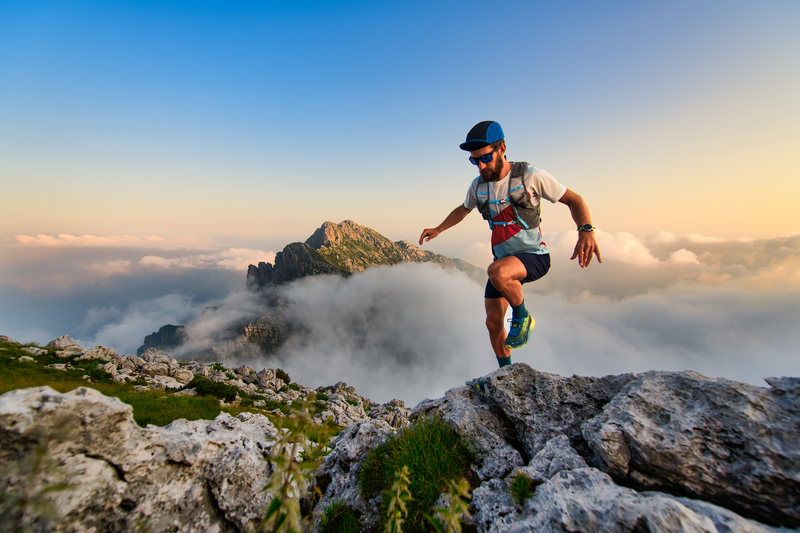
94% of researchers rate our articles as excellent or good
Learn more about the work of our research integrity team to safeguard the quality of each article we publish.
Find out more
SYSTEMATIC REVIEW article
Front. Pharmacol. , 20 December 2024
Sec. Experimental Pharmacology and Drug Discovery
Volume 15 - 2024 | https://doi.org/10.3389/fphar.2024.1418111
This article is part of the Research Topic Terrestrial and Marine Natural Products and Their Synthetic Scaffolds for Chronic Diseases: A Ray of Hope - Volume II View all 7 articles
Introduction: An abundance of experimental evidence indicates that icariin (ICA) could potentially exert an anti-tumor effect on ovarian cancer (OC). Nevertheless, the reliability of this evidence remains ambiguous. This study aimed to explore the impact of ICA on OC and the underlying mechanisms.
Methods: Bioinformatics analysis was employed to pinpoint ICA-targeted genes and signaling pathways implicated in OC, utilizing network pharmacology. Subsequently, PubMed, EMBASE, and Web of Science databases were systematically searched from 2001 through June 2023 for in vitro trials evaluating the anti-tumor efficacy of conventional ICA versus placebo in OC. The pathways and genes identified in the literature were recorded, and the therapeutic targets were statistically analyzed and compared with the predicted targets from network pharmacology to confirm the precision of the targets.
Results and Discussion: Fourteen target genes were validated with success. The pathways corresponding to the remaining genes—excluding these 14—were analyzed and found to be primarily associated with cell apoptosis, anti-tumor, and other related pathways. Out of the 76 studies retrieved, eight fulfilled the inclusion criteria. The subsequent meta-analysis suggested that ICA treatment was significantly correlated with reduced cell growth and induced apoptosis. This study demonstrated a certain efficacy of ICA compared to placebo in enhancing anti-tumor outcomes, characterized by increased abilities in reducing cell growth and inducing apoptosis. The pathways involved in the therapeutic effect may be linked to cell apoptosis and anti-tumor mechanisms.
Ovarian cancer (OC) ranks as the third most prevalent form of gynecological tumor (Zheng et al., 2021). The majority of OC cases arise from epithelial cells. Research has indicated that exposure to estrogen and progesterone may elevate the risk of OC, particularly among postmenopausal women (Zhang Y. F. et al., 2022). This malignancy can lead to reduced fertility in women (Zhang et al., 2021). In clinical settings, numerous medications that stimulate follicular development are hormone-based (Zhang et al., 2024), However, for individuals with OC, drugs that neither encourage cancer cell proliferation nor follicular development are preferable for those seeking to maintain fertility while managing the disease (Yu et al., 2023). Consequently, identifying such medications is a pressing necessity.
Over recent years, the advancement of clinical research and the comprehensive adoption of standardized diagnostic and treatment protocols have led to the widespread adoption of gene detection technologies, including BRCA and HRD (Morand et al., 2021). Moreover, the utilization of targeted therapeutic agents has ushered OC into an era of precision medicine. The full-course management treatment model, which encompasses surgery, chemotherapy, and maintenance therapy, has also seen continuous progress, offering increasing benefits to a growing number of patients and their families (Munoz-Zuluaga et al., 2020). In the realm of pharmaceuticals, ongoing research is being conducted, with medical researchers dedicated to discovering improved drug treatment options for patients (Moubarak et al., 2022).
Protecting the fertility in cancer patients has become an increasingly important issue. It is particularly difficult to protect fertility without stimulating cancer cell proliferation. Traditional Chinese medicine has played a unique role in this regard, becoming the first choice for many cross-disease patients due to its wide range of target areas and bidirectional regulatory function (Lu et al., 2023). The relatively minor side effects, high-quality and affordable nature of traditional Chinese medicine promote its clinical use. Many effective traditional Chinese medicines have been discovered. The discovery of Chinese medicines has been made. Further development of traditional Chinese medicines or herbal extracts would be an effective strategy for optimizing treatment plans for ovarian cancer patients seeking fertility (Chelliah and van der Graaf, 2022).
Although traditional chemotherapy drugs have strong anti-cancer effects, they also have significant side effects. Many patients cannot tolerate these side effects and often give up treatment after a few cycles of chemotherapy, choosing targeted drug therapy instead. Although some targeted drugs are effective and have fewer side effects compared to traditional chemotherapy drugs, they are more expensive and have a single target; thus, they cannot have a common effect on other diseases, such as monoclonal antibody drugs like Olaparib (Gao et al., 2022). Traditional Chinese classical prescriptions for the treatment of OC have multiple targets and relatively few side effects but their components are complex and difficult to separate and extract, such as Guizi-Fulling Wan (Wang X. et al., 2022). Extracts from Chinese medicine have significant therapeutic effects but many side effects, such as Paclitaxel (Lau et al., 2020), Bufalin (Dou et al., 2021), etc. Some drugs are still in the research stage, including synthetic drugs and traditional Chinese medicine extracts, which only have anti-tumour effects. These include Agrimonolide (Liu et al., 2022), Momordica charantia (Chan et al., 2020), Curcumin (Sun and Fang, 2021), FBP1 (Li et al., 2021), etc., and these do not have a strong promotional effect on female fertility. However, ICA not only has anti-tumour effects but also has protective effects on fertility via improving oocyte quality (Szabó et al., 2022). Therefore, ICA is the best choice for OC patients who are seeking fertility.
An extensive literature search revealed that the traditional Chinese medicine extract icariin (ICA) promotes the proliferation of ovarian granulosa cells and inhibits the proliferation of OC cells, and has a bidirectional regulatory effect on both normal and OC cells (Wang et al., 2019). ICA is the main effective component of the natural drug Epimedii herba, has a well-defined chemical structure, which has an active therapeutic effect on endocrine disorders, infertility and other reproductive diseases (Niu et al., 2022). ICA has a clear positive effect on the nervous system (Lifeng et al., 2023), reproductive system (Tao et al., 2017), circulatory system (Xie et al., 2015), digestive system (Zhang et al., 2008), respiratory system (Li et al., 2023), motor system (Liu and Fang, 2022), urinary system (Chen et al., 2022), endocrine and metabolic diseases (Su et al., 2023) and reproductive system diseases. It can also improve the quality of oocytes (Ye et al., 2017), has a clear anti-inflammatory (Jiao et al., 2018) and anti-oxidant (Park et al., 2022) effect, and can regulate endocrine metabolism (Wang et al., 2017), immune function (Zhao et al., 2019) and exert an anti-tumour effect (Xie et al., 2019).
However, the effect of this ICA on OC cells is still in the cell research stage and there is limited literature data to support its efficacy. Therefore, to accurately evaluate its effect on OC cells, a systematic evaluation of eight key literature reports was undertaken to analyse the pathways identified in the literature, gain a deep understanding of the molecular mechanism of ICA on OC and ascertain future research directions.
We employed network pharmacology to pinpoint the efficacious compounds in Epimedium, and subsequently, through a review of the literature, we ascertained the principal components. We then performed a cross-analysis with ovarian cancer-related pathways to identify those pertinent to both. The validation of these pathways was executed through an examination of published literature, utilizing meta-analysis to ascertain the studies with the highest reference value. The detailed methodology is as follows:
In this investigation, bioinformatics was employed to pinpoint genes and signaling pathways targeted by ICA in the context of OC. Through gene enrichment analysis, the study identified the KEGG pathways associated with both ICA-targeted genes and OC, along with the hub genes (Zhou et al., 2022). Employing a simulated approach, this research innovatively applied network pharmacology to forecast the therapeutic targets of ICA for OC treatment. Subsequently, a systematic evaluation of existing animal studies on ICA’s efficacy in treating OC was conducted. The therapeutic targets reported in the literature were statistically analyzed and compared with the predicted targets from network pharmacology to validate the latter’s precision. A common gene was identified between the two sets of targets, confirming the accuracy of the network pharmacology predictions.
The Chinese Medicine System Pharmacology (TCMSP) database was used to identify the main active compounds of Epimedii herba (Zhong et al., 2022), including ICA. The parameters used to select the active ingredients were an oral bioavailability (OB) of 30% and a drug-likeness (DL) of 0.18. PubChem (https://pubchem.ncbi.nlm.nih.gov) was used to extract the chemical structure of each active compound using SMILES. The predicted targets were found using the SMILES string in SwissTargetPrediction (www.swisstargetprediction.ch) (Zhao Y. et al., 2022). A probability of 0.1 was the screening condition for the target protein to predict the components (Zhao M. et al., 2023). The targets acquired were standardized to gene names by querying the UniProt Database (http://www.uniprot.org) using the “Homo sapiens” species as a filter (Zhao H. et al., 2023).
The DisGeNET (www.disgenet.org) and GeneCards (www.genecards.org) databases were used to identify the genes and mutation sites associated with human diseases (Zhang Y. et al., 2022). The Venn Diagram Tool v2.1.0 from the Bioinformatics platform (www.bioinformatics.com.cn) was used to identify the shared targets between the composition and the target (Zhang Q. et al., 2022).
The STRING (https://string-db.org/) database and Cytoscape v3.7.2 software were used to construct the PPI network (Zhang et al., 2023).
The Database for Annotation, Visualization and Integrated Discovery (DAVID) was used for comprehensive gene annotation and resource analysis (https://david.ncifcrf.gov/home.jsp) (Zhang D. Y. et al., 2022). The preanalysis species was set to “Homo sapiens”. The Gene Ontology (GO) analysis encompassed three principal categories: biological processes (BP), cellular components (CC), and molecular functions (MF). Subsequently, the Kyoto Encyclopedia of Genes and Genomes (KEGG) pathway enrichment analysis was conducted. The enriched GO terms and the enrichment dot plots were derived from data analysis executed on the Bioinformatics online platform (Zeng et al., 2023).
The ingredient-target-pathway network was constructed using Cytoscape software, incorporating intersection targets and pathways, and selecting six active ingredients. In this network, nodes of various colors signify different clusters, while the edges depict the interrelationships between these nodes (Yue et al., 2022).
The meta-analysis was conducted utilizing Review Manager 5.3, a tool provided by the Cochrane Collaboration, and adhered to the guidelines set forth in the Cochrane Handbook for Systematic Reviews of Interventions.
The PubMed, EMBASE and Web of Science databases were searched. Only English-language articles published from 2001 to June 2023 were included. The databases were searched using the following search terms in titles and abstracts (also in combination with MESH terms): (“Icariin” OR “ICA”) AND (“cancer” OR “ovarian cancer” OR “cancer cell” OR “tumour” OR “malignancy” OR “cancer cell line” OR “neoplasm cell line”) AND (“apoptosis” OR “cancer apoptosis” OR “apoptotic cancer cell” OR “apoptosis in vitro” OR “caspase-3”). The electronic search was complemented by manual searching of the references in the included publications. A study was included if it met specific inclusion and exclusion criteria (Table 1). No restrictions in terms of the year of publication were applied.
From the pool of eligible publications, the subsequent data—encompassing the first author, publication year, cell species, intervention duration and dosage, and outcome measures (including cell growth reduction capability, apoptosis induction capability, and pathways involved in OC)—were meticulously extracted by two independent authors (CB and SC). Discrepancies were reconciled through discussion, culminating in a consensus on all extracted data. Any disagreements among reviewers regarding data extraction were resolved via consultation with a third reviewer.
All the outcome measures were continuous data (e.g., cell growth reduction ability and apoptosis induction ability), so random-effects meta-analyses of these data with standardised mean differences (SMD) and 95% confidence intervals (95% CI) were performed. A p-value of < 0.05 was considered statistically significant. The analysis method can be found in previous articles (Cao et al., 2022). Sensitivity analysis was conducted to evaluate whether a single study affected the overall effect size by removing one study at each stage. This was conducted when an experimental study had a Stata value of greater than 5. Additionally, publication bias was evaluated quantitatively using Begg’s and Egger’s tests, which were conducted using Stata. A significant publication bias was indicated if the p-value was <0.05. Review Manager 5.3 (the Cochrane Collaboration) and STATA 16.0 (Stata Corporation) were used for the analysis.
OC-related targets were identified using DisGeNET and GeneCards. Genes with a relevance score above 1 were selected in the Genecard database and genes with an nSNPs number above 1 were selected in the DisGeNET database. After removing duplicates, 1,389 genes were obtained.
Based on the findings from the TCMSP, the UniProt, and the SwissTargetPrediction database, 1,075 targets associated with ICA and 187 targets related to OC therapy were identified. Figure 1 illustrates the Venn diagram representing these two sets of targets, with the overlapping targets considered to encompass potential OC treatment targets.
The intersection targets were imported into the STRING database to generate a PPI functional network. The PPI map contained 187 intersection targets (refer to Figure 2). Targets that were not connected to the network were subsequently removed, and the interactions of drug-targeted genes were constructed using Cytoscape version 3.7.2 (refer to Figure 3). Hub gene values were evaluated based on degree, betweenness, and closeness centrality. Genes colored yellow represented hub genes, which were most closely related to ICA, whereas genes colored green were ordinary genes, indicating secondary relevance to ICA. The roles of these targets in various diseases are discussed in detail below.
Employing the Database for Annotation, Visualization and Integrated Discovery (DAVID), a metascape enrichment analysis was conducted on the intersecting genes to explore biological processes (BP), cellular components (CC), and molecular functions (MF) under Gene Ontology (GO) terms for ICA (refer to Supplementary Figures S1-S3). The p-value established the outcomes of the correlation test. The leading 20 terms within each category were organized by their logP values, ranging from smallest to largest, and a histogram was subsequently generated. The prominent terms identified included response to ethanol, positive regulation of smooth muscle cell proliferation, response to L-ascorbic acid, and response to hypoxia within BPs; extracellular region, extracellular exosome, mitochondrion, and extracellular space within CCs; and haem binding, identical protein binding, and insulin receptor substrate binding within MFs.
The top 20 shared KEGG pathways associated with ICA-targeted genes and osteosarcoma (OS) were identified using a bubble chart (Figure 4) and categorized based on the logP value, ranging from the smallest to the largest. The concentrations and p-values denote the highly correlated manner in which the active ingredients manifest their therapeutic effects on OS.
The mechanisms and pathways through which ICA influences OC were elucidated in eight articles. The genes coding for the proteins were pinpointed using the STRING database and subsequently contrasted with the genes derived from network pharmacology analysis conducted in the preceding phase. A significant overlap was observed between the two gene sets, with 14 genes that were both implicated in the literature and anticipated by network pharmacology: BIRC5, MMP9, CDK2, PIK3CA, ERBB2, MTOR, IL2, KDR, PTGS2, AKT1, TNF, PTEN, BCL2, and RELA (refer to Supplementary Figure S4). The pathways associated with the remaining genes from the 187 intersection targets—excluding these 14 genes—were scrutinized using the DAVID database, as depicted in Supplementary Tables S1–S3 and Supplementary Figure S5. The top 20 shared KEGG pathways corresponding to ICA and OC intersection genes that had not been previously validated were identified using a bubble chart (Supplementary Figure S6) and ranked based on their logP values from smallest to largest.
The process of selecting studies is illustrated in Figure 5. An electronic search along with the examination of supplementary sources resulted in the identification of 76 publications. After removing duplicates, 68 studies were kept. Upon reviewing the titles and abstracts, 34 studies were excluded. As a result, eight full-text articles were considered suitable for assessment.
The eight chosen in vitro studies were published between 2001 and 2023. The cell species encompassed SKOV3, SKVCR, A2780, and OVCAR3. The concentration of ICA varied from 6.5 µM to 80 µg·mL–1, and the duration of drug exposure ranged from 24 to 72 h. The primary characteristics and outcomes of the included studies are summarized in Table 2.
The publications assessed the capacity to diminish cancer cell proliferation using two distinct metrics. The first metric was the rate of OC cell growth reduction, while the second was the IC50 (μM) value for the OC cells. Due to the differing calculation methods and units of these two metrics, they were analyzed independently. The IC50 for the ICA-treated group compared to the control group was as follows: n = 7, SMD = −8.28, 95% CI (−13.33, −3.23), p = 0.001; heterogeneity: X2 = 1.33, p = 0.25; I2 = 25%. The proliferation inhibition rate for the ICA-treated group compared to the control group was as follows: n = 13, SMD = −7.56, 95% CI (−11.06, −4.06), p < 0.0001; heterogeneity: X2 = 1.52, p = 0.68; I2 = 0%.
The test for subgroup differences result was P = 0.82, which was greater than the 0.05 threshold. The combined results indicated that ICA could significantly suppress cancer cell growth compared with the control group [n = 20, SMD = −7.80, 95% CI (−10.67, −4.92), p < 0.00001; heterogeneity: X2 = 2.90, p = 0.71; I2= 0%, Figure 6]. Funnel plots were constructed to show the asymmetry of the effects of ICA on cell growth reduction (Figure 8). Begg’s test revealed no statistical significance (p = 0.060), whereas Egger’s test revealed statistical significance [95% CI (−2.62, −0.72); p = 0.008].
The capacity to trigger apoptosis in cancer cells was assessed using two distinct methodologies as detailed in the publications. The first method involved the use of Annexin V/PI Flow Cytometry (FCM), while the second method utilized Western blot analysis to detect caspase-3 protein levels. Given that the calculation methods and units of measurement for these two techniques are different, two subgroups were established based on the varying units. The results for the apoptosis rate in the ICA-treated group compared to the control group, as determined by FCM, the results were as follows: n = 17, SMD = 5.10, 95% CI (3.02, 7.19), p < 0.00001; heterogeneity: X2 = 2.54, p = 0.64; I2 = 0%. For the Western blot of caspase3 protein in the ICA-treated group compared to that in the control group, the results were as follows: n = 6, SMD = −2.56, 95% CI (−4.43, −0.69), p < 0.00001; heterogeneity: X2 = 0.15, p = 0.70; I2 = 0%.
The test for subgroup differences yielded a result of P = 0.10 > 0.05, indicating that there was no significant difference between the groups and that the comparison between them was statistically significant. The combined results suggested that ICA could significantly enhance the induction of cancer cell apoptosis compared with the control group [n = 23, SMD = 5.51, 95% CI (3.47, 7.54), p < 0.00001; heterogeneity: X2 = 5.43, p = 0.49; I2 = 0%, Figure 7]. The funnel plots indicated asymmetry in the effects of ICA on apoptosis induction ability (Figures 8, 9). Begg’s test revealed statistical significance (p = 0.003), as did Egger’s test [95% CI (1.35, 2.32); p = 0.000].
The sensitivity analyses of cell growth reduction and apoptosis induction ability were conducted by removing one study at each stage. The results indicated that no individual study significantly affected the pooled effect sizes. The sensitivity analysis of cell growth reduction ability results [Estimate = −7.80, 95% CI (−10.67, −4.92), Figure 10] indicated that there was significantly greater cell growth reduction in the experimental group compared to the control group. The sensitivity analysis of apoptosis induction [Estimate = 5.35, 95% CI (3.25, 7.46), Figure 11] indicated that there was significantly greater apoptosis induction in the experimental group compared to the control group.
Network pharmacology is a new field in drug research (Yu et al., 2024). Conducting a network pharmacology analysis before conducting experiments on traditional Chinese medicine or natural drugs can more accurately determine the pathways that are involved in the mechanism of drug treatment of diseases (Xiong et al., 2024). A considerable volume of literature has confirmed the efficacy of ICA, which involves multiple pathways (Liu et al., 2021). Therefore, the pathways or genes identified in the literature should originate from genes and pathways that have been analysed in network pharmacology, so there should be an intersection of genes between the two gene sets (Wang Y. X. et al., 2022). To verify this hypothesis, the gene set obtained from network pharmacology was compared with the genes identified via experiments. The hypothesis was confirmed to be valid and it was concluded that the mechanism by which ICA acted in OC treatment involved these intersection genes and pathways.
Immunotherapy using T lymphocytes is an attractive strategy for treating various malignant tumors. However, due to the side effects and off-target effects of T cell immunotherapy, it is necessary to find a safe switch mechanism based on engineered T cells (Zhao et al., 2021). Adoptive cell therapy (ACT) using genetically engineered T cells has demonstrated high sensitivity, but some serious adverse events have been observed in several clinical studies (Jin et al., 2019). Optimal T-cell receptor (TCR) affinity in engineered T cells is crucial, and thus, the affinity of the receptor can determine the safety/efficacy of T-cell therapies (Xiang et al., 2021). Affinity is a major obstacle to the clinical success of adoptive cell therapy (ACT) due to the existence of targeted tumor extrinsic toxicity. When using antigen-specific receptors, affinity should be sufficiently high for proper T-cell activation in terms of efficacy (Yang et al., 2021). Conversely, interactions with low-affinity T-cell receptors are adequate to stimulate T cells, yet necessitate a higher affinity to sustain their proliferation. During phase I/II clinical trials of Adoptive Cell Transfer, T cells engineered with low affinity demonstrated safer profiles, albeit with diminished anti-tumor responses. Thus, the optimal affinity level is a pivotal determinant for balancing the safety and efficacy of Adoptive Cell Transfer (Wang F. et al., 2022).
Recent studies have expanded our understanding of ICA’s effects beyond ovarian cancer, highlighting its broad-spectrum anti-tumor potential: ICA has been shown to inhibit the proliferation of breast cancer cells by inducing apoptosis and cell cycle arrest. It downregulates the expression of Bcl-2 and upregulates Bax and caspase-3, promoting apoptotic pathways (Cheng et al., 2019). In non-small cell lung cancer (NSCLC), ICA suppresses tumor growth by inhibiting the PI3K/Akt/mTOR pathway. This inhibition leads to reduced cell proliferation and enhanced apoptosis, demonstrating ICA’s potential as a therapeutic agent in lung cancer treatment (Zhu and Ren, 2022). ICA has demonstrated anti-proliferative effects on prostate cancer cells by modulating the androgen receptor signaling pathway. It inhibits cell growth and induces apoptosis through the downregulation of AR and PSA expressions (Chen et al., 2023). Studies have shown that ICA can inhibit the growth and metastasis of colorectal cancer cells by modulating the Wnt/β-catenin signaling pathway. This pathway is crucial for cell proliferation and migration, and its inhibition by ICA leads to significant tumor suppression (Zhang et al., 2019). ICA has been found to induce apoptosis in leukemia cells by activating the intrinsic apoptotic pathway. It increases the expression of pro-apoptotic proteins like Bax and decreases anti-apoptotic proteins such as Bcl-2, thereby promoting cell death (Liu et al., 2023).
These studies offer a more comprehensive context for the application of ICA in ovarian cancer, illustrating its versatile anti-tumor properties across various cancer types. The mechanisms of action in these cancers frequently involve the modulation of key signaling pathways, including PI3K/Akt, Wnt/β-catenin, and intrinsic apoptotic pathways, akin to those observed in ovarian cancer.
The mechanism of action of ICA in treating ovarian cancer primarily involves multiple key signaling pathways. ICA activates the PI3K-Akt signaling pathway, which promotes cell survival and proliferation, and also enhances the sensitivity of cells to external signals, thereby inhibiting the spontaneous apoptosis of cancer cells to a certain extent. The regulation of the TNF signaling pathway by ICA is equally significant. It can increase the sensitivity of cells to apoptotic signals by affecting key proteins in this pathway, such as NF-κB. This dual regulatory effect allows ICA to demonstrate potent anti-tumor potential in the treatment of ovarian cancer. Through these mechanisms, ICA can not only inhibit the proliferation of ovarian cancer cells but also effectively promote programmed cell death, showcasing its potential as an adjunctive therapy. In contrast, the side effects of ICA are relatively mild, mainly manifested as mild gastrointestinal reactions and temporary blood pressure reduction, which makes ICA more acceptable to patients during long-term treatment. The anti-inflammatory and antioxidant properties of ICA provide additional protective effects in the treatment of ovarian cancer, helping to alleviate other complications caused by inflammation or oxidative stress. Therefore, ICA not only has potential in anti-tumor efficacy but its safety and pleiotropic effects also make it particularly important in modern ovarian cancer treatment.
The meta-analysis performed in this study aimed to ascertain the therapeutic efficacy of ICA on OC cells, revealing that treatment with ICA was significantly correlated with a reduction in cell growth [SMD = −7.80, 95% CI (−10.67, −4.92)] and apoptosis induction [SMD = 5.51, 95% CI (3.47, 7.54)]. The 14 overlapping genes identified from the literature and predicted by network pharmacology to be involved in the mechanisms through which ICA affects OC were primarily associated with the following pathways: pathways in cancer, PI3K-Akt signaling pathway, EGFR tyrosine kinase inhibitor resistance, hepatitis B, fluid shear stress and atherosclerosis, human papillomavirus infection, endocrine resistance, C-type lectin receptor signaling pathway, insulin resistance, HIF-1 signaling pathway, human T-cell leukemia virus 1 infection, TNF signaling pathway, measles, apoptosis, cellular senescence, and the sphingolipid signaling pathway. After excluding the 14 overlapping genes, the pathways corresponding to the remaining genes related to ICA’s impact on OC were also examined and encompassed: pathways in cancer, PI3K-Akt signaling pathway, endocrine resistance, hepatitis B, EGFR tyrosine kinase inhibitor resistance, melanoma, hepatocellular carcinoma, lipid and atherosclerosis, human cytomegalovirus infection, Kaposi sarcoma-associated herpesvirus infection, AGE-RAGE signaling pathway in diabetic complications, chronic myeloid leukemia, glioma, hepatitis C, cellular senescence, as well as other pathways pertinent to cell apoptosis and anti-tumor effects. This study offers a direction for future research in this area.
This study was subject to several limitations. Firstly, the meta-analysis encompassed only in vitro experiments, and due to the absence of a robust quality assessment framework for cellular studies, the conclusions drawn are inherently constrained. Secondly, the sensitivity analysis and asymmetry observed in the funnel plots indicated the presence of publication bias, which might have inflated the reported therapeutic effects of ICA. Thirdly, there is a paucity of research on the 187 intersection genes, which could introduce bias into the current findings. Incorporating additional data in the future may enhance our comprehension of this subject.
The study revealed that ICA exhibited a specific impact on anti-tumor function outcomes in comparison to placebo, characterized by enhanced cell growth reduction and the induction of apoptosis. Consequently, the pathways implicated in the therapeutic effects may be associated with cellular apoptosis and anti-tumor efficacy.
The original contributions presented in the study are included in the article/Supplementary Material, further inquiries can be directed to the corresponding author.
S-MC: Conceptualization, Formal Analysis, Funding acquisition, Project administration, Visualization, Writing–original draft. B-LC: Conceptualization, Funding acquisition, Investigation, Software, Writing–original draft. Z-ZZ: Formal Analysis, Funding acquisition, Methodology, Supervision, Writing–original draft. S-ZY: Methodology, Software, Supervision, Writing–review and editing. X-HF: Conceptualization, Project administration, Supervision, Writing–review and editing.
The author(s) declare that financial support was received for the research, authorship, and/or publication of this article. This work was supported by the Natural Science Foundation of Henan Province (222300420247), Postdoctoral Foundation of Henan Province (HN2024098), Central Government Guides Local Projects (Z20221343023), Medical science and technology research plan of Henan Province (LHGJ20221031) and the Scientific Research Project of Luohe Medical College in 2019 (2023ZD18).
The study thanks Professor En-kui Duan, President of the Chinese Academy of Sciences, Beijing, China, for providing technical guidance for this study. Thanks Rui Wang, Yun-Zhi Wang, Zuo-Min Wu, pharmacists of Pharmacy Department, Luohe Central Hospital, Henan Province Key Laboratory of Traditional Chinese Medicine Formulation and Processing, Henan Province Engineering Research Center for Modernization of Traditional Chinese Medicine Formulation and Clinical Application, for providing assistance for the research. And also thanks Yuan Zhuang, Yan-Qi He, doctors of Luohe Central Hospital, for providing assistance for the research.
The authors declare that the research was conducted in the absence of any commercial or financial relationships that could be construed as a potential conflict of interest.
All claims expressed in this article are solely those of the authors and do not necessarily represent those of their affiliated organizations, or those of the publisher, the editors and the reviewers. Any product that may be evaluated in this article, or claim that may be made by its manufacturer, is not guaranteed or endorsed by the publisher.
The Supplementary Material for this article can be found online at: https://www.frontiersin.org/articles/10.3389/fphar.2024.1418111/full#supplementary-material
Cao, S., Fu, X., Yang, S., and Tang, S. (2022). The anti-inflammatory activity of resveratrol in acute kidney injury: a systematic review and meta-analysis of animal studies. Pharm. Biol. 60 (1), 2088–2097. doi:10.1080/13880209.2022.2132264
Chan, D. W., Yung, M. M., Chan, Y. S., Xuan, Y., Yang, H., Xu, D., et al. (2020). MAP30 protein from Momordica charantia is therapeutic and has synergic activity with cisplatin against ovarian cancer in vivo by altering metabolism and inducing ferroptosis. Pharmacol. Res. 161 (1), 105157. doi:10.1016/j.phrs.2020.105157
Chelliah, V., and van der Graaf, P. H. (2022). Model-informed target identification and validation through combining quantitative systems pharmacology with network-based analysis. CPT Pharmacometrics Syst. Pharmacol. 11 (4), 399–402. doi:10.1002/psp4.12766
Chen, C., Wang, S., Wang, N., Zheng, Y., Zhou, J., Hong, M., et al. (2023). Icariin inhibits prostate cancer bone metastasis and destruction via suppressing TAM/CCL5-mediated osteoclastogenesis. Phytomedicine 120 (1), 155076. doi:10.1016/j.phymed.2023.155076
Chen, H., Song, L., Xu, X., Han, Z., Peng, F., Zhang, Q., et al. (2022). The effect of icariin on autoimmune premature ovarian insufficiency via modulation of Nrf2/HO-1/Sirt1 pathway in mice. Reprod. Biol. 22 (2), 100638. doi:10.1016/j.repbio.2022.100638
Cheng, X., Tan, S., Duan, F., Yuan, Q., Li, Q., and Deng, G. (2019). Icariin induces apoptosis by suppressing autophagy in tamoxifen-resistant breast cancer cell line MCF-7/TAM. Breast Cancer 26 (6), 766–775. doi:10.1007/s12282-019-00980-5
Dou, L., Zou, D., Song, F., Jin, Y., Li, Y., and Zhang, Y. (2021). Bufalin suppresses ovarian cancer cell proliferation via EGFR pathway. Chin. Med. J. Engl. 135 (4), 456–461. doi:10.1097/CM9.0000000000001879
Gao, Q., Zhu, J., Zhao, W., Huang, Y., An, R., Zheng, H., et al. (2022). Olaparib maintenance monotherapy in asian patients with platinum-sensitive relapsed ovarian cancer: phase III trial (L-MOCA). Clin. Cancer Res. 28 (11), 2278–2285. doi:10.1158/1078-0432.CCR-21-3023
Jiao, F., Tang, W., Huang, H., Zhang, Z., Liu, D., Zhang, H., et al. (2018). Icariin promotes the migration of BMSCs in vitro and in vivo via the MAPK signaling pathway. Stem Cells Int. 2018 (2), 2562105. doi:10.1155/2018/2562105
Jin, J., Wu, X., Yin, J., Li, M., Shen, J., Li, J., et al. (2019). Identification of genetic mutations in cancer: challenge and opportunity in the new era of targeted therapy. Front. Oncol. 9, 263. doi:10.3389/fonc.2019.00263
Lau, T. S., Chan, L. K. Y., Man, G. C. W., Wong, C. H., Lee, J. H. S., Yim, S. F., et al. (2020). Paclitaxel induces immunogenic cell death in ovarian cancer via TLR4/IKK2/SNARE-dependent exocytosis. Cancer Immunol. Res. 8 (8), 1099–1111. doi:10.1158/2326-6066.Cir-19-0616
Li, H., Qi, Z., Niu, Y., Yang, Y., Li, M., Pang, Y., et al. (2021). FBP1 regulates proliferation, metastasis, and chemoresistance by participating in C-MYC/STAT3 signaling axis in ovarian cancer. Oncogene 40 (40), 5938–5949. doi:10.1038/s41388-021-01957-5
Li, Q., Zhang, H., Yan, X., Zhao, Z., Qiu, J., Hu, L., et al. (2023). Up-regulation of PPAR-γ involved in the therapeutic effect of icariin on cigarette smoke-induced inflammation. Pulm. Pharmacol. Ther. 79 (10), 102197. doi:10.1098/rsos.181877
Lifeng, W., Guanfa, P., Li, C., Mingling, G., Bingyu, W., Yunmeng, Z., et al. (2023). Icariin reduces cognitive dysfunction induced by surgical trauma in aged rats by inhibiting hippocampal neuroinflammation. Front. Behav. Neurosci. 17 (5), 1162009. doi:10.3389/fnbeh.2023.1162009
Liu, F. Y., Ding, D. N., Wang, Y. R., Liu, S. X., Peng, C., Shen, F., et al. (2023). Icariin as a potential anticancer agent: a review of its biological effects on various cancers. Front. Pharmacol. 14, 1216363. doi:10.3389/fphar.2023.1216363
Liu, X., Liu, Z., Miao, Y., Wang, L., and Yin, H. (2021). Sex hormone-like Effects of Icariin on T-cells immune modulation in spontaneously hypertensive rats. J. Ethnopharmacol. 269 (12), 113717. doi:10.1016/j.jep.2020.113717
Liu, Y., and Fang, Y. (2022). Three-dimensional mesenchymal stem cell laden scaffold of icariin sustained-release for bone regeneration. Turk J. Biol. 46 (5), 414–425. doi:10.55730/1300-0152.2627
Liu, Y., Liu, X., Wang, H., Ding, P., and Wang, C. (2022). Agrimonolide inhibits cancer progression and induces ferroptosis and apoptosis by targeting SCD1 in ovarian cancer cells. Phytomedicine 101 (1), 154102. doi:10.1016/j.phymed.2022.154102
Lu, Z., Gao, F., Teng, F., Tian, X., Guan, H., Li, J., et al. (2023). Exploring the pathogenesis of depression and potential antidepressants through the integration of reverse network pharmacology, molecular docking, and molecular dynamics. Med. Baltim. 102 (44), e35793. doi:10.1097/MD.0000000000035793
Morand, S., Devanaboyina, M., Staats, H., Stanbery, L., and Nemunaitis, J. (2021). Ovarian cancer immunotherapy and personalized medicine. Int. J. Mol. Sci. 22 (12), 6532. doi:10.3390/ijms22126532
Moubarak, M., Harter, P., Ataseven, B., Traut, A., Welz, J., Baert, T., et al. (2022). Re-treatment with PARPi in patients with recurrent epithelial ovarian cancer: a single institutional experience. Gynecol. Oncol. Rep. 40, 100939. doi:10.1016/j.gore.2022.100939
Munoz-Zuluaga, C. A., Sardi, A., Sittig, M., Gushchin, V., King, M. C., Nieroda, C., et al. (2020). Critical analysis of stage IV epithelial ovarian cancer patients after treatment with neoadjuvant chemotherapy followed by cytoreductive surgery and hyperthermic intraperitoneal chemotherapy (CRS/HIPEC). Int. J. Surg. Oncol. 2020, 1467403. doi:10.1155/2020/1467403
Niu, Y., Lin, G., Pan, J., Liu, J., Xu, Y., Cai, Q., et al. (2022). Deciphering the myth of icariin and synthetic derivatives in improving erectile function from a molecular biology perspective: a narrative review. Transl. Androl. Urol. 11 (7), 1007–1022. doi:10.21037/tau-22-232
Park, C. O., Lee, S. E., Yoon, J. W., Park, H. J., Li, Z., Oh, S. H., et al. (2022). Comparison of three antioxidants in chemical and biological assays on porcine oocytes during ageing in vitro. Zygote 30 (4), 561–570. doi:10.1017/s0967199421000459
Su, B. L., Wang, L. L., Zhang, L. Y., Zhang, S., Li, Q., and Chen, G. Y. (2023). Potential role of microRNA-503 in Icariin-mediated prevention of high glucose-induced endoplasmic reticulum stress. World J. Diabetes 14 (8), 1234–1248. doi:10.4239/wjd.v14.i8.1234
Sun, S., and Fang, H. (2021). Curcumin inhibits ovarian cancer progression by regulating circ-PLEKHM3/miR-320a/SMG1 axis. J. Ovarian Res. 14 (1), 158. doi:10.1186/s13048-021-00916-8
Szabó, R., Rácz, C. P., and Dulf, F. V. (2022). Bioavailability improvement strategies for icariin and its derivates: a review. Int. J. Mol. Sci. 23 (14), 7519. doi:10.3390/ijms23147519
Tao, Z., Liu, J., Jiang, Y., Gong, L., and Yang, B. (2017). Synthesis of prenylated flavonols and their potents as estrogen receptor modulator. Sci. Rep. 7 (1), 12445. doi:10.1038/s41598-017-12640-9
Wang, F., Zheng, A., Zhang, D., Zou, T., Xiao, M., Chen, J., et al. (2022). Molecular profiling of core immune-escape genes highlights LCK as an immune-related prognostic biomarker in melanoma. Front. Immunol. 13, 1024931. doi:10.3389/fimmu.2022.1024931
Wang, P., Zhang, J., Xiong, X., Yuan, W., Qin, S., Cao, W., et al. (2019). Icariin suppresses cell cycle transition and cell migration in ovarian cancer cells. Oncol. Rep. 41 (4), 2321–2328. doi:10.3892/or.2019.6986
Wang, X., Liu, C., Xu, Y., Chen, P., Shen, Y., Xu, Y., et al. (2017). Combination of mesenchymal stem cell injection with icariin for the treatment of diabetes-associated erectile dysfunction. PloS one 12 (3), e0174145. doi:10.1371/journal.pone.0174145
Wang, X., Su, P., Hao, Q., Zhang, X., Xia, L., and Zhang, Y. (2022). A Chinese classical prescription Guizhi-Fuling Wan in treatment of ovarian cancer: an overview. Biomed. Pharmacother. 153 (11), 113401. doi:10.1016/j.biopha.2022.113401
Wang, Y. X., Yang, Z., Wang, W. X., Huang, Y. X., Zhang, Q., Li, J. J., et al. (2022). Methodology of network pharmacology for research on Chinese herbal medicine against COVID-19: a review. J. Integr. Med. 20 (6), 477–487. doi:10.1016/j.joim.2022.09.004
Xiang, S., Li, J., Shen, J., Zhao, Y., Wu, X., Li, M., et al. (2021). Identification of prognostic genes in the tumor microenvironment of hepatocellular carcinoma. Front. Immunol. 12, 653836. doi:10.3389/fimmu.2021.653836
Xie, R. F., Liu, S., Yang, M., Xu, J. Q., Li, Z. C., and Zhou, X. (2019). Effects and possible mechanism of Ruyiping formula application to breast cancer based on network prediction. Sci. Rep. 9 (1), 5249. doi:10.1038/s41598-019-41243-9
Xie, Y. F., Wang, M. G., Chen, K. M., Shi, W. G., Zhou, J., and Gao, Y. H. (2015). Icariin enhances differentiation and maturation of rat calvarial osteoblasts in collagen hydrogel three-dimensional culture. Zhejiang Da Xue Xue Bao Yi Xue Ban. 44 (3), 301–307. doi:10.3785/j.issn.1008-9292.2015.05.10
Xiong, M., Chen, X., Wang, H., Tang, X., Wang, Q., Li, X., et al. (2024). Combining transcriptomics and network pharmacology to reveal the mechanism of Zuojin capsule improving spasmolytic polypeptide-expressing metaplasia. J. Ethnopharmacol. 318 (Pt B), 117075. doi:10.1016/j.jep.2023.117075
Yang, Q., Chen, Y., Guo, R., Dai, Y., Tang, L., Zhao, Y., et al. (2021). Interaction of ncRNA and epigenetic modifications in gastric cancer: focus on histone modification. Front. Oncol. 11, 822745. doi:10.3389/fonc.2021.822745
Ye, R., Xu, S., Liu, Y., Pang, L., Lian, X., Zhong, Y., et al. (2017). Protective effect of icariin on the development of preimplantation mouse embryos against hydrogen peroxide-induced oxidative injury. Oxid. Med. Cell Longev. 2017 (3), 2704532. doi:10.1155/2017/2704532
Yu, L., Sun, J., Wang, Q., Yu, W., Wang, A., Zhu, S., et al. (2023). Ovulation induction drug and ovarian cancer: an updated systematic review and meta-analysis. J. Ovarian Res. 16 (1), 22. doi:10.1186/s13048-022-01084-z
Yu, X., Qin, W., Cai, H., Ren, C., Huang, S., Lin, X., et al. (2024). Analyzing the molecular mechanism of xuefuzhuyu decoction in the treatment of pulmonary hypertension with network pharmacology and bioinformatics and verifying molecular docking. Comput. Biol. Med. 169 (2), 107863. doi:10.1016/j.compbiomed.2023.107863
Yue, K., Liu, Z., Pi, Z., Li, H., Wang, Y., Song, F., et al. (2022). Network pharmacology combined with metabolomics approach to investigate the toxicity mechanism of paclobutrazol. Chem. Res. Toxicol. 35 (4), 626–635. doi:10.1021/acs.chemrestox.1c00404
Zeng, B., Qi, L., Wu, S., Liu, N., Wang, J., Nie, K., et al. (2023). Network pharmacology prediction and metabolomics validation of the mechanism of fructus phyllanthi against hyperlipidemia. J. Vis. Exp. 13 (194). doi:10.1007/s40199-023-00466-y
Zhang, D. Y., Peng, R. Q., Wang, X., Zuo, H. L., Lyu, L. Y., Yang, F. Q., et al. (2022). A network pharmacology-based study on the quality control markers of antithrombotic herbs: using Salvia miltiorrhiza - ligusticum chuanxiong as an example. J. Ethnopharmacol. 292 (1), 115197. doi:10.1016/j.jep.2022.115197
Zhang, H. F., Yang, T. S., Li, Z. Z., Wang, Y., Zhang, H., Zhuo, S., et al. (2008). Simultaneous extraction of epimedin A, B, C and icariin from Herba Epimedii by ultrasonic technique. Ultrason. Sonochem 15 (4), 376–385. doi:10.1016/j.ultsonch.2007.09.002
Zhang, N., Zhang, D., Zhang, Q., Zhang, R., and Wang, Y. (2023). Mechanism of Danggui Sini underlying the treatment of peripheral nerve injury based on network pharmacology and molecular docking: a review. Med. Baltim. 102 (19), e33528. doi:10.1097/MD.0000000000033528
Zhang, Q., Liang, J., and Zhou, Y. (2022). Network pharmacology analysis of molecular targets and related mechanisms of Guizhi decoction in treating of menopausal syndrome. Med. Baltim. 101 (29), e29453. doi:10.1097/md.0000000000029453
Zhang, W., Zhang, Z., and Qian, L. (2024). Prognostic and clinicopathological significance of C-reactive protein in patients with ovarian cancer: a meta-analysis. World J. Surg. Oncol. 22 (1), 8. doi:10.1186/s12957-023-03290-5
Zhang, Y., Cao, S., Zhuang, C., Chen, J., Chen, X., Sun, H., et al. (2021). ERCC1 rs11615 polymorphism and chemosensitivity to platinum drugs in patients with ovarian cancer: a systematic review and meta-analysis. J. Ovarian Res. 14 (1), 80. doi:10.1186/s13048-021-00831-y
Zhang, Y., Li, Z., Wei, J., Kong, L., Song, M., Zhang, Y., et al. (2022). Network pharmacology and molecular docking reveal the mechanism of Angelica dahurica against Osteosarcoma. Med. Baltim. 101 (44), e31055. doi:10.1097/MD.0000000000031055
Zhang, Y., Wei, Y., Zhu, Z., Gong, W., Liu, X., Hou, Q., et al. (2019). Icariin enhances radiosensitivity of colorectal cancer cells by suppressing NF-κB activity. Cell Biochem. Biophys. 69 (2), 303–310. doi:10.1007/s12013-013-9799-x
Zhang, Y. F., Fan, Y., Mu, Y., Zhang, P., Wang, M. Y., and Li, J. K. (2022). Reproductive and oncological outcomes of fertility-sparing surgery in patients with stage I epithelial ovarian cancer: a systematic review and meta-analysis. Med. Baltim. 101 (31), e29929. doi:10.1097/md.0000000000029929
Zhao, C. F., Li, Z. H., Li, S. J., Li, J. A., Hou, T. T., and Wang, Y. (2019). PLGA scaffold carrying icariin to inhibit the progression of osteoarthritis in rabbits. R. Soc. Open Sci. 6 (4), 181877. doi:10.1016/j.redox.2022.102297
Zhao, H., Wang, S., Williamson, P. T. F., Ewing, R. M., Tang, X., Wang, J., et al. (2023). Integrated network pharmacology and cellular assay reveal the biological mechanisms of Limonium sinense (Girard) Kuntze against Breast cancer. BMC Complement. Med. Ther. 23 (1), 408. doi:10.1186/s12906-023-04233-z
Zhao, M., Fu, L., Xu, P., Wang, T., and Li, P. (2023). Network pharmacology and experimental validation to explore the effect and mechanism of kanglaite injection against triple-negative breast cancer. Drug Des. Devel Ther. 17 (3), 901–917. doi:10.2147/DDDT.S397969
Zhao, Q., Jiang, Y., Xiang, S., Kaboli, P. J., Shen, J., Zhao, Y., et al. (2021). Engineered TCR-T cell immunotherapy in anticancer precision medicine: pros and cons. Front. Immunol. 12, 658753. doi:10.3389/fimmu.2021.658753
Zhao, Y., Li, H., Li, X., Sun, Y., Shao, Y., Zhang, Y., et al. (2022). Network pharmacology-based analysis and experimental in vitro validation on the mechanism of Paeonia lactiflora Pall. in the treatment for type I allergy. BMC Complement. Med. Ther. 22 (1), 199. doi:10.1186/s12906-022-03677-z
Zheng, Q., Ying, Q., Ren, Z., Zhang, Q., Lu, D., Wang, H., et al. (2021). First-degree family history of prostate cancer is associated the risk of breast cancer and ovarian cancer. Med. Baltim. 100 (4), e23816. doi:10.1097/MD.0000000000023816
Zhong, Y., Chen, R. F., and Fang, Y. F. (2022). Network pharmacology-based strategy to reveal the mechanism of cassiae semen against cataracts. Comput. Math. Methods Med. 2022 (6), 5654120. doi:10.1155/2022/5654120
Zhou, W., Zhang, H., Wang, X., Kang, J., Guo, W., Zhou, L., et al. (2022). Network pharmacology to unveil the mechanism of Moluodan in the treatment of chronic atrophic gastritis. Phytomedicine 95 (1), 153837. doi:10.1016/j.phymed.2021.153837
Keywords: anti-tumour, ovarian cancer, network pharmacology, meta-analysis, icariin
Citation: Cao S-M, Chen B-L, Zou Z-Z, Yang S-Z and Fu X-H (2024) Effect of icariin on ovarian cancer: a combined network pharmacology and meta-analysis of in vitro studies approach. Front. Pharmacol. 15:1418111. doi: 10.3389/fphar.2024.1418111
Received: 25 August 2024; Accepted: 19 November 2024;
Published: 20 December 2024.
Edited by:
Syed Shams ul Hassan, Shanghai Jiao Tong University, ChinaReviewed by:
Tao Yi, Hong Kong Baptist University, Hong Kong SAR, ChinaCopyright © 2024 Cao, Chen, Zou, Yang and Fu. This is an open-access article distributed under the terms of the Creative Commons Attribution License (CC BY). The use, distribution or reproduction in other forums is permitted, provided the original author(s) and the copyright owner(s) are credited and that the original publication in this journal is cited, in accordance with accepted academic practice. No use, distribution or reproduction is permitted which does not comply with these terms.
*Correspondence: Xiu-Hong Fu, ZnhoZm9yZXZAMTYzLmNvbQ==
Disclaimer: All claims expressed in this article are solely those of the authors and do not necessarily represent those of their affiliated organizations, or those of the publisher, the editors and the reviewers. Any product that may be evaluated in this article or claim that may be made by its manufacturer is not guaranteed or endorsed by the publisher.
Research integrity at Frontiers
Learn more about the work of our research integrity team to safeguard the quality of each article we publish.