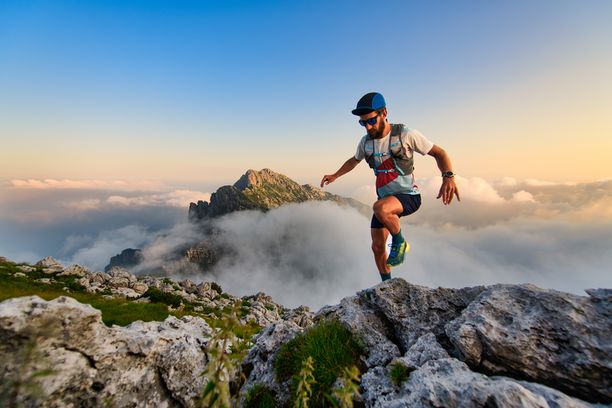
94% of researchers rate our articles as excellent or good
Learn more about the work of our research integrity team to safeguard the quality of each article we publish.
Find out more
REVIEW article
Front. Phys., 08 October 2024
Sec. Space Physics
Volume 12 - 2024 | https://doi.org/10.3389/fphy.2024.1425084
This article is part of the Research TopicFrontiers in Multi-Source Positioning, Navigation and Timing (PNT)View all 8 articles
The threat of spoofing interference has posed a severe challenge to the security application of Global Navigation Satellite System (GNSS). It is particularly urgent and critical to carry out in-depth defense research on spoofing interference. When combined with the inertial navigation system (INS), the GNSS/INS integrated navigation system offers distinct advantages in the field of anti-spoofing technology research, which has garnered significant attention in recent years. To summarize the current research achievements of GNSS/INS integrated navigation anti-spoofing technology, it is necessary to provide an overview of the three core technical aspects of spoofing attack principles and implementation strategies, spoofing detection, and spoofing mitigation. First, the principles and implementation strategies of spoofing interference attacks are introduced, and different classifications of spoofing interference attacks are given. Then, the performance characteristics and technical points of different spoofing detection and spoofing mitigation methods are compared and analyzed, and the shortcomings and challenges in the current development of GNSS/INS anti-spoofing technology are pointed out. Finally, based on the summary and shortcomings of the existing technology, a prospect for the future development of GNSS/INS integrated navigation anti-spoofing technology is discussed.
With the continuous development of the Global Navigation Satellite System (GNSS), more and more military weapons equipment, critical civil facilities, location forensic application and life safety services rely on the high-precision location, velocity and time information provided by GNSS [1–4]. However, due to the weak landing level and open civil signal structure, satellite signals are vulnerable to intentional and unintentional electromagnetic interference during transmission, which makes it a severe challenge to the application of GNSS [5]. Compared with unintentional interference, intentional interference causes more harm to GNSS and mainly includes suppression jamming and spoofing interference [6]. Suppression jamming suppresses the GNSS navigation and positioning services by transmitting high-power noise to cover the satellite signal. There are already many mature anti-jamming technologies [7]. Different from suppression jamming, spoofing interference involves transmitting false satellite signals to target users, leading them to receive inaccurate navigation information. Notably, in December 2011, the Iranian military exploited falsified the Global Positioning System (GPS) signals in a UAV navigation system and successfully trapped a United States stealth reconnaissance drone RQ-170 [8]. Furthermore, between 22 and 24 June 2017, over 20 ships in the Black Sea fell victim to extensive deceptive jamming attacks [9]. The escalation of GPS jamming/spoofing incidents in the Israeli-Palestinian conflict of 2023 underscores the rising trend of such attacks, with spoofing assaults on satellite navigation systems now emblematic of modern warfare. Consequently, research into anti-spoofing technologies for satellite navigation assumes paramount importance in fortifying the security and dependability of GNSS.
Since the 1990s, with the establishment and deployment of GPS, international scholars have initiated research into electronic protection and anti-jamming techniques [10]. Following a comprehensive assessment by the United States Department of Transportation in 2001 [11], which highlighted the vulnerabilities and risks associated with GPS and identified the looming threat of spoofing attacks in satellite navigation, the pursuit of GNSS anti-spoofing technologies gained momentum. Subsequently, scholars have introduced a range of innovative anti-spoofing solutions, encompassing spoofing detection and spoofing mitigation techniques. These anti-spoofing methodologies can be categorized based on distinct technical principles:
In recent years, scholars have focused extensively on the research and development of anti-spoofing technology based on GNSS/INS integrated Navigation System, supported by the Inertial Navigation System (INS). This heightened interest can be attributed to several key advantages of this approach compared to other technologies:
To leverage the anti-spoofing benefits offered by the GNSS/INS integrated navigation system and enhance its resilience against jamming attacks, this paper summarizes GNSS spoofing attacks and anti-spoofing measures. The remaining organization of this paper is as follows: in Section 2, the principal of spoofing attacks is introduced and the spoofing scenario of GNSS is analyzed; in Section 3, anti-spoofing technologies based on GNSS/INS integrated navigation system is described via two types of methods–spoofing detection and spoofing mitigation—and then the development status is introduced and analyzed respectively; in Section 4, the challenges and prospects of anti-spoofing based on GNSS/INS integrated navigation system are summarized. Finally, Section 5 summarizes the above discussion.
Spoofing and anti-spoofing are in a adversarial relationship. A profound comprehension of spoofing is pivotal for effective research in anti-spoofing measures. With the aim to better study anti-spoofing technologies in integrated navigation, it is necessary to elucidate the basic principles, implementation strategies, and classification of deception interference based on available literature.
The fundamental principle underlying spoofing involves the transmission of a deceptive signal by the spoofer, characterized by a slightly amplified power level compared to the authentic navigation signal, directed towards the targeted receiver. This act disrupts the receiver’s ability to accurately capture and track the authentic satellite signal, leading it to erroneously lock onto the false satellite signal instead. Therefore, the spoofer must accurately replicate the carrier, PRN/spread spectrum, data code, and Doppler range of the real navigation signal. The conventional satellite navigation signal as perceived by the receiver can be represented by the expression Equation 1:
where
where
where,
Figure 1. Spoofing attack model. The fake satellites are imaginary. The spoofed signals
The analysis above is based on the level of satellite navigation signals. When spoofing attack is directed towards the target receiver, its effects are most readily discernible at the information layer. Specifically, the influence on the pseudo-range information layer can be effectively modeled with Equation 4. Suppose that the true pseudo-range measurement model of the
where
where
where
Here, when
There are two methods for spoofer to generate spoofing signals in the form of Equation 2, namely generative spoofing attack methods and forwarding spoofing attack methods [22, 23]. These two methods are discussed in detail below.
Generative spoofing attack device directly generates spoofing signals on the premise of known signal pseudo-code and navigation message parameters. Consequently, in the context of Generative spoofing attack, the spoofer can generate deceptive signals independently of the GNSS system. Besides, it is possible for a spoofer to allow for flexible adjustment of various parameters according to their own requirements. However, the implementation of this method entails relatively high costs and complexity. Generative spoofing attacks pose a significant threat to civilian receivers lacking anti-spoofing capabilities. Conversely, for military signals with undisclosed signal structures, the feasibility of generative spoofing attack is limited, thereby restricting its application scope. The general model generative spoofing attack is illustrated in Figure 2.
Figure 2. The schematic of generative spoofing attack. Generative spoofing sources do not need to receive real satellite signals and can directly generate spoofed signals based on known signal structures.
In response to the inability of generative spoofing attack to tackle encrypted navigation signals like military codes, forwarding spoofing attack has emerged. Forwarding spoofing attack involves the deceptive jamming source receiving genuine satellite navigation signals through its own antenna and then, after appropriate delay and power amplification, transmitting them to the target receiver to achieve the spoofing effect. Therefore, a prominent feature of forwarding spoofing attack is that the time delay of the spoofing signal reaching the target receiver must be greater than that of the authentic signal. Obviously, this kind of spoofer do not needs to parse navigation signals but only requires power amplification and time delay. Consequently, compared to generative spoofer, forwarding spoofer has a simpler construction, mainly comprising receiving antennas, amplifiers, and transmitting antennas.
According to the different methods of receiving and processing satellite signals, forwarding spoofing attacks can be divided into two types as shown in Figure 3. The first type spoofer involves a single antenna, which is used to receive all available genuine satellite navigation signals within the area. These signals are then uniformly delayed and power-amplified before being retransmitted using a transmitting antenna. While the second type spoofer involves multi-antenna array, which utilizes lots of high-gain narrow-beam array antennas, with each receiving antenna corresponding to a specific satellite signal within the area. Different delays are applied to the various satellite signals before retransmission. Obviously, the first type of forwarding spoofing attack, due to the uniform delay, is more easily detectable by the receiver. The second type offers higher concealment and can deceive the receiver to a designated location, but it presents greater practical operational difficulty.
Figure 3. The diagram of forwarding spoofing attack, which is drawn with reference to the literature [65]. (A) The forwarding spoofing based on single antenna. (B) The forwarding spoofing based on multi-antenna array.
To sum up, the classification characteristics of spoofing attack based on signal generation mode are summarized in Table 1.
In the spoofing process, once the spoofing source successfully generates spoofing signals, it encounters the challenge of subtly injecting these signals into the tracking loop of the target receiver without detection.
Two strategies are employed to address the challenge: synchronous spoofing and asynchronous spoofing. Synchronous spoofing involves generating false signals that align with the real signal in terms of code phase and Doppler shift. Initially, the power of the spoofing signal is kept low to evade detection before entering the tracking loop. Subsequently, the power gradually increases upon entering the loop, prompting the receiver to lock onto the spoofing signal. The desired spoofing effect is achieved by adjusting the code phase and carrier phase. This strategy facilitates incremental spoofing and is depicted in Figure 4. Synchronous spoofing offers high concealment but presents technical complexities.
Figure 4. The schematic diagram of Synchronous spoofing implementation process.The spoofer captures each receiver channel by aligning the spoofed signal with the true signal from each visible satellite. It starts with low power and then gradually increases the power until it captures the receiver’s tracking loop. Finally the receiver is slowly lured to a false localization result.
On the other hand, asynchronous spoofing disrupts the target receiver by employing high-power interference to cause it to lose lock. Subsequently, spoofing signals are transmitted to allow the target receiver to capture them during reacquisition. Unlike synchronous spoofing, asynchronous spoofing does not require the interference source to generate false signals mirroring the real signal in code phase and Doppler shift. While asynchronous spoofing incurs lower technical costs, it lacks effective concealment compared to synchronous spoofing.
From the perspective of the published literature, research on anti-spoofing technology for GNSS/INS-based integrated navigation systems primarily focuses on two key areas: spoofing detection and spoofing mitigation. Spoofing detection aims to identify the presence of spoofing interference, while spoofing mitigation works to mitigate or eliminate the impact of spoofing interference. According to the difference of processing layers, spoofing detection technology for satellite navigation systems can be categorized into signal layer-based and information layer-based approaches. Currently, the predominant focus in the research area is on enhancing GNSS resilience against spoofing at the information layer by leveraging auxiliary data provided by the INS. There is comparatively less emphasis on research related to anti-spoofing efforts at the signal layer.
Spoofing detection is to determine whether there is a spoofing signal in the signal from the receiver. In addition to realizing the goal of detecting the spoofed signal, spoofing detection also hopes to achieve high detection accuracy and short detection time through algorithm design and setting the appropriate test statistics, with the purpose of reducing the effect of spoofed signals on the navigation system during the detection process. Based on existing literature, the spoofing detection algorithms based on the combined GNSS/INS navigation system can be further categorized according to the different test statistics: detection algorithms based on the measured values, detection algorithms based on the filtered innovation, and other spoofing detection algorithms.
The system measurement value refers to the direct measurement information resolved by the integrated navigation system and its subsystems such as position, velocity, acceleration, attitude, etc. Residual consistency detection method, which detect spoofing by utilizing the high positioning accuracy in a short period of time and independent characteristics of INS, is a typical example of this type of algorithm, e.g., position/velocity based residual consistency detection. The detection domain of literature [24] is position, and literature [25] investigates vehicle speed based spoofing detection. Figure 5 is the flow of the position/velocity consistency detection algorithm referring to [26].
Figure 5. The flow chart of position or velocity consistency detection algorithm. The consistency detection algorithm achieves spoofing detection by comparing the solved data from GNSS with the solved data from INS.
In addition, literature [27] describes a method for detecting GNSS spoofing signals using accelerometers. The method performs spoofing detection by comparing the acceleration estimated from the GNSS output with the acceleration output from the INS accelerometer. Literature [28] improves the detection performance by using both the residual acceleration and the north (or east) accelerometer error component as decision variables. Literature [29] detects the spoofing using pseudo-range rate, through comparing the constructed pseudo-range rate from INS and the pseudo-range rate solved by GNSS. Different from the pseudo-range detection, the pseudo-range rate detection is more sensitive to the slowly varying spoofing interference. For scenarios of spoofed attacks on selected satellites, literature [30] takes advantage of GNSS/INS tightly coupled integration that its navigation solving is possible even with only one visible satellite for spoofing detection. The traversal method is adopted to solve all visible satellites one by one, and then the results are compared with the receiver clock difference/clock drift equivalent distance deviation to detect spoofing. By this method, the influence of spoofed stars can be eliminated to ensure the positioning accuracy of the combination navigation system.
In the case of airborne vehicles, attitude can also play a role in spoofing detection. [31] conducted experimental tests using UAV platforms and discovered that spoofing attacks significantly impact pitch and roll angles, while minimally affecting heading angle. Additionally, [32] employed carrier phase double-difference observables for spoofing signal detection and integrated this with attitude data from the INS to successfully identify and counter forward spoofing interference.
However, the above-mentioned spoofing detection methods, focusing on a single dimension, may only address specific spoofing interferences and are susceptible to failure when attackers alter their tactics. By expanding the dimensionality of comparison information, these limitations can be overcome while enhancing detection performance. For instance, [33] employed a short-term pure inertial error propagation model to utilize position and velocity data from inertial guidance for predicting and estimating the guard’s pseudo-range and pseudo-range rate. They integrated actual system measurements to create pseudo-range and pseudo-range rate time series and conducted spoofing detection by parameter fitting of these time series. In another study referenced as [34], the impact of spoofing attacks on the navigation receiver’s time was leveraged, incorporating a consistent spoofing detection model in the time dimension alongside the position dimension.
Taken together, this type of detection algorithm is simple in principle and the test statistics are easy to obtain. However, this type of detection method is greatly affected by the accuracy of the inertial device, the higher the IMU accuracy, the better the detection performance. At the same time, it is affected by the cumulative effect of the inertial navigation device error. When the spoofing attacks exist for a longer period, this type of algorithm will no longer be applicable. Besides it cannot satisfy the detection requirement of induced slowly varying spoofing interference.
Filter innovation is defined as the difference between the actual observed value of a system state variable and the predicted value of the Kalman filter algorithm, which is the new information added to the observed value at the current moment. The spoofing attack directly affect the system measurement information, which in turn will cause the filter innovation to be affected. Therefore, test statistic constructed by the statistical characteristics of the normalized filter innovation can be used for spoofing detection. [35] analyzed the impact of spoofing attack on the Kalman filtering process, and the summary of the conclusions can be obtained as follows:
Currently, spoofing detection with filter innovation can be categorized into snapshot and sequential methods [36]. Snapshot method is to construct the test statistic only with the current moment of the innovation, while sequential method is to construct the test statistic using the innovation sequences and their covariance matrices within a time window. Typical snapshot methods include the chi-square test based on innovation [37, 38], and the multiple solution separation [39]. The chi-square detection method based on innovation is only effective for step spoofing with large amplitude fluctuations. The multiple solution separation method can effectively detect slowly varying spoofing, but not for the full satellite spoofing scenario. One of the typical sequential methods is Autonomous Integrity Monitoring Extrapolation (AIME) [40], which utilizes the sequence of Kalman filtering innovation to construct a test statistic. Literature [41] states that, compared to the snapshot method of detection, the extrapolation method is more suitable for satellite slowly varying spoofing detection. Spoofing offsets of position and velocity are very small during the filtering period when facing slowly varying spoofing signal attack, leading to the filter slowly correcting the output of the inertial navigation with a small correction amount. This property gives the snapshot method a long detection time and a high rate of missed alarms [42]. Meanwhile, the error tracking and closed-loop correction mechanism of Kalman filter are also the reasons for long detection delay problem of AIME when detecting slowly varying spoofing [43].
To enhance the detection performance and reduce the detection delay associated with slowly varying spoofing detection methods, literature [44] introduced a spoofing detection algorithm based on adaptive sequential probability ratio detection (SPRT). Combined with Bayes parameter estimation theory, SPRT can adaptively adjust the test statistic by modifying the risk parameter, thereby enhancing both the detection speed and performance of the algorithm. In addition to optimizing the innovation sequence algorithm, [45] proposed the detection algorithm that utilizing the changing rate of innovation to construct the test statistic. Integration of SPRT with AIME has significantly improved the detection efficiency of slowly varying spoofing detection. Additionally, [46] put forward a spoofing detection algorithm based on innovation skewness. It is experimentally demonstrated that the algorithm can improve the detection delay performance of induced retardation spoofing attacks by more than 35% compared to the general continuous method.
Robust estimation is a class of estimation methods that minimize the influence of observations in the presence of anomalous observations [47]. Therefore, robust estimation can not only be used to solve the problem of residual influence of fake calendar elements in the past for deception suppression, but also can solve the problem of error tracking and closed-loop correction feedback mechanism to improve the performance of spoofing detection algorithms. The spoofing detection algorithm based on robust estimation are designed to attenuate the effect of spoofing interference by selecting a suitable equivalent weight function to compute the weights [48–52]. The model of the detection method is shown in Figure 6, where
Figure 6. The flowchart of spoofing detection algorithm based on robust estimation. The algorithm constructs the test statistic
For the problem of high false alarm rate of the traditional innovation detection algorithms after the deception disappears, [53] established a mode adjustment criterion based on GNSS/INS tightly coupled system. Its core idea was employing sliding window detection to downgrade the innovation when the measurement value may be anomalous while other time remaining unchanged. By switching between the two modes, the computational burden of past observations and the detection delay were shortened. When subjected to intermittent spoofing attacks, the improved algorithm had higher detection sensitivity and could recover immediately after the spoofing disappeared. In addition, the response speed to the next spoofing attack was faster.
In order to avoid the effects of closed-loop correction mechanisms, other scholars have equivalently implemented closed-loop correction using an open-loop correction structure with cumulative error valuations [54]. Particularly, [55] combined the sliding window accumulation of chi-square detection based on innovation with the open-loop correction structure for spoofing detection of GNSS/INS tightly coupled system. Compared with the traditional chi-square detection method, this algorithm reduced the detection time for trap spoofing interference by 25% and improved the detection sensitivity for slowly varying spoofing interference.
Overall, the use of spoofing detection methods based on innovation can effectively identify trap spoofing. However, the detection time for slowly varying spoofing attacks may be prolonged due to error tracking and the negative feedback effect of Kalman filter. In some cases, the combined navigation system may already have been deceived by the spoofing attack before successful detection, allowing the spoofing to achieve its intended purpose. Additionally, many detection algorithms for slowly varying spoofing attacks may struggle to effectively detect when the deception disappears, potentially leading to harmful consequences.
With the rapid development of artificial intelligence (AI) in recent years, many scholars explore the use of neural networks for deception detection problems. The Probabilistic Neural Network (PNN), whose model structure is shown in Figure 7, stands out for its rapid convergence, high classification accuracy, and effectiveness in pattern recognition and fault detection [56]. For instance, [57] developed a PNN model for detecting forwarding spoofing, ensuring real-time detection of such interference. Additionally, researchers have leveraged innovative approaches such as generative adversarial networks (GANs) to combat GNSS spoofing by learning and analyzing spoofed signal features [58]. Furthermore, in literature [59], spoofing attacks were detected by creating a feature vector that captures the differences in velocity estimates from GNSS receivers and IMUs on genuine and spoofed trajectories, followed by training a neural network for detection. These methods have yielded good detection results. However, it is evident that AI-based spoofing detection methods require the collection of data related to spoofed and real signals in advance, and the detection model is poorly migratable, which constrains the widespread use of the algorithms.
Figure 7. The model structure of PNN. PNN consists of input layer, sample layer, addition layer and output layer. The core of PNN is the sample layer. The sampple layer is used to calculate the pattern distance of the samples to be recognized and then the radial basis function is used as the activation function.
In addition to AI-based approaches, some researchers have tapped into redundant information from alternative navigation augmentation systems like visual odometry (VO) to assist spoofing detection [60]. VO can serve as a good supplement to GNSS positioning. This article first used an optimized coupling framework to fuse the measurement results of VO and INS, and then monitored the deviation between the fusion results and GNSS. After successfully detecting deception, the optimized estimation algorithm is modified to prevent the system from being affected by deceptive GNSS data and enable it to continue localization. However, it is important to note that this detection method may necessitate adjustments to the hardware system and is typically applicable only to combined navigation systems that already include visual odometry.
Anti-spoofing technology not only needs to detect and identify the spoofing signals, but also needs to mitigate the effects of spoofing attacks as much as possible after spoofing detection.
Some scholars have proposed borrowing deception suppression methods from multipath suppression techniques. While the characteristics of multipath effects and deception attacks share similarities, there are key distinctions: (1) Signal delay difference: The multipath signal tends to lag behind the real satellite signals, while the deception signal may be ahead of the real signals; (2) Receiver Tracking Loop Impact: Multipath signals distort the correlation peaks of the tracking loop, affecting tracking accuracy. In contrast, deception signals can be separated from the correlation peaks of the spoofed signal using correlation strategies. This separation can lead the tracking loop to lock onto the spoofed signal, preventing the estimation of parameters for the genuine satellite signal by the Multipath Estimation Delay Locked Loop (MEDLL). Therefore, the spoofing suppression algorithm needs to control the receiver tracking loop according to the spoofing signal identification results to ensure that the receiver always locks on the real satellite signal. To deal with these distinctions, a spoofing mitigation algorithm must tailor the control of the receiver tracking loop based on the identified spoofing signals. This approach ensures that the receiver consistently locks onto the authentic satellite signal, mitigating the impact of deception attacks.
The utilization of MEDLL in a GNSS/INS integrated navigation system, as described in literature [61], represents a typical approach for spoofing mitigation. By leveraging INS information, this method can effectively identify and suppress spoofed signals. Furthermore, literature [62, 63] introduced the multi-correlator structure of MEDLL for the GNSS/INS integrated navigation system. When combined with the robust Kalman filtering algorithm, this structure resulted in an effective anti-spoofing algorithm. The algorithm reduced the position error under spoofing attacks from 600 m to 10.0 m [63]. However, it is important to note that while algorithms based on multipath suppression demonstrate strong spoofing detection and suppression capabilities, they are reliant on the presence of genuine satellite signals for their operation. In scenarios where genuine satellite signals are absent, these algorithms may not be effective. Therefore, further research and development may be necessary to address this limitation and ensure robust anti-spoofing capabilities in all operational conditions.
It is indeed well-recognized that integrating robust factor into filtering algorithms can effectively suppress the impact of spoofing attacks in combined navigation systems. Many contemporary research efforts focusing on spoofing mitigation algorithms within combined navigation systems have centered their improvements on the robust estimation algorithm. For instance, [64] analyzed the impact of spoofing attacks on GNSS/INS integration and explored an anti-spoofing method based on Adaptively Robust Kalman Filter. By this way, they succeeded in bolstering the system’s anti-spoofing interference capability and adaptive capacity.
Overall, current research on deception mitigation algorithms can be categorized into the following three types: a) Utilizing the MEDLL algorithm to recover genuine positioning results by distinguishing between authentic and spoofed signals; b) Incorporating the robust factor into filtering algorithms to mitigate the impact of spoofing on measurement information; c). The spoofing mitigation based on the relevant algorithms of AI. Generally speaking, the research on spoofing suppression algorithms is relatively small, and spoofing mitigation algorithms based on integrated navigation need to be studied deeper.
Anti-spoofing technology based on GNSS/INS integrated navigation system has become increasingly important for navigation security. Although some research progress has been made in this area, there are still many problems and challenges that need to be further explored and investigated. The following section will analyze the problems encountered and provide an outlook on future development trends for the research area.
Currently, anti-spoofing techniques for combined navigation systems mainly focus on spoofing detection and identification. But it is indeed crucial to not only focus on spoofing detection and identification but also on spoofing mitigation to enhance the safety and reliability of integrated navigation systems. By developing effective spoofing mitigation algorithms, the normal operation of the navigation system and the maintenance of high accuracy under spoofing attacks will be ensured. Research that delves deeper into the characteristics of spoofing signals and their propagation mechanisms will be essential for the advancement of anti-spoofing technologies. This will ultimately contribute to the development of more robust and secure integrated navigation systems in the future.
Existing anti-spoofing techniques often can only address a single type of spoofing attack and lack sufficient resistance to complex and variable spoofing methods. Therefore, future research will likely focus on improving the system’s ability to resist such attacks. With the continuous maturation of AI and machine learning algorithms, the GNSS/INS combined navigation system can integrate various anti-spoofing techniques, together with AI models to adaptively identify and cope with various spoofing attacks, thus achieving intelligent and adaptive anti-spoofing techniques. On the other hand, it is also necessary to strengthen research on spoofing interference techniques to provide support for feasibility testing of anti-spoofing techniques.
Under the dynamic environment, such as high-speed motion or complex terrain, anti-spoofing techniques are put to the test in terms of real-time and accuracy. The system must quickly and accurately distinguish between the real and spoofed signals, which places greater demands on the technique’s performance. To address this challenge, future research will focus on optimizing algorithms and data processing methods to improve the system’s real-time and accuracy. For instance, to reduce data processing time, one can use more efficient signal processing techniques. Additionally, to improve the system’s computational power and response speed, advanced hardware platforms and parallel computing techniques can be utilized.
Depending on the depth of information, the GNSS/INS integrated navigation system has three types of combined modes: loose integration, tight integration and deep integration. The performance and impact of these modes differ significantly when dealing with spoofing interference. There are few studies analyze the impact of spoofing and anti-spoofing research for deeply coupled systems. The existing literature primarily focuses more on anti-spoofing technology based on loosely coupled systems and tightly coupled systems. In recent years, with the continuous development of theoretical research and engineering practice in deeply coupled systems, the anti-spoofing need for deeply coupled systems has become increasingly prominent. Therefore, analyzing the impact of spoofing interference on the deep GNSS/INS integration system and developing appropriate anti-spoofing studies holds great theoretical significance and practical value.
This paper focuses on the anti-spoofing technology of GNSS/INS integrated navigation systems for enhancing the safety of integrated system. Firstly, the paper introduces the principle of spoofing interference technology and attack strategies, which have different classifications based on their generating modes, attack strategies, and manifestations. Secondly, the paper sorts out and summarizes the current research status of anti-spoofing technology of GNSS/INS combined navigation systems. This paper compares and analyzes the performance characteristics and technical aspects of detection methods based on the measured values, filter innovation, and other detection methods based on integrated navigation systems. Then, the paper sorts out the spoofing mitigation methods based on multipath suppression and robust estimation. Finally, with the purpose of providing solid technical support for the safe application of satellite navigation systems, this paper points out the difficulties faced by the development of GNSS/INS anti-spoofing technology and the future development direction.
LW: Conceptualization, Investigation, Methodology, Writing–original draft, Writing–review and editing. LC: Conceptualization, Investigation, Methodology, Writing–original draft, Writing–review and editing. BL: Funding acquisition, Supervision, Validation, Writing–review and editing. ZhL: Supervision, Writing–review and editing. ZoL: Validation, Writing–review and editing. ZuL: Funding acquisition, Methodology, Supervision, Writing–original draft, Writing–review and editing.
The author(s) declare that financial support was received for the research, authorship, and/or publication of this article. National Natural Science Foundation of China under Grant U20A0193.
The authors declare that the research was conducted in the absence of any commercial or financial relationships that could be construed as a potential conflict of interest.
All claims expressed in this article are solely those of the authors and do not necessarily represent those of their affiliated organizations, or those of the publisher, the editors and the reviewers. Any product that may be evaluated in this article, or claim that may be made by its manufacturer, is not guaranteed or endorsed by the publisher.
GNSS, Global Navigation Satellite System; GPS, Global Positioning System; INS, Inertial Navigation System; RAIM, Receiver Autonomous Integrity Monitoring; PRN, Pseudo-Random Noise; IMU, Inertial Measurement Unit; AIME, Autonomous Integrity Monitoring Extrapolation; SPRT, Adaptive sequential probability ratio detection; AI, Artificial intelligence; PNN, Probabilistic Neural Network; GAN, Generative Adversarial Network; VO, Visual Odometry; MEDLL, Multipath Estimation Delay Locked Loop.
1. Zhang H, Yang X, Liang J, Xu X, Sun X. Gps path tracking control of military unmanned vehicle based on preview variable universe fuzzy sliding mode control. Machines (2021) 9:304. doi:10.3390/machines9120304
2. Wang X, Zhao Q, Xi R, Li C, Li G, Li LA. Review of bridge structural health monitoring based on gnss: from displacement monitoring to dynamic characteristic identification. IEEE Access (2021) 9:80043–65. doi:10.1109/ACCESS.2021.3083749
3. Zhu H, Chen K, Chai H, Ye Y, Liu W. Characterizing extreme drought and wetness in guangdong, China using global navigation satellite system and precipitation data. Satellite Navigation (2024) 5:1. doi:10.1186/s43020-023-00121-6
4. Chen Q, Zhang Q, Niu X, Liu J. Semi-analytical assessment of the relative accuracy of the gnss/ins in railway track irregularity measurements. Satellite Navigation (2021) 2:25. doi:10.1186/s43020-021-00057-9
5. Li X, Chen L, Lu Z, Wang F, Liu W, Xiao W, et al. Overview of jamming technology for satellite navigation. Machines (2023) 11:768. doi:10.3390/machines11070768
6. Gao Y, Li G, Lv Z. Current situation and prospect of satellite navigation interference technology. Geomatics and Spat Inf Technol (2022) 45:13–8. doi:10.3969/j.issn.1672-5867.2022.06.005
7. Song J, Lu Z, Liu Z, Xiao Z, Dang C, Wang Z, et al. Review on the time-domain interference suppression of navigation receiver. Syst Eng and Electron (2023) 45:1164–76. doi:10.12305/i.issn.1001-506X.2023.04.25
8. Zhang L, Zhang C, Gao Y. Gnss spoofing and detection (i): typical events and development of spoofing technology. J Navigation Positioning (2021) 9:1–7. doi:10.16547/j.cnki.10-1096.20210301
10. Tang B, Zheng C, Zhang L, Wang Z. New progress and implication of United States navigation warfare. Navigation Positioning and Timing (2020) 7:110–6. doi:10.19306/i.cnki.2095-8110.2020.04.014
11. Volpe JA. Vulnerability assessment of the transportation infrastructure relying on the global positioning system (2001).
12. Dehghanian V, Nielsen J, Lachapelle G. Gnss spoofing detection based on signal power measurements: statistical analysis. Int J Navigation Observation (2012) 2012:1–8. doi:10.1155/2012/313527
13. Nielsen J, Dehghanian V, Lachapelle G. Effectiveness of gnss spoofing countermeasure based on receiver cnr measurements. Int J Navigation Observation (2012) 2012:1–9. doi:10.1155/2012/501679
14. Zhang X, Ding C, Chen S. Spoofing detection technique using carrier phase double difference of spin dual-antenna. Navigation Positioning and Timing (2023) 10:32–8. doi:10.19306/i.cnki.2095-8110.2023.02.005
15. Li J, Zhu X, Ouyang M, Shen D, Chen Z, Dai Z. Gnss spoofing detection technology based on Doppler frequency shift difference correlation. Meas Sci Technol (2022) 33:095109. doi:10.1088/1361-6501/ac672a
16. Wang S, Liu H, Tang Z, Ye B. Binary phase hopping based spreading code authentication technique. Satellite Navigation (2021) 2:4–9. doi:10.1186/s43020-021-00037-z
17. Kuhn MG. An asymmetric security mechanism for navigation signals. In: International workshop on information hiding; 23-25 May 2004; Toronto, ON, Canada. Springer (2004). p. 239–52.
18. Kerns AJ, Wesson KD, Humphreys TE. A blueprint for civil gps navigation message authentication. In: 2014 IEEE/ION Position, Location and Navigation Symposium-PLANS 2014; 05-08 May 2014; Monterey, CA, USA. IEEE (2014). p. 262–9.
19. Ghorbani K, Orouji N, Mosavi MR. Navigation message authentication based on one-way hash chain to mitigate spoofing attacks for gps l1. Wireless Personal Commun (2020) 113:1743–54. doi:10.1007/s11277-020-07289-z
20. Wu Z, Zhang Y, Liu R. Bd-ii nma&ssi: an scheme of anti-spoofing and open beidou ii d2 navigation message authentication. IEEE Access (2020) 8:23759–75. doi:10.1109/ACCESS.2020.2970203
21. Zhang L, Zhang C, Gao Y. Gnss spoofing and detection (iii): spoofing detection technology based on auxiliary information. J Navigation Positioning (2021) 9:13–9. doi:10.16547/j.cnki.10-1096.20210502
22. Li X, Lu Z, Yuan M, Liu W, Wang F, Yu y., et al. Tradeoff of code estimation error rate and terminal gain in scer attack. IEEE Trans Instrumentation Meas (2024) 73:1–12. doi:10.1109/TIM.2024.3406807
23. Gao Y, Lv Z, Zhou P, Jia Z, Zhang L, Cong D. Current status and prospects of satellite navigation deception interference technology. Geomatics and Spat Inf Technol (2019) 42:116–20. doi:10.3969/j.issn.1672-5867.2019.10.034
24. Broumandan A, Lachapelle G. Spoofing detection using gnss/ins/odometer coupling for vehicular navigation. Sensors (2018) 18:1305. doi:10.3390/s18051305
25. Curran JT, Broumendan A. On the use of low-cost imus for gnss spoofing detection in vehicular applications. In: International Technical Symposium on Navigation and Timing (ITSNT 2017); 14-17 Nov 2017; Toulouse, France (2017). p. 1–8.
26. Wu Z. Research on inertial assisted detection algorithm for induced GNSS deception. Master’s thesis. Changsha: National University of Defense Technology (2018).
27. Lee JH, Kwon KC, An DS, Shim DS. Gps spoofing detection using accelerometers and performance analysis with probability of detection. Int J Control Automation Syst (2015) 13:951–9. doi:10.1007/s12555-014-0347-2
28. Kwon K-C, Shim D-S. Performance analysis of direct gps spoofing detection method with ahrs/accelerometer. Sensors (2020) 20:954. doi:10.3390/s20040954
29. Chang H, Pang C, Zhang L, Guo Z, Lv M, Wu Q. An ins-assisted bds pseudorange rate consistency deception signal detection method. J Air Force Eng Univ (2022) 23:51–7. doi:10.3969/i.issn.2097-1915.2022.04.008
30. Liu K. Research on GNSS spoofing detection algorithm and experimental verification methods. Ph.D. thesis. Changsha: National University of Defense Technology (2019).
31. Guo Y. Research on covert spoofing algorithm of UAV based on INS/GNSS integrated navigation. Ph.D. thesis. Changsha: National University of Defense Technology (2019).
32. Li S, Liu y., Zhang H, Zhang X. Inertial measurements aided gnss spoofing detection technique. J Chin Inertial Technol (2013) 21:336–40+353. doi:10.13695/j.cnki.12-1222/o3.2013.03.006
33. Wu Z, Wu W, Liu K. Research on algorithm of gradually induced spoofing detection based on tightly coupled ins/gnss integration. Navigation Positioning and Timing (2019) 6:7–13. doi:10.19306/j.cnki.2095-8110.2019.01.002
34. Liu Y, Li S, Fu Q, Zhou Q. Chip-scale atomic clock aided ins/gnss integrated navigation system spoofing detection method. J Chin Inertial Technol (2019) 27:654–60. doi:10.13695/j.cnki.12-1222/o3.2019.05.014
35. Liu Y, Li S, Fu Q, Liu Z. Impact assessment of gnss spoofing attacks on ins/gnss integrated navigation system. Sensors (2018) 18:1433. doi:10.3390/s18051433
36. Liu Y, Li S, Fu Q, Liu Z, Zhou Q. Analysis of kalman filter innovation-based gnss spoofing detection method for ins/gnss integrated navigation system. IEEE Sensors J (2019) 19:5167–78. doi:10.1109/JSEN.2019.2902178
37. Abuhashim TS, Abdel-Hafez MF, Al-Jarrah MA. Building a robust integrity monitoring algorithm for a low cost gps-aided-ins system. Int J Control Automation Syst (2010) 8:1108–22. doi:10.1007/s12555-010-0520-1
38. Yang C, Mohammadi A, Chen Q-W. Multi-sensor fusion with interaction multiple model and chi-square test tolerant filter. Sensors (2016) 16:1835. doi:10.3390/s16111835
39. Liu H, Yue Y, Yang Y, Jiang D. Integrity monitoring for gnss/inertial based on multiple solution separation. J Chin Inertial Technol (2012) 20:63–8. doi:10.13695/j.cnki.12-1222/o3.2012.01.007
40. Ye Q, Gu Y, Li L, Du F, Li R. Integrity monitoring for gnss/ins integrated navigation based on improved aime. In: China satellite navigation conference. Springer (2023). p. 533–44. doi:10.1007/978-981-99-6944-9_46
41. Bhatti UI, Ochieng WY, Feng S. Integrity of an integrated gps/ins system in the presence of slowly growing errors. part i: a critical review. Gps Solutions (2007) 11:173–81. doi:10.1007/s10291-006-0048-2
42. Zhong L, Liu J, Li R, Wang R. Approach for detecting soft faults in gps/ins integrated navigation based on ls-svm and aime. The J Navigation (2017) 70:561–79. doi:10.1017/S037346331600076X
43. Wang S, Zhan X, Pan W, et al. Gnss/ins tightly coupling system integrity monitoring by robust estimation. J Aeronautics, Astronautics Aviation (2018) 50:61–80. doi:10.6125/JoAAA.201803_50(1).06
44. Zhong L, Liu J, Yu L, Zhang Z. Slowly varying spoofing interference detection algorithm based on adaptive sprt. J Signal Process (2022) 38:2144–54. doi:10.16798/j.issn.1003-0530.2022.10.015
45. Bhatti UI, Ochieng WY, Feng S. Performance of rate detector algorithms for an integrated gps/ins system in the presence of slowly growing error. GPS solutions (2012) 16:293–301. doi:10.1007/s10291-011-0231-y
46. Xie F, Lin H, Yu J, Mou W. Research on spoofing detection of gnss/ins tightly coupled system based on skewness test. In: 2023 5th International Conference on Electronic Engineering and Informatics (EEI); 30 June 2023 - 02 July 2023; Wuhan, China. IEEE (2023). p. 254–61. doi:10.1109/EEI59236.2023.10212511
47. Zhou J. Classical error theory and robust estimation. Acta Geodaetica et Cartographica Sinica (1989) 18:115–20.
48. Jiang Y, Pan S, Ye F, Gao W, Ma C, Wang H. Approach for detection of slowly growing fault based on robust estimation and improved alme. Syst Eng Electron (2022) 44:2894–902. doi:10.12305/i.issn.1001-506x.2022.09.24
49. Zhang C, Zhao X, Pang C, Wang Y, Zhang L, Feng B. Improved fault detection method based on robust estimation and sliding window test for ins/gnss integration. J Navigation (2020) 73:776–96. doi:10.1017/S0373463319000778
50. Ke Y, Lv Z, Zhou M, Deng X, Zhou S, Ai H. Innovation optimal robust estimation spoofing detection algorithm of tightly coupled gnss/ins integration. J Chin Inertial Technol (2022) 30:272–80. doi:10.13695/j.cnki.12-1222/o3.2022.02.020
51. Zhang C, Lv Z, Zhang L, Gao Y. A spoofing detection algorithm for ins/gnss integrated navigation system based on innovation rate and robust estimation. J Chin Inertial Technol (2021) 29:328–33. doi:10.13695/j.cnki.12-1222/o3.2021.03.008
52. Ke Y, Lv Z, Zhang C, Deng X, Zhou W, Song D. Tightly coupled gnss/ins integration spoofing detection algorithm based on innovation rate optimization and robust estimation. IEEE Access (2022) 10:72444–57. doi:10.1109/ACCESS.2022.3186305
53. Ren L, Zhao X, Pang C, Zhang C, Zhang L. Improved integrity monitoring method based on robust estimation of gnss/ins integrated navigation. Aerospace Control (2021) 39:21–6. doi:10.16804/j.cnki.issn1006-3242.2021.05.004
54. Zou S, Zhang Q, Ding Z. Using accumulated errors to realize close-loop rectification of integrated navigation system. Acta Electronica Sinica (2001) 29:1221–4.
55. Zhong L, Liu J. Research on spoofing attacks detection technology based on tightly integrated navigationt. In: The 5th Chinese aeronautics science and technology conference. Jiaxing, China: Beihang University Press (2021). p. 418–23. doi:10.26914/c.cnkihy.2021.064888
56. Zhang Y, Jia Y, Wu W, Su X, Shi X. Application of probabilistic neural network to typical fault diagnosis of vehicle gearbox. Automotive Eng (2020) 42:972–7. doi:10.19562/j.chinasae.qegc.2020.07.018
57. Pang C, Guo Z, Lv M, Zhang L, Zhai D, Zhang C. Bds against repeater deception iamming detection algorithm based on pnn. J Chin Inertial Technol (2021) 29:554–60. doi:10.13695/j.cnki.12-1222/o3.2021.04.021
58. Li J, Zhu X, Ouyang M, Li W, Chen Z, Fu Q. Gnss spoofing jamming detection based on generative adversarial network. IEEE Sensors J (2021) 21:22823–32. doi:10.1109/JSEN.2021.3105404
59. Guizzaro C, Formaggio F, Tomasin S. Gnss spoofing attack detection by imu measurements through a neural network. In: 2022 10th Workshop on Satellite Navigation Technology (NAVITEC); 05-07 April 2022; Noordwijk, Netherlands. IEEE (2022). p. 1–6. doi:10.1109/NAVITEC53682.2022.9847562
60. Gu N, Xing F, You Z. Gnss spoofing detection based on coupled visual/inertial/gnss navigation system. Sensors (2021) 21:6769–90. doi:10.3390/s21206769
61. Xu R, Ding M, Meng Q, Liu J. Spoofing interference identification technique of medll aided gnss/ins system. J Chin Inertial Technol (2018) 26:223–30. doi:10.13695/j.cnki.12-1222/o3.2018.02.013
62. Shang X, Sun F, Zhang L, Wang D, Ke Y. Ins aided gnss spoofing identification and suppression method. J Chin Inertial Technol (2022) 30:181–7. doi:10.13695/j.cnki.12-1222/o3.2022.02.007
63. Shang X, Sun F, Zhang L, Cui J, Zhang Y. Detection and mitigation of gnss spoofing via the pseudorange difference between epochs in a multicorrelator receiver. GPS solutions (2022) 26:37. doi:10.1007/s10291-022-01224-4
64. Hao Y, Shi C, Xu A, Sui X, Xia M. Revealing methods of gnss spoofing mitigation through analyzing the spoofing impacts on adaptively robust estimation-based rtk/ins tightly coupled integration. IEEE Sensors J (2023) 23:25165–78. doi:10.1109/JSEN.2023.3303199
Keywords: anti-spoofing, GNSS/INS integrated navigation, spoofing interference, spoofing detection, spoofing mitigation
Citation: Wang L, Chen L, Li B, Liu Z, Li Z and Lu Z (2024) Development status and challenges of anti-spoofing technology of GNSS/INS integrated navigation. Front. Phys. 12:1425084. doi: 10.3389/fphy.2024.1425084
Received: 29 April 2024; Accepted: 23 September 2024;
Published: 08 October 2024.
Edited by:
Jay R. Johnson, Andrews University, United StatesCopyright © 2024 Wang, Chen, Li, Liu, Li and Lu. This is an open-access article distributed under the terms of the Creative Commons Attribution License (CC BY). The use, distribution or reproduction in other forums is permitted, provided the original author(s) and the copyright owner(s) are credited and that the original publication in this journal is cited, in accordance with accepted academic practice. No use, distribution or reproduction is permitted which does not comply with these terms.
*Correspondence: Baiyu Li, bGliYWl5dUBudWR0LmVkdS5jbg==
†These authors have contributed equally to this work and share first authorship
Disclaimer: All claims expressed in this article are solely those of the authors and do not necessarily represent those of their affiliated organizations, or those of the publisher, the editors and the reviewers. Any product that may be evaluated in this article or claim that may be made by its manufacturer is not guaranteed or endorsed by the publisher.
Research integrity at Frontiers
Learn more about the work of our research integrity team to safeguard the quality of each article we publish.