- School of Life Science, Ludong University, Yantai, Shandong, China
The protein tyrosine phosphatase 1B (PTP1B) is a critical therapeutic target for type 2 diabetes mellitus (T2DM). Many PTP1B inhibitors have been reported, however, most of them lack high specificity and have adverse effects. Designing effective PTP1B inhibitors requires understanding the molecular mechanism of action between inhibitors and PTP1B. To this end, molecular dynamics (MD) simulations and molecular mechanics Poisson Boltzmann Surface Area (MM-PB/SA) methods were used to observe the binding patterns of compounds with similar pentacyclic triterpene parent ring structures but different inhibition abilities. Through structure and energy analysis, we found that the positions of cavities and substituents significantly affect combining capacity. Besides, we constructed a series of potential inhibitor molecules using LUDI and rational drug design methods. The ADMET module of Discovery Studio 2020 was used to predict the properties of these inhibitor molecules. Lastly, we obtained compounds with low toxicity and significant inhibitory activity. The study will contribute to the treatment of T2DM.
1 Introduction
Diabetes mellitus (DM) is a common systemic disease of the endocrine system (Ma et al., 2021; Singh et al., 2022). This major public health issue and socioeconomic burden endangers human health worldwide (Hünenberger et al., 1995; Lam and LeRoith, 2012). In 2045, there will be 220 million people living with diabetes worldwide, according to the International Diabetes Federation (Paul et al., 2023). A majority of people with diabetes are aware of type 2 diabetes, which represents 90%–95% of diabetes cases. Insulin resistance (IR) and relative insulin deficiency are characteristics of diabetes mellitus type 2 (T2DM) (Verma et al., 2017; Jiang and Gao, 2019).
The proteins of protein tyrosine phosphatases (PTP) family are a class of phosphatases that play an essential role in signal transduction pathways that regulate the progression of cell growth, division, adhesion, and motility (Hunter, 1995; Hunter, 2000). Disruption of PTP catalytic activity will lead to abnormal tyrosine phosphorylation, resulting in the development and progression of various diseases (Peters et al., 2003). Protein tyrosine phosphatase 1B (PTP1B), an essential member of the PTP family, is a negative regulator of insulin receptor (IR) signaling that negatively regulates insulin signaling through dephosphorylation of the insulin receptor and its substrates, thereby diminishing the effect of insulin (Combs, 2010; Lessard et al., 2010). In addition, PTP1B knockout mice exhibit insulin sensitivity and glycemic control, are resistant to obesity, and have significantly lower triglycerides levels (Elchebly et al., 1999; Bence et al., 2006; Comeau et al., 2010). Hence, PTP1B has emerged as a novel promising therapeutic target for the treatment of T2DM.
Protein tyrosine phosphatases have high structural conserved properties at the active site, which makes the design and modification of selective PTP1B inhibitors very difficult (Andersen et al., 2001). TCPTP and PTP1B showed 72% sequence identity in the catalytic region, while mice knocked out by TCPTP showed hematopoietic defects (Shinde and Sobhia, 2013). Therefore, competitive inhibitors targeting the catalytic site of PTP1B may also bind to the catalytic site of TCPTP, causing hematopoietic dysfunction. Compared with competing inhibitors, PTP1B allosteric inhibitors have low side effects and do not cause PTP1B aggregation (Krishnan et al., 2014; Krishnan and Tonks, 2015; Krishnan et al., 2018). At the preclinical level, trodusquemine, an allosteric inhibitor of PTP1B, demonstrated the ability to significantly reduce fat and insulin levels in obese mice (Lantz et al., 2010; Cho and Litwack, 2013; Olloquequi et al., 2022), which means that allosteric inhibitors can exert inhibitory activities that are no less potent than competitive inhibitors. Therefore, the design of novel allosteric inhibitors can be considered.
PTP1B has an N-terminal catalytic phosphatase domain, as well as a C-terminal membrane localization domain (Tonks et al., 1988). The active site of PTP1B consists of three parts: (1) P-loop with Cys215 as the catalytic center; (2) WPD-loop responsible for substrate identification; (3) Q-loop containing Gln262 residues (Tonks, 2003). The closed pose of the WPD ring is the result of the interaction between the α3 (Glu186-Glu200) and α6 (Ala264-Ile279) helixes (Shinde and Sobhia, 2013). The active site actually includes α7(Val287-Ser295), but α7 unspins when it binds to an allosteric inhibitors (Wiesmann et al., 2004a). While the movement of the WPD ring is the result of the hydrogen-bond network among α7-α3-α6 helixes. While, allosteric inhibitors can destroy this hydrogen-bond network by placing themselves among these helixes, thereby blocking the open or closed conformation of the PTP1B protein, rendering it unable to function. To this, it is important to discover novel PTP1B inhibitors with good inhibitory activity and selectivity.
Currently, the majority of studies are centered on the movement pattern of PTP1B function and the search for inhibitors of PTP1B. Regrettably, these literatures carried their own limitations. For instance, in the articles investigating the movement process, collaboration, and interaction of PTP1B through molecular dynamics, the majority failed to present viewpoints on inhibiting the activity of PTP1B (Akyol and Kilic, 2021), while the literatures devoted to finding or designing inhibitors of PTP1B did not undertake subsequent exploration of the action mode for the discovered inhibitors (Maccari et al., 2023; Zheng et al., 2024).
In the present study, molecular dynamics (MD) simulations combined with molecular mechanics Poisson Boltzmann Surface Area (MM-PB/SA) calculations, which have proved to be robust and valuable tools (Pan et al., 2016; Coskuner and Uversky, 2017; Wen et al., 2017; Shi et al., 2018; Wang et al., 2018; Alamri et al., 2023; Gao et al., 2023; Hassan et al., 2023), were used to explore the interaction and binding capacity between inhibitors and PTP1B. AMDET property prediction is used to evaluate compounds’ molecular properties and select and optimize lead compounds according to their properties. The parent structures with analogous configurations were exploited to investigate the binding action mode and motion mode between the intermolecular inhibitors and PTP1B. Based on this, drug design strategies were proposed. Eventually, the feasibility of our results was verified via the analysis of binding free energy and druggability. Our work may provide valuable clues for drug modification and improve binding affinity to combat drug resistance.
2 Materials and methods
2.1 Initial structures
The initial structure of PTP1B protein was derived from Protein Data Bank (PDB code: 1T49) (Figure 1) (Wiesmann et al., 2004b). Compared with other PDB structures(7KEN, 5T19, 1T48, or 5QDE), 1T49 has relatively high resolution (1.9 Å), mutation-free amino acid sequence, and the binding ligand in the crystal structure is similar to those in the present study (Jiang et al., 2022). Protein pretreatment and energy minimization of small molecules are performed by Discovery Studio 2020 (DS 2020). The position of the ligand of the original PDB crystal structure is used as the binding site. The ligands used in this article were all pentacyclic triterpenoids reported in the previous literature (Figure 2) (Tables 1, 2) (Xu et al., 2018; Huang et al., 2022). These studies have experimentally identified several compounds with inhibitory activity against PTP1B in Quercus liaotungensis, Paeonia suffruticosa, and Paeonia delavayi. The majority of these compounds are pentacyclic triterpenoids. Compounds with different IC50 values were selected to compare the structure-activity relationships of different residues. These compounds possess similar structures yet distinct substituents. Following molecular dynamics, the interaction between the compounds and the protein can be delineated more lucidly. Upon discovering these compounds, the existing literature did not provide information on the type of inhibitors. We individually docked these compounds to the catalytic and allosteric sites of PTP1B.DS2020 was used for molecular docking to dock Ligand 1-7 to specific sites on the PTP1B protein. After docking, we named these docking systems Complex1-7 based on the names of the docked ligands, for example, the ligand name in the complex-1 system is Ligand-1. The protonation states of ionizable residues were determined at pH = 7.4 using H++ server (Gordon et al., 2005). The partial charges and missing force field parameters for inhibitors were obtained by the Antechamber module of AMBER 18 software (Wang et al., 2006). The general AMBER force field (GAFF) (Wang et al., 2004) and ff14SB force field (Maier et al., 2015) were used for inhibitors and PTP1B, respectively, just as the methods employed by Lima Silva and Ferreira de Freitas (2023); Park et al. (2023). The missing atoms of proteins in the models were added using the t-Leap module of AMBER 18. To keep the whole system in an electric neutral state, sodium ions were added based on a Coulomb potential grid using t-Leap module of AMBER 18 software (Case et al., 2017). Then, each system was solvated with the TIP3P water model (Jorgensen et al., 1983) in a truncated octahedron box with a 10.0 Å distance around the solute.
2.2 Molecular dynamics (MD) simulations
AMBER18 software package (Case et al., 2017) was used for molecular dynamics simulation. First, 10,000 steps of minimization (steepest 4,000 steps, followed by 6,000 steps of conjugation gradient) with proteins and inhibitors constrained (500 kcal mol-1 Å-2). Then, the minimization is repeated without any constraints. Thereafter, each system was gradually heated from 0 K–310 K over a period of 300 ps with 5.0 kcal mol-1 Å-2 restrain on the solute and then another 1 ns equilibrium simulation was followed at 310 K with 2.0 kcal mol-1 Å-2 restrain on the solute. Finally, 200 ns MD simulations were performed for every system under NPT conditions to obtain the simulated trajectories. The temperature was maintained at 310 K by coupling to a Langevin heatbath (Uberuaga et al., 2004) using a collision frequency of 1 ps-1, and a constant isotropic pressure was maintained at 1 atm using the Berendsen barostat (Berendsen et al., 1984). Short range interactions were cut off at 10.0 Å, while the long-range electrostatic interactions were handled using the particle mesh Ewald (PME) method (Darden et al., 1993). The SHAKE algorithm was used to restrict all covalent bonds involving hydrogen atoms (Ryckaert et al., 1977). The time step was set to 2 fs.
2.3 MM-PB/SA calculations
Binding free energy of each complex was calculated by MM-PB/SA (Kollman et al., 2000; Sun et al., 2014; Kong et al., 2018; Federico et al., 2021) method in AMBER 18. In our calculation, the last 10,000 snapshots are extracted from each simulated trajectory to calculate the binding free energy. The equations are as follows:
In Equation 1, Gcomplex, Greceptor, and Gligand are the free energies of the complex, the receptor, and ligands, respectively. In Equation 2, the ∆EMM, ∆Gsol, and T∆S represent molecular mechanics component in the gas phase, the desolvation free energy, and a vibrational entropy term, respectively. And in Equation 3, ∆EMM is the summation of internal interaction (∆Eint), Coulomb interaction (∆Eele), and van der Waals (vdW) interaction (∆EvdW) terms. In Equation 4, Gsol can be separated into an electrostatic solvation energy (∆GGB) and nonelectrostatic solvation energy (∆GSA). For obtaining the detailed view of protein and ligands interaction, MM-PB/SA method was employed to calculate the binding free energy of each residue. We selected the stable trajectory after MD simulation to calculate entropy.
2.4 Structure-based inhibitor design
LUDI (Böhm, 1994) is a fragment-based de novo drug design algorithm. In this present study, we used the pentacyclic triterpene parent structure as a starting point in this study, with amino acids from the α3 and α6 helical regions of PTP1B acting as receptor action regions, and the LUDI module in DS2020 added design fragments to the drug structure (Oner et al., 2023). In addition, we also conduct rational drug design based on the intermolecular interactions between inhibitors and amino acid residues.
2.5 ADMET properties prediction
ADMET properties refer to the absorption, distribution, metabolism, excretion, and toxicity of molecules in the organic body (Dong et al., 2018). Predictable properties of ADMET include water solubility, blood-brain barrier penetration (BBB), hepatotoxicity, human intestinal absorption (HIA), aqueous solubility (LogSw), and plasma protein binding. In this experiment, we applied LUDI modification and rational design compounds to ADMET prediction on DS 2020.
2.6 TOPKAT and druggability analysis
TOPKAT is based on the 2D structure of the molecule to calculate and verify the toxicity and environmental effects of the compound (Ma et al., 2008). The rat oral LD50 for all ligands was calculated and measured by the TOPKAT module in DS 2020. Druggability Analysis is run through DrugFlow (www.drugfow.com).
3 Results and discussion
3.1 Stability of ligands in MD simulations
The structural stability was investigated by calculating average root-mean-square deviation (RMSD) of backbone atoms with respect to the first frame. Details of these complexes systems are shown in Supplementary Figure S1.
Supplementary Figure S1 shows that the fluctuation range of RMSD of these complexes is 0.5–1.5 Å in the first 100 ns and stabilizes at about 1 Å in the last 100 ns. In the whole simulation process, all the complex structures are relatively stable. Therefore, for all systems, the last 100 ns trajectories are selected for further analyses.
To analyze the variation of flexibility in seven complexes, average root-mean-square fluctuations (RMSF) of backbone atoms were calculated. The RMSF of the corresponding complexes systems was calculated. By comparing the unbound complex with the one bound to a natural PTP1B inhibitor, it is evident that the fluctuation of the WPD-loop (Thr177-Pro185) in the protein without ligands is more pronounced, reaching approximately 2.0 Å (Supplementary Figure S5), this result indicates that the protein without ligands is indeed less stable. According to the literature, the movement of the allosteric site can influence the conformational dynamics of the WPD-loop (Wang et al., 2020). Additionally, binding of an inhibitor may hinder the movement of the allosteric site, thereby impeding the conformational changes in the WPD-loop and leading to inhibition of PTP1B. These findings are consistent with our results. Moreover, in the α3 helix region, we observed a fluctuation of approximately 2.5 Å in protein without ligands, whereas this fluctuation reduced to about 1 Å on average in ligand-bound protein. Similarly, in the α6 helix region, we noted a fluctuation of about 1 Å without bound ligand compared to approximately 0.5 Å with bound ligand. These observations indicate that ligand binding enhances stability by reducing protein fluctuations.
Supplementary Figure S2 shows similar fluctuations between the different systems, which indicates that the complex systems are stable as a whole and do not lead to an increase in the structural motion of the residues. Under the restriction imposed by the inhibitor, the flexibility will naturally decrease if the inhibitor is successfully combined and interacts. It has been shown in previous studies (Wang et al., 2020) that the RMSF values of the WPD-loop of PTP1B are different between the PTP1B-open and close configuration. Open conformation has a RMSF value of about 2 Å. Close conformation has a RMSF value of about 0.5 Å (Figure 3). The results show that the RMSF value of the PTP1B protein (open conformation) in the WPD loop region of all complex systems after binding to the allosteric inhibitor is no higher than 1 Å. The results indicate that our docked ligand stably binds to the allosteric site of the PTP1B protein, affecting the movement of the helical region, which affects WPD-loop structural changes. The fluctuation of α3 helical residues in the complex-2 system is the smallest, and the fluctuation of α3 helical residues in the complex-1 system is the largest. This result show Ligand-2 exhibits the strongest intermolecular interaction, while Ligand-1 exhibits the weakest interaction. However, the RMSF curve for the complex-5 system at the α6 helix is small, showing that the intermolecular interactions of Ligand-2 and Ligand-3 are more potent than that of Ligand-1 and Ligand-5. Considering other compounds with poor inhibitory ability, substituents at C-23 and C-1 may affect the binding ability of the compounds (Figure 2).
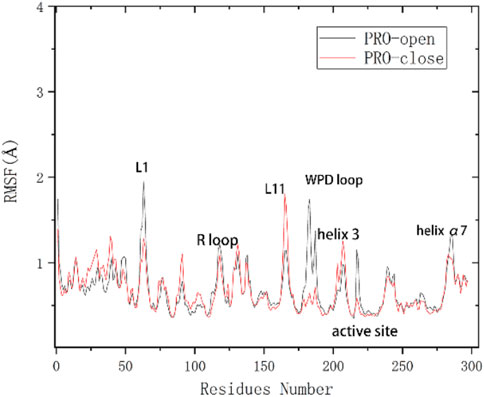
Figure 3. Flexibility changes in amino acid residues of the PTP1B protein (unbound inhibitor) (Wang et al., 2020).
Supplementary Figure S3 indicate that the RMSD values of the PTP1B complex system bound to the catalytic site. While Complex-4 exhibits larger fluctuations in the first 100 ns, it stabilizes in the subsequent 100 ns. The remaining compounds demonstrate general stability, with fluctuations ranging from 0.5–1.5 Å, leading us to conclude that overall system stabilization occurs in the latter 100 ns, during which subsequent binding free energy calculations were conducted. The RMSF values of the complex in Supplementary Figure S4, where the ligand binds to a competitive site, exhibit more pronounced overall fluctuations compared to those observed in the allosteric system. The final confirmation of the inhibitor’s type still necessitates validation through free energy analysis.
In addition, the other three compounds can also bind to the allosteric region of PTP1B to limit the movement of residues. However, whether their binding is stable and the energy effect is strong remains to be determined.
3.2 Energetic analysis of complex
3.2.1 Binding free energy analysis
Subsequently, upon system stabilization, we computed the binding free energy of these triterpenoid compounds at their respective sites to assess and confirm their inhibitor type.
Table 8 shows the binding free energies (ΔGbind) for seven complexes were calculated by the MM-PB/SA approach. The ΔGbind values of the seven compounds at the PTP1B allosteric site calculated are summarized in the Table 3 and the PTP1B catalytic site are summarized in Table 4. Table 3 shows that the order of binding energy is complex-1 > complex-2 > complex-5 > complex-3 > complex-7 > complex-6 > complex-4. ΔGbind are −17.6 kcal mol−1, −17.3 kcal mol−1 −12.1 kcal mol−1, −9.4 kcal mol−1, −2.5 kcal mol−1, −2.2 kcal mol−1, 0.8 kcal mol−1, respectively, which are consistent with the trend of inhibition of PTP1B reported in the literature. However, the absolute values of the binding free energies in Table 4 are not consistent with the trends reported in the literature. Interestingly, in the course of our molecular dynamics simulations, we observed the some initial structures of ligands bound to the catalytic site, followed by their gradual transition away from the catalytic site towards the allosteric site over time. Based on the observed ligand movement during the MD process and the calculated binding free energy of the MM-PB/SA complex, it can be inferred that these compounds exhibit characteristics consistent with allosteric inhibition.
In these complexes, the binding free energy is mainly determined by ΔGnonp. ΔGnonp is the sum of ΔEvdw and ΔESA, most of which comes from ΔEvdw. The value of ΔEvdw gradually decreases with the difference and number of substituents of these ligands. ΔGpol is the sum of ΔEele and ΔGSA, and entropy changes (-TΔS) adversely affect complexes and inhibitors. In other words, the larger the absolute value of Gbind is, the stronger the binding ability between the compound and the ligand indicates. Table 3 shows that the absolute value of ΔEele of complex-7 is greater than that of the other systems (−22.1 kcal mol−1), indicating that the contribution of electrostatic interaction in this system is relatively larger. However, complex-3 (−10.5 kcal mol−1) and complex-6 (−9.5 kcal mol−1) exhibit a medium degree of electrostatic interaction, and the ΔEele of the other systems is relatively smaller, this result indicates that the electrostatic interaction formed between amino acid residues and ligands within the system is relatively minor. However, relatively speaking, the absolute value of ΔEvdw in all systems is extremely large. Possibly because the spatial position occupied by Ligand-1 is substantial, it can interact with more amino acids and has the highest van der Waals interaction. Whereas compounds with low activity demonstrate lower ΔEele and ΔEvdw or higher ΔGGB.
Complex-1, complex-2, complex-3, and complex-5 have excellent binding free energies and IC50 values. These four compounds will be discussed in more detail later.
3.2.2 Decomposition energy of different key residues
In order to explore residues that contribute significantly to receptor and ligand binding, we calculated the binding free energy for each residue in the four complexes (Ligand-1, Ligand-2, Ligand-3, Ligand-5) (Table 5). The energy of different key residues is divided into van der Waals energy, electrostatic interaction, polar solvation-free energy, and non-polar solvation-free energy (Supplementary Tables S1–S4). In this study, four compounds (Ligand-1, Ligand-2, Ligand-3, Ligand-5) have relatively stable free binding energies. Residues with binding free energy < -0.5 kcal mol−1 are considered vital residues.
By analysing the contribution for each residue in the receptor, 12 residues (Ala189, Leu192, Asn193, Leu195, Phe196, Lys197, Leu232, Met235, Ala278, Lys279, Phe280, and Ile281) are essential. Besides van der Waals interaction rather than electrostatic contribute the most. In complex-1, Asn193 and Lys279 have higher electrostatic interactions. Met235 only showed higher binding capacity in complex-1, which may be attributed to the fact that Ligand 1 has a long side chain and is spatially closer to Met235. Lys197 and Lys279 have significantly lower free energies in complex-1, 2, and 5 with identical substituents at different positions than in complex-3. In addition, Phe196 and Phe280 are amino acid residues worthy of attention. Their free energy contribution in different systems is higher than that of other amino acid residues, especially in the Complex-2 system. This may indicate that two amino acids participate in the key residues for anchoring the compound.
3.3 Cluster analysis
Further exploration of the reasons for the strong binding ability and intermolecular interactions of these different inhibitors is required. It is possible to identify stable and representative conformations through cluster analysis in order to explore mechanisms of action between ligands and proteins. After the system is stabilized, the dominant conformation in each system is taken as the most representative conformation. Based on Table 6, cluster-1 is the dominant conformation. The Figure 4 clearly shows that the spatial differences between different ligands vary, while Ligand-1 can occupy the cavity position to a large extent, and can form more intermolecular interactions.
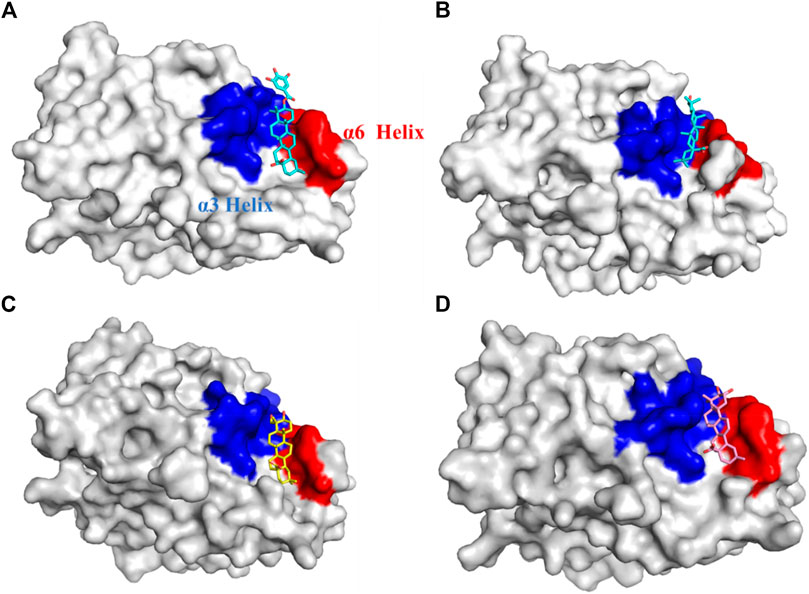
Figure 4. Cavity position and morphology of the four ligands in the PTP1B protein (Red: α6 Helix; Blue: α3 Helix). (A) Ligand-1 binds to the PTP1B protein. (B) Ligand-2 binds to the PTP1B protein. (C) Ligand-3 binds to the PTP1B protein. (D) Ligand-5 binds to the PTP1B protein.
The detailed intermolecular interactions are shown in Figures 5, 6. A hydrophobic interaction is formed by the parent ring structure of these compounds (Leu192, Leu195, Phe196, Leu232, Met235, and Phe280), and some of the substituents or side chains can form hydrogen bonds (Asn193, Asp236, and Ala278). It is also important to note that different ligands form different interactions due to their parent rings and substitutes, and the properties of these substitutes may provide a basis for improving drugs. Despite the fact that Ligand-1 has fewer hydroxyl substituents than other ligands, it is still capable of exerting interaction with sufficient residues. Compared to Ligand-2, 3, and 5, it has limited substituents and side chains. However, the hydroxyl group at C-3 is a hydrophilic substitute that can stabilize the ligand’s existence, but Ligand-4 and Ligand-7 are deficient because C-2 and C-23 possess hydroxyl groups simultaneously. The methyl group of C-23 can exert a hydrophobic interaction on Leu192, thereby affecting the binding affinity. As a result, side chains can be added to C-23 to enhance the compound’s hydrophobic interaction.
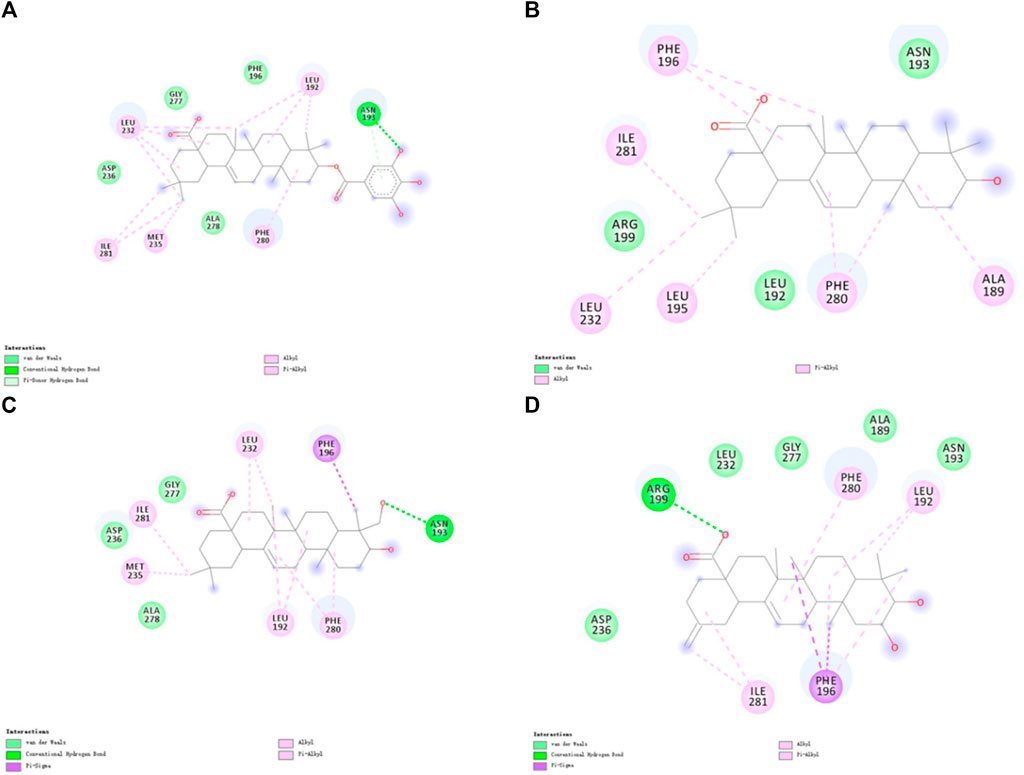
Figure 5. 2D diagram of the interactions of four ligands with PTP1B proteins molecules. (A) 2D diagram of the interaction of Ligand-1 with PTP1B protein. (B) 2D diagram of the interaction of Ligand-2 with PTP1B protein. (C) 2D diagram of the interaction of Ligand-3 with PTP1B protein. (D) 2D diagram of the interaction of Ligand-5 with PTP1B protein.
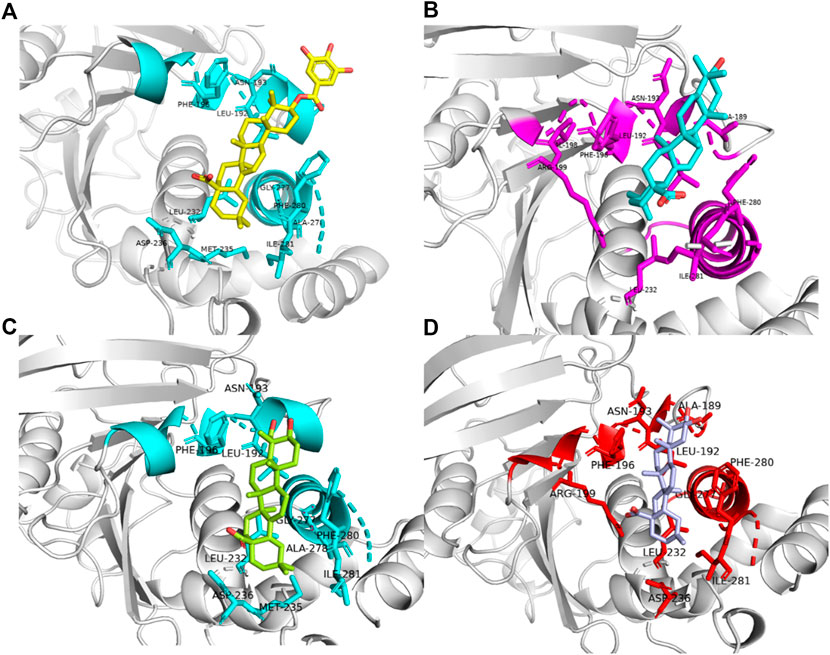
Figure 6. Binding patterns of four different ligands(Amino acid residues with interactive relationships are displayed in Stick mode, otherwise they are displayed in NewCartoon form, and different colors are used to distinguish Ligand-1, 2, 3 and 5.). (A) Ligand-1 binding to residues in complex-1 systems. (B) Ligand-2 binding to residues in complex-2 systems. (C) Ligand-3 binding to residues in complex-3 systems. (D) Ligand-5 binding to residues in complex-5 systems.
Furthermore, by comprehensively considering the binding energy and structure, the simple structure-activity relationship reveals that the different positions of hydroxyl groups can impact the activity of the compounds, and the energy analysis demonstrates that there are indeed certain patterns. Following the augmentation in the quantity of hydroxyl groups, the polar desolvation energy of the inhibitor molecule is elevated, which is not beneficial for the contribution of the binding free energy. Ligand-1 possesses an O-Gal substituent, and the benzene ring establishes a π-Donor hydrogen bond with Asn193, thereby contributing a portion of the van der Waals interaction. Nevertheless, during the experiment, ligand-4 contains a preponderance of hydroxyl groups and deviate from the complex structure in the simulation process. Furthermore, given that the quantity of hydroxyl groups remains the same (Ligand-3, Ligand-5, Ligand-6), the compounds featuring hydroxyl groups at the C-23 or C-24 positions exhibit a greater ΔGGB. In the complex-3 compound system, the hydroxyl group at C-23 forms a conventional hydrogen bond with Asn193, thereby contributing a relatively strong binding free energy. The hydrophobic interaction furnished by Phe280 is relatively insignificant, whereas complexes 1, 2, and 5 suggest that Phe280 and Ile281 have a relatively high contribution to the binding free energy. Perhaps owing to the location of the hydroxyl groups, the ligand undergoes movement during the simulation process. This result implies that reducing the number of hydroxyl groups, or modifying the position of hydroxyl groups and enhancing the number of hydrophobic groups are all beneficial for increasing the stability of the compounds and ligands.
3.4 Combined mode of action analysis
For analysis and discussion, we divided the binding site into three helical regions to analyze the main interaction of these four natural product inhibitors in binding to PTP1B (Figure 7).
Region 1 is the α3 helix region (Glu186-Glu200). Cluster analysis results show that Leu192, Asn193, Phe196, Arg199 had interactions in region 1. During molecular docking, hydrogen bonds or van der Waals interactions were observed between Arg199 and the ligand. Arg199 is positively charged, and the ligand is negatively charged, forming a salt bridge interaction under electrostatic interaction. Molecular dynamics results show no corresponding salt bridge interaction formed in other complex systems. This may be because Ligand-1 has a long side chain. Different systems formed hydrophobic solid interactions with Phe196, indicating that pentacyclic triterpenoids are the core structure of anchoring inhibitors. Likewise, Leu192 forms hydrophobic interactions with different ligands and can interact with the parent structure of the ligand rather than its substituents or functional groups. We speculate that the Asn193 residue is structurally close to the inhibitor and only forms hydrogen bonds or van der Waals interactions. Compared with Complex-2 and Complex-3, Complex-1 exhibits this phenomenon most obviously. Asn193 forms a hydrogen bond with the O-Gal (galloyl group) at C-3 in Complex-1.
Due to the distance between region 2 (Gly220-Lys237) and the ligand, there is no obvious intermolecular interaction. Some amino acid residues still contribute to a higher binding free energy. Leu232 and Met235, for example, are between Complex-1, 2 and 5. The main interaction of these residues is hydrophobic, and we can consider increasing the length or substituents of the C chain structure at the head or tail of the parent structure. In order to form hydrogen bond interactions with Leu232 or Met235, act on the residue helix in region 2, or add substituents to the six-membered rings in the middle of the parent ring.
Region 3 consists of the α6 helical region (Ala264-Ile279). While the Phe280 substituent does not belong to this helical region, it produces a strong hydrophobic interaction in all systems. As phenylalanine itself has a benzene ring, the ligand compound moves under the influence of various residues, and finally, the six-membered ring and the benzene ring form a vertical stacking interaction. Phe280 contributes a high binding free energy through this interaction. As a result, we can consider modifying the six-membered ring, such as changing the single carbon-carbon bond to a double carbon-carbon bond to increase the intermolecular interaction.
Although Complex-4, 6, and 7 showed weak PTP1B inhibitory activity, we also explored their intermolecular interactions. The binding sites of these three compounds were identical, but they did not produce significant effects. Molecular dynamics results indicate that these three ligands only interact with a few amino acid residues. In particular, ligand-4 forms hydrophobic interactions with Asn193, Phe196, Lys197, Arg199, Glu200, Asp236, and Ile281. Different functional groups affect van der Waals and hydrogen bond interactions. The presence of hydroxyl groups at both C-1 and C-23 will have a significant impact on the reaction. Ligand-6 does not have this situation, but still has a low inhibitory activity. The framework consists of a terpene parent structure except for the hydrogen bond formed by the carboxyl substituent and Arg199. The structure of Ligand-6 is relatively simple, so although the compound forms an interaction with some key amino acids, the strength of the interaction is relatively low. Both Ligand-7 and Ligand-4 contain carboxyl groups at C-23 and C-1. The spatial positions of these two carboxyl groups are opposite, which may have a repulsive effect and prevent Ligand-7 from binding to the corresponding region. MD simulations verified that Ligand-4 and Ligand-7 deviated when interacting with the α3 helix region.
It may be possible to design inhibitors based on observations above. Increasing the interaction between Phe280 and Phe196, or considering the electrostatic interaction between Arg199 and Phe196, as well as considering the spatial position of the functional group, will facilitate the binding of the inhibitor to PTP1B.
3.5 Drug design and evaluation
3.5.1 Growth or replacement of the molecular skeleton
LUDI algorithms can help new molecular skeletons or modify existing fragments to improve the binding capacity of ligands and PTP1B. We modified the design of fragments where small molecules can bind to critical regions of PTP1B. Finally, 16 compounds were obtained according to the fragment interaction screening of LUDI (Supplementary Figure S5).
3.5.2 Rational design of inhibitors
In addition to the LUDI design, in order to obtain ligands with better affinity, we also carried out rational design of inhibitors. The rational design of inhibitors requires stable interactions with amino acid residues in key regions. In the design process, we divided into two steps (Singh et al., 2022): Substituents of drugs are modified, added, or removed to improve stability (Ma et al., 2021). The structure of the compound is optimized and treated to pass the blood-brain barrier. Display of cluster analysis results add some groups to hydrophilic substituents or transform some residues into hydrophobic groups to better interact with Phe280 and Phe196. Additionally, some large groups may be added to reduce P-glycoprotein (P-gp) efflux. By removing or modifying carboxyl groups, compounds can be more likely to cross the blood-brain barrier and form stronger intermolecular interactions with key residues.
Figures 5, 6 illustrate the position and molecular interaction of the cavity in which the ligand is located. Increasing the number of substituents for some untouched parts is also possible, which may increase the hydrophobic interaction. Consider adding some hydrophilic groups to the side exposed to the water environment to stabilize the ligand’s binding capacity. In addition, there are some other charged groups in the helix design, and the extension of the carboxyl group of the original compound and transformation into an electrostatic interaction will also contribute to increasing binding free energy. In addition, to enhance the binding ability of the ligand, substituents or extended side chains are added to the side close to the helix, which allows the ligand to fill the protein cavity, resulting in a higher number and stability of interactions with the α6 helix residues (Glu276, Gly277, Ala278, Ile281).
Furthermore, as per previous research reports, the -CF3 groups have been shown to enhance ligand binding affinity through orthogonal dipolar C-F···C = O interactions with the protein’s backbone carbonyl groups. This effect has also been demonstrated in a study by Ledy De-la-Cruz-Martinez et al. (De-la-Cruz-Martínez et al., 2021), where compounds containing -CF3 groups significantly boosted PTP1B inhibitory activity. Additionally, the -CF3 groups can increase lipophilicity and improve blood-brain barrier permeability, aligning with our anticipated outcomes (Design-11 and Design-12). We can validate this through computation of binding free energy.
3.5.3 ADMET prediction
By modifying the ligands and substituents’ structure, we obtained 16 LUDI-modified compounds and 14 rationally designed compounds and predicted their ADMET properties. Figure 8 shows that only a small number of design results fall within the 99% confidence interval of the BBB, HIA, and Log(Sw) model, and this molecule’s prediction is considered relatively reliable. Table 7 shows the prediction details of ADMET for selected compounds. LUDI designed a total of 16 compounds, rationally designed 14 compounds, and the compounds that can pass ADMET may have the possibility of successful design.
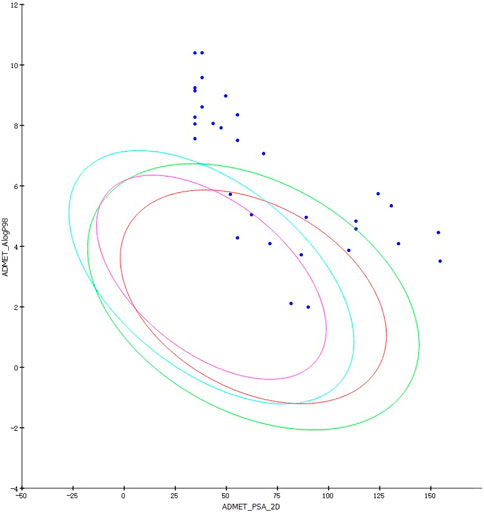
Figure 8. ADMET predictions for LUDI and rationally designed drugs. Blue ellipse: 99% confidence interval of the BBB model. Green ellipse: 99% confidence interval of the HIA model.
Finally, we obtained seven compounds (LUDI-6, LUDI-7, Design-5, Design-11, Design-12, Design-13, and Design-14) (Figure 9) and docked them to the PTP1B protein, then performed a 200 ns MD simulation and calculated the binding free energy. The structures of other compounds are shown in Supplementary Figures S11, S12.
3.5.4 Molecular dynamics simulations of designed compounds
An MD simulation was performed on the 7 designed compounds and the MD conditions were identical to those used for the 7 natural inhibitors of PTP1B.Supplementary Figures S5, S6 show the RMSD values of the designed drug system, and the fluctuation range of these seven designed drugs is between 0.75 and 1.75 Å, fluctuating about 1 Å. Supplementary Figures S7, S8 show that the RMSF value of the designed drug system has decreased fluctuations in the α3 and α6 helix regions, and it can be speculated that these compounds can be stably bound in the α3 and α6 helix regions. After conducting molecular dynamics simulations, the designed compounds were compared with the original protein structure (Supplementary Figures S9, S10). The results indicated that only the Design-12 complex exhibited higher fluctuations than the protein without the ligand at Thr230-Leu250, while the other complex systems displayed a more stable trend. This may be attributed to the force-inducing effect of the -CF3 group (De-la-Cruz-Martínez et al., 2021).
3.5.5 Energy calculation of inhibitors
Table 8 shows the free binding energies of these seven potential inhibitor ligands. It can be seen that the ΔGbind of LUDI-7 and Design-14 is poor. Due to their weaker binding free energies than complex-1 and complex-2, LUDI-6 and Design-5 were also excluded from the design (Figure 9).
MM-PB/SA calculations were performed for these compounds by selecting the last 100 ns stable trajectory. The three compounds with the best free binding energy are Design-11, 12, and 13, which are −23.6 kcal mol−1, −19.1 kcal mol−1, and −21.0 kcal mol-1, respectively. A comparison of the decomposition energies of key residues in different complex systems is shown in Supplementary Tables S5–S11. The contributions of the three inhibitors to the van der Waals interaction and electrostatic interaction were significantly greater than those of the previous compounds. Intermolecular interactions and energies indicate that the design of these three compounds merits further investigation. We believe that the results of these designs will provide a potential idea and a more convenient theoretical basis for the design of future drugs. Our findings, derived from the integration of free energy calculations, align with those reported by Ledy De-la-Cruz-Martinez et al., providing further evidence that the -CF3 group can enhance the efficacy of PTP1B inhibitors (De-la-Cruz-Martínez et al., 2021).
3.6 TOPKAT and druggability analysis
To evaluate the compounds’ toxicity, use the TOPKAT module in DS2020 and utilize the Drugflow platform to analyze druggability. Artificial intelligence (AI) is utilized on the Drugflow platform, which includes machine learning algorithms and deep learning algorithms that are more accurate, resulting in more accurate predictions. Using Drugflow’s selected MERT (Pre-train) method, we will analyze the druggability of these LUDI-designed and rationally designed compounds (Table 9).
Through toxicity testing and druggability analysis of the compounds, we finally found that compound Design-12 almost met all our needs, not only compared to Ligand-1 (−17.6 kcal mol−1) with a higher IC50 (2.10 μM) has better binding ability (Design-12, -19.1 kcal mol−1). Although the binding ability of Design-13 is less than that of Design-11 and Design-13, it has a very high safety risk, whether it is drug loss or liver damage. The binding free energy of Design-11 is the best of all compounds in terms of toxicity and oral LD50 in rats. The compound also exhibits selectivity for PTP1B, liver toxicity and oral LD50 in rats. Lipinski Rule does not accept it because it is relatively excellent.
Furthermore, the drugs designed in the experiment are easy to synthesize. Consequently, our research indicates that Design-12 may be an effective treatment for type 2 diabetes. The Design-11 has poor efficacy, but it still has the potential to be useful. In addition, Design-13 has excellent inhibitory properties.
4 Discussion
In this study, initially, natural pentacyclic triterpene PTP1B inhibitors sharing the identical parent molecule were retrieved from previous literature reports (Xu et al., 2018; Huang et al., 2022), and their interaction sites and interaction patterns were investigated through molecular docking and molecular dynamics. Subsequently, the binding free energy and the total interaction patterns were analyzed in accordance with the notions and approaches of Wang and Zheng (2018). Nevertheless, the research they carried out has constraints. For example, they investigated the inhibitory impacts of multiple compounds on the protein; however, whether the same holds true for compounds of the same type remains to be deliberated. Additionally, only straightforward toxicity verification was performed. This study aims to explore the binding mode of a class of compounds and conduct structural analysis by comparing compounds with the same parent molecule but different substituents, a method that is largely applicable to other pentacyclic triterpenoid compounds as well. Furthermore, the structural analysis of the system can investigate the motion-bound mode of the allosteric site of PTP1B. For instance, Phe196 establishes π-π stacking interactions and hydrophobic interactions with the pentacyclic triterpene, which can be detected in all the simulated systems. This phenomenon also suggests that the framework of the triterpenoid compound functions in anchoring the compound. Additionally, Phe280 can also establish supplementary π-stacking interactions in certain systems. This is similar to the results obtained by De-la-Cruz-Martínez et al. (2021); Ali et al. (2023); Sánchez-Alonso et al. (2021). Unfortunately, their study simply failed to account for the fact that the spatial positions of substituents also exert an influence on the activity of compounds, such as the matter regarding the hydrophilicity or hydrophobicity of the substituents in the directions at the C-3 and C-4 regions. Nevertheless, the structural analysis in this research might be capable of elucidating this aspect and conducting a more meticulous analysis of the spatial positions and states of the compounds. After conducting structural analysis, based on its conclusions, it is feasible to investigate whether elongating the length of substituents can enhance the stability of the compound or make modifications that are more liable to interact with the protein, thereby offering new concepts and insights for the compound to bind more readily to the protein. The results also suggest that the compounds subsequent to structural analysis frequently exhibit more superior binding capabilities. As hypothesized earlier, reducing the number of hydroxyl groups truly can enhance the contribution of binding free energy and attain a more stable system. The Design-12 compound did not decrease the number of hydroxyl groups but rather altered the position of the hydroxyl groups, and the contribution of the binding free energy was higher. The results manifested that the position of the hydroxyl groups could indeed cause the compound to undergo deflection motions in distinct directions.
5 Conclusion
In this study, Molecular dynamics (MD) simulation and Molecular Mechanics Poisson Boltzmann Surface Area (MM-PB/SA) were used to study the binding patterns of allosteric sites between compounds and PTP1B previously reported. By comparing the configurations of different ligands and the corresponding IC50 values, we found that the van der Waals interaction contributes the most energy, while electrostatic interactions contribute less energy. If the inhibitor can interact well with the critical residues of the corresponding site, it can show high inhibitory ability. Pentacyclic triterpenoids form mostly hydrophobic interactions with the α3 and α6 helical regions of PTP1B, among which Phe196 and Phe280 contribute the most hydrophobic interactions. We design 30 novel inhibitor molecules through LUDI and rational design methods. We calculated the binding free energy and analyzed the drugability of the designed LUDI-6, LUDI-7, Design-5, Design-11, Design-12, Design-13 and Design-14. The results showed that Design-12 was the most optimal inhibitor. Although Design-14 has good druggability analysis results, its binding free energy is deficient, thus, it will not be considered. There is a more stable binding energy in Design-12, which shows a reasonable range in various druggability analysis indicators. Even though the results of Design-11 are not acceptable, it has a higher binding free energy than Design-12, indicating a more significant inhibitory effect. The Design-13 also has excellent properties, but its oral LD50 in rats is inferior to that of Design-12. Our findings will provide new understanding and suggestions for future researchers to design new and effective inhibitors of PTP1B.
Data availability statement
The original contributions presented in the study are included in the article/Supplementary Material, further inquiries can be directed to the corresponding authors.
Author contributions
ZL: Formal Analysis, Supervision, Validation, Writing–original draft, Writing–review and editing. HG: Funding acquisition, Resources, Software, Supervision, Writing–review and editing. JZ: Formal Analysis, Methodology, Supervision, Writing–review and editing.
Funding
The author(s) declare that financial support was received for the research, authorship, and/or publication of this article. This work was financially supported by Natural Science Foundation of Shandong, China (Grant No. ZR2023MC059), High-end Talent Introduction “Double Hundred Plan” Special Foundation of Yantai City (Grant No. 612211012002) and High-end Talent Team Construction Foundation (Grant No. 108-10000318).
Conflict of interest
The authors declare that the research was conducted in the absence of any commercial or financial relationships that could be construed as a potential conflict of interest.
Publisher’s note
All claims expressed in this article are solely those of the authors and do not necessarily represent those of their affiliated organizations, or those of the publisher, the editors and the reviewers. Any product that may be evaluated in this article, or claim that may be made by its manufacturer, is not guaranteed or endorsed by the publisher.
Supplementary material
The Supplementary Material for this article can be found online at: https://www.frontiersin.org/articles/10.3389/fphar.2024.1423029/full#supplementary-material
References
Akyol, K., and Kilic, D. (2021). Discovery of novel and selective inhibitors targeting protein tyrosine phosphatase 1b (Ptp1b): virtual screening and molecular dynamic simulation. Comput. Biol. Med. 139, 104959. doi:10.1016/j.compbiomed.2021.104959
Alamri, M. A., Alawam, A. S., Alshahrani, M. M., Kawsar, S. M. A., and Prinsa, S. S. (2023). Establishing the role of iridoids as potential kirsten rat sarcoma viral oncogene homolog G12C inhibitors using molecular docking; molecular docking simulation; molecular mechanics Poisson-Boltzmann Surface Area; frontier molecular orbital theory; molecular electrostatic potential; and absorption, distribution, metabolism, excretion, and toxicity analysis. Excretion, Toxic. Analysis 28 (13), 5050. doi:10.3390/molecules28135050
Alberga, D., Trisciuzzi, D., Mansouri, K., Mangiatordi, G. F., and Nicolotti, O. (2019). Prediction of acute oral systemic toxicity using a multifingerprint similarity approach. Toxicol. Sci. 167 (2), 484–495. Epub 2018/10/30. doi:10.1093/toxsci/kfy255
Ali, M. Y., Park, S. E., Seong, S. H., Zamponi, G. W., Jung, H. A., and Choi, J. S. (2023). Ursonic acid from artemisia Montana exerts anti-diabetic effects through anti-glycating properties, and by inhibiting Ptp1b and activating the pi3k/akt signaling pathway in insulin-resistant C2c12 cells. Chemico-Biological Interact. 376, 110452. doi:10.1016/j.cbi.2023.110452
Andersen, J. N., Mortensen, O. H., Peters, G. H., Drake, P. G., Iversen, L. F., Olsen, O. H., et al. (2001). Structural and evolutionary relationships among protein tyrosine phosphatase domains. Mol. Cell. Biol. 21 (21), 7117–7136. doi:10.1128/MCB.21.21.7117-7136.2001
Bence, K. K., Delibegovic, M., Xue, B., Gorgun, C. Z., Hotamisligil, G. S., Neel, B. G., et al. (2006). Neuronal Ptp1b regulates body weight, adiposity and leptin action. Nat. Med. 12 (8), 917–924. doi:10.1038/nm1435
Berendsen, H. J. C., Postma, J. P. M., Gunsteren, W. F. V., Dinola, A., and Haak, J. R. (1984). Molecular dynamics with coupling to an external bath. J. Chem. Phys. 81, 3684–3690. doi:10.1063/1.448118
Böhm, H.-J. (1994). On the use of Ludi to search the fine chemicals directory for ligands of proteins of known three-dimensional structure. J. Computer-Aided Mol. Des. 8 (5), 623–632. doi:10.1007/BF00123669
Case, D., Cerutti, D. S., Cheatham, T., Darden, T., Duke, R., Giese, T. J., et al. (2017). Amber 2017. San Francisco: University of California.
Cho, H. (2013). “Chapter seventeen - protein tyrosine phosphatase 1b (Ptp1b) and obesity,” in Vitamins and hormones. Editor G. Litwack (Academic Press), 405–424.
Combs, A. P. (2010). Recent advances in the Discovery of competitive protein tyrosine phosphatase 1b inhibitors for the treatment of diabetes, obesity, and cancer. J. Med. Chem. 53 (6), 2333–2344. doi:10.1021/jm901090b
Comeau, A. B., Critton, D. A., Page, R., and Seto, C. T. (2010). A focused library of protein tyrosine phosphatase inhibitors. J. Med. Chem. 53 (18), 6768–6772. Epub 2010/08/25. doi:10.1021/jm100528p
Coskuner, O., and Uversky, V. N. (2017). Bmp-2 and bmp-9 binding specificities with alk-3 in aqueous solution with dynamics. J. Mol. Graph. Model. 77, 181–188. doi:10.1016/j.jmgm.2017.08.005
Darden, T., York, D., and Pedersen, L. (1993). Particle mesh Ewald: an N⋅log(N) method for Ewald sums in large systems. Large Syst. 98 (12), 10089–10092. doi:10.1063/1.464397
De-la-Cruz-Martínez, L., Duran-Becerra, C., González-Andrade, M., Páez-Franco, J. C., Germán-Acacio, J. M., Espinosa-Chávez, J., et al. (2021). Indole- and pyrazole-glycyrrhetinic acid derivatives as Ptp1b inhibitors: synthesis, in vitro and in silico studies. Molecules 26 (14), 4375. doi:10.3390/molecules26144375
Dong, J., Wang, N.-N., Yao, Z.-J., Zhang, L., Cheng, Y., Ouyang, D., et al. (2018). Admetlab: a platform for systematic admet evaluation based on a comprehensively collected admet database. J. Cheminformatics 10 (1), 29. doi:10.1186/s13321-018-0283-x
Elchebly, M., Payette, P., Michaliszyn, E., Cromlish, W., Collins, S., Loy, A. L., et al. (1999). Increased insulin sensitivity and obesity resistance in mice lacking the protein tyrosine phosphatase-1b gene. Sci. (New York, NY) 283 (5407), 1544–1548. Epub 1999/03/05. doi:10.1126/science.283.5407.1544
Federico, L. B., Silva, G. M., Gomes, S. Q., Francischini, I. A. G., Barcelos, M. P., dos Santos, C. B. R., et al. (2021). Potential colchicine binding site inhibitors unraveled by virtual screening, molecular dynamics and Mm/Pbsa. Comput. Biol. Med. 137, 104817. doi:10.1016/j.compbiomed.2021.104817
Gao, S., Wang, L., Bai, F., and Xu, S. (2023). In silico Discovery of food-derived phytochemicals against asialoglycoprotein receptor 1 for treatment of hypercholesterolemia: pharmacophore modeling, molecular docking and molecular dynamics simulation approach. J. Mol. Graph. Model. 125, 108614. doi:10.1016/j.jmgm.2023.108614
Gordon, J. C., Myers, J. B., Folta, T., Shoja, V., Heath, L. S., and Onufriev, A. (2005). H++: a server for estimating pkas and adding missing hydrogens to macromolecules. Nucleic acids Res. 33 (Web Server issue), W368–W371. Epub 2005/06/28. doi:10.1093/nar/gki464
Hassan, H. A., Abdelwahab, S. F., Al-Khdhairawi, A., Al, Z. M. K., Rehman, H. M., Abdel-Rahman, I. M., et al. (2023). Exploring the therapeutic potential of galidesivir analogs against zaire ebolavirus protein 24 (V24): database screening, molecular docking, drug-relevant property evaluation and molecular dynamics simulations. J. Biomol. Struct. Dyn., 1–11. doi:10.1080/07391102.2023.2236720
Huang, Q., Pan, Y., Wu, S.-L., Huang, X.-Y., Hu, J., Ma, Y.-B., et al. (2022). Antidiabetic triterpenoids from the leaves of Paeonia suffruticosa and Paeonia delavayi. Phytochem. Lett. 48, 87–93. doi:10.1016/j.phytol.2022.02.009
Hünenberger, P. H., Mark, A. E., and van Gunsteren, W. F. (1995). Fluctuation and cross-correlation analysis of protein motions observed in nanosecond molecular dynamics simulations. J. Mol. Biol. 252 (4), 492–503. doi:10.1006/jmbi.1995.0514
Hunter, T. (1995). Protein kinases and phosphatases: the yin and yang of protein phosphorylation and signaling. Cell 80 (2), 225–236. doi:10.1016/0092-8674(95)90405-0
Hunter, T. (2000). Signaling--2000 and beyond. Cell 100 (1), 113–127. Epub 2000/01/27. doi:10.1016/s0092-8674(00)81688-8
Jiang, Y., and Gao, H. (2019). Pharmacophore-based drug design for the identification of novel butyrylcholinesterase inhibitors against alzheimer's disease. Phytomedicine 54, 278–290. doi:10.1016/j.phymed.2018.09.199
Jiang, Y., Yang, R.-Y., Qu, Z.-X., Hou, G.-G., Cong, W., Wang, C.-H., et al. (2022). Valeriaquinone a, a unique anthraquinone–coumarin hybrid with selective inhibition of Ptp1b from knoxia valerianoides. Chin. Chem. Lett. 33 (6), 2919–2922. doi:10.1016/j.cclet.2021.10.072
Jorgensen, W. L., Chandrasekhar, J., Madura, J. D., Impey, R., and Klein, M. L. (1983). Comparison of simple potential functions for simulating liquid water. Water 79, 926–935. doi:10.1063/1.445869
Kollman, P. A., Massova, I., Reyes, C., Kuhn, B., Huo, S., Chong, L., et al. (2000). Calculating structures and free energies of complex molecules: combining molecular mechanics and continuum models. Accounts Chem. Res. 33 (12), 889–897. Epub 2000/12/22. doi:10.1021/ar000033j
Kong, X., Sun, H., Pan, P., Zhu, F., Chang, S., Xu, L., et al. (2018). Importance of protein flexibility in molecular recognition: a case study on type-I1/2 inhibitors of Alk. Phys. Chem. Chem. Phys. 20 (7), 4851–4863. doi:10.1039/C7CP08241J
Krishnan, N., Konidaris, K. F., Gasser, G., and Tonks, N. K. (2018). A potent, selective, and orally bioavailable inhibitor of the protein-tyrosine phosphatase Ptp1b improves insulin and leptin signaling in animal models. J. Biol. Chem. 293 (5), 1517–1525. doi:10.1074/jbc.C117.819110
Krishnan, N., Koveal, D., Miller, D. H., Xue, B., Akshinthala, S. D., Kragelj, J., et al. (2014). Targeting the disordered C terminus of Ptp1b with an allosteric inhibitor. Nat. Chem. Biol. 10 (7), 558–566. Epub 2014/05/23. doi:10.1038/nchembio.1528
Krishnan, N., and Tonks, N. K. (2015). Anxious moments for the protein tyrosine phosphatase Ptp1b. Trends Neurosci. 38 (8), 462–465. doi:10.1016/j.tins.2015.06.006
Lam, D. W., and LeRoith, D. (2012). The worldwide diabetes epidemic. Curr. Opin. Endocrinol. Diabetes Obes. 19 (2), 93–96. doi:10.1097/MED.0b013e328350583a
Lantz, K. A., Hart, S. G., Planey, S. L., Roitman, M. F., Ruiz-White, I. A., Wolfe, H. R., et al. (2010). Inhibition of Ptp1b by trodusquemine (Msi-1436) causes fat-specific weight loss in diet-induced obese mice. Obes. (Silver Spring, Md) 18 (8), 1516–1523. Epub 2010/01/16. doi:10.1038/oby.2009.444
Lessard, L., Stuible, M., and Tremblay, M. L. (2010). The two faces of Ptp1b in cancer. Biochimica Biophysica Acta (BBA) - Proteins Proteomics 1804 (3), 613–619. doi:10.1016/j.bbapap.2009.09.018
Lima Silva, W. J., and Ferreira de Freitas, R. (2023). Assessing the performance of docking, fep, and Mm/Gbsa methods on a series of Klk6 inhibitors. J. Comput. Aided Mol. Des. 37 (9), 407–418. Epub 2023/06/28. doi:10.1007/s10822-023-00515-3
Ma, X. H., Wang, R., Xue, Y., Li, Z. R., Yang, S. Y., Wei, Y. Q., et al. (2008). Advances in machine learning prediction of toxicological properties and adverse drug reactions of pharmaceutical agents. Curr. drug Saf. 3 (2), 100–114. Epub 2008/08/12. doi:10.2174/157488608784529224
Ma, Y., Ding, T.-T., Liu, Y.-Y., Zheng, Z.-H., Sun, S.-X., Zhang, L.-S., et al. (2021). Design, synthesis, biological evaluation and molecular dynamics simulation studies of imidazolidine-2,4-dione derivatives as novel Ptp1b inhibitors. Biochem. Biophysical Res. Commun. 579, 40–46. doi:10.1016/j.bbrc.2021.09.047
Maccari, R., Wolber, G., Genovese, M., Sardelli, G., Talagayev, V., Balestri, F., et al. (2023). Designed multiple ligands for the treatment of type 2 diabetes mellitus and its complications: Discovery of (5-arylidene-4-oxo-2-thioxothiazolidin-3-Yl)Alkanoic acids active as novel dual-targeted ptp1b/akr1b1 inhibitors. Eur. J. Med. Chem. 252, 115270. doi:10.1016/j.ejmech.2023.115270
Maier, J. A., Martinez, C., Kasavajhala, K., Wickstrom, L., Hauser, K. E., and Simmerling, C. (2015). Ff14sb: improving the accuracy of protein side chain and backbone parameters from Ff99sb. J. Chem. theory Comput. 11 (8), 3696–3713. Epub 2015/11/18. doi:10.1021/acs.jctc.5b00255
Olloquequi, J., Cano, A., Sanchez-López, E., Carrasco, M., Verdaguer, E., Fortuna, A., et al. (2022). Protein tyrosine phosphatase 1b (Ptp1b) as a potential therapeutic target for neurological disorders. Biomed. and Pharmacother. 155, 113709. doi:10.1016/j.biopha.2022.113709
Oner, E., Demirhan, I., Miraloglu, M., Yalin, S., and Kurutas, E. B. (2023). Investigation of antiviral substances in covid 19 by molecular docking: in silico study. Afr. health Sci. 23 (1), 23–36. Epub 2023/08/07. doi:10.4314/ahs.v23i1.4
Pan, D., Niu, Y., Ning, L., Zhang, Y., Liu, H., and Yao, X. (2016). Computational study on the binding and unbinding mechanism of Hcv Ns5b with the inhibitor Gs-461203 and substrate using conventional and steered molecular dynamics simulations. Chemom. Intelligent Laboratory Syst. 156, 72–80. doi:10.1016/j.chemolab.2016.05.015
Park, J., Lee, H.-S., Kim, H., and Choi, J.-M. (2023). Conformational landscapes of artificial peptides predicted by various force fields: are we ready to simulate Β-amino acids? Phys. Chem. Chem. Phys. 25 (10), 7466–7476. doi:10.1039/D2CP05998C
Paul, A., Sarkar, A., Banerjee, T., Maji, A., Sarkar, S., Paul, S., et al. (2023). Structural and molecular insights of protein tyrosine phosphatase 1b (Ptp1b) and its inhibitors as anti-diabetic agents. J. Mol. Struct. 1293, 136258. doi:10.1016/j.molstruc.2023.136258
Peters, G. H., Branner, S., Møller, K. B., Andersen, J. N., and Møller, N. P. H. (2003). Enzyme kinetic characterization of protein tyrosine phosphatases. Biochimie 85 (5), 527–534. doi:10.1016/S0300-9084(03)00036-1
Ryckaert, J.-P., Ciccotti, G., and Berendsen, H. J. C. (1977). Numerical integration of the cartesian equations of motion of a system with constraints: molecular dynamics of N-Alkanes. J. Comput. Phys. 23 (3), 327–341. doi:10.1016/0021-9991(77)90098-5
Sánchez-Alonso, P., Griera, M., García-Marín, J., Rodríguez-Puyol, M., Alajarín, R., Vaquero, J. J., et al. (2021). Pyrrolo[1,2-a]Quinoxal-5-Inium salts and 4,5-Dihydropyrrolo[1,2-a]Quinoxalines: synthesis, activity and computational docking for protein tyrosine phosphatase 1b. Bioorg. and Med. Chem. 44, 116295. doi:10.1016/j.bmc.2021.116295
Shi, D., Bai, Q., Zhou, S., Liu, X., Liu, H., and Yao, X. (2018). Molecular dynamics simulation, binding free energy calculation and unbinding pathway analysis on selectivity difference between Fkbp51 and Fkbp52: insight into the molecular mechanism of isoform selectivity. Proteins 86 (1), 43–56. Epub 2017/10/13. doi:10.1002/prot.25401
Shinde, R. N., and Sobhia, M. E. (2013). Binding and discerning interactions of Ptp1b allosteric inhibitors: novel insights from molecular dynamics simulations. J. Mol. Graph. Model. 45, 98–110. doi:10.1016/j.jmgm.2013.08.001
Singh, S., Singh Grewal, A., Grover, R., Sharma, N., Chopra, B., Kumar Dhingra, A., et al. (2022). Recent updates on development of protein-tyrosine phosphatase 1b inhibitors for treatment of diabetes, obesity and related disorders. Bioorg. Chem. 121, 105626. doi:10.1016/j.bioorg.2022.105626
Sun, H., Li, Y., Shen, M., Tian, S., Xu, L., Pan, P., et al. (2014). Assessing the performance of mm/Pbsa and Mm/Gbsa methods. 5. Improved docking performance using high solute dielectric constant Mm/Gbsa and Mm/Pbsa Rescoring. Phys. Chem. Chem. Phys. 16 (40), 22035–22045. doi:10.1039/C4CP03179B
Tonks, N. K. (2003). Ptp1b: from the sidelines to the front lines. FEBS Lett. 546 (1), 140–148. doi:10.1016/S0014-5793(03)00603-3
Tonks, N. K., Diltz, C. D., and Fischer, E. H. (1988). Characterization of the major protein-tyrosine-phosphatases of human placenta. J. Biol. Chem. 263 (14), 6731–6737. doi:10.1016/S0021-9258(18)68703-4
Uberuaga, B. P., Anghel, M., and Voter, A. F. (2004). Synchronization of trajectories in canonical molecular-dynamics simulations: observation, explanation, and exploitation. J. Chem. Phys. 120 (14), 6363–6374. doi:10.1063/1.1667473
Verma, M., Gupta, S. J., Chaudhary, A., and Garg, V. K. (2017). Protein tyrosine phosphatase 1b inhibitors as antidiabetic agents – a brief review. Bioorg. Chem. 70, 267–283. doi:10.1016/j.bioorg.2016.12.004
Wang, H., Kellogg, G. E., Xu, P., and Zhang, Y. (2018). Exploring the binding mechanisms of diaminopimelic acid analogs to meso-diaminopimelate dehydrogenase by molecular modeling. J. Mol. Graph. Model. 83, 100–111. doi:10.1016/j.jmgm.2018.05.011
Wang, J., Wang, W., Kollman, P. A., and Case, D. A. (2006). Automatic atom type and bond type perception in molecular mechanical calculations. J. Mol. Graph. and Model. 25 (2), 247–260. Epub 2006/02/07. doi:10.1016/j.jmgm.2005.12.005
Wang, J., Wolf, R. M., Caldwell, J. W., Kollman, P. A., and Case, D. A. (2004). Development and testing of a general amber force field. J. Comput. Chem. 25 (9), 1157–1174. Epub 2004/04/30. doi:10.1002/jcc.20035
Wang, Q., Fu, X.-Q., and Zheng, Q.-C. (2020). Exploring the allosteric mechanism of protein tyrosine phosphatase 1b by molecular dynamics simulations. J. Biomol. Struct. Dyn. 38 (13), 4040–4047. doi:10.1080/07391102.2019.1682049
Wang, X.-S., and Zheng, Q.-C. (2018). Theoretical research in structure characteristics of different inhibitors and differences of binding modes with cbp bromodomain. Bioorg. and Med. Chem. 26 (3), 712–720. doi:10.1016/j.bmc.2017.12.040
Wen, J., Scoles, D. R., and Facelli, J. C. (2017). Effects of the enlargement of polyglutamine segments on the structure and folding of ataxin-2 and ataxin-3 proteins. J. Biomol. Struct. and Dyn. 35 (3), 504–519. Epub 2016/02/11. doi:10.1080/07391102.2016.1152199
Wiesmann, C., Barr, K. J., Kung, J., Zhu, J., Erlanson, D. A., Shen, W., et al. (2004a). Allosteric inhibition of protein tyrosine phosphatase 1b. Nat. Struct. and Mol. Biol. 11 (8), 730–737. doi:10.1038/nsmb803
Wiesmann, C., Barr, K. J., Kung, J., Zhu, J., Erlanson, D. A., Shen, W., et al. (2004b). Allosteric inhibition of protein tyrosine phosphatase 1b. Nat. Struct. Mol. Biol. 11 (8), 730–737. Epub 2004/07/20. doi:10.1038/nsmb803
Xu, J., Cao, J., Yue, J., Zhang, X., and Zhao, Y. (2018). New triterpenoids from acorns of Quercus liaotungensis and their inhibitory activity against α -glucosidase, α -amylase and protein-tyrosine phosphatase 1B. J. Funct. Foods 41, 232–239. doi:10.1016/j.jff.2017.12.054
Keywords: protein tyrosine phosphatase 1B, inhibitor, MD simulations, rational design, LUDI
Citation: Zhan J, Liu Z and Gao H (2024) Theoretical study on the design of allosteric inhibitors of diabetes associated protein PTP1B. Front. Pharmacol. 15:1423029. doi: 10.3389/fphar.2024.1423029
Received: 25 April 2024; Accepted: 05 August 2024;
Published: 22 August 2024.
Edited by:
Mohamed Abdo Rizk, Mansoura University, EgyptReviewed by:
Francisco Cortés-Benítez, Autonomous Metropolitan University Xochimilco Campus, MexicoIsman Kurniawan, Telkom University, Indonesia
Copyright © 2024 Zhan, Liu and Gao. This is an open-access article distributed under the terms of the Creative Commons Attribution License (CC BY). The use, distribution or reproduction in other forums is permitted, provided the original author(s) and the copyright owner(s) are credited and that the original publication in this journal is cited, in accordance with accepted academic practice. No use, distribution or reproduction is permitted which does not comply with these terms.
*Correspondence: Hongwei Gao, Z2FvaG9uZ3czNjlAbGR1LmVkdS5jbg==
†These authors have contributed equally to this work and share first authorship