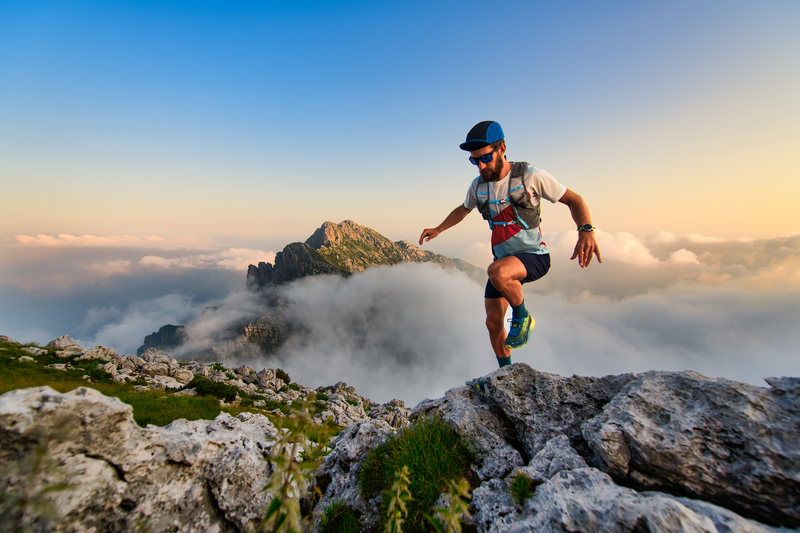
94% of researchers rate our articles as excellent or good
Learn more about the work of our research integrity team to safeguard the quality of each article we publish.
Find out more
ORIGINAL RESEARCH article
Front. Pharmacol.
Sec. Drug Metabolism and Transport
Volume 16 - 2025 | doi: 10.3389/fphar.2025.1517446
The final, formatted version of the article will be published soon.
You have multiple emails registered with Frontiers:
Please enter your email address:
If you already have an account, please login
You don't have a Frontiers account ? You can register here
Amis: Chemotherapy-induced hepatotoxicity (CIH) is a significant concern in colorectal cancer (CRC) patients treated with the CAPEOX (capecitabine and oxaliplatin) regimen. Identifying predictive factors for CIH is crucial for clinical management.This study analyzed colorectal tissue (CRT), plasma, and urine samples from CRC patients. Differentially expressed metabolites (DEMs) across these tissues were integrated for multi-omics analysis, and predictive models for CIH susceptibility were developed. An independent set of 75 plasma samples was used for validation.Results: A total of 492 differentially expressed compounds were identified in samples from 63 CRC patients, including 105, 149, and 238 DEMs in CRT, plasma, and urine, respectively. Lipids and lipidlike molecules were predominant in all samples. Among these, urine samples exhibited the highest variability and provided the strongest predictive power for CIH susceptibility. Principal component analysis (PCA) effectively differentiated normal patients from those with CIH. The study revealed steatosis as the primary pathological feature of CIH, with disrupted lipid metabolism emerging as a key characteristic. Predictive models constructed from multi-tissue metabolites profile exhibited high accuracy, with the plasma model achieving an AUC of 0.933 in external validation set. Our study underscores the importance of individual metabolic variations in CIH susceptibility, reflecting the complex interplay of genetic, environmental, and lifestyle factors.This study emphasizes the critical role of alterations in lipid, polyamine, and purine metabolism, as well as impaired tissue repair mechanisms, were identified as key endogenous factors underlying CIH susceptibility. The developed predictive models demonstrate potential for clinical application in assessing CIH risk in CRC patients undergoing CAPEOX chemotherapy.
Keywords: Chemotherapy-induced hepatotoxicity, Multi-omics analysis, CapeOX, Endogenous susceptible biomarkers, Metabolomics
Received: 28 Oct 2024; Accepted: 19 Mar 2025.
Copyright: © 2025 XU, Li, Yao, Chen, Chen, Hou, Yang, Yu, Lin, Zhu, Wang, Qiu, Liu, Wang, Tao and Liu. This is an open-access article distributed under the terms of the Creative Commons Attribution License (CC BY). The use, distribution or reproduction in other forums is permitted, provided the original author(s) or licensor are credited and that the original publication in this journal is cited, in accordance with accepted academic practice. No use, distribution or reproduction is permitted which does not comply with these terms.
* Correspondence:
Xia Tao, Department of Pharmacy, Second Affiliated Hospital of Naval Medical University, Shanghai, China
Lei Liu, Institutes of Biomedical Sciences and Intelligent Medicine Institute, Fudan University, Shanghai, China
Disclaimer: All claims expressed in this article are solely those of the authors and do not necessarily represent those of their affiliated organizations, or those of the publisher, the editors and the reviewers. Any product that may be evaluated in this article or claim that may be made by its manufacturer is not guaranteed or endorsed by the publisher.
Research integrity at Frontiers
Learn more about the work of our research integrity team to safeguard the quality of each article we publish.