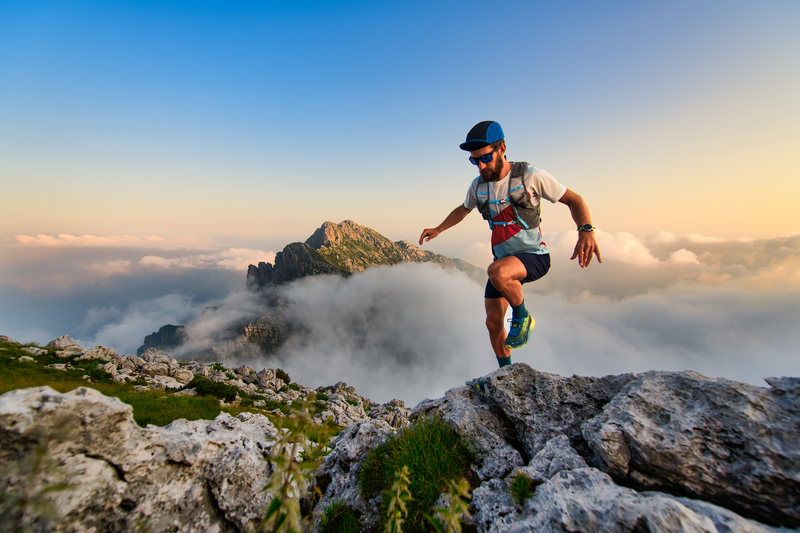
94% of researchers rate our articles as excellent or good
Learn more about the work of our research integrity team to safeguard the quality of each article we publish.
Find out more
ORIGINAL RESEARCH article
Front. Phys. , 16 February 2023
Sec. Social Physics
Volume 11 - 2023 | https://doi.org/10.3389/fphy.2023.1113714
Modern technology has brought novel types of wealth. In contrast to hard cash, digital currency does not have a physical form. It exists in electronic forms only. To date, it has not been clear what impacts its ongoing growth will have, if any, on wealth distribution. Here, we propose to identify all forms of contemporary wealth into two classes: ‘distinguishable’ or ‘identical’. Traditional tangible moneys are all distinguishable. Financial assets and cryptocurrencies, such as bank deposits and Bitcoin, are boson-like, while non-fungible tokens are fermion-like. We derived their ownership-based distributions in a unified manner. Each class follows essentially the Poisson or the geometric distribution. We contrast their distinct features such as Gini coefficients. Furthermore, aggregating different kinds of wealth corresponds to a weighted convolution where the number of banks matters and Bitcoin follows Bose–Einstein distribution. Our proposal opens a new avenue to understand the deepened inequality in modern economy, which is based on the statistical physics property of wealth rather than the individual ability of owners. We call for verifications with real data.
When two one-dollar banknotes are randomly gifted to two people, there occur total four possible ways of distributions. While counting so, it has been naturally assumed that both notes are distinguishable from each other, since they are for sure distinct physical objects, not to mention the different serial numbers printed on them. In contrast, when two cents are credited to a pair of savings bank accounts, there are three possibilities because the two cents as deposits are indistinguishable. Deposits do not have a physical form. They exist in the form of abstract numbers by ‘claim’ and ‘trust’ between the bank and the account holders. While one’s can add up to a natural number, say
all the one’s are intrinsically identical and indistinguishable from one another. The notion of being indistinguishable, or interchangeably identical, is a fundamental property of elementary particles in physics: bosons can share quantum states but fermions subject to the Pauli’s exclusion principle cannot. Consequently, their statistical distributions differ significantly. While the identical property holds certainly for particles at quantum scale, there appears no clear-cut limit of applicability to larger macroscopic objects. In this paper, we propose to identify all kinds of wealth into two classes: distinguishable or identical. All the traditional tangible moneys, i.e., hard cash including minted coins and banknotes, are of physical existence and belong to the distinguishable class. In contrast, financial assets such as bank deposits, stocks, bonds, and loans belong to the boson-like identical class. Furthermore, all the electronic forms of wealth share the identical property. At deep down level of information technology or atomic physics, they comprise of chain of bits which have finite length. The pieces of information stored are accordingly limited mostly to the amounts and, hence, are abstract like the deposit or the natural number (1). With no restriction on the amount of possession, cryptocurrencies, e.g., Bitcoin [1], are boson-like. Contrarily, having unique digital identifiers, non-fungible tokens (NFTs) may be identified as fermions. Having said so, we shall demonstrate that generic identical wealth can be universally and effectively described by Gentile statistics [2] which postulates a cutoff for the maximal amount of possession. It is an established fact that distinguishable, bosonic, and fermionic particles follow, respectively, the Maxwell–Boltzmann, Bose–Einstein, and Fermi–Dirac statistics, which are all about the number of the particles themselves for a given energy. On the contrary, our primary interest in this work is to derive the ownership-based distributions of wealth, i.e., the number of owners who possess a certain amount of wealth, while the owners are assumed to be always distinguishable. Furthermore, it is our working assumption that wealth is distributed in a ‘random’ manner. This should be the case if ideally the owners were all equal. It goes beyond the scope of the present paper to test the hypothesis against real data.
Basic scheme through elemental examples: We start with an elementary example of distributing M number of minted one-cent coins to N number of people in a random manner. We let nk be the number of people each of whom owns k number of coins, k = 0, 1, 2, ⋯. As we focus on ‘private ownership’ meaning no allowance of sharing, the opposite notion “kn” does not make sense (except kn=1), which in a way breaks the symmetry between people and coins both of which are distinguishable. There are two constraints nk’s satisfy.
Irrespective of our notation, an effective upper bound in the sums exists such as 0 ≤ k ≤ M. Our primary aim is to compute the total number of all possible or ‘degenerate’ ways of distributions for a given set
So, that for the M coins to be grouped into
is, as the coins are distinguishable,
Crucially, for each case in ϒ, any of Φ can equally occur. Thus, the total number of possible distributions for a given set
We turn to savings accounts. We consider the M cents to be now credited to distinguishable N savings accounts. Since deposits are boson-like identical, the total number of possible distributions Ω is essentially ϒ (3) itself up to multiplying an insignificant overall constant. This irrelevant degeneracy can arise when the bank accounts keep records of all the details of the crediting of the deposits, e.g. the time of transaction, which would make the credited M cents to appear seemingly distinguishable. However, all the information of each credit are recorded in a chain of bits which has a finite length, say l = l0 + l1 that decomposes into l0 for the very record of the amount k and l1 reserved for any extra information. While the former is rigidly fixed, the extra pieces of information are rather stochastic and, hence, contribute to ln Φ by a constant shift, l1 ln 2, which is, hence, ignorable.1
Last, fermion-like wealth or NFTs set M = 1 and, thus, fix the ownership-based distribution rather trivially:
For a unifying general analysis, we consider distinguishable and identical wealth together. We call each unit of wealth an object and postulate that there are
and the total number of the Ith-kind objects is
Hereafter,
On one hand, as the owners are distinguishable, the number of partitions or groupings of the N owners into the different ownerships of
On the other hand for the partitions of the objects, only the distinguishable class of objects contributes, as in (5).
For each partition of owners in ϒ, any of the partitions of distinguishable objects in Φ can equally occur. Therefore, the final, total number of possible outputs for a given set
We proceed to apply the variation method to ln Ω and aim to acquire the extremal solution of
Namely, the total number of owners and those of distinguishable objects of each kind are all conserved, as we assume them to be indestructible. For the identical class of objects, since they may transform to other species, we impose that only their total value
is conserved. To proceed, we employ a well-known approximation for the factorial,
Around the extremal distribution, this variation should vanish, while
we should have for every
This gives the desired extremal solution.
where
To write this, we have solved α in terms of N and the normalization constants,
It remains to determine
which quantify the ‘popularity’ (or inverse ‘rarity’ c.f. [4]) of the digital wealth. As the geometric distribution is essentially the exponential Boltzmann–Gibbs law, we may identify
The distribution of the total value follows
where
while the truncated Poisson distribution Pi(ki) (16) with a finite cutoff Λi can be applicable to rare valuable items that are not necessarily hard cash; henceforth, for simplicity, we set Λi = ∞ (distinguishable) and
are then the elemental ‘atomic’ distributions in (16). Here, m > 0 is the mean value in each distribution. For the fermionic distribution, it should be less than one, such as m = 1/N. Furthermore, the variance is m or m(1 ± m) for the distinguishable or bosonic/fermionic cases. In the vanishing limit m → 0, they all reduce to a Kronecker-delta distribution:
Poisson versus Geometric: As relevant to both financial assets and cryptocurrencies, here we make various comparisons between Pp(m, k) and
While
That is to say, the Poisson distribution is on-peak for the owners of the averaged wealth m = M/N, namely, the ‘middle class’. Furthermore, the ratio of the two distributions
shows that the geometric distribution has a thicker tail than Poisson one for k ≫ m. Yet, complementary to this, an inequality holds:
which implies that the probability for k > m is larger in the Poisson distribution compared to the geometric one, see Figure 1. In fact, in the large m limit, we have [7]
FIGURE 1. The probability to own more than mean value m: ∑k>mPp(m, k) (Poisson for distinguishable wealth, red) vs.
Thus, 50% or about 37% of the holders have more than the mean value in the Poisson or geometric distribution.
We compare Shannon entropy, S = ∑k − P(k) ln P(k). Since, both P(k) and −ln P(k) are non-negative, the entropy is bounded S ≥ 0. The saturation occurs when everyone has the equal amount of wealth i.e. the average value m implying
The entropy of the Poisson distribution Pp(m, k) [8],
is then roughly half of the maximum (26) for large m.
We draw the Lorenz curves of Pp(m, k) and
and obtain an analytic expression of the Lorenz curve:
of which the large m limit is known [9].
FIGURE 2. Lorenz curves of the Poisson distribution Pp(m, k) for distinguishable wealth. i) m = ∞,
FIGURE 3. Lorenz curves of the geometric distribution
At last, we compute the Gini coefficient defined by
For Pp(m, k), from
For
We note then
Especially in the large m limit, we get
More than one bank: we now consider the deposits of savings accounts at more than one bank which allow external transfers and adopt the same minimal unit of currency. That corresponds to the equal-weighted convolution (19) of the geometric distributions: with
where
The fact k⋆ < m implies that
An intuitive explanation is as follows. When the number of the banks is infinite, each bank has most likely zero or only one unit of the deposits. The identical wealth then effectively becomes distinguishable by the distinct banks. In this way,
Boson-like Bitcoin: As a cryptocurrency, Bitcoin [1] belongs to the identical class of wealth. Although, each UTXO has its unique cryptographic hash, it generates insignificant ignorable pieces of information. UTXOs of a common value are identical, while those of different values are distinguishable, c.f. [11, 12]. The value of every UTXO is discretized in a minimal unit called ‘satoshi’. In this unit, we have
The generating function of the total value (20) is then
and thus, for
where
We need to determine
Practically putting
and fix
Furthermore, from the Hardy–Ramanujan formula of the partition, we obtain for large enough v,
such that its maximum
is positioned at v⋆ which is smaller than the mean value,
This inequality implies that, despite the large
According to [13], as of 2022, the total number of addresses reads N ∼ 109, and the total value of all the UTXOs is roughly
Discussion: To conclude, traditional tangible moneys are distinguishable; yet financial assets and cryptocurrencies are all identical. The usage of the boson-like wealth results in more unequal geometric-type distribution compared to the Poisson-type distribution of the distinguishable wealth. While so aggregating different kinds of wealth leads to a weighted convolution. In particular, the existence of more than one bank softens the economic inequality of the geometric distribution by a monopolistic bank. Similar to (36) which is for bosonic geometric distributions, the equal-weighted-convolution of fermionic geometric distributions (21) also converges to a Poisson distribution in the large limit of total amount
converges to a Poisson distribution,
This provides an alternative derivation of the Poisson distribution of distinguishable objects. Even though hard cash is distinguishable, each of them is unique and thus its distribution should coincide with that of NFT, i.e. the fermionic geometric distribution (21). After considering multiple of them of the same value, through the equal-weighted-convolution, the Poisson distribution emerges consistently out of the bosonic as well as fermionic geometric distributions, (36) and (47).
The distribution of Bitcoin is given by the number-theory partition. For completeness, the convolution of a geometric and a Poisson distribution, as for hard cash and savings account, reads
which carries a power-law tail
Putting
where m1 ≥ 0 and m2 ≥ 0 are the mean values of deposit and debt respectively. In particular, for
A priori, the Poisson and geometric distributions (21) depend on the mean ‘number’ m = M/N (dimensionless), rather than any ‘value’ (“dimensionful”). Therefore, any adjustment of the minimal unit, e.g., demolishing cents and keeping euros only, can change the number M and affect the distributions.
It would be of interest to investigate any phase transition for the master distribution (15) through the changes of variables, even if N is finite [14]. As Bitcoin is boson-like, one may wonder about Bose–Einstein condensation especially to the minimal
We have restricted our work to be theoretical. Yet, the resulting distributions including Figure 2, 3 appear consistent with real data, for example [15–17]. In addition, the (truncated) Poisson-type distribution (16) can be applied not only to tangible moneys, but also to various objects, including citations of research papers [18].
Taking into account the individual differences of owners, or other extra factors, may weaken the assumed ‘randomness’. Even so, we expect that the difference of inequality in distributions persists depending on the class of wealth, distinguishable or identical. We call for thorough verifications with wide applications.
Last, while we have borrowed the notion of indistinguishability from particle and statistical physics for the description of financial wealth, namely, econophysics [19–21], our results such as (36) may help to understand how macroscopic objects formed by many identical particles appear distinguishable, i.e., through the generation of large degeneracy of quantum states.
The original contributions presented in the study are included in the article; further inquiries can be directed to the corresponding author.
J-HP conceived the idea of identical wealth and derived the distributions. ZYK brought the cryptocurrencies and electronic tokens to the project.
This study was supported by the Basic Science Research Program through the National Research Foundation of Korea (NRF) Grants NRF-2016R1D1A1B01015196 and NRF-2020R1A6A1A03047877 (Center for Quantum Spacetime).
The authors wish to thank Marc Jourdan, Chunghyoung Lee, Sukgeun Lee, Hocheol Lee, and Glassnode Support Team for helpful communications. This work was supported by the Basic Science Research Program through the National Research Foundation of Korea (NRF) Grants NRF-2016R1D1A1B01015196 and NRF-2020R1A6A1A03047877 (Center for Quantum Spacetime).
The authors declare that the research was conducted in the absence of any commercial or financial relationships that could be construed as a potential conflict of interest.
All claims expressed in this article are solely those of the authors and do not necessarily represent those of their affiliated organizations, or those of the publisher, the editors, and the reviewers. Any product that may be evaluated in this article, or claim that may be made by its manufacturer, is not guaranteed or endorsed by the publisher.
1In this reason, we prefer to say credits are boson-like rather than (precisely) bosons. Furthermore, we note that the extra pieces of information are generically postdictive: they do not preexist before the transactions take place, or before the ownerships settle down.
2The geometric distribution
3Alternative to (30), we may compute the Gini coefficient through an integral of the Lorenz curve (29),
which differs from
4In contrast, rather natural from the very distinguishability, the equal-weighted convolution of the Poisson distributions is closed:
5For
1. Nakamoto S. Bitcoin: A peer-to-peer electronic cash system. Available at: https://bitcoin.org/en/https://bitcoin.org/bitcoin.pdf (Accessed October 31, 2008).
2. Gentile J. itOsservazioni sopra le statistiche intermedie. Nuovo Cim (1940) 17:493–7. doi:10.1007/BF02960187
3. Huang K. Introduction to statistical physics. Chapman and Hall/CRC (2009). doi:10.1201/9781439878132
4. Mekacher A, Bracci A, Nadini M, Martino M, Alessandretti L, Aiello LM, et al. Heterogeneous rarity patterns drive price dynamics in NFT collections. Sci Rep (2022) 12:13890. doi:10.1038/s41598-022-17922-5
5. Dragulescu A, Yakovenko VM. Statistical mechanics of money. Eur Phys J B-Condensed Matter Complex Syst (2000) 174:723–9. doi:10.1007/s100510070114
6.Multi Token Standard ERC-1155. The Ethereum improvement proposal repository. Multi Token Standard ERC-1155 (2018). Available at: https://github.com/ethereum/EIPs/issues/1155.
7.NIST. NIST digital library of mathematical functions (2022). Available at: https://dlmf.nist.gov/8.11.
8. Evans RJ, Boersma J. The entropy of a Poisson distribution (C. Robert Appledorn). SIAM Rev (1988) 30(2):314–7. doi:10.1137/1030059
9. Dragulescu A, Yakovenko VM. Evidence for the exponential distribution of income in the USA. Eur Phys J B-Condensed Matter Complex Syst (2001) 20:585–9. doi:10.1007/pl00011112
10. Ramasubban TA. The mean difference and the mean deviation of some discontinuous distributions. Biometrika (1958) 45(3-4):549–56. doi:10.1093/biomet/45.3-4.549
11. Kahn CM. Tokens vs. accounts: Why the distinction still matters, on the economy. Federal Reserve Bank of St. Louis (2020). Available at: https://www.stlouisfed.org/on-the-economy/2020/october/tokens-accounts-why-distinction-matters.
12. Milne AKL. Argument by false analogy: The mistaken classification of Bitcoin as token money. Available at SSRN: https://ssrn.com/abstract=3290325 (Accessed November 25, 2018).
13.Studio Glassnode. Studio Glassnode (2022). Available at: https://studio.glassnode.com/metrics?a=BTC.
14. Park J-H, Kim SW. Thermodynamic instability and first-order phase transition in an ideal Bose gas. Phys Rev A (2010) 81:063636. doi:10.1103/PhysRevA.81.063636
15. Lundberg J, Waldenström D. Wealth inequality in Sweden: What can we learn from capitalized income tax data? Rev Income Wealth (2018) 64:517–41. doi:10.1111/roiw.12294
16. Juelsrud RE. Deposit concentration at financial intermediaries. Econ Lett (2021) 199(2021):109719. ISSN 0165-1765. doi:10.1016/j.econlet.2020.109719
17. Dragulescu A, Yakovenko VM. Exponential and power-law probability distributions of wealth and income in the United Kingdom and the United States. Physica A: Stat Mech its Appl (2001) 2991-2:213–21. doi:10.1016/s0378-4371(01)00298-9
18. Berg J. Journal impact factors –Fitting citation distribution curves. Blog-post, Science (2016). Available at https://www.science.org/content/blog-post/journal-impact-factors—fitting-citation-distribution-curves.
19. Mantegna R, Stanley HE. Introduction to econophysics. Cambridge University Press (1999). Available at: https://EconPapers.repec.org.
20. Stanley HE, Amaral LAN, Canning D, Gopikrishnan P, Liu Y. Econophysics: Can physicists contribute to the science of economics? Physica A: Stat Mech its Appl (1999) 269(1):156–69. ISSN 0378-4371. doi:10.1016/S0378-4371(99)00185-5
Keywords: econophysics, wealth distribution, statistical physics, boson, fermion, indistinguishability
Citation: Kim ZY and Park J-H (2023) Distinguishable cash, bosonic bitcoin, and fermionic non-fungible token. Front. Phys. 11:1113714. doi: 10.3389/fphy.2023.1113714
Received: 01 December 2022; Accepted: 23 January 2023;
Published: 16 February 2023.
Edited by:
Haroldo V. Ribeiro, State University of Maringá, BrazilReviewed by:
Ervin Kaminski Lenzi, Universidade Estadual de Ponta Grossa, BrazilCopyright © 2023 Kim and Park. This is an open-access article distributed under the terms of the Creative Commons Attribution License (CC BY). The use, distribution or reproduction in other forums is permitted, provided the original author(s) and the copyright owner(s) are credited and that the original publication in this journal is cited, in accordance with accepted academic practice. No use, distribution or reproduction is permitted which does not comply with these terms.
*Correspondence: Jeong-Hyuck Park, cGFya0Bzb2dhbmcuYWMua3I=
Disclaimer: All claims expressed in this article are solely those of the authors and do not necessarily represent those of their affiliated organizations, or those of the publisher, the editors and the reviewers. Any product that may be evaluated in this article or claim that may be made by its manufacturer is not guaranteed or endorsed by the publisher.
Research integrity at Frontiers
Learn more about the work of our research integrity team to safeguard the quality of each article we publish.