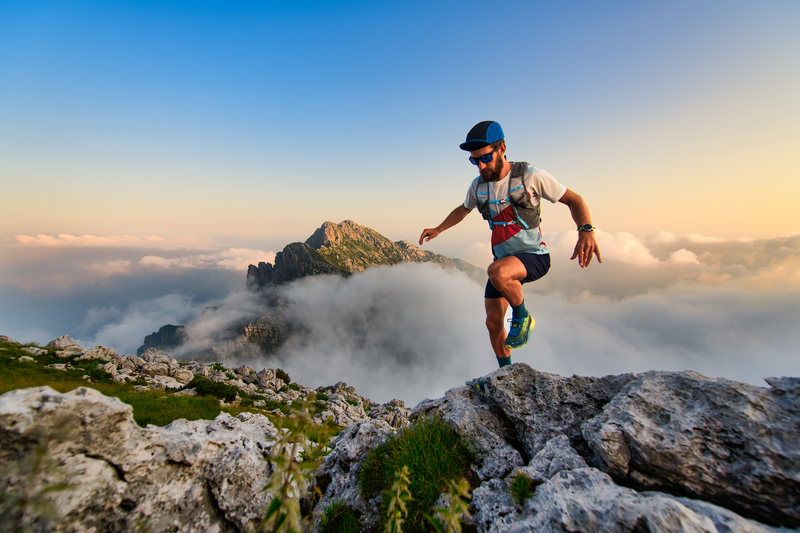
95% of researchers rate our articles as excellent or good
Learn more about the work of our research integrity team to safeguard the quality of each article we publish.
Find out more
ORIGINAL RESEARCH article
Front. Pharmacol. , 31 March 2025
Sec. Experimental Pharmacology and Drug Discovery
Volume 16 - 2025 | https://doi.org/10.3389/fphar.2025.1576467
This article is part of the Research Topic Morphological Changes in Immune Cells for Precision Sepsis Treatment View all 4 articles
Sepsis is a life-threatening condition characterized by a dysregulated host response to infection, resulting in high mortality rates and complex clinical management. This study leverages transcriptomics and machine learning (ML) to identify critical biomarkers and therapeutic targets in sepsis. Analyzing microarray data from the Gene Expression Omnibus (GEO) datasets GSE28750, GSE26440, GSE13205, and GSE9960, we discovered three pivotal biomarkers that BMX (bone marrow tyrosine kinase gene on chromosome X), GRB10 (growth factor receptor bound protein 10), and GADD45A (growth arrest and DNA damage inducible alpha), exhibiting exceptional diagnostic accuracy (AUC >0.9). Functional enrichment analyses revealed that these genes play key roles in reactive oxygen species metabolism and immune response regulation. Specifically, GADD45A was positively correlated with eosinophils and inversely associated with activated NK cells, CD8 T cells, and activated memory CD4 T cells. BMX showed positive correlations with eosinophils, mast cells, and neutrophils, while GRB10 was linked to eosinophils and M2 macrophages. Additionally, we constructed a comprehensive mRNA-miRNA-lncRNA regulatory network, identifying key interactions that may drive sepsis pathogenesis. Molecular docking and dynamics simulations validated Bendroflumethiazide, Cianidanol, and Hexamidine as promising therapeutic agents targeting these biomarkers. In conclusion, this integrated approach provides profound insights into the molecular mechanisms underlying sepsis, pinpointing BMX, GRB10, and GADD45A as pivotal biomarkers and therapeutic targets. These findings significantly enhance our understanding of sepsis pathophysiology and lay the groundwork for developing personalized diagnostic and therapeutic strategies aimed at improving patient outcomes.
Sepsis is a severe, life-threatening condition characterized by a dysregulated host response to infection, leading to systemic inflammation, multi-organ dysfunction, and high mortality rates (Laura et al., 2021). Globally, sepsis affects approximately 48.9 million individuals annually and accounts for over 11 million deaths, making it a critical public health concern (Kristina et al., 2020). Beyond its immediate lethality, sepsis survivors often endure long-term functional impairments, underscoring the urgent need for more precise diagnostic and therapeutic strategies (Evangelos et al., 2024).
Although widely used clinical biomarkers such as procalcitonin and C-reactive protein provide some prognostic value, they fail to capture the full complexity and dynamic nature of sepsis (Saxena et al., 2024). The pathophysiology of sepsis involves a delicate balance between hyperinflammatory and immunosuppressive responses, complicating efforts to develop effective treatments (Liu D. et al., 2022). Consequently, there is a pressing need for more comprehensive and specific biomarkers that can enhance diagnostic accuracy, predict clinical outcomes, and guide targeted therapies (Cohen and Banerjee, 2024). Traditional approaches to biomarker discovery typically focus on a limited set of molecular factors and often do not account for the multifaceted biological interactions that drive sepsis progression (Pierrakos et al., 2020).
Recent advancements in transcriptomics have significantly improved our understanding of the molecular mechanisms underlying sepsis. Transcriptomic studies have revealed numerous genes implicated in immune dysregulation and disease progression, offering valuable insights into the complex pathophysiology of sepsis (Liu B. et al., 2022). However, analyzing these high-dimensional datasets and identifying clinically meaningful biomarkers remains a challenge (Mohanty et al., 2023). Machine learning (ML) algorithms provide a powerful solution to this complexity. By leveraging computational techniques capable of handling vast amounts of transcriptomic data, ML methods can identify subtle patterns, complex interactions, and critical features that conventional statistical approaches may overlook (Ke et al., 2023). This approach is particularly novel and promising in the context of sepsis, as it enables the comprehensive analysis of thousands of molecular factors and their relationships to immune infiltration patterns. Identifying key transcriptomic biomarkers associated with immune cell dynamics and sepsis outcomes can illuminate disease mechanisms and reveal new therapeutic targets (You et al., 2023). Moreover, ML-driven biomarker discovery has the potential to substantially improve patient risk stratification, inform personalized treatment strategies, and facilitate earlier, more accurate interventions, ultimately improving survival rates and quality of life for sepsis patients.
This study leverages advancements in transcriptomics and ML methodologies to uncover biomarkers and therapeutic targets that can improve sepsis diagnosis and treatment. By combining differential gene expression analysis, weighted gene co-expression network analysis, ML-driven feature selection, functional enrichment analyses, immune cell infiltration profiling, mRNA-miRNA-lncRNA network construction, and in silico drug target prediction, we uncover key biomarkers involved in sepsis pathogenesis and explore their therapeutic potential. This integrated approach provides valuable insights into novel therapeutic strategies for sepsis, paving the way for more targeted diagnostic tools and precision therapies in clinical sepsis management.
High-throughput microarray expression sequencing data for sepsis were retrieved from the Gene Expression Omnibus (GEO) database (https://www.ncbi.nlm.nih.gov/geo/) (Barrett et al., 2013). Four datasets were selected for this study: GSE28750, GSE26440, GSE13205, and GSE9960. The datasets GSE28750, GSE26440, and GSE13205, which include a total of 132 sepsis patients and 60 healthy controls, were used as the training set. These datasets underwent log transformation and batch effect correction using the “Combat” function from the “sva” package in R (Leek et al., 2012). The GSE9960 dataset, consisting of 54 sepsis patients and 16 healthy controls, was designated as the validation set. Detailed information regarding the sample types, group sizes, and inclusion criteria is provided in Supplementary Table S1.
Differentially expressed genes (DEGs) between sepsis patients and healthy controls were identified using the “limma” package in R, with statistical thresholds set at |log2 fold change (FC)| > 2 and adjusted P-value (Padj) < 0.05 (Ritchie et al., 2015). The distribution and significance of DEGs were visualized using heatmaps generated by the “pheatmap” and “ggplot2” packages (Ito and Murphy, 2013). To identify gene modules associated with sepsis, we performed weighted gene co-expression network analysis (WGCNA) using the “WGCNA” package in R (Langfelder and Horvath, 2008). All samples were initially clustered to identify and exclude outliers, and genes with similar expression patterns were grouped into modules based on a topological overlap matrix (TOM) derived from the adjacency matrix. The analysis was performed with a deep splitting level of 2, a minimum module size of 100, and a soft-threshold power of 15. Gene significance (GS) and module membership (MM) were calculated for each gene, and modules with a correlation coefficient greater than 0.7 were identified as hub modules for further analysis.
To identify robust biomarkers, 5 ML algorithms were applied to the training datasets. Least Absolute Shrinkage and Selection Operator (LASSO) regression, implemented with the “glmnet” package, was used to shrink regression coefficients and select key features (Waldorp and Haslbeck, 2024). The Random Forest (RF) model, constructed using the “randomForestSRC” package, ranked features based on mean decrease in accuracy (Hu and Szymczak, 2023). Support Vector Machines with Recursive Feature Elimination (SVM-RFE) was performed using the “caret” package, iteratively removing less informative features to optimize prediction accuracy (Sanz et al., 2018). Neural networks were built using the “nnet” package, and Gradient Boosting Machine (GBM) were implemented with the “gbm” package (Salditt et al., 2023). Common features identified across all five methods were visualized using a Venn diagram (Jia et al., 2021). Diagnostic accuracy was assessed using Receiver Operating Characteristic (ROC) curves, with area under the curve (AUC) calculated for each gene.
The diagnostic performance of the selected biomarkers, BMX (bone marrow tyrosine kinase gene on chromosome X), GRB10 (growth factor receptor bound protein 10), and GADD45A (growth arrest and DNA damage inducible alpha), was validated using the GSE9960 dataset. Gene expression levels were visualized with violin plots generated using “ggplot2” in R. ROC curves were generated to evaluate diagnostic accuracy, and AUC values were calculated. Prognostic significance was assessed using cox proportional hazards regression, with hazard ratios (HRs) and 95% confidence intervals visualized in forest plots (Cioci et al., 2021).
Gene Ontology (GO), Kyoto Encyclopedia of Genes and Genomes (KEGG), and Disease Ontology (DO) enrichment analyses were performed to understand the functions of genes associated with sepsis, using the “clusterProfiler” and “DOSE” packages in R (Yu et al., 2012). The significance threshold was set at p < 0.05, and the top 15 most significant GO terms and KEGG pathways were visualized with “ggplot2”. Gene Set Enrichment Analysis (GSEA) was used to predict significant biological processes and pathways associated with hub genes, while Gene Set Variation Analysis (GSVA) compared gene set variations between groups, using the “clusterProfiler”, “enrichplot”, and “ggplot2” packages (Kleino et al., 2022). Protein-protein interaction (PPI) networks were constructed using the STRING database (https://cn.string-db.org/) with a confidence score >0.7, and further visualized with Cytoscape (version 3.9.1) (Elisa et al., 2021).
Immune cell infiltration patterns in sepsis and healthy controls were evaluated using the CIBERSORT algorithm, which estimates the relative abundance of 22 immune cell types. Single-sample Gene Set Enrichment Analysis (ssGSEA) was used to score immune-related pathway activities (Kleino et al., 2022). Correlation analyses were conducted to examine the relationships between gene expression levels and immune cell proportions, using Spearman’s rank correlation. The results were visualized as scatter plots, violin plots, and heatmaps.
Drug-gene interactions were identified using the Comparative Toxicogenomic Database (CTDbase) (https://ctdbase.org/) and Enrichr (https://maayanlab.cloud/Enrichr/). Protein structures were retrieved from UniProt (https://www.uniprot.org/) (Bateman et al., 2022), and molecular docking simulations were performed using AutoDock Vina (version 1.2.0) (Jerome et al., 2021).
A 100-ns molecular dynamics (MD) simulation was conducted using GROMACS 2023 to evaluate the reliability of the protein-drug docking results (Gabriel et al., 2023). The protein structure was parameterized using the CHARMM36 force field, and drug topology was generated with the GAFF2 force field. The protein-drug complex was solvated in a cubic box using the TIP3P water model, and electrostatic interactions were treated with the particle mesh Ewald (PME) method and the Verlet algorithm. Van der Waals and Coulomb interactions were computed with a cutoff of 1.0 nm. The system underwent a 100-ns MD simulation under constant temperature (300 K) and pressure (1 bar) to ensure stability and validate the docking results.
Transcriptome data analysis, ML model construction, and validation were performed using R (version 4.3.3). Molecular docking simulations were conducted using AutoDock Vina (version 1.2.0), and molecular dynamics simulations were performed using GROMACS 2023. Statistical significance was set at p < 0.05.
To explore the potential molecular mechanisms of sepsis, we merged and standardized the training datasets, creating an expression matrix with 15,748 genes from 132 sepsis patients and 60 healthy controls (Supplementary Figure S1). Differential expression analysis revealed 175 upregulated and 45 downregulated genes (Figure 1A). A weighted gene co-expression network was constructed with a soft threshold of 15, achieving an R2 value of 0.850 (Figures 1B–D). The topological overlap matrix (TOM) was used to perform hierarchical clustering, identifying 13 gene modules, with the MEgreen module (930 genes) showing the strongest positive correlation with sepsis (r = 0.7) (Figures 1E–G). A total of 181 candidate genes were identified from the intersection of DEGs and the MEgreen module (Figure 1H), highlighting their potential role in sepsis pathogenesis.
Figure 1. Identification of key candidate genes in sepsis through DEGs and WGCNA. (A) Heatmap of differentially expressed genes (DEGs) between sepsis patients and healthy controls. (B) Scale-free topology model fit plot for the soft thresholding power. (C, D) Mean connectivity for various soft thresholding powers. (E) Clustering dendrogram of genes with dissimilarity based on topological overlap, along with assigned module colors. (F) The heatmap visualizes the topological overlap matrix (TOM) among the selected genes. (G) Correlation between module eigengenes and sepsis status, highlighting the MEgreen module. (H) Venn diagram showing the intersection of DEGs and genes in the MEgreen module.
To identify key biomarkers with diagnostic potential for sepsis, we applied 5 ML algorithms: SVM-RFE, LASSO, Random Forest, NNET, and GBM (Table 1). SVM-RFE identified 17 genes with the lowest root mean square error (Figures 2A, B), while Random Forest ranked the top 10 genes based on their importance in sepsis-related pathways (Figure 2C). LASSO regression revealed 15 key features at a lambda of 0.040 (Figures 2D, E). NNET and GBM identified 10 genes each, highlighting nonlinear relationships (Figures 2F, G). ROC analysis confirmed the diagnostic potential of all models, with AUC values exceeding 0.7 (Figure 2H). Integration of the outputs from all five algorithms identified BMX, GRB10, and GADD45A as the core biomarkers for sepsis (Figure 2I), reinforcing their reliability for diagnosis.
Figure 2. Core biomarkers for sepsis identified through ML approaches. (A, B) Root mean square error for Support Vector Machine-Recursive Feature Elimination (SVM-RFE). (C) Importance ranking of the top 10 genes identified by Random Forest (RF). (D) Coefficient profiles of Least Absolute Shrinkage and Selection Operator (LASSO). (E) Optimal lambda selection in LASSO. (F) Gene importance scores identified by the Neural Network (NNET) model. (G) Gene importance scores identified by the Gradient Boosting Machine (GBM) model. (H) Receiver Operating Characteristic (ROC) curves showing the diagnostic accuracy of ML-models. (I) Venn diagram highlighting BMX, GRB10, and GADD45A as shared optimal feature genes identified by five ML-models.
We examined the expression of BMX, GRB10, and GADD45A in sepsis patients, observing significantly elevated expression in sepsis compared to healthy controls (Figures 3A–C). ROC curve analysis demonstrated excellent diagnostic potential, with AUC values of 0.942 for BMX, 0.900 for GRB10, and 0.954 for GADD45A (Figures 3D–F). Validation with the GSE9960 dataset confirmed the upregulation of these genes, with AUCs >0.9 (Figures 3G, H). Cox regression analysis further showed that increased expression of these biomarkers correlated with higher risk of sepsis (HR > 1, Figure 3I), underscoring their diagnostic relevance.
Figure 3. Expression analysis and validation of sepsis-related feature genes. (A–C) Violin plots of BMX, GRB10, and GADD45A expression levels in sepsis patients vs. healthy controls. (D–F) ROC curves for BMX, GRB10, and GADD45A in the training datasets. (G, H) Expression levels and ROC curves of BMX, GRB10, and GADD45A in the validation dataset. (I) Forest plot of hazard ratios (HR) from cox regression analyses for BMX, GRB10, and GADD45A.
To explore the biological roles of the identified biomarkers, functional enrichment analyses were performed. GO analysis revealed strong associations with processes such as reactive oxygen species metabolism, cellular stress response, and immune response (Figure 4A). The chord diagram highlighted BMX, GRB10, and GADD45A’s involvement in reactive oxygen species metabolism, a critical pathway in sepsis (Figure 4B). KEGG pathway analysis identified pathways related to complement activation, staphylococcus aureus infection, and neutrophil extracellular trap formation (Figure 4C). Among the genes, BMX and GRB10 are particularly associated with immune-regulating signaling pathways, while GADD45A is mainly involved in the defense response to bacterial infections (Figure 4D). DO analysis further linked these genes to bacterial diseases such as tuberculosis (Figure 4E). PPI network analysis revealed interactions with key proteins like CD177, S100P, and S100A9, suggesting their roles in immune cell activation and inflammation (Figure 4F). GSEA showed that BMX, GRB10, and GADD45A are involved in several upregulated pathways, such as inflammatory response for BMX, cytokine signaling for GRB10, and regulation of apoptosis for GADD45A (Figures 4G–I). These findings demonstrate that BMX, GRB10, and GADD45A play central roles in the immune and inflammatory mechanisms of sepsis, offering insights into its pathogenesis and potential avenues for intervention.
Figure 4. Functional enrichment and pathway analysis of BMX, GRB10, and GADD45A in sepsis. (A) Top 15 significant Gene Ontology (GO) terms (Biological Process, Cellular Component, Molecular Function) associated with BMX, GRB10, and GADD45A. (B) Chord diagram linking BMX, GRB10, and GADD45A to GO terms. (C) Kyoto Encyclopedia of Genes and Genomes (KEGG) pathway analysis highlighting key sepsis-related pathways. (D) Chord diagram mapping BMX, GRB10, and GADD45A to their respective KEGG pathways. (E) Disease Ontology (DO) analysis indicating bacterial infectious diseases. (F) Protein-Protein Interaction (PPI) network of sepsis-related genes. (G–I) Gene Set Enrichment Analysis (GSEA) plots for pathways involving BMX, GRB10, and GADD45A.
We applied the ceRNA hypothesis to predict the interactions between miRNAs and lncRNAs for the three biomarkers (BMX, GADD45A, and GRB10) (Figure 5). BMX was found to interact with 5 miRNAs and 16 lncRNAs, with the BMX-miR-758-3p-AC079586.1 pathway showing the highest connectivity. GADD45A interacted with 6 miRNAs and 12 lncRNAs, with the GADD45A-miR-1226-5p-CTB-60B18.18 pathway showing significant correlation. GRB10 exhibited the most extensive network, with 53 interactions, and the GRB10-miR-15a-5p-RP11-483P21.6 pathway demonstrated the highest correlation. These findings underscore the regulatory roles of BMX, GADD45A, and GRB10 in sepsis via complex interactions with miRNAs and lncRNAs.
Figure 5. Prediction of miRNA and lncRNA regulatory networks. Competing endogenous RNA (ceRNA) network for BMX, GRB10, and GADD45A showing significant miRNA and lncRNA interactions.
Previous studies have shown that pathogenic genes can alter the immune microenvironment of sepsis (Nicole and Hongbo, 2022). Using the CIBERSORT algorithm, we estimated the abundance of 22 immune cell types and observed significant alterations in immune cell infiltration in sepsis (Figure 6A). Sepsis was associated with increased infiltration of naive CD4 T cells, M0 and M2 macrophages, and activated mast cells, while a decrease was seen in CD8 T cells, resting memory CD4 T cells, and eosinophils (Figure 6B). Further analysis revealed that BMX was positively correlated with eosinophils, activated mast cells, and naive CD4 T cells, but negatively correlated with resting dendritic cells and activated NK cells (Figures 6C, F; Supplementary Figure S2A). GRB10 exhibited positive correlations with eosinophils and M2 macrophages, and negative correlations with follicular helper T cells and activated NK cells (Figures 6D, G; Supplementary Figure S2B). Similarly, GADD45A showed positive correlations with eosinophils and negative correlations with activated NK cells and CD8 T cells (Figures 6E, H; Supplementary Figure S2C). GSVA analysis further confirmed the roles of BMX, GRB10, and GADD45A in immune dysregulation during sepsis (Figure 6I), highlighting their involvement in immune environment alterations.
Figure 6. Immune microenvironment alterations in sepsis. (A) Barplot of immune cell type proportions in sepsis and healthy samples. (B) Violin plot showing significant differences in immune cell infiltration between sepsis and healthy samples. (C–H) Correlation plots of BMX, GRB10, and GADD45A with various immune cell types. (I–K) Gene Set Variation Analysis (GSVA) of immune pathways involving BMX, GRB10, and GADD45A.
To identify effective therapeutic molecules targeting the hub biomarkers BMX, GRB10, and GADD45A, we retrieved protein structures from the UniProt database and selected full-length models from AlphaFold predictions (BMX-HUMAN, GRB10-HUMAN, and GADD45A-HUMAN; Figures 7A–C). We then conducted virtual screening of 2115 FDA-approved small molecules from the ZINC database, selecting the top 10 drugs for each target based on their combined binding scores (Table 2). Molecular docking simulations revealed that Hydrochlorothiazide, Bendroflumethiazide, and Benzthiazide are strong binders for BMX; Chloroquine, Cianidanol, and Quercetin for GRB10; and Warfarin, Hexamidine, and Ethacrynic Acid for GADD45A (Figures 7D–F). Detailed binding interactions, including energy values, bond lengths, and hydrogen bond formations, are summarized in Table 3.
Figure 7. Protein structures and molecular drug docking for BMX, GRB10, and GADD45A. (A–C) The protein structures of BMX, GRB10 and GADD45A. (D–F) Protein target-small molecule drug docking models showing interactions and binding sites.
To confirm the stability of these drug-protein complexes, we performed molecular dynamics simulations. The root mean square deviation (RMSD) values for Bendroflumethiazide-BMX, Cianidanol-GRB10, and Hexamidine-GADD45A were 17.7 Å, 16.4 Å, and 3.6 Å, respectively (Figure 8). Radius of gyration (Rg) and Solvent Accessible Surface Area (SASA) analyses indicated reduced protein flexibility, suggesting stable binding. Additionally, root mean square fluctuation (RMSF) analysis and hydrogen bond data further confirmed strong interactions (Figure 8). These results suggest that Bendroflumethiazide, Cianidanol, and Hexamidine are promising therapeutic candidates for sepsis treatment.
Figure 8. Molecular dynamics simulation results for drug target complexes. Simulation results including Root Mean Square Deviation (RMSD), Radius of Gyration (Rg), Solvent Accessible Surface Area (SASA), Root Mean Square Fluctuation (RMSF), and hydrogen bond numbers for (A) Bendroflumethiazide-BMX, Benzthiazide-BMX, and Hydrochlorothiazide-BMX complexes; (B) Quercetin-GRB10, Cianidanol-GRB10, and Chlo roquine-GRB10 complexes, and (C) Ethacrynic-GADD45A, Hexamidine-GADD45A, and Warfarin-GADD45A complexes.
This study utilized an integrated transcriptomics and ML approach to uncover key biomarkers and therapeutic targets in sepsis. The analysis identified BMX, GRB10, and GADD45A as crucial biomarkers with high diagnostic accuracy (AUC >0.9). Functional enrichment and immune cell infiltration analyses highlighted the involvement of these biomarkers in reactive oxygen species metabolism and immune response regulation. Additionally, we constructed a comprehensive mRNA-miRNA-lncRNA regulatory network, identifying critical interactions that may influence sepsis pathogenesis. Docking and molecular dynamics studies further pinpointed potential therapeutic agents, including Bendroflumethiazide, Cianidanol, and Hexamidine, which demonstrated promising binding affinities with these biomarkers.
Previous sepsis research has largely focused on traditional biomarkers such as procalcitonin, C-reactive protein, IL-6, and TNF-α, which are critical mediators of the early inflammatory response. However, their diagnostic utility is constrained by a short detection window and high variability (Pierre et al., 2021). In contrast, our integrative transcriptomics and machine learning approach has identified more specific and robust biomarkers, as demonstrated by the superior diagnostic accuracy of BMX, GRB10, and GADD45A. These biomarkers not only exhibit greater specificity but also provide valuable insights into the immune and metabolic dynamics of sepsis, potentially enhancing their applicability across different disease stages. Although these genes have not been extensively characterized in sepsis, their known functions in other disease contexts provide important clues. BMX, a non-receptor tyrosine kinase, participates in inflammatory signaling and can influence vascular integrity (Xiuxiu et al., 2023). GRB10 modulates insulin signaling and growth factor pathways, thereby affecting cell growth and metabolic homeostasis (Ashlin et al., 2019). GADD45A is associated with DNA damage repair, apoptosis, and immune regulation (Mengbing et al., 2024; Markus and KJMRRMR, 2019). These attributes suggest that BMX may help regulate endothelial stability and leukocyte trafficking, GRB10 could shape the metabolic and proliferative states of immune cells, and GADD45A might enable immune cells to adapt to prolonged inflammatory stress (Dominic et al., 2012; Deng et al., 2020; She et al., 2023). Collectively, these features position BMX, GRB10, and GADD45A as potential key contributors to the interplay of hyperinflammation, immunosuppression, and oxidative stress that underlies sepsis progression.
Building on these insights, our functional enrichment analyses revealed that BMX, GRB10, and GADD45A are closely linked to critical pathways governing immune responses and reactive oxygen species metabolism. Such pathways are central to sepsis pathogenesis, where a dysregulated immune response and oxidative stress contribute to multi-organ failure (Wang and Liu, 2023). The correlations observed between these biomarkers and specific immune cell subsets further underscore their potential roles in modulating immune cell infiltration, activity, and overall inflammatory balance within the septic milieu. For example, GADD45A’s positive correlation with eosinophils and negative correlation with CD8 T cells is consistent with its involvement in calibrating proinflammatory and regulatory immune dynamics, in line with previous evidence of its role in inflammation (Dominic et al., 2012). BMX’s positive associations with eosinophils, activated mast cells, and neutrophils align with its capacity to promote inflammatory responses (Deng et al., 2020), while GRB10’s correlation with eosinophils and M2 macrophages supports its putative contribution to anti-inflammatory or homeostatic processes (She et al., 2023). These findings highlight the intricate relationships between these biomarkers and immune cell populations, reinforcing the notion that BMX, GRB10, and GADD45A may influence sepsis progression through complex immune regulatory networks.
The construction of the mRNA-miRNA-lncRNA network provides further mechanistic insights. For instance, the BMX-miR-758-3p-AC079586.1 and GRB10-miR-15a-5p-RP11-483P21.6 axes highlight potential regulatory mechanisms through which non-coding RNAs may influence gene expression and sepsis progression (Tian et al., 2024). Previous studies have demonstrated the critical role of miRNAs and lncRNAs in sepsis by regulating gene expression at the post-transcriptional level. For example, miR-758-3p has been implicated in inflammatory response regulation and cell apoptosis (Peng et al., 2020), while miR-15a-5p has been shown to modulate immune responses and oxidative stress (González-López et al., 2023). The involvement of lncRNAs, such as AC079586.1 and RP11-483P21.6, in sepsis further underscores their potential as therapeutic targets. This network approach underscores the complexity of gene regulation in sepsis and highlights potential targets for therapeutic intervention.
Our docking studies identified several promising therapeutic agents targeting BMX, GRB10, and GADD45A, offering opportunities for drug repurposing and targeted therapy. Specifically, Bendroflumethiazide exhibited strong binding affinity with BMX, Cianidanol showed significant interaction with GRB10, and Hexamidine formed stable complexes with GADD45A. The repositioning of these FDA-approved drugs could accelerate the development of effective sepsis treatments by targeting these newly identified biomarkers.
Despite our robust findings, several limitations must be addressed to fully utilize BMX, GRB10, and GADD45A as sepsis biomarkers and therapeutic targets. Experimental validation is crucial to confirm their roles in sepsis pathogenesis, necessitating cell-based assays with monocytes and endothelial cells using gene overexpression and CRISPR/Cas9-mediated knockdown. Additionally, animal models, such as LPS-induced sepsis and cecal ligation and puncture (CLP) mouse models, will be employed to assess the therapeutic effects of compounds like Bendroflumethiazide, Cianidanol, and Hexamidine on multi-organ damage and inflammation. A further increase in the sample size, along with the support of multicenter studies, is necessary to verify their diagnostic value across diverse populations through qRT-PCR and Western blot analysis of patient samples for clinical validation. Furthermore, experimentally confirming the interactions within the mRNA-miRNA-lncRNA network and integrating additional omics data, such as proteomics and metabolomics, will enhance our understanding of sepsis pathogenesis. These efforts aim to incorporate BMX, GRB10, and GADD45A into diagnostic panels and personalized treatment strategies, thereby improving sepsis management and patient outcomes.
In conclusion, this study leverages integrated transcriptomics and ML approaches to identify BMX, GRB10, and GADD45A as pivotal biomarkers and therapeutic targets in sepsis. These findings enhance our understanding of sepsis pathophysiology and offer new directions for diagnostic and therapeutic strategies. The identified biomarkers exhibit high diagnostic accuracy and are involved in key pathogenic pathways, providing potential targets for personalized medicine.
Publicly available datasets were analyzed in this study. These data can be found here: https://www.ncbi.nlm.nih.gov/geo/, using accession numbers GSE28750, GSE26440, GSE13205, and GSE9960.
YC: Conceptualization, Funding acquisition, Writing – original draft, Writing – review and editing. HP: Data curation, Methodology, Software, Writing – original draft, Writing – review and editing. QC: Formal Analysis, Validation, Writing – original draft, Writing – review and editing. LQ: Conceptualization, Writing – original draft, Writing – review and editing. LX: Conceptualization, Resources, Writing – original draft, Writing – review and editing.
The author(s) declare that financial support was received for the research and/or publication of this article. This work was supported by the Young Scientists Fund of the National Natural Science Foundation of China to YC (No. 82402529), and the Henan Province Medical Science and Technology Co-construction Project to YC (LHGJ20220016) and LX (LHGJ20230838), and the present work was supported by the Research and practice project of education and teaching reform in Zhengzhou university to LX (2023ZZUJGXM261).
The authors declare that the research was conducted in the absence of any commercial or financial relationships that could be construed as a potential conflict of interest.
The author(s) declare that no Generative AI was used in the creation of this manuscript.
All claims expressed in this article are solely those of the authors and do not necessarily represent those of their affiliated organizations, or those of the publisher, the editors and the reviewers. Any product that may be evaluated in this article, or claim that may be made by its manufacturer, is not guaranteed or endorsed by the publisher.
The Supplementary Material for this article can be found online at: https://www.frontiersin.org/articles/10.3389/fphar.2025.1576467/full#supplementary-material
AUC, area under the curve; BMX, bone marrow tyrosine kinase gene on chromosome X; CLP, cecal ligation and puncture; DEGs, differentially expressed genes; DO, Disease Ontology; GADD45A, growth arrest and DNA damage inducible alpha; GEO, Gene Expression Omnibus; GO, Gene Ontology; GRB10, growth factor receptor bound protein 10; GS, Gene significance; GSEA, Gene Set Enrichment Analysis; GSVA, Gene Set Variation Analysis; LASSO, Least Absolute Shrinkage and Selection Operator; MD, molecular dynamics; ML, machine learning; MM, module membership; KEGG, Kyoto Encyclopedia of Genes and Genomes; Rg, Radius of gyration; RF, Random Forest; ROC, Receiver Operating Characteristic; RMSD, root mean square deviation; RMSF, root mean square fluctuation; SASA, solvent accessible surface area; SVM-RFE, Support Vector Machines with Recursive Feature Elimination; ssGSEA, Single-sample Gene Set Enrichment Analysis; TOM, topological overlap matrix; WGCNA, weighted gene co-expression network analysis.
Ashlin, M. E., Olivia, A., and Bjajpem, S. A. (2019). Role of Grb10 in mTORC1-dependent regulation of insulin signaling and action in human skeletal muscle cells. 318(2) doi:10.1152/ajpendo.00025.2019
Barrett, T., Wilhite, S., Ledoux, P., Evangelista, C., Kim, I., Tomashevsky, M., et al. (2013). NCBI GEO: archive for functional genomics data sets--update. Nucleic Acids Res. 41, D991–D995. doi:10.1093/nar/gks1193
Bateman, A., Martin, M. J., Orchard, S., Magrane, M., Ahmad, S., Alpi, E., et al. (2022). UniProt: the universal protein knowledgebase in 2023. J. Nucleic Acids Res. 51 (0), D523–D531. doi:10.1093/nar/gkac1052
Cioci, A., Cioci, A., Mantero, A., Parreco, J., Yeh, D., and Rjsi, R. (2021). Advanced statistics: multiple logistic regression, cox proportional hazards, and propensity scores. Cox Proportional Hazards, Propensity Scores 22 (6), 604–610. doi:10.1089/sur.2020.425
Cohen, M., and Banerjee, D. J. J. (2024). Biomarkers in sepsis: a current review of new technologies. J. Intensive Care Med. 39 (5), 399–405. doi:10.1177/08850666231194535
Deng, Y., Ren, E., Yuan, W., Zhang, G., Wu, Z., and Xie, Q. J. D. (2020). GRB10 and E2F3 as diagnostic markers of osteoarthritis and their correlation with immune infiltration. Diagn. (Basel). 10 (3), 171. doi:10.3390/diagnostics10030171
Dominic, M. S., Jennifer, S. T., Barbara, H., and Dan, A. LJJCP (2012). Gadd45a and Gadd45b modulate innate immune functions of granulocytes and macrophages by differential regulation of p38 and JNK signaling. J. Cell. Physiol. 227 (11), 3613–3620. doi:10.1002/jcp.24067
Elisa, M., Sara, C., Francesco, M., and Gianpiero, GJFC (2021). Mapping, structure and modulation of PPI. Front. Chem. 9 (0), 718405. doi:10.3389/fchem.2021.718405
Evangelos, J. G.-B., Anna, C. A., Michael, B., Christoph, B., Thierry, C., Irit, G.-V., et al. (2024). The pathophysiology of sepsis and precision-medicine-based immunotherapy. Nat. Immunol. 25 (1), 19–28. doi:10.1038/s41590-023-01660-5
Gabriel, O., Florian, vdE., and Åjjctc, J. (2023). Efficient empirical valence bond simulations with GROMACS. J. Chem. Theory Comput. 19 (17), 6037–6045. doi:10.1021/acs.jctc.3c00714
González-López, P., Álvarez-Villarreal, M., Ruiz-Simón, R., López-Pastor, A., de Ceniga, M., Esparza, L., et al. (2023). Role of miR-15a-5p and miR-199a-3p in the inflammatory pathway regulated by NF-κB in experimental and human atherosclerosis. Clin. Transl. Med. 13 (8), e1363. doi:10.1002/ctm2.1363
Hu, J., and Szymczak, S. J. (2023). A review on longitudinal data analysis with random forest. Brief. Bioinform. 24 (2), bbad002. doi:10.1093/bib/bbad002
Ito, K., and Murphy, D. J. Cp (2013). Application of ggplot2 to pharmacometric graphics. CPT. Pharmacometrics Syst. Pharmacol. 2 (10), e79. doi:10.1038/psp.2013.56
Jerome, E., Diogo, S.-M., Andreas, F. T., and Stefano, FJJCIM (2021). AutoDock Vina 1.2.0: new docking methods. Expand. Force Field, Python Bind. 61 (8). doi:10.1021/acs.jcim.1c00203
Jia, A., Xu, L., and Wang, Y. J. B. (2021). Venn diagrams in bioinformatics. Brief. Bioinform. 22 (5), bbab108. doi:10.1093/bib/bbab108
Ke, L., Lu, Y., Gao, H., Hu, C., Zhang, J., Zhao, Q., et al. (2023). Identification of potential diagnostic and prognostic biomarkers for sepsis based on machine learning. Comput. Struct. Biotechnol. J. 21, 2316–2331. doi:10.1016/j.csbj.2023.03.034
Kleino, I., Frolovaitė, P., Suomi, T., Elo, L. J. C., and journal, sb (2022). Computational solutions for spatial transcriptomics, Comput. solutions spatial Transcr. 20:4870–4884. doi:10.1016/j.csbj.2022.08.043
Kristina, E. R., Sarah Charlotte, J., Kareha, M. A., Katya Anne, S., Derrick, T., Daniel Rhodes, K., et al. (2020). Global, regional, and national sepsis incidence and mortality, 1990-2017: analysis for the Global Burden of Disease Study. Lancet. 395(10219).
Langfelder, P., and Horvath, S. J. B. (2008). WGCNA: an R package for weighted correlation network analysis. BMC Bioinforma. 9, 559. doi:10.1186/1471-2105-9-559
Laura, E., Andrew, R., Waleed, A., Massimo, A., Craig, M. C., Craig, F., et al. (2021). Surviving sepsis campaign: international guidelines for management of sepsis and septic shock. Intensive Care Med. 47 (11), 1181–1247. doi:10.1007/s00134-021-06506-y
Leek, J., Johnson, W., Parker, H., Jaffe, A., and Storey, J. J. B. (2012). The sva package for removing batch effects and other unwanted variation in high-throughput experiments. 28(6):882–883. doi:10.1093/bioinformatics/bts034
Liu, B., Ao, S., Tan, F., Ma, W., Liu, H., Liang, H., et al. (2022b). Transcriptomic analysis and laboratory experiments reveal potential critical genes and regulatory mechanisms in sepsis-associated acute kidney injury. injury 10 (13), 737. doi:10.21037/atm-22-845
Liu, D., Huang, S., Sun, J., Zhang, H., Cai, Q., Gao, C., et al. (2022a). Sepsis-induced immunosuppression: mechanisms, diagnosis and current treatment options. Mil. Med. Res. 9 (1), 56. doi:10.1186/s40779-022-00422-y
Markus, C., and Kjmrrmr, B. (2019). Epigenetic regulation of DNA repair genes and implications for tumor therapy. Mutat. Res. 780 (0), 15–28. doi:10.1016/j.mrrev.2017.10.001
Mengbing, H., Ji, W., Wei, L., and Hongyan, ZJFN (2024). Advances in the role of the GADD45 family in neurodevelopmental, neurodegenerative, and neuropsychiatric disorders. Front. Neurosci. 18 (0), 1349409. doi:10.3389/fnins.2024.1349409
Mohanty, T., Karlsson, C., Chao, Y., Malmström, E., Bratanis, E., Grentzmann, A., et al. (2023). A pharmacoproteomic landscape of organotypic intervention responses in Gram-negative sepsis. Nat. Commun. 14 (1), 3603. doi:10.1038/s41467-023-39269-9
Nicole, M. C., and Hongbo, C. J. I. (2022). Metabolic adaptation of lymphocytes in immunity and disease. Immunity 55 (1), 14–30. doi:10.1016/j.immuni.2021.12.012
Peng, L., Zhang, Y., and Hjjocb, X. (2020). lncRNA SNHG3 facilitates acute myeloid leukemia cell growth via the regulation of miR-758-3p/SRGN axis. 121(2):1023–1031. doi:10.1002/jcb.29336
Pierrakos, C., Velissaris, D., Bisdorff, M., Marshall, J., and Vincent, J. J. C. (2020). Biomarkers of sepsis: time for a reappraisal. Crit. Care 24 (1), 287. doi:10.1186/s13054-020-02993-5
Pierre, H., Neus, R. B., Cristian, M. I., Marta, C. A., Adria Mendoza, M., Julie, P., et al. (2021). Monocyte distribution width (MDW) performance as an early sepsis indicator in the emergency department: comparison with CRP and procalcitonin in a multicenter international European prospective study. Crit. Care 25 (1), 227. doi:10.1186/s13054-021-03622-5
Ritchie, M., Phipson, B., Wu, D., Hu, Y., Law, C., Shi, W., et al. (2015). Limma powers differential expression analyses for RNA-sequencing and microarray studies. Nucleic Acids Res. 43 (7), e47. doi:10.1093/nar/gkv007
Salditt, M., Humberg, S., and Nestler, S. J. M. (2023). Gradient tree boosting for hierarchical data. Multivar. Behav. Res. 58 (5), 911–937. doi:10.1080/00273171.2022.2146638
Sanz, H., Valim, C., Vegas, E., Oller, J., and Fjbb, R. (2018). SVM-RFE: selection and visualization of the most relevant features through non-linear kernels. BMC Bioinforma. 19 (1), 432. doi:10.1186/s12859-018-2451-4
Saxena, J., Das, S., Kumar, A., Sharma, A., Sharma, L., Kaushik, S., et al. (2024). Biomarkers in sepsis, Clin. Chim. Acta., 562:119891 doi:10.1016/j.cca.2024.119891
She, H., Tan, L., Wang, Y., Du, Y., Zhou, Y., Zhang, J., et al. (2023). Integrative single-cell RNA sequencing and metabolomics decipher the imbalanced lipid-metabolism in maladaptive immune responses during sepsis. Front. Immunol. 14, 1181697. doi:10.3389/fimmu.2023.1181697
Tian, M., Zhan, Y., Cao, J., Gao, J., Sun, J., and Zhang, L. J. B. (2024). Targeting blood-brain barrier for sepsis-associated encephalopathy: regulation of immune cells and ncRNAs. Brain Res. Bull. 209, 110922. doi:10.1016/j.brainresbull.2024.110922
Waldorp, L., and Haslbeck, J. J. M. (2024). Network inference with the lasso. Multivar. Behav. Res. 59 (4), 738–757. doi:10.1080/00273171.2024.2317928
Wang, W., and Liu, C. J. W. W. (2023). Sepsis heterogeneity. Sepsis heterog. 19 (10), 919–927. doi:10.1007/s12519-023-00689-8
Xiuxiu, L., Michael, B., Christopher, G., Mohammad, A., Yoon-Mi, C., Chenyao, W., et al. (2023). BMX controls 3βHSD1 and sex steroid biosynthesis in cancer. J. Clin. Invest. 133 (2), e163498. doi:10.1172/jci163498
You, G., Zhao, X., Liu, J., Yao, K., Yi, X., Chen, H., et al. (2023). Machine learning-based identification of CYBB and FCAR as potential neutrophil extracellular trap-related treatment targets in sepsis. Front. Immunol. 14, 1253833. doi:10.3389/fimmu.2023.1253833
Keywords: sepsis, biomarkers, transcriptomics, machine learning, therapeutic targets, immune regulation
Citation: Cheng Y, Peng H, Chen Q, Xu L and Qin L (2025) Machine learning-based transcriptmics analysis reveals BMX, GRB10, and GADD45A as crucial biomarkers and therapeutic targets in sepsis. Front. Pharmacol. 16:1576467. doi: 10.3389/fphar.2025.1576467
Received: 14 February 2025; Accepted: 18 March 2025;
Published: 31 March 2025.
Edited by:
Erxi Wu, Baylor Scott and White Health, United StatesReviewed by:
Lynnette H. Cary, Uniformed Services University of the Health Sciences, United StatesCopyright © 2025 Cheng, Peng, Chen, Xu and Qin. This is an open-access article distributed under the terms of the Creative Commons Attribution License (CC BY). The use, distribution or reproduction in other forums is permitted, provided the original author(s) and the copyright owner(s) are credited and that the original publication in this journal is cited, in accordance with accepted academic practice. No use, distribution or reproduction is permitted which does not comply with these terms.
*Correspondence: Lijie Qin, cWlubGlqaWUxODE5QDE2My5jb20=; Lijun Xu, eHVsaWp1bjEyMTlAMTI2LmNvbQ==
†These authors have contributed equally to this work and share first authorship
Disclaimer: All claims expressed in this article are solely those of the authors and do not necessarily represent those of their affiliated organizations, or those of the publisher, the editors and the reviewers. Any product that may be evaluated in this article or claim that may be made by its manufacturer is not guaranteed or endorsed by the publisher.
Research integrity at Frontiers
Learn more about the work of our research integrity team to safeguard the quality of each article we publish.