- 1Department of Genetic Medicine, Faculty of Medicine, King Abdulaziz University, Jeddah, Saudi Arabia
- 2Princess Al-Jawhara Al-Brahim Centre of Excellence in Research of Hereditary Disorders, King Abdulaziz University, Jeddah, Saudi Arabia
- 3Molecular Diagnostic Laboratory at King Abdulaziz University Hospital, Jeddah, Saudi Arabia
- 4Department of Pathology, Faculty of Medicine, King Abdulaziz University, Jeddah, Saudi Arabia
- 5Research and Development Unit, Alborg Diagnostics, Jeddah, Saudi Arabia
- 6Department of Medical Laboratory Technology, Faculty of Applied Medical Sciences, King Abdulaziz University, Jeddah, Saudi Arabia
- 7Department of Clinical Biochemistry, Faculty of Medicine, King Abdulaziz University, Jeddah, Saudi Arabia
Background: Colorectal cancer (CRC) is the leading cancer among Saudis, and mutations in BRAF, KRAS, and NRAS genes are therapeutically significant due to their association with pathways critical for cell cycle regulation. This study evaluates the prevalence and frequency of somatic mutations in these actionable genes in Saudi CRC patients and assesses their pathogenicity with bioinformatics methods.
Methodology: The study employed the TruSight Tumor 15 next-generation sequencing (NGS) panel on 86 colorectal cancer (CRC) samples to detect somatic mutations in BRAF, KRAS, and NRAS genes. Bioinformatic analyses of NGS sequences included variant annotation with ANNOVAR, pathogenicity prediction, variant reclassification with CancerVar, and extensive structural analysis. Additionally, molecular docking assessed the binding of Encorafenib to wild-type and mutant BRAF proteins, providing insights into the therapeutic relevance of pathogenic variants.
Results: Out of 86 tumor samples, 40 (46.5%) harbored somatic mutations within actionable genes (BRAF: 2.3%, KRAS: 43%, NRAS: 2.3%). Fourteen missense variants were identified (BRAF: n = 1, KRAS: n = 11, NRAS: n = 2). Variants with strong clinical significance included BRAF V600E (2.32%) and KRAS G12D (18.60%). Variants with potential clinical significance included several KRAS and an NRAS mutation, while variants of unknown significance included KRAS E49K and NRAS R102Q. One variant was novel: NRAS R102Q, and two were rare: KRAS E49K and G138E. We further extended the CancerVar prediction capability by adding new pathogenicity prediction tools. Molecular docking demonstrated that Encorafenib inhibits the V600E variant BRAF protein less effectively compared to its wild-type counterpart.
Conclusion: Overall, this study highlights the importance of comprehensive molecular screening and bioinformatics in understanding the mutational landscape of CRC in the Saudi population, ultimately improving targeted drug treatments.
1 Introduction
Colorectal cancer (CRC) ranks as the third most prevalent cancer around the world, with estimations of approximately 1.9 million new cases and around 935,000 deaths in the year 2022 (Bray et al., 2024). Further estimations suggest an increase to 3.2 million new cases by the year 2040 (Morgan et al., 2023). Even as most of the cases occur among those older than 50 years, there is a significant rise in the rates of younger-onset colorectal cancers (Patel et al., 2022). In Saudi Arabia, CRC ranks first among males at 15.3% and third among females at 9.8% (Elwali et al., 2023). Most patients are present in advanced stages with symptoms of altered bowel habits, abdominal pain, rectal bleeding, and anemia (Dekker et al., 2019). CRC results from molecular changes in the colon or rectum, where there is the formation of a benign polyp that can take 5–15 years to advance into cancer (Huck and Bohl, 2016). The cause of this disease is multifactorial, with interactions between genes and the environment. About 70% of cases are considered sporadic, while 30% are inherited, with 5% being due to syndromes such as Lynch and familial adenomatous polyposis (Jasperson et al., 2010). The genetic basis of CRC has been posed to be consistent with that of the Fearon and Vogelstein model, which describes the progression from a benign adenoma to carcinoma (Fearon and Vogelstein, 1990). This model is thought to account for 70%–90% of CRC cases (Li et al., 2021). A less common way through which CRC arises is the microsatellite instability pathway, due to defects in DNA mismatch repair genes, accounting for 15%–20% of cases (Gupta et al., 2021). Lifestyle factors associated with the development of CRC include obesity, low physical activity, tobacco smoking, consumption of alcohol, and a diet high in processed red meat (Keum and Giovannucci, 2019). Endoscopic testing is considered the gold standard for diagnosing CRC, and surgical removal remains the main form of treatment for the disease (Montminy et al., 2020). In metastatic CRC (mCRC), genetic testing is used to personalize treatment protocols based on actionable somatic mutations on specific genes, namely, BRAF, KRAS, and NRAS, among others (Van Cutsem et al., 2016; Biller and Schrag, 2021). For patients who are not positively testing for such mutations, chemotherapy is administered with combined EGFR inhibitors. The KRAS and NRAS mutation-positive patients are treated with chemotherapy only (FOLFOX or FOLFIRI protocols) (Van Cutsem et al., 2016). Patients with the BRAF V600E mutation slightly benefit from chemotherapy in combination with VEGF inhibitors or a mixture of BRAF and EGFR inhibitors (Santarpia et al., 2012). The determination of somatic mutations in actionable genes (such as BRAF, KRAS, and NRAS) is vital with regards to prognosis and in offering personalized therapies. BRAF, KRAS, and NRAS encode core proteins in the RAS-RAF-MEK-MAPK pathway, which, if altered, can induce aberrant proliferation through dysregulating apoptosis and cell cycle progression from G1 to S phase (Seruca et al., 2009). Importantly, some detection of rare or novel variants in these actionable genes may help in the identification of new biomarkers for better therapeutic outcomes (Mikolajcik et al., 2021). In Saudi Arabia, a retrospective study from two hospitals reported a somatic mutational rate of 2.5% in the BRAF gene and 28.6% in KRAS (Siraj et al., 2014). Moreover, a similar study from another hospital revealed a 2.4% rate in BRAF and 30.1% in KRAS (Beg et al., 2015). Furthermore, a study for patients from the Gulf countries who were treated at two hospitals in the United States showed a mutational rate of 4% in BRAF, 44% in KRAS, and 4% in NRAS (Al-Shamsi et al., 2016). A more recent study from one Saudi hospital has shown slightly different findings for BRAF (0.4%), KRAS (49.6%), and NRAS (2%) (Alharbi et al., 2021). Additionally, a recent review has combined mutational rates from several other Saudi hospitals, showing 0.4%–2.5% rate for BRAF, 28.6%–56% in KRAS, and 2%–2.2% in NRAS (Alfahed, 2023) Our understanding of the mutational landscape of CRC in the Saudi population is lacking, and the percentage of common somatic mutations reported from Saudi hospitals is sparse. Moreover, diagnostic labs may sometimes ignore the rare mutations in CRC tumors, which could affect treatment outcomes. Overall, the genetic characterization of CRC is largely incomplete due to its complex nature. We analyzed CRC samples for somatic mutations in the BRAF, KRAS, and NRAS genes for the TruSight Tumor 15 gene panel (TST 15). The 15 genes covered by the TST 15 panel include AKT1, BRAF, EGFR, ERBB2, FOXL2, GNA11, GNAQ, KIT, KRAS, MET, NRAS, PDGFRA, PIK3CA, RET, and TP53 genes, which are frequently altered in solid tumors (Kopetz et al., 2015; Yan et al., 2015; Van Cutsem et al., 2016; Voskoboynik et al., 2016). Therefore, to understand the pathogenicity and clinical relevance of both known and novel variants, an extensive computational analysis was performed. We have combined raw DNA sequence analysis, variant clinical interpretation, pathogenicity prediction, functional domain mapping, secondary structure and stability analyses, and 3D structure superimposition. In addition, molecular docking was performed on Encorafenib against both the BRAF V600E variant protein and its wild-type counterpart. This integrated approach aims to provide insight not only into the novel biomarkers but also to extend the knowledge of the mutational spectrum of CRC in the Saudi population, helping to further refine precision oncology interventions.
2 Materials and methods
2.1 Patient selection and data collection
This study utilized CRC patient data from King Abdulaziz University Hospital (KAUH), Jeddah, Saudi Arabia. The ethical approval was obtained from the King Abdulaziz University College of Medicine Ethical Committee (No. 487-22). All data, including raw DNA sequences, were collected from the Molecular Diagnostics Laboratory at KAUH over 4 years (2018–2021). Molecular screening was conducted using the TST 15 panel on the MiSeqDx next-generation sequencing (NGS) platform (Illumina, San Diego, CA, United States).
2.2 Inclusion/exclusion criteria
The study included ∼140 mm2 colorectal tissue samples with above 30% tumor content from male and female patients. Surgeons at KAUH performed biopsies on those patients, and the KAUH Pathology Department examined these samples histopathologically. The histopathological types considered were adenoma, adenocarcinoma, cystic, mucinous, serous neoplasms, neoplasms not otherwise specified (NOS), squamous cell neoplasms, ductal and lobular neoplasms, complex epithelial neoplasms, mature B-cell lymphomas, epithelial neoplasms NOS, lipomatous neoplasms, and chronic colon inflammation. Samples that originated from tumors outside the colon or rectum or derived from whole blood were excluded. The collected data included patient demographics, clinical and histopathology diagnoses, molecular screening results, and raw DNA sequence files for the complete gene panel (AKT1, BRAF, EGFR, ERBB2, FOXL2, GNA11, GNAQ, KIT, KRAS, MET, NRAS, PDGFRA, PIK3CA, RET, TP53). After excluding tumors of other types of cancer, 86 samples out of 90 collected were eligible for this study. Corresponding to those samples, 49 raw DNA sequence files were available. The remaining files were missing due to patient requests.
2.3 Molecular screening
The TST 15 panel screens 15 genes frequently mutated in solid tumors, targeting hotspot exonic regions for single nucleotide variants (SNVs) and small insertions/deletions (Indels). The panel design is based on international guidelines by the College of American Pathologists (CAP), the International Association for the Study of Lung Cancer (IASLC), the Association for Molecular Pathology (AMP), and the American Society of Clinical Oncology (ASCO) (Andre et al., 2012; Gonzalez et al., 2013; Lindeman et al., 2013; Sepulveda et al., 2017). DNA extractions were performed from formalin-fixed paraffin-embedded (FFPE) colorectal tissue samples (∼140 mm2 tissue with above 30% tumor content) using the QIAamp DNA FFPE Tissue Kit. DNA libraries were prepared using the TruSight Tumor 15 MiSeqDx kit according to the manufacturer’s instructions. Sequencing was achieved by the MiSeqDx NGS system, generating 2 × 150 bp reads using a 5% variant allele frequency (VAF) detection threshold. The Local Run Manager software was used to analyze the data, and runs took about 36 h to complete. Quality control relied on the following metrics: mean depth of coverage: ∼30×, Q-score: ≥80% of bases higher than Q30. Demultiplexing, generation of FASTQ files, alignment to the hg19 reference genome, and variant calling in variant call format (VCF) were all done on the TruSight Tumor 15 Analysis Module on the MiSeqDx instrument. These VCF files then served as input in subsequent bioinformatic analysis that aimed at identifying and interpreting the somatic variants in CRC samples.
2.4 Annotation, filtration, and clinical interpretation of variants within CRC actionable genes
Functional annotation highly facilitates NGS data analysis. We utilized the Annotate Variation (ANNOVAR) webserver tool (wANNOVAR) to annotate the raw DNA sequence files (https://wannovar.wglab.org) (Wang et al., 2010; Chang and Wang, 2012; Yang and Wang, 2015). The input settings used in wANNOVAR were default while selecting hg19 as a reference genome. The gene panel used in this study comprises 15 genes. Only variants from 3 genes (BRAF, KRAS, NRAS), however, were used in our computational analysis since they hold established therapeutic relevance in CRC. Accordingly, variant data were filtered by the 3 genes of interest, coding region, and functional effect (non-synonymous). Only the most common gene transcripts (BRAF: ENST00000288602.6, KRAS: ENST00000311936.3, NRAS: ENST00000369535.4) were used. The resulting list of variants (Table 1) was cross-checked with molecular screening results reported by the laboratory. Missing SNP ID numbers were manually retrieved from the National Center for Bioinformatics database (NCBI) (http://www.ncbi.nlm.nih.gov). To interpret variants based on clinical significance, we have used the 2017 four-tiered system by the Association for Molecular Pathology (AMP), the College of American Pathologists (CAP), and the American Society for Clinical Oncology (ASCO). The system uses 12 criteria for variant classification: 1) Evidence of being a therapeutic biomarker for FDA-approved or investigational drugs in professional guidelines. 2) Evidence of being diagnostic in professional guidelines or with consensus. 3) Evidence of being prognostic in professional guidelines or with consensus. 4) Mutation type: activating or loss of function, copy number variation (CNV), or gene fusion. 5) Variant allele frequency (50% or 100%). 6) Potential germline: tested as germline in normal tissue. 7) low frequency in population databases. 8) Reported as pathogenic in ClinVar or the Human Gene Mutation Database (HGMD). 9) Reported in the Catalogue of Somatic Mutations in Cancer (COSMIC), My Cancer Genome (MCG), the International Cancer Genome Consortium (ICGC), or the Cancer Genome Atlas Program (TCGA). 10) Predicted as pathogenic by pathogenicity prediction tools. 11) Association with disease or pathway. 12) Availability of convincing functional or population studies (Li et al., 2017).
To facilitate variant interpretation, we used the CancerVar (Cancer Variant Interpretation) webserver (https://cancervar.wglab.org). CancerVar is a deep learning rule-based scoring software containing clinical records for 13 million variants from 1911 cancer census genes (Li et al., 2022). The input parameters specified were cancer type: colorectal cancer, genome build: hg19, and the amino acid position. The resulting interpretation along with the output for population frequencies were recorded (Table 1).
Pathogenicity prediction tools are helpful means for estimating the ability of genetic variants to cause disease. To classify variants as pathogenic or benign CancerVar uses seven tools, which are FATHMM (Shihab et al., 2013), GERP++RS (Davydov et al., 2010), MetaLR (Chen et al., 2023), MetaSVM (Kim et al., 2017), Mutation Assessor (Reva et al., 2011), Polyphen2_HDIV (Adzhubei et al., 2013), and SIFT (Ng and Henikoff, 2003). We have selected a different set of tools [BayesDel_addAF (Feng, 2017), CADD_Phred (Rentzsch et al., 2019), DANN (Quang et al., 2015), FATHMM-MKL (Shihab et al., 2015), MPC (Samocha et al., 2017), REVEL (Ioannidis et al., 2016), PrimateAI (Sundaram et al., 2018)] to improve pathogenicity predictions of identified variants. The reason behind selecting this tool set is to increase the diversity of principles used in prediction while offering alternative tools with more advanced algorithms. We have chosen FATHMM-MKL, for example, since it is an updated version of FATHMM, which uses multiple kernel learning to capture different types of data. Selecting CADD_phred and REVEL was based on their ability to combine the results of several tools into one comprehensive prediction. DANN and PrimateAI were selected for their advanced deep neural network algorithms that enable them to analyze complex patterns. We chose CADD_Phred and BayesDel_addAF since they incorporate diverse data types, including functional annotations, evolutionary conservation, and allele frequencies. MPC was chosen since it is tailored for missense mutations to increase prediction precision. PrimateAI is built with specific primate data, which enhances prediction for human variants; thus, it was added to our set. Therefore, this combination was consolidated to provide a more holistic approach with an expectation for increased overall prediction accuracy. We compared the performance of these tools with CancerVar by testing truly pathogenic (14 identified variants—Table 1) and truly benign (≥1% minor allele frequency) datasets. Variant Effect Predictor (VEP) was used to generate the prediction scores (McLaren et al., 2016) by inputting genomic coordinates for pathogenic variants and SNP IDs for benign variants. CancerVar tools prediction scores were obtained through its webserver, which were normalized to match the selected tools’ cutoff points. The scores for both sets (14 tools) were plotted in a receiver operating characteristic curve (ROC) using the EasyROC webtool (http://www.biosoft.erciyes.edu.tr/app/easyROC).
2.5 Genotype-protein-phenotype analysis of variants in CRC actionable genes
Gene-protein-phenotype characterization of cancer genes offers insights for assigning new predictive or prognostic biomarkers or designing targeted drugs. Multiple bioinformatics analyses were performed for variants found in samples that tested positive for at least one clinically actionable CRC gene (BRAF, KRAS, or NRAS) (Sepulveda et al., 2017). Other concomitant variants found only upon examining raw DNA sequences were also included in the analyses. Functional domain mapping, pathogenicity predictions, secondary structure analysis, thermodynamic stability analysis, 3D structure superimposition, and molecular docking of BRAF wild-type and mutant proteins to Encorafenib (a BRAF inhibitor) were performed. All actionable gene variants, including concomitant variants, were missense variants in the coding region. To identify novel or rare variants, a thorough search was conducted in major population databases [1000 genomes, Avon Longitudinal Study of Parents and Children (ALSPAC), The Exome Aggregation Consortium (ExAC), The Genome Aggregation Database (GnomAD), National Center for Biotechnology Information Allele Frequency Aggregator (NCBI ALFA), NHLBI Exome Sequencing Project (ESP6500), TWINSUK, UK10K], and cancer-specific databases (COSMIC, MCG, and TCGA).
2.5.1 Functional domain mapping
All of the identified missense variants in BRAF, KRAS, and NRAS genes were mapped to exons, domains, and subdomains based on the primary sequence of amino acids from literature consensus. The chromosome mapping location, mRNA transcripts, and corresponding exon numbers were collected from the Ensembl Database (https://www.ensembl.org/index.html).
2.5.2 Secondary structure and stability analyses
Secondary structure analysis illustrates differences in secondary structure elements caused by variations in amino acids. Amino acid sequences of wild-type proteins (BRAF, KRAS, and NRAS) were manually substituted in a text file with each variation, and sequences were analyzed using the NetSurfP 3.0 webtool (https://www.dtu.biolib.com/NetSurfP-3) to generate graphical images. Those images were visually compared to see whether any changes occurred in α-helices, ß-pleated sheets, or loops between wild-type and variant secondary structures.
Thermodynamic stability analysis estimates the mutational effect on protein stability. BRAF, KRAS, and NRAS missense variants were analyzed using the DUET webserver, predicting the effect of SNVs on protein stability in the form of ΔΔG (kcal/mol). DUET integrates mCSM and SDM estimations to predict a combined score (Pires et al., 2014).
2.5.3 3D structure mapping and superimposition
Variant tertiary structure generation and alignment to the respective wild-type model were achieved using the homology modeling method. Obtained from the Protein Databank (PDB) (https://www.rcsb.org), folded human 3D structures of BRAF (PDB: 6UAN), KRAS (PDB: 7KYZ), and NRAS (PDB: 6ZIO) were used as templates to generate the wild-type forms of the proteins. Wild-type 3D structures were generated using SWISS-MODEL webserver (https://www.swissmodel.expasy.org) (Waterhouse et al., 2018), except for BRAF, which was achieved throughthe I-Tasser webserver (https://www.zhanggroup.org) (Zhang, 2008; Roy et al., 2010; Yang et al., 2015), since it needed input from multiple structures to generate the full form. The mutant forms were made by the DUET webserver (Pires et al., 2014). Deviations were estimated by superimposing mutant residues on wild-type counterparts on YASARA software (https://www.yasara.org) (Land and Humble, 2018), with root mean square deviation (RMSD) values generated as outputs. The PyMol2 software (PyMOL Molecular Graphics System Ver. 2.6.0, Schrödinger, LLC) was used to visualize the aligned protein structures along with affected amino acids.
2.5.4 Computational binding of BRAF with Encorafenib
According to American guidelines, Encorafenib (a kinase inhibitor) in addition to Cetuximab (an EGFR inhibitor) can be used as a second line of treatment for mCRC patients with the BRAF V600E mutation (Morris et al., 2023). Moreover, the maximum inhibitory concentration (IC50) of Encorafenib in vitro is known to be similar in wild-type and V600E mutant BRAF proteins (Koelblinger et al., 2018). To gain more insight on Encorafenib binding behavior, molecular docking assays were performed to compare the binding affinities of Encorafenib with wild-type and V600E mutant BRAF proteins. AutoDock Vina algorithm of the SWISS-DOCK webserver (https://www.swissdock.ch) was utilized to assess two assays: one for the drug-wild-type and the other for the drug-variant (Eberhardt et al., 2021; Bugnon et al., 2024). For the docking setup, the ligand was obtained from the ZINC database in MOL2 format (zinc.docking.org) (catalogue no.: ZINC68249103) and uploaded into the SWISS-DOCK server. The molecule sketcher option was used to prepare the uploaded ligand. The previously generated wildtype and variant models (methods 2.5.3) were used in PDBQ format to prepare target molecules. For each model, specific amino acid coordinates (inhibitor binding residues) were entered in the search box center of grid box settings (Martinez Fiesco et al., 2022). The search box size and sample exhaustivity options were left on default. The drug-protein complexes with the lowest binding energies were chosen, and PyMol2 was used to record hydrogen bonds and interacting residues and visualize the results.
3 Results
3.1 Data collection
The sample’s data collected for a 4-year period (2018–2021) comprise information on clinical diagnosis, demographics, histopathology diagnosis, and molecular screening results for the 3 CRC actionable genes (BRAF, KRAS, NRAS). Some raw DNA sequence files for the full gene panel were also collected. Upon curation and filtration, the data collection process has yielded a total of 86 CRC sample data, with 49 raw DNA sequence files corresponding to some of those samples. Among the 86 samples, 41 harbored somatic mutations in CRC actionable genes, and 45 were negative for those genes. All 86 samples’s data displayed diverse clinical presentations. These include colon or rectal polyps, transverse, descending, sigmoid, rectosigmoid, non-specified colon, or rectal cancers, and chronic colon inflammation. The demographic data shows a higher number of samples for males (n = 51, 59.30%) compared to females (n = 35, 40.70%). The majority of these samples belonged to patients between the ages of 50 and 59 years old (n = 29, 33.72%), followed by patients between 60 and 69 years old (n = 22, 25.58%), 70 and 79 years old (n = 18, 20.93%), 40 and 49 years old (n = 12, 13.95%), and 30 and 39 (n = 3, 3.49%). Other remaining samples were for a patient between <30 years old (n = 1, 1.16%) and a patient >80 years old (n = 1, 1.16%). The histopathology diagnosis data of 86 patients shows a diverse range of histologic types, with each type present in small numbers. Therefore, to simplify observation for these data, each patient tissue diagnosis was broadly grouped into either adenoma (precursor lesion), adenocarcinoma (malignant form), or chronic colon inflammation. The majority of sample tissues were diagnosed with adenocarcinoma (n = 71, 82.5%), followed by adenoma (n = 9, 10.5%), and chronic colon inflammation (n = 6, 7%).
3.2 Molecular screening and variant interpretation
The 14 detected variants in 3 actionable genes among 86 samples were as follows: BRAF, n = 1 (1.16%); KRAS, n = 11 (12.79%); and NRAS, n = 2 (2.32%). The single BRAF variant was V600E. Among the 11 KRAS variants, 5 were in codon 12 (G12A, G12D, G12R, G12S, G12V) and 1 in codon 13 (G13D). Two variants of KRAS, E49K and G138E, were a concomitant pair from a single patient. The other variants of KRAS included codon 61 Q61H, codon 117 K117N, and codon 146 A146T. The 2 NRAS variants included a codon 61 mutation (Q61K) and a rare mutation at codon 102 (R102Q). The 14 variant identifications were based on molecular screening records and 49 corresponding raw DNA sequence files. All variants found in the molecular screening records were present in raw DNA sequence files, except for NRAS R102Q, which was only identified in those files. Moreover, all 14 variants were found above the 5% VAF threshold except for NRAS R102Q, which was detected at 1.4%. It was included, however, in subsequent computational analyses as it may hold potential clinical relevance upon further investigation. Furthermore, the NRAS R102Q variant was found concomitantly with another variant (KRAS G12D) in the same patient. The most common variant detected was KRAS G12D (n = 16, 18.60%). According to CancerVar interpretation, BRAF V600E and KRAS G12D were classified as Tier 1 (strong clinical significance), while KRAS E49K and NRAS R102Q were Tier III (unknown clinical significance). KRAS E49K is a rare variant, and NRAS R102Q is a novel variant. According to the COSMIC database, KRAS E49K is only reported twice from large intestine samples and once from a lung sample (COSV55695731), while the NRAS R102Q is reported only once from a thyroid sample (COSV106063871), but never from large intestines. No clinical significance data, however, for these variants is available or reported in other databases. The remaining variants were Tier II (potential clinical significance): KRAS G12A, G12R, G12S, G12V, G13D, Q61H, K117N, G138E, A146T, and NRAS Q61K. KRAS G138E is a rare variant, only reported in COSMIC twice from large intestine samples and once from an endometrium sample (COSV55967074). Moreover, this variant was found in the ExAC database at a very low allele frequency (0.00000824%). Furthermore, all 14 variants are mostly absent or present at very low allele frequencies in population databases (Table 1).
3.3 Functional domain mapping
The single variant of BRAF (V600E) is situated at exon 15 in the BRAF kinase binding domain’s CR3 lobe (residues ∼442–724) (Cope et al., 2018) (Figure 1A). KRAS codon 12 and 13 variants (G12A, G12D, G12R, G12S, G12V, and G13D) are located at the second exon in the catalytic (G) domain’s embedded P-loop region (residues ∼10-16 and 56–59). KRAS E49K and Q61H are in exon 3, with Q61H within the S2 domain (residues ∼58–76). KRAS K117N, G138E, and A146T variants are located in exon 5 near the hypervariable region (HVR) (residues ∼167–188) (Figure 1B). The NRAS Q61K and R102Q variants are within the G domain (residues ∼1–165), with Q61K in exon 3 and the S2 domain, and R102Q in exon 4, not associated with other domains (Johnson et al., 2017) (Figure 1C).
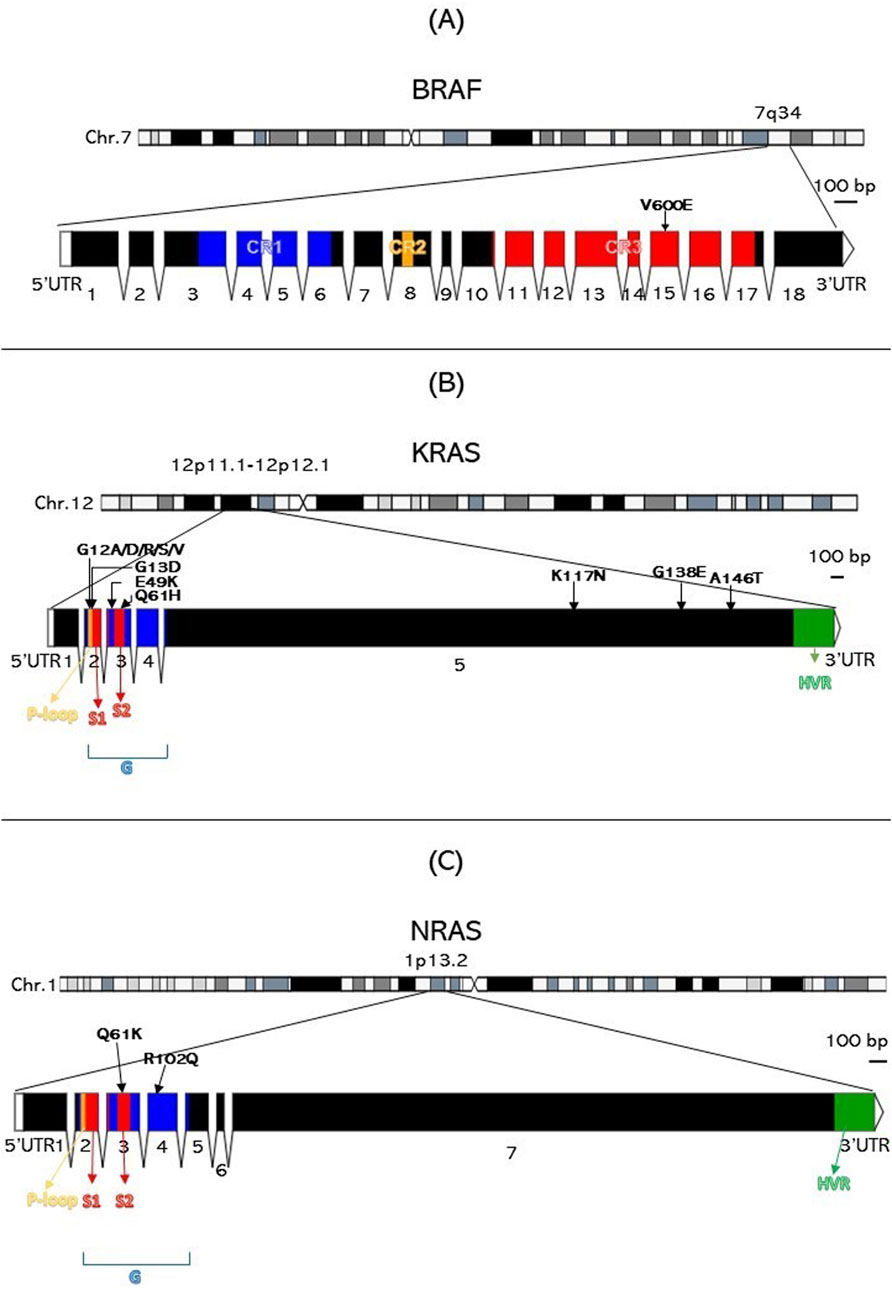
Figure 1. Genomic architecture and variant mapping for BRAF, KRAS, and NRAS. This figure is divided into three parts: (A) for BRAF, (B) for KRAS, and (C) for NRAS, each illustrating the chromosome location, exon structure, functional domains, and the specific locations of the 14 variants identified in the study. HVR, Hypervariable region. Numbers indicate exons.
3.4 Pathogenicity predictions
Our pathogenicity prediction analysis was performed on the 14 variants using two sets of tools: 7 CancerVar tools and our 7 study-selected tools. The output was evaluated based on the 0.5 threshold (>0.5 = pathogenic and <0.5 = benign). For each set, we consider a variant to be pathogenic if >3 tools scored above the threshold, while we consider the variant to be benign if ≤3 tools scored above the threshold. Between the 7 CancerVar tools and our 7 selected tools, with better prediction algorithms, there was great variability in pathogenicity predictions. CancerVar tools predicted three variants—KRAS G12S, E49K, and NRAS R102Q—to be benign (42.85%, 28.57%, and 28.57, respectively), but our tools identified them as pathogenic (100%, 85.71%, and 100%, respectively).
KRAS G12S, predicted benign by 3 CancerVar tools (SIFT, GERP++RS, and PolyPhen2_HDIV), and other 4 tools considered it as pathogenic. But all selected tools (100%) predicted it as pathogenic. Similarly, KRAS E49K was predicted as benign by CancerVar (2 tools: SIFT, GERP++RS) but pathogenic by 6 tools (85.71%) in our study (MPC, BayesDel_addAF, REVEL, FATHMM-MKL, PrimateAI, DANN). Lastly, NRAS R102Q, predicted benign by CancerVar (2 tools: SIFT, GERP++RS), was considered pathogenic by all 7 tools (100%) in our study (MPC, BayesDel_addAF, CADD_Phred, REVEL, FATHMM-MKL, PrimateAI, DANN). All other variants (BRAF V600E, KRAS G12A, G12D, G12R, G12V, G13D, Q61H, K117N, G138E, A146T, NRAS Q61K) were predicted as pathogenic by both sets of tools (Tables 2, 3).
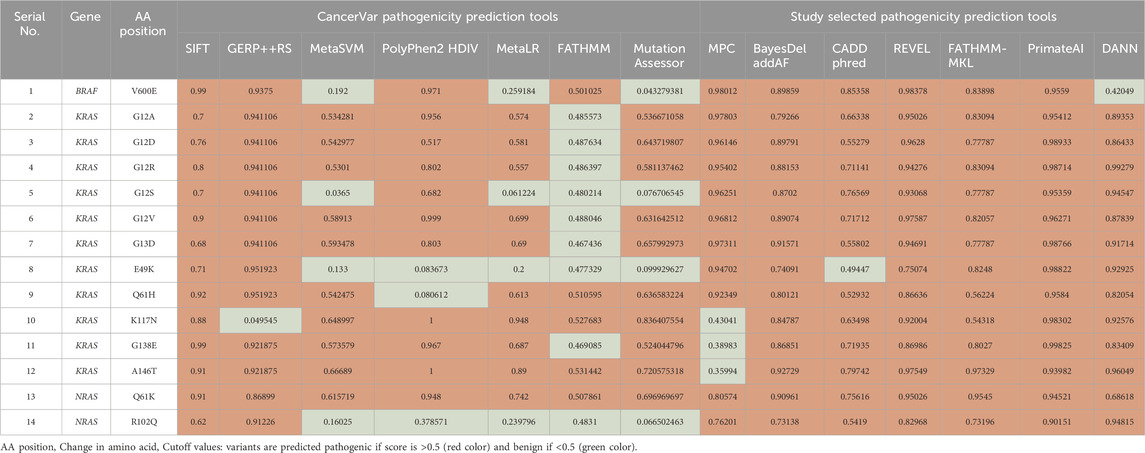
Table 2. Pathogenicity prediction analysis: comparison of pathogenicity prediction scores generated by 2 different sets of tools (CancerVar tools versus study selected tools) for 14 missense variants identified in CRC patients.
ROC curves can be a good indicator of the performance of different pathogenicity prediction tools. By plotting the true positive and true negative rates, area under the curve (AUC) can be calculated. A perfect performance for a given tool is 1, and unreliable tools score 0.5 or below. Upon comparison in ROC analysis, CancerVar’s 7 tools (SIFT, GERP++RS, MetaSVM, PolyPhen2_HDIV, MetaLR, FATHMM, MutationAssessor) and our 7 selected tools (MPC, BayesDel_addAF, CADD_Phred, REVEL, FATHMM-MKL, PrimateAI, DANN) displayed wide variations in terms of performance (Figure 2). Five of the selected tools (PrimateAI, BayesDel_addAF, REVEL, FATHMM-MKL, and CADD_Phred) demonstrated outstanding performance, with AUC values above 0.90 (0.98292, 0.96854, 0.92479, 0.90957, and 0.90922, respectively). Additionally, DANN showed a slightly lower AUC value (0.87218), which also indicates a high performance. MPC was the least performing among this group with an AUC of 0.72212. Therefore, the top six performing prediction tools in this analysis (PrimateAI, BayesDel_addAF, REVEL, FATHMM-MKL, CADD_Phred, and DANN) were among our study-selected tools. CancerVar tools showed lower AUC values for GERP++RS, SIFT, and Mutation Assessor (0.84312, 0.83093, and 0.80124, respectively) compared to the top 6 performing selected tools. FATHMM and MetaSVM had even lower AUC values (0.77022, 0.73343, respectively) but were still better than the selected MPC tool. MetaLR and PolyPhen2_HDIV were the least performing among all other tools, with AUC values below 0.70 (0.63529 and 0.44089, respectively). All results were statistically significant (p-value <0.05) (Table 4). Overall, our selected tools, with an average AUC of 0.89848, significantly improved prediction ability by approximately 24.4% compared to CancerVar tools, which had an average AUC of 0.72216.3.5.
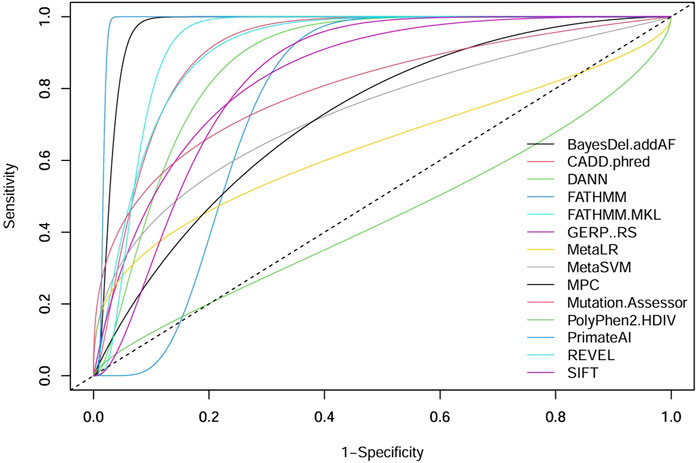
Figure 2. Comparative performance of 14 pathogenicity prediction tools. Two different sets of pathogenicity prediction tools, each containing 7 tools are compared to demonstrate their ability to detect pathogenic from benign variants. One set (SIFT, GERP++RS, MetaSVM, PolyPhen2 HDIV, MetaLR, FATHMM, and Mutation Assessor) are used by the CancerVar somatic variant interpretation tool, and the other set (MPC, BayesDel addAF, CADD phred, REVEL, FATHMM-MKL, PrimateAI, and DANN) are selected by our study to be used as a better predictor for pathogenicity.
3.5 Secondary structure, stability, and structural deviation analyses
Secondary structure analysis determines how amino acid changes in the protein may affect the size or shape of secondary structure elements (α-helices, ß-pleated sheets, and loops). Interestingly, the output generated by the NetSurfP 3.0 tool showed that 12 variants are falling within loop regions without notable changes in length or shape of the loops (BRAF V600E, KRAS G12A, G12D, G12R, G12S, G12V, G13D, Q61H, K117N, G138E, A146T, and NRAS Q61K). KRAS E49K variant falls within a ß-pleated sheet region, and NRAS R102Q within an α-helix. Both of these variants did not cause any visible change in their respective secondary structure elements (Supplementary Figure 1).
Thermodynamic stability analysis predicts the impact of mutations on protein folding. Variants making changes to protein thermodynamic stability are predicted to affect protein function. Actionable gene missense variants were analyzed using different measurements to estimate changes in energy. The DUET webtool combines the output of two different tools, mutation cutoff scanning matrix (mCSM) and site-directed mutator (SDM), to make its final prediction. DUET webserver predicted the BRAF V600E variant to be destabilizing the protein structure due to the negative free energy value (−2.118 kcal/mol). Seven KRAS variants were also shown to have a destabilizing effect: G12D (−0.323 kcal/mol), G12R (−0.044 kcal/mol), G12S (−0.274 kcal/mol), G13D (−0.461 kcal/mol), K117N (−0.455 kcal/mol), G138E (−1.28 kcal/mol), and A146T (−0.737 kcal/mol). Other 4 KRAS variants were found to further stabilize the protein structure due to the positive energy change: G12A (0.056 kcal/mol), G12V (0.168 kcal/mol), E49K (0.063 kcal/mol), and Q61H (0.256 kcal/mol). Both NRAS variants were also found to cause increased protein stability: Q61K (0.486 kcal/mol) and R102Q (0.012 kcal/mol). Compared to other analyzed variants, BRAF V600E was shown to be severely destabilizing the protein structure due to its large negative value, and NRAS R102Q to be weakly stabilizing its protein due to the small positive value (Table 5).
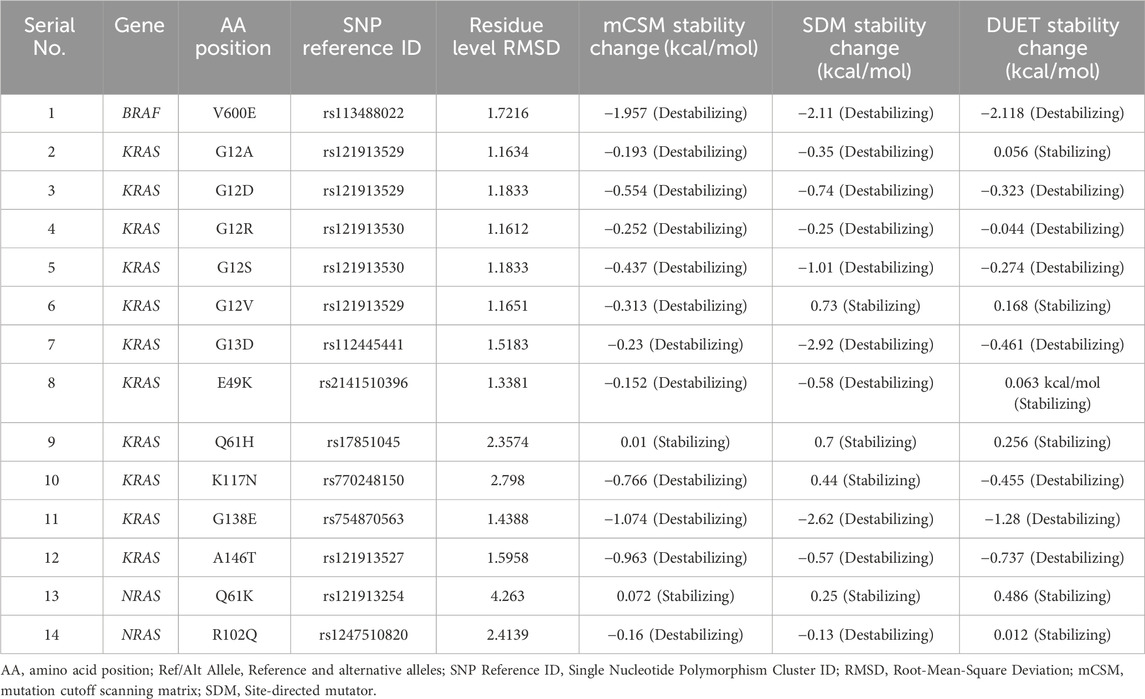
Table 5. RMSD and thermodynamic stability scores of 14 missense variants identified in CRC patients.
The 3D structure superimposition of the folded mutant proteins on their wild-type counterparts was performed to estimate deviations at the amino acid level. Three variants revealed significant structural deviations (>2Å) by RMSD analysis scores. These were KRAS Q61H (2.3574 Å), K117N (2.798 Å), and NRAS R102Q (2.4139 Å). The remaining 11 variants fell below the 2Å threshold (BRAF V600E, KRAS G12A, G12D, G12R, G12S, G12V, G13D, E49K, G138E, A146T, and NRAS Q61K) (Table 5). Overall, the superimposed 3D structures of all 14 variants showed subtle changes at residue level (Figure 3).
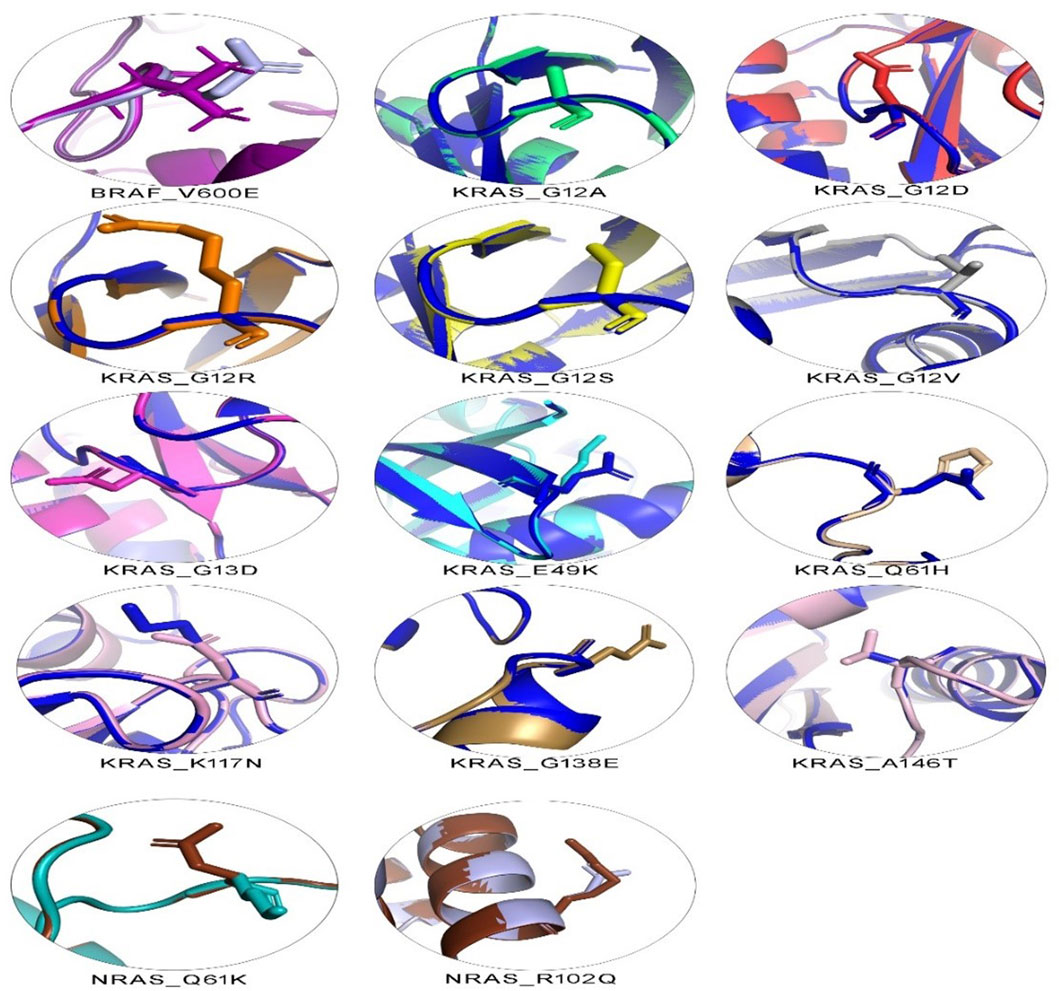
Figure 3. 3D structural analysis of variant and wild-type BRAF, KRAS, and NRAS proteins. The figure shows 14 variant amino acid 3D structures superimposed on their wild-type counterparts. Each superimposed structure highlights the effect of its respective mutation on protein shape. Contrasting colors are shown for each variant and wild-type pair. Wild-type BRAF is shown in dark purple, wild-type KRAS in dark blue, and wild-type NRAS in dark brown.
3.6 Computational binding of BRAF with Encorafenib
BRAF is a promising drug target for mCRC patients with the V600E mutation. Moreover, Encorafenib (a kinase inhibitor) can be used as a targeted therapy for those patients as a second- or third-line treatment option. To catch a glimpse of the efficacy of this drug, molecular docking was performed to estimate the binding affinity of this kinase inhibitor against wild-type BRAF protein and its V600E variant. The binding affinity between Encorafenib and wild-type BRAF protein was computed to −5.915 kcal/mol. It is predicted to form hydrogen bonds with two residues, D742 and A762, in the protein. However, the BRAF V600E mutant protein showed lower binding affinity with Encorafenib, with a computed value of −3.539 kcal/mol and one hydrogen bond at residue Y746 of the protein (Figure 4).
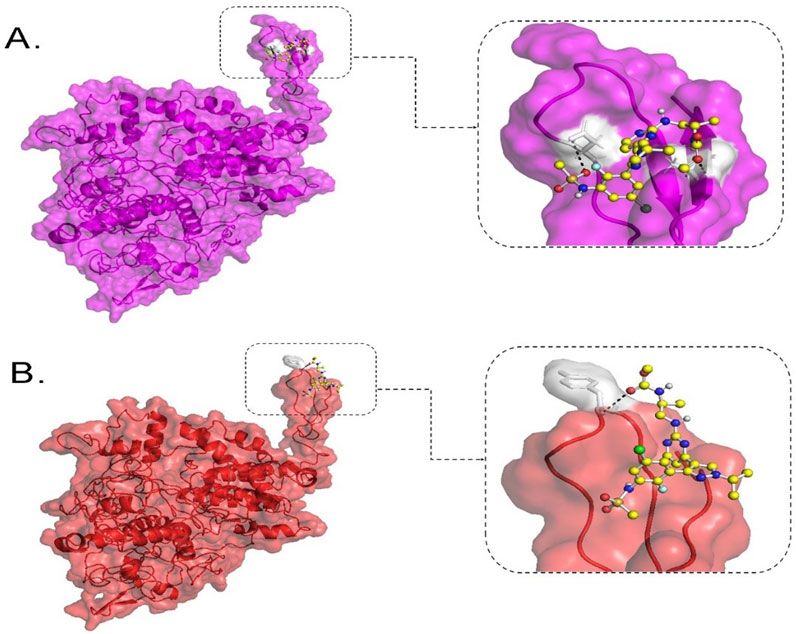
Figure 4. Molecular docking analysis of BRAF protein with Encorafenib. This figure shows the binding configuration of wild-type BRAF (A) and BRAF V600E variant protein (B) with Encorafenib. Encorafenib is depicted as a small molecule in a ball-and-stick illustration, with white shaded pockets indicating hydrogen bond formation sites. The dotted lines represent hydrogen bonds. In (A), the wild-type BRAF protein is shown in purple on the left, with an enlarged view of the protein-ligand interaction site on the right. In (B), the BRAF V600E variant protein is shown in red on the left, with an enlarged view of the protein-ligand interaction site on the right.
4 Discussion
CRC is a challenging disease. Twenty percent of patients present at a metastatic stage and 50% of localized cases progress to mCRC (Dekker et al., 2019). Despite the genetic heterogeneity of CRC tumors, targeted treatments remain limited. BRAF, KRAS, and NRAS express vital cascade signaling proteins that act in the RAS-RAF-MEK-MAPK pathway, ultimately influencing the cell cycle G1 checkpoint. While cell commitment to DNA synthesis is largely controlled by retinoblastoma tumor suppressor protein (Rb), mutations in these signaling proteins can promote cell cycle progression from G1 to S phase by increasing expression of cyclins and cyclin-dependent kinases that are known to nullify the effects of Rb. Such mutations in these actionable genes disrupt normal cell cycle regulation, leading to uncontrolled proliferation and carcinogenesis (Sherr, 1995; Smalley et al., 2008; Puyol et al., 2010). In this study, we collected 86 samples from KAUH hospital: 40 (46.5%) were positive and the rest were negative for mutations in these actionable genes. Fourteen variants were identified through molecular screening and raw DNA sequence analysis: 1 in BRAF, 11 in KRAS, and 2 in NRAS, including NRAS R102Q, previously unreported in CRC data. BRAF mutations, particularly the V600E variant, occur in 66% of melanomas and less frequently in other cancers (OMIM:164757). Mutations in BRAF codon 600, KRAS codons 12 and 13, and NRAS codon 61 confer resistance to anti-EGFR treatments, while BRAF V600E mutations indicate aggressive disease but potential benefit from VEGF inhibitors (Ciardiello et al., 2022). This variant disrupts kinase activity, increasing signaling independently of RAS, with a 138-fold rise in oncogenic activity (Oikonomou et al., 2014). It is a key biomarker in thyroid (Koh et al., 2013), melanoma (Cheng et al., 2018), and CRC (Ciardiello et al., 2022). BRAF mutations are present in 8%–12% of European mCRC patients and associated with poor survival (Van Cutsem et al., 2016; Tabernero et al., 2022). In this study, CancerVar identified this variant as Tier I (Table 1), consistent with ClinVar (RCV001030023.12). The frequency of BRAF mutations in our data is 2.32% (Table 1), aligning with Saudi data (0.4%–4%) (Siraj et al., 2014; Beg et al., 2015; Al-Shamsi et al., 2016; Alharbi et al., 2021; Alfahed, 2023).
The BRAF gene, located at chromosome 7q34, consists of 18 exons encoding a 766-amino acid protein (Huang et al., 2013). BRAF is a serine/threonine kinase that performs several functions in the RAS-RAF-MEK-MAPK pathway (Rauch et al., 2011). Under physiological conditions, growth factors activate RAS proteins at the cell membrane, which then activate RAF proteins, thus amplifying the signal. Balanced regulatory signals autoinhibit RAF as a means of turning off growth signals when not needed. BRAF V600E, a type 1 mutation, functions as a monomer, increasing signaling without RAS dimerization and resisting regulated inhibition (Ciombor et al., 2022). BRAF protein has three conserved domains: CR1, CR2, and CR3. CR1 (residues 120–280), near the N-terminus, includes cysteine-rich and RAS-binding (residues 155–227) subdomains. The cysteine-rich subdomain controls kinase activity by autoinhibition (Cutler et al., 1998; Zaman et al., 2019), while the RAS-binding domain upregulates BRAF at the plasma membrane (Freeman et al., 2013). CR2, a serine/threonine-rich region, links CR1 and CR3 and contains a 14-3-3 scaffold binding site. CR3 (residues 457–717) (Zaman et al., 2019), near the C-terminus, is a kinase-binding domain that activates upon phosphorylation, comprising a glycine-rich ATP-phosphate binding lobe (residues 462–469) and a longer C-terminal lobe (residues 593–623) (Śmiech et al., 2020; Maloney et al., 2021). The most common mutation, V600E, is situated in the CR3 region of BRAF, disrupting its autoinhibition mechanism, hence contributing to oncogenic activity due to destabilization within the inactive form of BRAF (Park et al., 2019).
In our secondary structure analysis, we predicted that BRAF V600E falls into a loop structure identified by Park et al. (2019), as the inhibitory loop (Park et al., 2019). Loops are one important determinant in the stability and functions of proteins. Superimposing BRAF V600E on its wild-type counterpart gave a high RMSD value of 1.7216; DUET thermodynamic stability analysis also predicted that this mutation would highly be destabilizing, with a value of −2.118 kcal/mol. The results predict that this missense variant will have a profound impact on the structure of the protein and severely disrupt normal function. Computational binding assays for Encorafenib with BRAF wild-type and its V600E variant in Figure 3 showed differential binding behavior. The binding energy of the wild-type-Encorafenib complex was −5.915 kcal/mol, displaying two hydrogen interactions at D742 and A762. By contrast, V600E-Encorafenib had only one hydrogen bond interaction at Y746 shown with a binding energy of −3.509 kcal/mol for the conformational pose. With this low binding energy score with two hydrogen bond interactions in the case of a wild-type-Encorafenib complex, it indicates that the wild-type protein binds with the inhibitor very tightly and, hence, is effective under normal conditions. The V600E-Encorafenib has a higher binding energy score and, therefore, a weaker binding affinity, though it is still predicted to be able to inhibit its target protein. Both complexes interacted in proximity to the residues expected to interact with the inhibitors near the C-tail inhibition binding sites, F743 and A749, respectively (Martinez Fiesco et al., 2022), and both complexes interacted nearby. Our results indicate that Encorafenib may have a reduced efficacy in V600E variant proteins. The lower inhibition ability of Encorafenib in patients with the V600E variant could be a factor explaining why these patients may not respond to monotherapy, and often require a combination with other drugs such as Cetuximab (an EGFR inhibitor), and Binimetinib a (MEK inhibitor) to gain benefit from the treatment. It is because blocking the BRAF activity causes an over activation of EGFR, the latter must also be inhibited to gain a better response (Kopetz et al., 2019). Therefore, Encorafenib might be better at targeting wildtype BRAF proteins whether blocking this member of the pathway is needed in designing a new drug regimen for patients without the V600E variant. KRAS and NRAS are gene homologs belonging to the RAS superfamily, which play crucial roles in the RAS-RAF-MEK-MAPK signaling pathways (Fernández-Medarde and Santos, 2011) and PI3K-AKT-mTOR pathways (Castellano and Downward, 2011). Approximately 19% of cancer patients develop a RAS mutation, including approximately 3.4 million new cases yearly that are positive for RAS. Moreover, 75% of mutated RAS isoforms are KRAS (Prior et al., 2020). KRAS mutations occur in 11.6% of all cancer types, as per TCGA data. They are mostly prevalent in pancreatic ductal adenocarcinoma (PDAC) (81.72%), CRC (37.97%), and non-small cell lung cancer (NSCLC) (21.20%) (Yang et al., 2023). In mCRC patients, ∼60% have KRAS mutations (Peeters et al., 2015), primarily in codons 12, 13, 61, 117, and 146 (Serebriiskii et al., 2019). Over 90% of KRAS mutations occur at codons 12 and 13, part of the p-loop of the GTP binding domain, with codon 12 being more associated with oncogenesis and higher mortality than codon 13 (Colussi et al., 2013). The most prevalent KRAS mutations in CRC are G12D (28.04%), followed by G12V (18.50%) and G13D (18.10%), according to http://cBioPortal.org (Yang et al., 2023). Among the 86 samples in our study, 18.60% carried the G12D mutation, 5.81% carried the G12V mutation, and 3.48% carried the G13D, G12A, and G138E mutations. Additionally, 2.32% of samples had K117N and A146T mutations, while 1.16% of samples had G12R, G12S, E49K, or Q61H mutations. Except for E49K and G138E, all identified KRAS mutations are within hotspots. KRAS mutations have been reported in Saudi Arabia in the past at a frequency of 28.6%–56%, with G12D and G12V being the most prevalent variants (Siraj et al., 2014; Beg et al., 2015; Al-Shamsi et al., 2016; Alfahed, 2023). In comparison, our data demonstrated ∼10% lower incidences of G12D than international and regional studies, which may be related to the limited sample size. Surprisingly, G138E, a rare variant that was only reported in COSMIC twice from large intestine samples, was detected in three cases and thus could be more common in Saudi or Arab CRC patients. One patient carried a rare KRAS mutation (E49K), which was concomitantly found with another KRAS mutation (G138E). It was reported only twice so far from large intestine samples in COSMIC. CancerVar has classified G12D as Tier I, strong clinical significance, and nine variants as Tier II, potential clinical significance: G12A; G12R; G12S; G12V; G13D; Q61H; K117N; G138E; and A146T. E49K was classified as Tier III with unknown clinical significance. The clinical significance of these variants aligns with expectations, except for G138E and E49K, which lack sufficient clinical data.
In the cell, KRAS is expressed in two isoforms (KRAS-4B and KRAS-4A) due to alternative splicing of the exon 4. The term KRAS refers to the KRAS-4B transcript, as it is more highly expressed than KRAS-4A (Nuevo-Tapioles and Philips, 2022). The KRAS gene, located at chromosome 12p11.1-12p12.1, encodes a protein of 188 amino acids, divided into five exons (Huang et al., 2021). KRAS is a cascade signaling protein that is activated by GTP and deactivated by GDP, a process that needs to be balanced for normal protein function (Zhu et al., 2021). KRAS and NRAS share two main conserved domains. The GTP-binding (G) catalytic domain (residues 1–165) is highly conserved and identical in both RAS forms. It comprises two lobes: the effector lobe (residues 1–86) contains switch 1 (S1) (residues 30–38), switch 2 (S2) (residues 60–76), and the phosphate-binding region (P-loop) (residues 10–17), all essential for GTP binding. The allosteric lobe (residues 87–166) is less conserved, with 70%–80% similarity. The hypervariable region (HVR) (residues 167–188) at the C-terminus is the least conserved and crucial for post-translational modifications for membrane anchoring (Jancík et al., 2010; Zeitouni et al., 2016; Pantsar, 2020; Han et al., 2021; Nuevo-Tapioles and Philips, 2022). Our identified mutations in codons 12 and 13 (G12A, G12D, G12R, G12S, G12V, and G13D) are in the P-loop region of the G domain. These mutations disturb the active site’s geometry, preventing GTP hydrolysis and locking the RAS protein in its active state (Malumbres and Barbacid, 2003). The rare E49K and the Q61H mutations, located in exon 3, affect the G domain; Q61H is in the S2 region, potentially disrupting GTPase activity by blocking GTP transition to GDP. Mutations K117N, G138E, and A146T are in exon 5, near the HVR. K117N and A146T, found in hotspot codons, are known to predict resistance to Cetuximab plus Irinotecan (Loupakis et al., 2009). Codon 146 mutations occur in ∼4% of CRCs and are more common than codon 61 mutations (Edkins et al., 2006). Functional studies on Q61H and K117N show increased proliferation and high levels of GTP-bound KRAS, indicating they affect GTPase activity by maintaining the active form (Stolze et al., 2015). G138E is a rare mutation located near the HVR with no current functional data. Secondary structure analysis of KRAS variants revealed all variants were in loop regions except E49K, which is on a ß-pleated sheet. No obvious changes were seen in secondary structure elements, except an increased loop size in the G138E mutant (Supplementary Figure 1). Loop regions are important for protein stability, and mutations can disturb this stability. E49K showed no obvious effects. Superimposing KRAS mutant and wild-type forms revealed significant alterations at affected residues for all variants (Figure 3), with deviations ranging from 1.1634 to 1.5958, except for Q61H and K117N, which showed higher deviations (2.3574 and 2.789, respectively). These deviations likely affect the binding site geometry, particularly at codon 12, where sidechain addition disturbs GTP hydrolysis complex binding. Q61H and K117N significantly disrupt the amino acid structure, altering overall protein function and GTPase activity. Protein stability analysis indicated seven KRAS variants to destabilize the structure (G12D, G12R, G12S, G13D, K117N, G138E, A146T). Other four variants further stabilize the structure (G12A, G12V, E49K, and Q61H). The increased or decreased free energy values demonstrate the influence of these mutations on protein stability. A146T and K117N are highly destabilizing, predicting decreased catalytic ability, while Q61H is highly stabilizing. E49K weakly increases stability, demonstrating low impact on function.
NRAS is a homolog to KRAS, with similar protein structure and function (Colicelli, 2004). NRAS mutations occur in 3.03% of all cancers (Consortium et al., 2017), predominantly in skin (10%–25%) (Jakob et al., 2012), acute myeloid leukemia (9.76%) (Consortium et al., 2017), and colon (∼4%) (Cicenas et al., 2017). In CRC, the majority of NRAS mutations occur at codons 12, 13, and 61, with codon 61 being the most frequently mutated (Vaughn et al., 2011). In this study, two NRAS variants were identified, Q61K and R102Q (2.32%). Previous studies from Saudi Arabia have reported NRAS variants occurring at a rate of 2%–4% (Al-Shamsi et al., 2016; Alharbi et al., 2021; Alfahed, 2023). Our findings align with this but are 2% lower than the international CRC prevalence. The R102Q variant, although present in low VAF, is considered novel and has not been previously reported in CRC. The low VAF suggests it could be an artifact, present in a small subset of cancer cells, or with potential biological relevance (i.e., low- frequency driver mutations arising in later-stage subclones). CancerVar classifies Q61K as Tier II—potential clinical significance, as expected. R102Q is predicted to be benign by CancerVar pathogenicity prediction tools and is classified as a Tier III variant of unknown significance, though our study’s selected tools suggest it is pathogenic. Therefore, its true pathogenicity remains to be functionally tested for confirmation. NRAS is located at chromosome 1p13.2 (Mitchell et al., 2008) and encodes a protein of 189 amino acids across seven exons (Eisfeld et al., 2014). The Q61K variant is mapped to the S2 region of exon 3, within the G catalytic domain. Due to this location, it is expected to affect NRAS proteins by disrupting GTP hydrolysis, thus activating the protein. R102Q is located in exon 4, also a part of the catalytic domain. Therefore, it is predicted to similarly influence the catalytic site’s conformation. 3D structural modeling showed significant deviations between the variant and wild-type structures of Q61K (4.263) and R102Q (2.4139). Both variants increased protein stability (Q61K: 0.486; R102Q: 0.012). Q61K likely makes the G domain more rigid, altering the binding site, while R102Q’s impact on G domain function cannot be predicted based on our data.
We compared our selected tools with better algorithms against CancerVar tools to make accurate predictions for identified somatic variants. Our pathogenicity prediction tools showed a significant difference against CancerVar tools. Variants such as KRAS G12S, E49K, and NRAS R102Q were predicted as benign by CancerVar but pathogenic by our tools. The other variants were consistently identified as pathogenic (Tables 2, 3). KRAS G12S, located at codon 12, is expected to confer drug resistance due to increased activation. CancerVar’s tools mostly predicted it as benign, while our tools as pathogenic. ROC analysis demonstrated the superior performance of 6/7 (85%) of our selected tools (PrimateAI, BayesDel_addAF, REVEL, FATHMM-MKL, CADD_Phred). Significant z-scores further supported these findings, highlighting tool performance variability. Overall, study-selected tools outperformed CancerVar tools, probably due to the advanced machine learning algorithms these tools use to integrate diverse data sources. The best-performing tools in this study rely on the more complex algorithms such as deep learning, multiple kernel learning, support vector machines, deep neural networks, and advanced Bayesian statistical frameworks (PrimateAI, REVEL, FATHMM-MKL, CADD_Phred, DANN, and BayesDel_addAF, respectively). They also incorporate additional features, such as evolutionary conservation, functional annotations, and population allele frequencies, providing different angles of prediction. By comparison, the CancerVar tools SIFT, FATHMM, and PolyPhen2_HDIV are older and employ simpler algorithms and most likely out-of-date training data. Being foundational models, their much lower complexity may be affecting their accuracy relative to newer tools. The newer tools also have the largest and most diverse training datasets, covering more variant types and contexts. This study emphasizes that advanced and diverse prediction tools, when possible, must be used in combination for comprehensive variant assessment. It is, however, paramount to complement such predictions with clinical or functional evidence for the correct determination of pathogenicity for a somatic variant.
5 Conclusion
In conclusion, our study, one of the few from Saudi Arabia, supports pathogenicity for some known variants and predicts the pathogenicity of other rare variants. Functional studies, however, are needed for validation. We reported on the prevalence of somatic variants from CRC patients in a Saudi hospital, identifying 14 variants in actionable genes, including one novel variant (NRAS R102Q) and two rare variants (KRAS E49K and G138E). KRAS G138E, present in three patients, was previously reported only twice from large intestine samples and might be more common in Saudi or Middle Eastern populations. The KRAS E49K co-occurred with KRAS G138E, while the NRAS R102Q co-occurred with KRAS G12D. Wild-type BRAF proteins showed higher binding affinity to Encorafenib, suggesting more effective inhibition compared to the variant protein V600E. Our genotype-protein-phenotype analyses emphasized the pathogenicity of identified variants, highlighting the necessity of effective targeted therapies. Whether the identified rare or novel variants were functionally shown to be activating their respective proteins, clinical decisions can be made to tailor treatment for patients harboring these variants. The prevalence of somatic variants in Saudi Arabia within the BRAF, KRAS, and NRAS genes is roughly 47.7%, which is consistent with global estimates. As cell cycle modulators, these genes continue to be attractive therapeutic targets. Overall, this study highlights the significance of comprehensive molecular screening and bioinformatics in understanding the mutational landscape of CRC in the Saudi population, with the goal of improving targeted drug therapy.
Data availability statement
The raw data supporting the conclusions of this article will be made available by the authors, without undue reservation.
Ethics statement
The studies involving humans were approved by King Abdulaziz University College of Medicine Ethical Committee. The studies were conducted in accordance with the local legislation and institutional requirements. The human samples used in this study were acquired from a by-product of routine care or industry. Written informed consent for participation was not required from the participants or the participants’ legal guardians/next of kin in accordance with the national legislation and institutional requirements.
Author contributions
TA: Data curation, Formal Analysis, Visualization, Writing–original draft, Writing–review and editing. NS: Conceptualization, Methodology, Supervision, Validation, Writing–review and editing. RA: Data curation, Investigation, Resources, Writing–review and editing. RB: Data curation, Project administration, Resources, Writing–review and editing. MA-M: Project administration, Resources, Writing–review and editing. HA: Validation, Writing–review and editing. NA-R: Methodology, Writing–review and editing. AE-H: Supervision, Writing–review and editing. RE: Conceptualization, Writing–review and editing. BB: Writing–review and editing, Methodology, Supervision. ZA: Writing–review and editing.
Funding
The author(s) declare that financial support was received for the research, authorship, and/or publication of this article. This publication is based upon work supported by the Khalifa University (KU) and King Abdulaziz University (KAU) Join Research Program Award No. KAUKUJRP-1M-2021.
Conflict of interest
The authors declare that the research was conducted in the absence of any commercial or financial relationships that could be construed as a potential conflict of interest.
Publisher’s note
All claims expressed in this article are solely those of the authors and do not necessarily represent those of their affiliated organizations, or those of the publisher, the editors and the reviewers. Any product that may be evaluated in this article, or claim that may be made by its manufacturer, is not guaranteed or endorsed by the publisher.
Supplementary material
The Supplementary Material for this article can be found online at: https://www.frontiersin.org/articles/10.3389/fphar.2024.1498295/full#supplementary-material
SUPPLEMENTARY FIGURE 1 | Secondary structure analysis for BRAF, KRAS, and NRAS variant proteins; (A–C), respectively. For each variant protein, the upper segment of the comparison illustrates the wild-type secondary structure (WT), while the lower segment displays the variant secondary structure.
References
Adzhubei, I., Jordan, D. M., and Sunyaev, S. R. (2013). Predicting functional effect of human missense mutations using PolyPhen-2. Curr. Protoc. Hum. Genet. Chapter 7, Unit7.20. doi:10.1002/0471142905.hg0720s76
Alfahed, A. (2023). Molecular pathology of colorectal cancer: the Saudi situation in perspective. Saudi Med. J. 44 (9), 836–847. doi:10.15537/smj.2023.44.9.20230257
Alharbi, A., Bin Dokhi, H., Almuhaini, G., Alomran, F., Masuadi, E., and Alomran, N. (2021). Prevalence of colorectal cancer biomarkers and their impact on clinical outcomes in Riyadh, Saudi Arabia. PLoS One 16 (5), e0249590. doi:10.1371/journal.pone.0249590
Al-Shamsi, H. O., Jones, J., Fahmawi, Y., Dahbour, I., Tabash, A., Abdel-Wahab, R., et al. (2016). Molecular spectrum of KRAS, NRAS, BRAF, PIK3CA, TP53, and APC somatic gene mutations in Arab patients with colorectal cancer: determination of frequency and distribution pattern. J. Gastrointest. Oncol. 7 (6), 882–902. doi:10.21037/jgo.2016.11.02
Andre, F., Nowak, F., Arnedos, M., Lacroix, L., Viens, P., and Calvo, F. (2012). Biomarker discovery, development, and implementation in France: a report from the French National Cancer Institute and cooperative groups. Clin. Cancer Res. 18 (6), 1555–1560. doi:10.1158/1078-0432.Ccr-11-2201
Beg, S., Siraj, A. K., Prabhakaran, S., Bu, R., Al-Rasheed, M., Sultana, M., et al. (2015). Molecular markers and pathway analysis of colorectal carcinoma in the Middle East. Cancer 121 (21), 3799–3808. doi:10.1002/cncr.29580
Biller, L. H., and Schrag, D. (2021). Diagnosis and treatment of metastatic colorectal cancer: a review. Jama 325 (7), 669–685. doi:10.1001/jama.2021.0106
Bray, F., Laversanne, M., Sung, H., Ferlay, J., Siegel, R. L., Soerjomataram, I., et al. (2024). Global cancer statistics 2018: GLOBOCAN estimates of incidence and mortality worldwide for 36 cancers in 185 countries. CA Cancer J. Clin. 68, 394–424. doi:10.3322/caac.21492
Bugnon, M., Röhrig, U. F., Goullieux, M., Perez, M. A. S., Daina, A., Michielin, O., et al. (2024). SwissDock 2024: major enhancements for small-molecule docking with attracting cavities and AutoDock Vina. Nucleic Acids Res. 52, W324–W332. doi:10.1093/nar/gkae300
Castellano, E., and Downward, J. (2011). RAS interaction with PI3K: more than just another effector pathway. Genes Cancer 2 (3), 261–274. doi:10.1177/1947601911408079
Chang, X., and Wang, K. (2012). wANNOVAR: annotating genetic variants for personal genomes via the web. J. Med. Genet. 49 (7), 433–436. doi:10.1136/jmedgenet-2012-100918
Chen, Y., Liu, L., Li, J., Jiang, H., Ding, C., and Zhou, Z. (2023). “MetaLR: meta-tuning of learning rates for transfer learning in medical imaging,” in Medical image computing and computer assisted intervention – MICCAI 2023. Editors H. Greenspan, A. Madabhushi, P. Mousavi, S. Salcudean, J. Duncan, T. Syeda-Mahmoodet al. (Springer Nature Switzerland), 706–716.
Cheng, L., Lopez-Beltran, A., Massari, F., MacLennan, G. T., and Montironi, R. (2018). Molecular testing for BRAF mutations to inform melanoma treatment decisions: a move toward precision medicine. Mod. Pathol. 31 (1), 24–38. doi:10.1038/modpathol.2017.104
Ciardiello, F., Ciardiello, D., Martini, G., Napolitano, S., Tabernero, J., and Cervantes, A. (2022). Clinical management of metastatic colorectal cancer in the era of precision medicine. CA A Cancer J. Clin. 72 (4), 372–401. doi:10.3322/caac.21728
Cicenas, J., Tamosaitis, L., Kvederaviciute, K., Tarvydas, R., Staniute, G., Kalyan, K., et al. (2017). KRAS, NRAS and BRAF mutations in colorectal cancer and melanoma. Med. Oncol. 34 (2), 26. doi:10.1007/s12032-016-0879-9
Ciombor, K. K., Strickler, J. H., Bekaii-Saab, T. S., and Yaeger, R. (2022). BRAF-mutated advanced colorectal cancer: a rapidly changing therapeutic landscape. J. Clin. Oncol. 40 (24), 2706–2715. doi:10.1200/jco.21.02541
Colicelli, J. (2004). Human RAS superfamily proteins and related GTPases. Sci. STKE 2004 (250), Re13. doi:10.1126/stke.2502004re13
Colussi, D., Brandi, G., Bazzoli, F., and Ricciardiello, L. (2013). Molecular pathways involved in colorectal cancer: implications for disease behavior and prevention. Int. J. Mol. Sci. 14 (8), 16365–16385. doi:10.3390/ijms140816365
Consortium, A. P. G., Consortium, A. P. G., André, F., Arnedos, M., Baras, A. S., Baselga, J., et al. (2017). AACR Project GENIE: powering precision medicine through an international consortium. Cancer Discov. 7 (8), 818–831. doi:10.1158/2159-8290.CD-17-0151
Cope, N., Candelora, C., Wong, K., Kumar, S., Nan, H., Grasso, M., et al. (2018). Mechanism of BRAF activation through biochemical characterization of the recombinant full-length protein. Chembiochem 19 (18), 1988–1997. doi:10.1002/cbic.201800359
Cutler, R. E., Stephens, R. M., Saracino, M. R., and Morrison, D. K. (1998). Autoregulation of the Raf-1 serine/threonine kinase. Proc. Natl. Acad. Sci. U. S. A. 95 (16), 9214–9219. doi:10.1073/pnas.95.16.9214
Davydov, E. V., Goode, D. L., Sirota, M., Cooper, G. M., Sidow, A., and Batzoglou, S. (2010). Identifying a high fraction of the human genome to be under selective constraint using GERP++. PLoS Comput. Biol. 6 (12), e1001025. doi:10.1371/journal.pcbi.1001025
Dekker, E., Tanis, P. J., Vleugels, J. L. A., Kasi, P. M., and Wallace, M. B. (2019). Colorectal cancer. Lancet 394 (10207), 1467–1480. doi:10.1016/s0140-6736(19)32319-0
Eberhardt, J., Santos-Martins, D., Tillack, A. F., and Forli, S. (2021). AutoDock Vina 1.2.0: new docking methods, expanded force field, and Python bindings. J. Chem. Inf. Model. 61 (8), 3891–3898. doi:10.1021/acs.jcim.1c00203
Edkins, S., O'Meara, S., Parker, A., Stevens, C., Reis, M., Jones, S., et al. (2006). Recurrent KRAS codon 146 mutations in human colorectal cancer. Cancer Biol. Ther. 5 (8), 928–932. doi:10.4161/cbt.5.8.3251
Eisfeld, A. K., Schwind, S., Hoag, K. W., Walker, C. J., Liyanarachchi, S., Patel, R., et al. (2014). NRAS isoforms differentially affect downstream pathways, cell growth, and cell transformation. Proc. Natl. Acad. Sci. U. S. A. 111 (11), 4179–4184. doi:10.1073/pnas.1401727111
Elwali, N. E., Jarrah, O., Alzahrani, S. G., Alharbi, M. B., Alhejaily, A. G., Alsharm, A. A., et al. (2023). Colorectal cancer in Saudi Arabia: the way forward. Asian Pac J. Cancer Prev. 24 (1), 13–19. doi:10.31557/apjcp.2023.24.1.13
Fearon, E. R., and Vogelstein, B. (1990). A genetic model for colorectal tumorigenesis. Cell 61 (5), 759–767. doi:10.1016/0092-8674(90)90186-i
Feng, B. J. (2017). PERCH: a unified framework for disease gene prioritization. Hum. Mutat. 38 (3), 243–251. doi:10.1002/humu.23158
Fernández-Medarde, A., and Santos, E. (2011). Ras in cancer and developmental diseases. Genes Cancer 2 (3), 344–358. doi:10.1177/1947601911411084
Freeman, A. K., Ritt, D. A., and Morrison, D. K. (2013). Effects of Raf dimerization and its inhibition on normal and disease-associated Raf signaling. Mol. Cell 49 (4), 751–758. doi:10.1016/j.molcel.2012.12.018
Gonzalez, D., Fearfield, L., Nathan, P., Tanière, P., Wallace, A., Brown, E., et al. (2013). BRAF mutation testing algorithm for vemurafenib treatment in melanoma: recommendations from an expert panel. Br. J. Dermatol 168 (4), 700–707. doi:10.1111/bjd.12248
Gupta, S., Mathur, M., Miao, B., Suravajhala, P., and Bandapalli, O. R. (2021). “Role of DNA mismatch repair genes in colorectal cancer,” in Colon cancer diagnosis and therapy. Editors N. K. Vishvakarma, G. P. Nagaraju, and D. Shukla (Cham: Springer International Publishing), 2, 209–223. doi:10.1007/978-3-030-64668-4_10
Han, C. W., Jeong, M. S., and Jang, S. B. (2021). Understand KRAS and the quest for anti-cancer drugs. Cells 10 (4), 842. doi:10.3390/cells10040842
Huang, L., Guo, Z., Wang, F., and Fu, L. (2021). KRAS mutation: from undruggable to druggable in cancer. Signal Transduct. Target. Ther. 6 (1), 386. doi:10.1038/s41392-021-00780-4
Huang, T., Karsy, M., Zhuge, J., Zhong, M., and Liu, D. (2013). B-Raf and the inhibitors: from bench to bedside. J. Hematol. and Oncol. 6 (1), 30. doi:10.1186/1756-8722-6-30
Huck, M. B., and Bohl, J. L. (2016). Colonic polyps: diagnosis and surveillance. Clin. Colon Rectal Surg. 29 (4), 296–305. doi:10.1055/s-0036-1584091
Ioannidis, N. M., Rothstein, J. H., Pejaver, V., Middha, S., McDonnell, S. K., Baheti, S., et al. (2016). REVEL: an ensemble method for predicting the pathogenicity of rare missense variants. Am. J. Hum. Genet. 99 (4), 877–885. doi:10.1016/j.ajhg.2016.08.016
Jakob, J. A., Bassett, R. L., Ng, C. S., Curry, J. L., Joseph, R. W., Alvarado, G. C., et al. (2012). NRAS mutation status is an independent prognostic factor in metastatic melanoma. Cancer 118 (16), 4014–4023. doi:10.1002/cncr.26724
Jancík, S., Drábek, J., Radzioch, D., and Hajdúch, M. (2010). Clinical relevance of KRAS in human cancers. J. Biomed. Biotechnol. 2010, 150960. doi:10.1155/2010/150960
Jasperson, K. W., Tuohy, T. M., Neklason, D. W., and Burt, R. W. (2010). Hereditary and familial colon cancer. Gastroenterology 138 (6), 2044–2058. doi:10.1053/j.gastro.2010.01.054
Johnson, C. W., Reid, D., Parker, J. A., Salter, S., Knihtila, R., Kuzmic, P., et al. (2017). The small GTPases K-Ras, N-Ras, and H-Ras have distinct biochemical properties determined by allosteric effects. J. Biol. Chem. 292 (31), 12981–12993. doi:10.1074/jbc.M117.778886
Keum, N., and Giovannucci, E. (2019). Global burden of colorectal cancer: emerging trends, risk factors and prevention strategies. Nat. Rev. Gastroenterol. Hepatol. 16 (12), 713–732. doi:10.1038/s41575-019-0189-8
Kim, S., Jhong, J. H., Lee, J., and Koo, J. Y. (2017). Meta-analytic support vector machine for integrating multiple omics data. BioData Min. 10, 2. doi:10.1186/s13040-017-0126-8
Koelblinger, P., Thuerigen, O., and Dummer, R. (2018). Development of encorafenib for BRAF-mutated advanced melanoma. Curr. Opin. Oncol. 30 (2), 125–133. doi:10.1097/cco.0000000000000426
Koh, J., Choi, J. R., Han, K. H., Kim, E. K., Yoon, J. H., Moon, H. J., et al. (2013). Proper indication of BRAF(V600E) mutation testing in fine-needle aspirates of thyroid nodules. PLoS One 8 (5), e64505. doi:10.1371/journal.pone.0064505
Kopetz, S., Desai, J., Chan, E., Hecht, J. R., O'Dwyer, P. J., Maru, D., et al. (2015). Phase II pilot study of vemurafenib in patients with metastatic BRAF-mutated colorectal cancer. J. Clin. Oncol. 33 (34), 4032–4038. doi:10.1200/jco.2015.63.2497
Kopetz, S., Grothey, A., Yaeger, R., Van Cutsem, E., Desai, J., Yoshino, T., et al. (2019). Encorafenib, Binimetinib, and cetuximab in BRAF V600e-mutated colorectal cancer. N. Engl. J. Med. 381 (17), 1632–1643. doi:10.1056/NEJMoa1908075
Land, H., and Humble, M. S. (2018). YASARA: a tool to obtain structural guidance in biocatalytic investigations. Methods Mol. Biol. 1685, 43–67. doi:10.1007/978-1-4939-7366-8_4
Li, J., Ma, X., Chakravarti, D., Shalapour, S., and DePinho, R. A. (2021). Genetic and biological hallmarks of colorectal cancer. Genes Dev. 35 (11-12), 787–820. doi:10.1101/gad.348226.120
Li, M. M., Datto, M., Duncavage, E. J., Kulkarni, S., Lindeman, N. I., Roy, S., et al. (2017). Standards and guidelines for the interpretation and reporting of sequence variants in cancer: a joint consensus recommendation of the association for molecular pathology, American society of clinical oncology, and College of American Pathologists. J. Mol. Diagn 19 (1), 4–23. doi:10.1016/j.jmoldx.2016.10.002
Li, Q., Ren, Z., Cao, K., Li, M. M., Wang, K., and Zhou, Y. (2022). CancerVar: an artificial intelligence-empowered platform for clinical interpretation of somatic mutations in cancer. Sci. Adv. 8 (18), eabj1624. doi:10.1126/sciadv.abj1624
Lindeman, N. I., Cagle, P. T., Beasley, M. B., Chitale, D. A., Dacic, S., Giaccone, G., et al. (2013). Molecular testing guideline for selection of lung cancer patients for EGFR and ALK tyrosine kinase inhibitors: guideline from the College of American Pathologists, international association for the study of lung cancer, and association for molecular pathology. J. Thorac. Oncol. 8 (7), 823–859. doi:10.1097/JTO.0b013e318290868f
Loupakis, F., Ruzzo, A., Cremolini, C., Vincenzi, B., Salvatore, L., Santini, D., et al. (2009). KRAS codon 61, 146 and BRAF mutations predict resistance to cetuximab plus irinotecan in KRAS codon 12 and 13 wild-type metastatic colorectal cancer. Br. J. Cancer 101 (4), 715–721. doi:10.1038/sj.bjc.6605177
Maloney, R. C., Zhang, M., Jang, H., and Nussinov, R. (2021). The mechanism of activation of monomeric B-Raf V600E. Comput. Struct. Biotechnol. J. 19, 3349–3363. doi:10.1016/j.csbj.2021.06.007
Malumbres, M., and Barbacid, M. (2003). RAS oncogenes: the first 30 years. Nat. Rev. Cancer 3 (6), 459–465. doi:10.1038/nrc1097
Martinez Fiesco, J. A., Durrant, D. E., Morrison, D. K., and Zhang, P. (2022). Structural insights into the BRAF monomer-to-dimer transition mediated by RAS binding. Nat. Commun. 13 (1), 486. doi:10.1038/s41467-022-28084-3
McLaren, W., Gil, L., Hunt, S. E., Riat, H. S., Ritchie, G. R. S., Thormann, A., et al. (2016). The Ensembl variant effect predictor. Genome Biol. 17 (1), 122. doi:10.1186/s13059-016-0974-4
Mikolajcik, P., Lasabova, Z., Loderer, D., Grendar, M., Kalman, M., Kasubova, I., et al. (2021). Detection of therapeutically relevant and concomitant rare somatic variants in colorectal cancer. Neoplasma 68 (6), 1331–1340. doi:10.4149/neo_2021_210616N804
Mitchell, E. L. D., Jones, D., White, G. R. M., Varley, J. M., and Santibanez Koref, M. F. (2008). Determination of the gene order of the three loci CD2, NGFB, and NRAS at human chromosome band 1p13 and refinement of their localisation at the subband level by fluorescence in situ hybridisation. Cytogenet. Cell Genet. 70 (3-4), 183–185. doi:10.1159/000134028
Montminy, E. M., Jang, A., Conner, M., and Karlitz, J. J. (2020). Screening for colorectal cancer. Med. Clin. North Am. 104 (6), 1023–1036. doi:10.1016/j.mcna.2020.08.004
Morgan, E., Arnold, M., Gini, A., Lorenzoni, V., Cabasag, C. J., Laversanne, M., et al. (2023). Global burden of colorectal cancer in 2020 and 2040: incidence and mortality estimates from GLOBOCAN. Gut 72 (2), 338–344. doi:10.1136/gutjnl-2022-327736
Morris, V. K., Kennedy, E. B., Baxter, N. N., III, A. B. B., Cercek, A., Cho, M., et al. (2023). Treatment of metastatic colorectal cancer: ASCO guideline. J. Clin. Oncol. 41 (3), 678–700. doi:10.1200/jco.22.01690
Ng, P. C., and Henikoff, S. (2003). SIFT: predicting amino acid changes that affect protein function. Nucleic Acids Res. 31 (13), 3812–3814. doi:10.1093/nar/gkg509
Nuevo-Tapioles, C., and Philips, M. R. (2022). The role of KRAS splice variants in cancer biology. Front. Cell Dev. Biol. 10, 1033348. doi:10.3389/fcell.2022.1033348
Oikonomou, E., Koustas, E., Goulielmaki, M., and Pintzas, A. (2014). BRAF vs RAS oncogenes: are mutations of the same pathway equal? Differential signalling and therapeutic implications. Oncotarget 5 (23), 11752–11777. doi:10.18632/oncotarget.2555
Pantsar, T. (2020). The current understanding of KRAS protein structure and dynamics. Comput. Struct. Biotechnol. J. 18, 189–198. doi:10.1016/j.csbj.2019.12.004
Park, E., Rawson, S., Li, K., Kim, B.-W., Ficarro, S. B., Pino, G.G.-D., et al. (2019). Architecture of autoinhibited and active BRAF–MEK1–14-3-3 complexes. Nature 575 (7783), 545–550. doi:10.1038/s41586-019-1660-y
Patel, S. G., Karlitz, J. J., Yen, T., Lieu, C. H., and Boland, C. R. (2022). The rising tide of early-onset colorectal cancer: a comprehensive review of epidemiology, clinical features, biology, risk factors, prevention, and early detection. Lancet Gastroenterol. Hepatol. 7 (3), 262–274. doi:10.1016/s2468-1253(21)00426-x
Peeters, M., Kafatos, G., Taylor, A., Gastanaga, V. M., Oliner, K. S., Hechmati, G., et al. (2015). Prevalence of RAS mutations and individual variation patterns among patients with metastatic colorectal cancer: a pooled analysis of randomised controlled trials. Eur. J. Cancer 51 (13), 1704–1713. doi:10.1016/j.ejca.2015.05.017
Pires, D. E. V., Ascher, D. B., and Blundell, T. L. (2014). DUET: a server for predicting effects of mutations on protein stability using an integrated computational approach. Nucleic Acids Res. 42 (W1), W314–W319. doi:10.1093/nar/gku411
Prior, I. A., Hood, F. E., and Hartley, J. L. (2020). The frequency of Ras mutations in cancer. Cancer Res. 80 (14), 2969–2974. doi:10.1158/0008-5472.Can-19-3682
Puyol, M., Martín, A., Dubus, P., Mulero, F., Pizcueta, P., Khan, G., et al. (2010). A synthetic lethal interaction between K-Ras oncogenes and Cdk4 unveils a therapeutic strategy for non-small cell lung carcinoma. Cancer Cell 18 (1), 63–73. doi:10.1016/j.ccr.2010.05.025
Quang, D., Chen, Y., and Xie, X. (2015). DANN: a deep learning approach for annotating the pathogenicity of genetic variants. Bioinformatics 31 (5), 761–763. doi:10.1093/bioinformatics/btu703
Rauch, J., Volinsky, N., Romano, D., and Kolch, W. (2011). The secret life of kinases: functions beyond catalysis. Cell Commun. Signal. 9 (1), 23. doi:10.1186/1478-811X-9-23
Rentzsch, P., Witten, D., Cooper, G. M., Shendure, J., and Kircher, M. (2019). CADD: predicting the deleteriousness of variants throughout the human genome. Nucleic Acids Res. 47 (D1), D886-D894–d894. doi:10.1093/nar/gky1016
Reva, B., Antipin, Y., and Sander, C. (2011). Predicting the functional impact of protein mutations: application to cancer genomics. Nucleic Acids Res. 39 (17), e118. doi:10.1093/nar/gkr407
Roy, A., Kucukural, A., and Zhang, Y. (2010). I-TASSER: a unified platform for automated protein structure and function prediction. Nat. Protoc. 5 (4), 725–738. doi:10.1038/nprot.2010.5
Samocha, K. E., Kosmicki, J. A., Karczewski, K. J., O’Donnell-Luria, A. H., Pierce-Hoffman, E., MacArthur, D. G., et al. (2017). Regional missense constraint improves variant deleteriousness prediction. BioRxiv 148353. doi:10.1101/148353
Santarpia, L., Lippman, S. M., and El-Naggar, A. K. (2012). Targeting the MAPK-RAS-RAF signaling pathway in cancer therapy. Expert Opin. Ther. Targets 16 (1), 103–119. doi:10.1517/14728222.2011.645805
Sepulveda, A. R., Hamilton, S. R., Allegra, C. J., Grody, W., Cushman-Vokoun, A. M., Funkhouser, W. K., et al. (2017). Molecular biomarkers for the evaluation of colorectal cancer: guideline from the American society for clinical pathology, College of American Pathologists, association for molecular pathology, and the American society of clinical oncology. J. Clin. Oncol. 35 (13), 1453–1486. doi:10.1200/jco.2016.71.9807
Serebriiskii, I. G., Connelly, C., Frampton, G., Newberg, J., Cooke, M., Miller, V., et al. (2019). Comprehensive characterization of RAS mutations in colon and rectal cancers in old and young patients. Nat. Commun. 10 (1), 3722. doi:10.1038/s41467-019-11530-0
Seruca, R., Velho, S., Oliveira, C., Leite, M., Matos, P., and Jordan, P. (2009). Unmasking the role of KRAS and BRAF pathways in MSI colorectal tumors. Expert Rev. Gastroenterol. Hepatol. 3 (1), 5–9. doi:10.1586/17474124.3.1.5
Sherr, C. J. (1995). D-type cyclins. Trends Biochem. Sci. 20 (5), 187–190. doi:10.1016/s0968-0004(00)89005-2
Shihab, H. A., Gough, J., Cooper, D. N., Stenson, P. D., Barker, G. L. A., Edwards, K. J., et al. (2013). Predicting the functional, molecular, and phenotypic consequences of amino acid substitutions using hidden markov models. Hum. Mutat. 34 (1), 57–65. doi:10.1002/humu.22225
Shihab, H. A., Rogers, M. F., Gough, J., Mort, M., Cooper, D. N., Day, I. N., et al. (2015). An integrative approach to predicting the functional effects of non-coding and coding sequence variation. Bioinformatics 31 (10), 1536–1543. doi:10.1093/bioinformatics/btv009
Siraj, A. K., Bu, R., Prabhakaran, S., Bavi, P., Beg, S., Al Hazmi, M., et al. (2014). A very low incidence of BRAF mutations in Middle Eastern colorectal carcinoma. Mol. Cancer 13 (1), 168. doi:10.1186/1476-4598-13-168
Smalley, K. S. M., Lioni, M., Palma, M. D., Xiao, M., Desai, B., Egyhazi, S., et al. (2008). Increased cyclin D1 expression can mediate BRAF inhibitor resistance in BRAF V600E–mutated melanomas. Mol. Cancer Ther. 7 (9), 2876–2883. doi:10.1158/1535-7163.Mct-08-0431
Śmiech, M., Leszczyński, P., Kono, H., Wardell, C., and Taniguchi, H. (2020). Emerging BRAF mutations in cancer progression and their possible effects on transcriptional networks. Genes (Basel) 11 (11), 1342. doi:10.3390/genes11111342
Stolze, B., Reinhart, S., Bulllinger, L., Fröhling, S., and Scholl, C. (2015). Comparative analysis of KRAS codon 12, 13, 18, 61, and 117 mutations using human MCF10A isogenic cell lines. Sci. Rep. 5, 8535. doi:10.1038/srep08535
Sundaram, L., Gao, H., Padigepati, S. R., McRae, J. F., Li, Y., Kosmicki, J. A., et al. (2018). Predicting the clinical impact of human mutation with deep neural networks. Nat. Genet. 50 (8), 1161–1170. doi:10.1038/s41588-018-0167-z
Tabernero, J., Ros, J., and Élez, E. (2022). The evolving treatment landscape in BRAF-V600e–mutated metastatic colorectal cancer. Am. Soc. Clin. Oncol. Educ. Book 42 (42), 1–10. doi:10.1200/edbk_349561
Van Cutsem, E., Cervantes, A., Adam, R., Sobrero, A., Van Krieken, J. H., Aderka, D., et al. (2016). ESMO consensus guidelines for the management of patients with metastatic colorectal cancer. Ann. Oncol. 27 (8), 1386–1422. doi:10.1093/annonc/mdw235
Vaughn, C. P., Zobell, S. D., Furtado, L. V., Baker, C. L., and Samowitz, W. S. (2011). Frequency of KRAS, BRAF, and NRAS mutations in colorectal cancer. Genes Chromosom. Cancer 50 (5), 307–312. doi:10.1002/gcc.20854
Voskoboynik, M., Mar, V., Mailer, S., Colebatch, A., Fennessy, A., Logan, A., et al. (2016). Clinicopathological characteristics associated with BRAFK601E and BRAFL597 mutations in melanoma. Pigment Cell and Melanoma Res. 29 (2), 222–228. doi:10.1111/pcmr.12450
Wang, K., Li, M., and Hakonarson, H. (2010). ANNOVAR: functional annotation of genetic variants from high-throughput sequencing data. Nucleic acids Res. 38 (16), e164. doi:10.1093/nar/gkq603
Waterhouse, A., Bertoni, M., Bienert, S., Studer, G., Tauriello, G., Gumienny, R., et al. (2018). SWISS-MODEL: homology modelling of protein structures and complexes. Nucleic Acids Res. 46 (W1), W296-W303–w303. doi:10.1093/nar/gky427
Yan, L., Zou, L., Zhao, W., Wang, Y., Liu, B., Yao, H., et al. (2015). Clinicopathological significance of c-KIT mutation in gastrointestinal stromal tumors: a systematic review and meta-analysis. Sci. Rep. 5, 13718. doi:10.1038/srep13718
Yang, H., and Wang, K. (2015). Genomic variant annotation and prioritization with ANNOVAR and wANNOVAR. Nat. Protoc. 10 (10), 1556–1566. doi:10.1038/nprot.2015.105
Yang, J., Yan, R., Roy, A., Xu, D., Poisson, J., and Zhang, Y. (2015). The I-TASSER Suite: protein structure and function prediction. Nat. Methods 12 (1), 7–8. doi:10.1038/nmeth.3213
Yang, Y., Zhang, H., Huang, S., and Chu, Q. (2023). KRAS mutations in solid tumors: characteristics, current therapeutic strategy, and potential treatment exploration. J. Clin. Med. 12 (2), 709. doi:10.3390/jcm12020709
Zaman, A., Wu, W., and Bivona, T. G. (2019). Targeting oncogenic BRAF: past, present, and future. Cancers (Basel) 11 (8), 1197. doi:10.3390/cancers11081197
Zeitouni, D., Pylayeva-Gupta, Y., Der, C. J., and Bryant, K. L. (2016). KRAS mutant pancreatic cancer: No lone path to an effective treatment. Cancers (Basel) 8 (4), 45. doi:10.3390/cancers8040045
Zhang, Y. (2008). I-TASSER server for protein 3D structure prediction. BMC Bioinforma. 9 (1), 40. doi:10.1186/1471-2105-9-40
Keywords: somatic mutations, BRAF V600E, TruSight tumor 15 panel, targeted drug therapy, colorectal cancer
Citation: Aljuhani TA, Shaik NA, Alqawas RT, Bokhary RY, Al-Mutadares M, Al Mahdi HB, Al-Rayes N, El-Harouni AA, Elango R, Banaganapalli B and Awan ZA (2024) Exploring somatic mutations in BRAF, KRAS, and NRAS as therapeutic targets in Saudi colorectal cancer patients through massive parallel sequencing and variant classification. Front. Pharmacol. 15:1498295. doi: 10.3389/fphar.2024.1498295
Received: 18 September 2024; Accepted: 31 October 2024;
Published: 20 November 2024.
Edited by:
Udhaya Kumar, Baylor College of Medicine, United StatesReviewed by:
Ab Rauf Shah, University of Nebraska Medical Center, United StatesHuma Khan, Shoolini University, India
Ganji Purnachandra Nagaraju, University of Alabama at Birmingham, United States
Copyright © 2024 Aljuhani, Shaik, Alqawas, Bokhary, Al-Mutadares, Al Mahdi, Al-Rayes, El-Harouni, Elango, Banaganapalli and Awan. This is an open-access article distributed under the terms of the Creative Commons Attribution License (CC BY). The use, distribution or reproduction in other forums is permitted, provided the original author(s) and the copyright owner(s) are credited and that the original publication in this journal is cited, in accordance with accepted academic practice. No use, distribution or reproduction is permitted which does not comply with these terms.
*Correspondence: Babajan Banaganapalli, YmJhYmFqYW5Aa2F1LmVkdS5zYQ==; Zuhier Ahmad Awan, emF3YW5Aa2F1LmVkdS5zYQ==
†These authors share first authorship