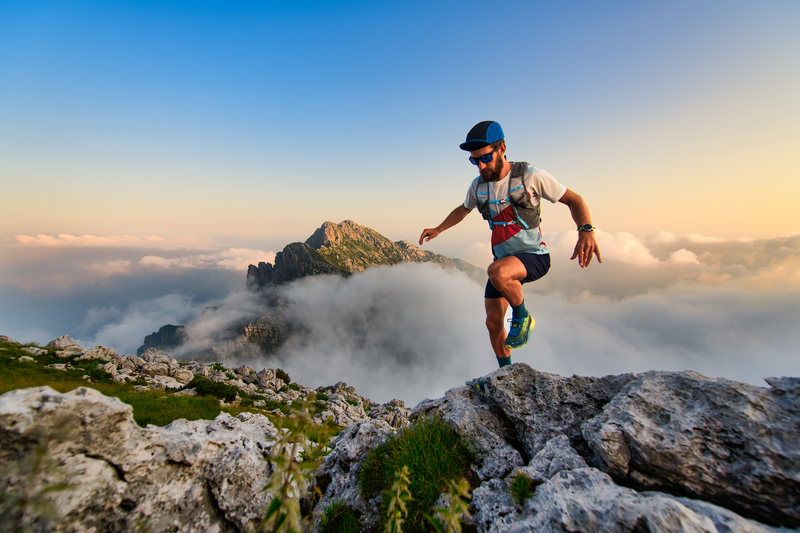
94% of researchers rate our articles as excellent or good
Learn more about the work of our research integrity team to safeguard the quality of each article we publish.
Find out more
REVIEW article
Front. Pharmacol.
Sec. Ethnopharmacology
Volume 16 - 2025 | doi: 10.3389/fphar.2025.1504230
This article is part of the Research Topic Emerging Trends in the Quality Check of Herbal Medicines, Supplements and 'Botanicals' View all 5 articles
The final, formatted version of the article will be published soon.
You have multiple emails registered with Frontiers:
Please enter your email address:
If you already have an account, please login
You don't have a Frontiers account ? You can register here
One-class modeling is a supervised multivariate botanical identification method based on principal component analysis (PCA) that constructs a model based only on the characteristics of the reference samples and uses the Q statistic as a combined metric. Test samples are judged to be similar (authentic) if their combined metric falls within the model limits or different (adulterated or contaminated) if the metric falls outside the model limits. This review initially considers 3 major factors affecting identification: the number of variables (univariate versus multivariate), the number of classes (one-class versus multi-class), and the type of analysis (quantitative versus qualitative). Multivariate analysis is commonly used for identification, providing a broader coverage of the identity specifications of the samples. With a combined metric, multivariate methods are analogous to univariate methods. One-class modeling and multi-class modeling employ different approaches for identification with one-class modeling being more flexible. While most methods to date have had a quantitative basis, qualitative methods are possible. This review focuses on multivariate, one-class modeling based on PCA. Examples are presented for the application of one-class modeling to identification of American ginseng (Panax quinquefolius), Echinacea purpurea, Black Cohosh (Actaea racemosa), and Maca (Lepidium meyenii). These examples demonstrate the utility and flexibility of one-class modeling.
Keywords: PCA, one-class mdeling, review, authentication, multivariate analysis
Received: 30 Sep 2024; Accepted: 19 Feb 2025.
Copyright: © 2025 Harnly. This is an open-access article distributed under the terms of the Creative Commons Attribution License (CC BY). The use, distribution or reproduction in other forums is permitted, provided the original author(s) or licensor are credited and that the original publication in this journal is cited, in accordance with accepted academic practice. No use, distribution or reproduction is permitted which does not comply with these terms.
* Correspondence:
James Harnly, Beltsville Human Nutrition Research Center, Agricultural Research Service (USDA), Beltsville, United States
Disclaimer: All claims expressed in this article are solely those of the authors and do not necessarily represent those of their affiliated organizations, or those of the publisher, the editors and the reviewers. Any product that may be evaluated in this article or claim that may be made by its manufacturer is not guaranteed or endorsed by the publisher.
Research integrity at Frontiers
Learn more about the work of our research integrity team to safeguard the quality of each article we publish.