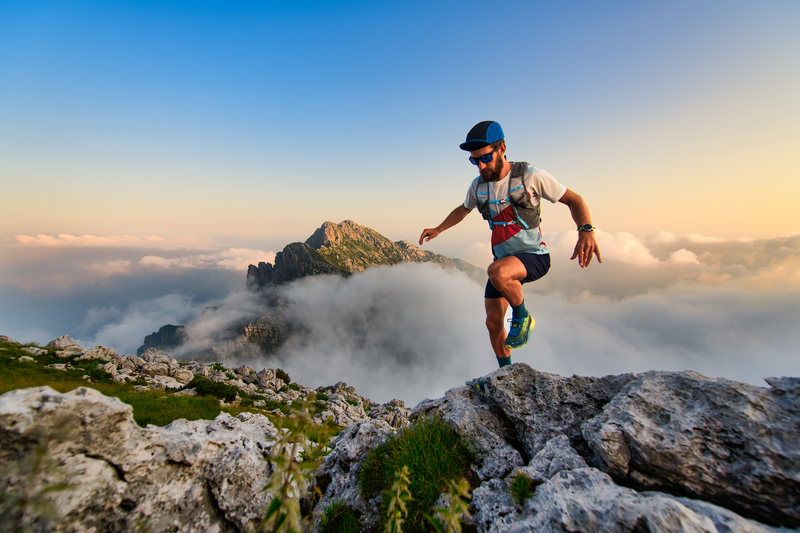
94% of researchers rate our articles as excellent or good
Learn more about the work of our research integrity team to safeguard the quality of each article we publish.
Find out more
ORIGINAL RESEARCH article
Front. Phys. , 25 October 2024
Sec. Condensed Matter Physics
Volume 12 - 2024 | https://doi.org/10.3389/fphy.2024.1431810
This article is part of the Research Topic Current Research On Spin Glasses View all 11 articles
We introduce a tensor network algorithm for the solution of p-spin models. We show that bond compression through rank-revealing decompositions performed during the tensor network contraction resolves logical redundancies in the system exactly and is thus lossless, yet leads to qualitative changes in runtime scaling in different regimes of the model. First, we find that bond compression emulates the so-called leaf-removal algorithm, solving the problem efficiently in the “easy” phase. Past a dynamical phase transition, we observe superpolynomial runtimes, reflecting the appearance of a core component. We then develop a graphical method to study the scaling of contraction for a minimal ensemble of core-only instances. We find subexponential scaling, improving on the exponential scaling that occurs without compression. Our results suggest that our tensor network algorithm subsumes the classical leaf removal algorithm and simplifies redundancies in the p-spin model through lossless compression, all without explicit knowledge of the problem’s structure.
Spin glass physics appears in disciplines far-removed from its origin in condensed matter, including theoretical computer science [1], biology [2], and machine learning [3]. Spin glass models are generally easy to describe, yet hard to solve. One reason is that such models exhibit rugged energy landscapes [4], trapping optimization algorithms in local minima and leading to exponentially long run times.
A notable counterexample is the
In this work, we introduce a tensor network algorithm for solving
Here, we show that compressed TN contraction applied to the
We can write the
where
By letting
where
In its most general form, a SAT problem is the problem of deciding whether a logic formula built from a set of boolean variables
The constraint stipulating that every clause must consist of exactly
The variant of the #
The #
where
When one generates
Given a
where all operations are modulo 2, as in applying GE. #
In Ref. [34], the authors studied the time and memory requirements for solving Equation 2 for
The authors also presented a “smart” version of GE, where one first looks for the variable appearing in the least number of equations left to be solved (ties broken arbitrarily), then solves for that variable and substitutes it into the remaining equations. They argued that this smarter version of GE will solve the problem in
When one solves an equation that contains a variable which only appears in that equation, one can interpret the process graphically as a “leaf removal” algorithm [5]. We describe it below because it provides intuition as to why the “smart” version of GE is more efficient and will help explain the behaviour of our TN algorithm.
Suppose we have an instance for
This algorithm is called leaf removal [5], and it allows us to simplify the
In Ref. [5], the authors showed that, for the ensemble where
We also note that when no core remains at the end of leaf removal, one can interpret the algorithm as finding a permutation of the rows and columns of the matrix
In the case of the
There exist graphical rules, such as the leaf removal explained in Section 2.2.3, that let us simplify a
The first example is the Hopf law [35], where a clause involves the same variable multiple times. In this case, since
Figure 1. Graphical representation of the Hopf law. Clause nodes are blue squares, and variable nodes are green circles.
The second example is the bialgebra law [35], where a set of clause nodes are all connected to a set of variable nodes. An example for two clauses and two variables is shown in Figure 2. These structures simplify to a single clause and single variable, as shown in Figure 2.
Figure 2. Graphical representation of the bialgebra law. Clause nodes are blue squares, and variable nodes are green circles.
These simplifications correspond to eliminating redundancies in the problem. Resolving these redundancies can be exploited to solve the problem faster.
TNs are a data structure that encodes a list of tensor multiplications. Intuitively, one can imagine a TN as a graph where each node represents a tensor, and edges represent the common axes along which one multiplies two tensors1. By contracting together neighboring nodes—multiplying the corresponding tensors together—one can sometimes efficiently compute a variety of quantities, making it a useful numerical method. Originally developed to efficiently evaluate quantum expectation values and partition functions of many-body systems, this tool now has applicability in many domains, including quantum circuit simulation [36] and machine learning [37]. As shown in [22], this tool can also be used for
For our work, contracting all of the tensors in the network together will yield the number of solutions to Equation 2. Below, we review the main ideas for TN methods that are relevant for us and determine the performance of our algorithm. These elements are: how to perform contractions, the importance of contraction ordering, and how to locally optimize the sizes of the tensors (which affect the memory requirements). We then describe our TN algorithm for the #
A single tensor is a multidimensional array of values. Graphically, the number of axes (or rank) of the tensor is the degree of the corresponding node, and the size of the tensor is the number of elements (the product of the dimensions of the axes). The size of the TN is then the sum of all the tensor sizes. For any TN algorithm, one must keep track of the size of the TN to ensure the memory requirements do not exceed one’s computational limits. In particular, one must consider how contracting tensors together changes the TN’s size.
A simple example of contraction is the matrix-vector multiplication, which is represented graphically in Figure 3.Here, the vector
In general, one can write the contraction of a TN by this summation over all the common (shared) axes. We will sometimes call tensors with common axes adjacent, in reference to a TN’s graphical depiction.
When contracting tensors where each axis has the same dimension, we can graphically determine the resulting size by looking at the degree of the new node. In Figure 3, the resulting tensor has rank 1, which is the same as
Though we can carry out the contraction of a TN in any order, the size of the TN in intermediate steps of the contraction can vary widely. Ideally, a contraction will choose an order that limits the memory required to store the TN during all steps of the contraction, making it feasible. Given a contraction order, we can define the contraction width
For the graphical representation,
Bond compression involves, in its simplest form, performing a contraction-decomposition operation on adjacent tensors within the TN. The term “bond” refers to the common index between tensors. The decomposition step primarily uses rank-revealing methods such as QR or singular value decomposition (SVD). Of these, the SVD plays a central role in TN algorithms. By setting a threshold value for singular values, either absolute or relative, we retain only the singular values above the threshold and corresponding singular vectors, thereby approximating subsequent contractions. This approach facilitates the contraction of larger TNs by reducing the contraction width during the process. However, in general, this comes at the expense of approximating the final result.
We implement bond compression as follows. Given two adjacent tensors
The first equality comes after applying a QR decomposition to the tensors. Since the QR decomposition operates solely on matrices, we first need to reshape those tensors into matrices before decomposing them. Concretely, if we have a tensor
As shown in Ref. [22], we can map any
where the indices
where the indices are also boolean,
As explained in Section 2.3.2, we can evaluate the contraction width
There are several possible simplifications for a
We will use bond compression to contract and decompose all adjacent tensors in the TN, a process commonly called a sweep, which is standard practice in TN methods. However, we will not remove any nonzero singular values in the decomposition. If the tensors are full-rank, this is useless; the tensors remain unchanged after performing bond compression. On the other hand, TNs representing
An interesting fact with this method is that applying bond compression to the bond between a rank-1 variable tensor and a rank-
Figure 5. Applying bond compression on a rank-1 variable tensor (green circular node labelled
The contraction width will be the figure of merit for the performance of this algorithm because of its relation with the maximum intermediate tensor size (see Equation 6).
When
To bypass this bottleneck and provide further scaling evidence, we develop a graphical algorithm that allows us to study the contraction width throughout a contraction by only studying the connectivity of the instance’s graph. As discussed in Section 2.3.1, this is always possible for any exact contraction of a TN, since one simply needs to keep track of the tensor ranks at each step of the contraction (regardless of the tensors’ contents). However, because we seek to study the performance of our TN algorithm that detects simplifications through bond compression, we must also encode the graphical patterns that will lead to simplifications. We will make use of the graphical simplifications discussed in Section 2.2.4, as well as more discussed in Section 3 of Ref. [35].
The graphical algorithm works as follows. Starting from a graph
The rank of an intermediate tensor is the number of outgoing edges from a cluster, and its size is:
Taking the maximum number of outgoing edges over all contraction steps and clusters directly yields the contraction width.
We now interpret the sweeping method as implementing graphical simplifications. Recall that the TN contraction is a sum over all the boolean indices of the tensors and only the indices which satisfy the logic of the TN will contribute 1 to the sum (and 0 otherwise), yielding the solution count to the problem. Therefore, any simplifications from bond compression must correspond to redundancy in specifying the logic of the TN. Suppose the algorithm is compressing the bonds between tensors
For example, in the leaf removal algorithm, compressing the bond of a rank-1 variable tensor
The algorithm must detect and simplify any tensor that our TN algorithm would simplify. For the (2,3)-biregular graph ensemble (
The fusion rule says that neighboring clause nodes in the same cluster can be contracted together to form a bigger clause node, and the same is true for variable nodes. In this case, we actually replace the two nodes with a single node representing them. Their corresponding tensor representations would then be exactly those of a clause or variable tensor of larger rank. This rule is schematically shown in Figure 6. One can also apply the same rule for nodes of the same type which share multiple edges. However, for clause nodes, there will be an overall numerical factor of
Figure 6. The fusion rule on two nodes that are in the same cluster, identified as red here. Nodes of diamond shape represent nodes that could be either of type clause or of type variable.
The generalized Hopf law ensures that if a clause node and a variable node share
The triangle simplification is an implementation of the Hopf law between two clusters that, between them, contain a “triangle” of nodes. Those triangles contain two nodes of one type (clause or variable) and one of the other. Because we always contract nodes of the same type within a cluster using the fusion rule, a triangle simplification can only occur when the nodes of the same type are in different clusters. When we sweep between these clusters, applying the fusion rule and then a basic Hopf law will remove edges, as shown in Figure 7.
Figure 7. One of the two possible cases of the triangle simplification. Node
The simplification of multiple edges between nodes of the same type is a variant of the fusion rule. Consider the example in Figure 8. If the nodes are in different clusters, sweeping would not contract the nodes, but would simplify all the edges except one in the same way as a the fusion rule (ignoring once again an overall factor).
Figure 8. The multiple edges between nodes of the same type simplification. The nodes are in different clusters (yellow and red), and initially share multiple edges. After a sweep, only one edge is needed to represent the same tensor structure.
Finally, the scalar decomposition occurs when there are two nodes of the same type and at least one shares all its edges with the other. A sweep will merge the two nodes, and then only factor out a scalar (degree-0 node) in the decomposition to return to two tensors. However, the sweep will remove all edges between the tensors.
We now argue that these simplifications are sufficient to characterize any possible simplification present in the (2,3)-biregular graph ensemble. Each variable node has degree 2, so the bialgebra law and any higher-order generalizations cannot occur because they require variable nodes of degree at least 3. Because we replace any degree-2 variable node in a cluster by an edge and the fusion rule combines clause nodes within a cluster, most clusters will be a single clause node of some degree. Our rules above capture simplifications between such clusters. The one exception is that variable nodes are their own clusters at the start of the algorithm before being contracted with other nodes. In this case, the simplifications given by Figure 7 may apply. Therefore, our set of graphical rules should be sufficient to capture all possible simplifications in this ensemble. We also provide evidence of this claim in Section 4.2.
To generate our instances at a given
This rank is defined as the number of times that a variable is present in the problem. In the language of Equation 2, we randomly place
Since we are mainly concerned with the scaling of resources for instances which contain a core, we choose a minimal ensemble with this property. We will study the ensemble of connected 3-regular graphs on
For TN contractions, we use quimb, a Python package for manipulating TNs [40]. For the graphical method, we use igraph, an efficient network analysis library [41], in order to work with node attributes on the graph directly. Those attributes let us define the node types (clause and variable) and the nodes’ clusters.
The TN contraction order, as discussed in Section 2.3.2, determines the contraction width. Without applying our sweeping method, one can track this quantity without actually performing the tensor contraction. One must simply keep track of the ranks of the tensors at any point in the contraction, noting as in Section 2.3.1 that combining two tensors yields a new tensor of known rank. We use cotengra, a Python package for TN contractions, to track this quantity [38]. In order to track this quantity when sweeps are applied, we use quimb in order to read the tensors’ sizes during the contraction and calculate the contraction width using Equation 6.
For random TNs such as ours, there exist multiple heuristic algorithms for finding contraction orderings [30, 38] which lower the contraction width and are practically useful for carrying out computations. For the results in Section 4, we determine the ordering using a community detection algorithm based on the edge betweenness centrality [42] (EBC) of the network. This algorithm is implemented as community_edge_betweenness in the Python package igraph [41]. We use the EBC algorithm because it looks for communities in the graph, thus contracting dense sections first. This is useful in random TNs because it minimizes the chances of having to work with huge tensors quickly, which could result in a tensor of large rank (and therefore, large contraction width). This algorithm is also deterministic, ensuring reproducibility of the contraction orderings. Furthermore, in Section 4.2, we compare the results obtained using this contraction ordering with two others: KaHyPar [43, 44] and greedy, both from the Python package cotengra.
Even with these better contraction orderings, exactly contracting these random TNs without bond compression will generally result in an exponential growth in
To ensure lossless compression in bond sweeping, we set the relative threshold for zero singular values to be
Numerical TN contractions were performed on an AMD EPYC 7F72 @ 3.2 GHz processor, with a maximum allocated RAM of 1 TB. Each point in the figures of this section corresponds to the median contraction width or contraction runtime over
In Figure 9, we show the average contraction width with and without compression (sweeping) for
We studied larger values of
Figure 10. Scaling of the contraction width and runtime of compressed TN contraction for the 3-spin model. (A) The median contraction width. (B) The same data as in (A), but on a logarithmic horizontal scale to accentuate the curves which follow a logarithmic scale (which can be fitted with straight lines). (C) Our algorithm’s compressed contraction median runtime. This panel shows the exponential scaling by straight lines. (D) The same data as in (C), but shown on a horizontal logarithmic scale to accentuate the curves which follow polynomial scaling (straight lines).
The results in Figure 10A highlight linear scaling of the curves for
The logarithmic scaling for
In Figure 10C, we see the scaling of the median contraction runtime (in seconds) with a logarithmic vertical axis and the same data is shown with a logarithmic horizontal axis in Figure 10D. Accordingly with the contraction width scaling, we find polynomial curves for
At
For the leaf-free ensemble, each point in the figures has been averaged over 200 random leaf-free instances. With the graphical method, the contraction widths are extracted from the number of clusters’ outgoing edges during the TN contraction, as explained in Section 3.3 (see Equation 10). All the results for the contraction width obtained with this graphical method are shown in Figures 11, 12.
Figure 11. (A) Scaling of the average contraction width for instances in the (2,3)-biregular graph ensemble (
Figure 12. All the results were obtained using graphical contractions. (A) Scaling of the average contraction width for instances in the (2,3)-biregular graph ensemble (
Now having the possibility to study larger TNs without being limited by the memory, we can compare the contraction width of the algorithm on different contraction orderings. In Figure 11A, we compare two of them: EBC and Random. The Random method chooses the next tensors to be contracted completely randomly. It can thus only be usefully studied with this graphical method because it quickly scales to astronomical contraction widths, as seen in Figure 11A.
From Figure 11A, we see that a good contraction ordering is an important factor for the success of the sweeping method during the contraction of a given TN that models a
The results demonstrate that the sweeping method finds enough simplifications for instances in the (2,3)-biregular graph ensemble so that the scaling of the average contraction width changes from linear to sublinear for the EBC, KaHyPar and greedy contraction orderings. From those results, after
Note that the (2,3)-biregular graph ensemble we consider offers a simplification of the corresponding
We have verified that the graphical contraction method of Section 3.3 yields tensor sizes identical to those found via numerical contraction at each contraction step by comparing the two methods for 100 random instances with
In this work, we have applied compressed TN contraction to the
The raw data supporting the conclusions of this article will be made available by the authors, without undue reservation.
BL: Conceptualization, Data curation, Formal Analysis, Investigation, Methodology, Software, Validation, Visualization, Writing–original draft, Writing–review and editing. JC: Conceptualization, Formal Analysis, Investigation, Methodology, Supervision, Validation, Writing–original draft, Writing–review and editing. SK: Conceptualization, Formal Analysis, Funding acquisition, Methodology, Project administration, Resources, Software, Supervision, Writing–original draft, Writing–review and editing.
The author(s) declare that financial support was received for the research, authorship, and/or publication of this article. This work was supported by the Ministère de l’Économie, de l’Innovation et de l’Énergie du Québec through its Research Chair in Quantum Computing, an NSERC Discovery grant, and the Canada First Research Excellence Fund. This work made use of the compute infrastructure of Calcul Québec and the Digital Research Alliance of Canada.
The authors declare that the research was conducted in the absence of any commercial or financial relationships that could be construed as a potential conflict of interest.
All claims expressed in this article are solely those of the authors and do not necessarily represent those of their affiliated organizations, or those of the publisher, the editors and the reviewers. Any product that may be evaluated in this article, or claim that may be made by its manufacturer, is not guaranteed or endorsed by the publisher.
1Though this does not factor into our work, it is also possible to have “free” edges with only one end connected to a node, indicating an axis in which no tensor multiplication occurs.
2The algorithm can also remove edges within a cluster, if it is part of the simplification (see Figure 7).
3Note that we choose
1. Kirkpatrick S, Toulouse G. Configuration space analysis of travelling salesman problems. J Phys France (1985) 46:1277–92. doi:10.1051/jphys:019850046080127700
2. Bryngelson JD, Wolynes PG. Spin glasses and the statistical mechanics of protein folding. Proc Natl Acad Sci (1987) 84:7524–8. doi:10.1073/pnas.84.21.7524
3. Venkataraman G, Athithan G. Spin glass, the travelling salesman problem, neural networks and all that. Pramana (1991) 36:1–77. doi:10.1007/BF02846491
4. Stein DL, Newman CM. Spin glasses and complexity. Princeton University Press (2013). doi:10.23943/princeton/9780691147338.001.0001
5. Mézard M, Ricci-Tersenghi F, Zecchina R. Two solutions to diluted p-spin models and XORSAT problems. J Stat Phys (2003) 111:505–33. doi:10.1023/A:1022886412117
6. Ricci-Tersenghi F. Being glassy without being hard to solve. Science (2010) 330:1639–40. doi:10.1126/science.1189804
7. Bernaschi M, Bisson M, Fatica M, Marinari E, Martin-Mayor V, Parisi G, et al. How we are leading a 3-xorsat challenge: from the energy landscape to the algorithm and its efficient implementation on gpus(a). Europhysics Lett (2021) 133:60005. doi:10.1209/0295-5075/133/60005
8. Kanao T, Goto H. Simulated bifurcation for higher-order cost functions. Appl Phys Express (2022) 16:014501. doi:10.35848/1882-0786/acaba9
9. Aadit NA, Nikhar S, Kannan S, Chowdhury S, Camsari KY. All-to-all reconfigurability with sparse Ising machines: the XORSAT challenge with p-bits (2023). doi:10.1088/arXiv:2312.08748
10. Jörg T, Krzakala F, Semerjian G, Zamponi F. First-order transitions and the performance of quantum algorithms in random optimization problems. Phys Rev Lett (2010) 104:207206. doi:10.1103/PhysRevLett.104.207206
11. Farhi E, Gosset D, Hen I, Sandvik AW, Shor P, Young AP, et al. Performance of the quantum adiabatic algorithm on random instances of two optimization problems on regular hypergraphs. Phys Rev A (2012) 86:052334. doi:10.1103/PhysRevA.86.052334
12. Hen I. Equation planting: a tool for benchmarking ising machines. Phys Rev Appl (2019) 12:011003. doi:10.1103/PhysRevApplied.12.011003
13. Bellitti M, Ricci-Tersenghi F, Scardicchio A. Entropic barriers as a reason for hardness in both classical and quantum algorithms. Phys Rev Res (2021) 3:043015. doi:10.1103/PhysRevResearch.3.043015
14. Kowalsky M, Albash T, Hen I, Lidar DA. 3-regular three-xorsat planted solutions benchmark of classical and quantum heuristic optimizers. Quan Sci Technology (2022) 7:025008. doi:10.1088/2058-9565/ac4d1b
15. Patil P, Kourtis S, Chamon C, Mucciolo ER, Ruckenstein AE. Obstacles to quantum annealing in a planar embedding of XORSAT. Phys Rev B (2019) 100:054435. doi:10.1103/PhysRevB.100.054435
16. Haanpää H, Järvisalo M, Kaski P, Niemelä I. Hard satisfiable clause sets for benchmarking equivalence reasoning techniques. J Satisfiability, Boolean Model Comput (2006) 2:27–46. doi:10.3233/SAT190015
18. Jia H, Moore C, Selman B. From spin glasses to hard satisfiable formulas. In: HH Hoos, and DG Mitchell, editors. Theory and applications of satisfiability testing. Berlin, Heidelberg: Springer Berlin Heidelberg (2005). p. 199–210.
19. Barthel W, Hartmann AK, Leone M, Ricci-Tersenghi F, Weigt M, Zecchina R. Hiding solutions in random satisfiability problems: a statistical mechanics approach. Phys Rev Lett (2002) 88:188701. doi:10.1103/PhysRevLett.88.188701
20. Ricci-Tersenghi F, Weigt M, Zecchina R. Simplest randomK-satisfiability problem. Phys Rev E (2001) 63:026702. doi:10.1103/PhysRevE.63.026702
21. Guidetti M, Young AP. Complexity of several constraint-satisfaction problems using the heuristic classical algorithm walksat. Phys Rev E (2011) 84:011102. doi:10.1103/PhysRevE.84.011102
23. Biamonte JD, Morton J, Turner J. Tensor network contractions for# sat. J Stat Phys (2015) 160:1389–404. doi:10.1007/s10955-015-1276-z
24. Kourtis S, Chamon C, Mucciolo ER, Ruckenstein AE. Fast counting with tensor networks. Scipost Phys (2019) 7:060. doi:10.21468/SciPostPhys.7.5.060
25. Meichanetzidis K, Kourtis S. Evaluating the jones polynomial with tensor networks. Phys Rev E (2019) 100:033303. doi:10.1103/PhysRevE.100.033303
26. de Beaudrap N, Kissinger A, Meichanetzidis K. Tensor network rewriting strategies for satisfiability and counting. EPTCS (2021) 340:46–59. doi:10.4204/eptcs.340.3
27. Schuch N, Wolf MM, Verstraete F, Cirac JI. Computational complexity of projected entangled pair states. Phys Rev Lett (2007) 98:140506. doi:10.1103/PhysRevLett.98.140506
28. Evenbly G, Vidal G. Tensor network renormalization. Phys Rev Lett (2015) 115:180405. doi:10.1103/PhysRevLett.115.180405
29. Evenbly G. Algorithms for tensor network renormalization. Phys Rev B (2017) 95:045117. doi:10.1103/PhysRevB.95.045117
30. Gray J, Chan GK-L. Hyperoptimized approximate contraction of tensor networks with arbitrary geometry. Phys Rev X (2024) 14:011009. doi:10.1103/PhysRevX.14.011009
31. Alkabetz R, Arad I. Tensor networks contraction and the belief propagation algorithm. Phys Rev Res (2021) 3:023073. doi:10.1103/PhysRevResearch.3.023073
32. Pancotti N, Gray J. One-step replica symmetry breaking in the language of tensor networks. (2023).
33. Garey MR, Johnson DS. Computers and intractability: a guide to the theory of NP-completeness. San Francisco, CA: W. H. Freeman (1979).
34. Braunstein A, Leone M, Ricci-Tersenghi F, Zecchina R. Complexity transitions in global algorithms for sparse linear systems over finite fields. J Phys A: Math Gen (2002) 35:7559–74. doi:10.1088/0305-4470/35/35/301
35. Denny SJ, Biamonte JD, Jaksch D, Clark SR. Algebraically contractible topological tensor network states. J Phys A: Math Theor (2011) 45:015309. doi:10.1088/1751-8113/45/1/015309
36. Seitz P, Medina I, Cruz E, Huang Q, Mendl CB. Simulating quantum circuits using tree tensor networks. Quantum (2023) 7:964. doi:10.22331/q-2023-03-30-964
37. Wang M, Pan Y, Xu Z, Yang X, Li G, Cichocki A. Tensor networks meet neural networks: a survey and future perspectives. arXiv preprint arXiv:2302.09019 (2023). doi:10.48550/arXiv.2302.09019
38. Gray J, Kourtis S. Hyper-optimized tensor network contraction. Quantum (2021) 5:410. doi:10.22331/q-2021-03-15-410
39. Viger F, Latapy M. Efficient and simple generation of random simple connected graphs with prescribed degree sequence. J Complex Networks (2015) 4:15–37. doi:10.1093/comnet/cnv013
40. Gray J. quimb: a python package for quantum information and many-body calculations. J Open Source Softw (2018) 3:819. doi:10.21105/joss.00819
41. Csardi G, Nepusz T. The igraph software package for complex network research. InterJournal Complex Syst (2006) 1695.
42. Girvan M, Newman MEJ. Community structure in social and biological networks. Proc Natl Acad Sci (2002) 99:7821–6. doi:10.1073/pnas.122653799
43. Schlag S, Henne V, Heuer T, Meyerhenke H, Sanders P, Schulz C. (????). ¡italic¿k¡/italic¿-way Hypergraph Partitioning via ¡italic¿n¡/italic¿-Level Recursive Bisection 53–67. doi:10.1137/1.9781611974317.5
44. Akhremtsev Y, Heuer T, Sanders P, Schlag S. Engineering a direct ¡italic¿k¡/italic¿-way Hypergraph Partitioning Algorithm. In: 2017 Proceedings of the Ninteenth Workshop on Algorithm Engineering and Experiments (ALENEX), 28–42 (2017). doi:10.1137/1.9781611974768.3
45. Zhu Z, Katzgraber HG. Do tensor renormalization group methods work for frustrated spin systems? arXiv preprint arXiv:1903.07721 (2019). doi:10.48550/arXiv.1903.07721
46. Fattal D, Cubitt TS, Yamamoto Y, Bravyi S, Chuang IL. Entanglement in the stabilizer formalism. arXiv (2004). doi:10.48550/arXiv.quant-ph/0406168
47. Hamma A, Ionicioiu R, Zanardi P. Bipartite entanglement and entropic boundary law in lattice spin systems. Phys Rev A (2005) 71:022315. doi:10.1103/PhysRevA.71.022315
Keywords: spin glass (theory), tensor network algorithms, disordered magnetic systems, satisfiability (SAT), model counting
Citation: Lanthier B, Côté J and Kourtis S (2024) Tensor networks for
Received: 13 May 2024; Accepted: 03 October 2024;
Published: 25 October 2024.
Edited by:
Federico Ricci-Tersenghi, Sapienza University of Rome, ItalyReviewed by:
Pan Zhang, Chinese Academy of Sciences (CAS), ChinaCopyright © 2024 Lanthier, Côté and Kourtis. This is an open-access article distributed under the terms of the Creative Commons Attribution License (CC BY). The use, distribution or reproduction in other forums is permitted, provided the original author(s) and the copyright owner(s) are credited and that the original publication in this journal is cited, in accordance with accepted academic practice. No use, distribution or reproduction is permitted which does not comply with these terms.
*Correspondence: Stefanos Kourtis, c3RlZmFub3Mua291cnRpc0B1c2hlcmJyb29rZS5jYQ==
Disclaimer: All claims expressed in this article are solely those of the authors and do not necessarily represent those of their affiliated organizations, or those of the publisher, the editors and the reviewers. Any product that may be evaluated in this article or claim that may be made by its manufacturer is not guaranteed or endorsed by the publisher.
Research integrity at Frontiers
Learn more about the work of our research integrity team to safeguard the quality of each article we publish.