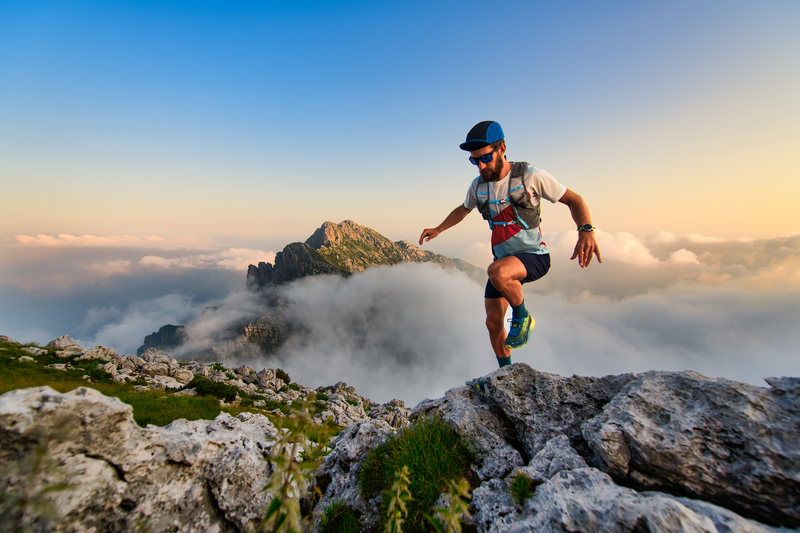
95% of researchers rate our articles as excellent or good
Learn more about the work of our research integrity team to safeguard the quality of each article we publish.
Find out more
ORIGINAL RESEARCH article
Front. Pharmacol. , 25 October 2024
Sec. Ethnopharmacology
Volume 15 - 2024 | https://doi.org/10.3389/fphar.2024.1412593
This article is part of the Research Topic Traditional Clinical Symptoms and Signs: How Can They Be Used to Investigate Medications in the Context of Pharmacology? View all 12 articles
Objective: The purpose of this study was to predict the four cold–heat patterns in patients who have the subjective symptoms of the cold–heat pattern described in the International Classification of Diseases Traditional Medicine Conditions – Module 1 by applying a machine learning algorithm.
Methods: Subjects were first-visit Kampo outpatients at six institutions who agreed to participate in this multicenter prospective observational study. The cold pattern model and the heat pattern model were created separately with 148 symptoms, body mass index, blood pressure (systolic and diastolic), age, and sex. Along with a single cold or heat pattern, the tangled heat/cold pattern is defined as being predicted by both cold and heat patterns, while the moderate (heat/cold) pattern is defined as being predicted by neither the cold pattern nor the heat pattern.
Results: We included 622 participants (mean age ±standard deviation, 54.4 ± 16.9; with female 501). The accuracy, macro-recall, precision, and F1-score of a combination of the two prediction models were 96.7%, 93.2%, 85.6%, and 88.5% respectively. The important items were compatible with the definitions of the cold–heat pattern.
Conclusion: We developed a prediction model on cold–heat patterns with data from patients whose subjective cold/heat-related symptoms matched the cold–heat pattern diagnosis by the physician.
The 11th revision of the International Classification of Diseases (ICD-11), effective January 2022 (World Health Organization, 2023), includes for the first time a chapter on traditional medicine conditions— Module 1 (ICD-11TM1)—including traditional East Asian medicine practiced mainly in China, Korea, and Japan. Kampo diagnosis is based on a synthesis of findings obtained through inspection, listening and smell, inquiring, and palpation, and relies heavily on the physician’s five senses, experience, and tacit knowledge. The selection of an appropriate Kampo medicine is based on traditional Kampo diagnosis. Even for a single conventional biomedicine diagnosis, such as perimenopausal symptoms or atopic dermatitis, Kampo specialists use various treatment options based on pattern diagnosis such as a heat–cold pattern. With the inclusion of Kampo diagnosis in ICD-11, it is necessary to visualize and quantify subjective information on the Kampo specialist involved in the diagnosis, which has rarely been done, as reproducible information.
In Japan, a single medical license allows physicians to prescribe both conventional biomedicines and Kampo medicines, and over 90% of physicians have experience prescribing Kampo medicines (Moschik et al., 2012). However, most physicians are not specialists in Kampo medicine and lack detailed knowledge of it. Therefore, it is crucial for non-specialists in Kampo medicine to easily grasp the patient’s traditional pattern diagnosis when choosing an appropriate Kampo medicine.
We have developed a model to predict a deficiency–excess and cold–heat pattern from patient questionnaires and have identified important items to predict the deficiency–excess and cold–heat pattern (Katayama et al., 2014; Maeda-Minami et al., 2019; Maeda-Minami et al., 2020). We have also identified the important items for the predicting deficiency–excess and cold–heat pattern (Katayama et al., 2014; Maeda-Minami et al., 2019; Maeda-Minami et al., 2020). In addition to our previous study, there have been previous reports on prediction models for the cold–heat pattern (Lee B. J. et al., 2018; Lee J. et al., 2018).
In Japan, there is a moderate (heat/cold) pattern in addition to the cold pattern, heat pattern, and tangled heat/cold pattern in the cold–heat pattern (Yakubo et al., 2014; Maeda-Minami et al., 2021). A moderate (heat/cold) pattern is defined in ICD-11TM1 as “a pattern characterized by the absence of findings that indicate the heat pattern (TM), such as heat intolerance, red complexion, and hot limbs, or cold pattern (TM), such as cold intolerance, pale complexion, and cold limbs. It may be explained by the average level of metabolic activity” (World Health Organization, 2023). However, the cold–heat pattern prediction models in previous studies have been able to predict only the cold and heat patterns among the four cold–heat patterns (Katayama et al., 2014; Maeda-Minami et al., 2020; Lee BJ et al., 2018; Lee J et al., 2018). No model predicts the tangled heat/cold pattern and moderate (heat/cold) pattern as well.
In addition, in constructing the cold–heat pattern prediction model, we investigated our data carefully and found that the ICD-11TM1 definition of the cold–heat pattern includes many complaints about patients’ cold–heat pattern, but the patients’ cold–heat pattern complaints did not match the physician’s diagnosis of the cold–heat pattern. The physician’s diagnosis of the cold–heat pattern and the patient’s symptoms of the cold–heat pattern were consistent in only 33.6% of all patients. We have also reported that using training data with smaller deviations from the definition leads to models that are more realistic and have a higher accuracy rather than using pragmatic data that include a wider variety of patients (Maeda-Minami et al., 2022).
The purpose of this study was to predict the four cold–heat patterns in patients who have the subjective symptoms of the cold–heat pattern described in ICD-11TM1 by applying a machine learning algorithm.
This is a multicenter prospective observational study.
Subjects were first-visit Kampo outpatients at six institutions, Chiba University, Iizuka Hospital, Keio University, University of Toyama, Kameda General Hospital, and Jichi Medical University, who agreed to participate in the study. The data collection periods for each institution were as follows: Chiba University from March 2012 to January 2015, Iizuka Hospital from January 2012 to February 2015, Keio University from June 2012 to March 2013, University of Toyama from June 2012 to February 2015, Kameda General Hospital from January 2012 to March 2013, and Jichi Medical University was from January 2012 to March 2012. From previous studies, we found no remarkable differences among the six institutions in terms of participants’ demographic characteristics, including mean age, female-to-male ratio, mean body mass index (BMI), mean blood pressure, physician-diagnosed patterns, and important items in the prediction models in previous studies using the same dataset. Therefore, data from all institutions were combined for this present analysis (Maeda-Minami et al., 2019; Maeda-Minami et al., 2020).
Exclusion criteria were various data deficits (blood pressure, height, weight, etc.), age less than 20 years, fewer than 20 questionnaire items answered, and discrepancy in the cold–heat pattern between the physician’s diagnosis and the patient’s symptom. We have already reported that it was difficult to predict the pattern from the questionnaire items alone in patients with fewer than 20 items (Katayama et al., 2014; Maeda-Minami et al., 2019; Maeda-Minami et al., 2020).
The patients were selected based on their responses to the cold–heat pattern questionnaire, referring to the definition in ICD-11TM1 (World Health Organization, 2023). A part of the questionnaire was administered to patients using the visual analog scale (VAS, 0–100 mm). A VAS value of 0 was considered to indicate that the patient responded that he/she did not have the symptom, and a VAS value greater than 0 was considered to indicate that the patient responded that he/she had the symptom. The cold–heat pattern was determined from the patient’s symptoms, according to the classification in Table 1.
Only those patients were selected for whom the pattern determined from the patient’s symptoms regarding ICD-11TM1 matched the pattern diagnosed by the physician. We used the traditional pattern diagnosis diagnosed by specialists in Kampo medicine as the ground truth. The specialists in Kampo medicine employed in this study had held active biomedical licenses, completed residencies such as internal medicine or obstetrics and gynecology, and were board-certified by the Japan Society for Oriental Medicine with 3 or more years of clinical fellowship training. We ensured consistency by also restricting patients whose responses to interview items met the ICD-11TM1 definition.
A total of 154 medical information items were obtained from patients, 148 interview items (including 29 binary items and 119 VAS items), vital data [height, weight, and blood pressure (systolic and diastolic)], and attribute data (age and sex). Since BMI was the most important item in the prediction model for the deficiency–excess pattern in previous studies (Katayama et al., 2014; Maeda-Minami et al., 2019; Maeda-Minami et al., 2020), height(m) and weight (kg) were converted to BMI instead of using them as they were when creating the prediction model. Therefore, the independent variables used in the prediction models were 153 items.
For the construction of the cold pattern prediction model and the heat pattern prediction model, the algorithm used a random forest classification derived by Breiman (1996), Breiman (2001), and Breiman et al. (2008). Random forest generates multiple sample datasets from the training data using the bootstrap method and constructs decision trees for each dataset (Breiman, 2001). Each decision tree is independently trained, using a random subset of features to determine the branching points (Breiman, 2001). This ensemble learning method combines multiple decision trees for prediction (Breiman, 2001). The classification algorithm used in this study makes predictions on unknown data by taking the majority vote of each decision tree’s classification result (Breiman, 2001). Results are expressed as vote values ranging from 0 to 1. We have previously reported that random forests had the best accuracy for predicting deficiency–excess and cold–heat patterns (Katayama et al., 2014; Maeda-Minami et al., 2019; Maeda-Minami et al., 2020). The parameters for random forest include the number of decision trees, the number of features used for each tree, and the number of samples in the end nodes, which were set to 500, 12, and 1, respectively.
By creating a random forest model, we can determine the importance of each item in the prediction, which is calculated based on the decrease in the Gini coefficient when the variable is excluded from the model (mean decrease Gini). Higher values indicate that the item significantly contributes to the prediction.
The explanatory variables for the prediction models were 148 symptoms (including 29 binary items and 119 VAS items), BMI, blood pressure (systolic and diastolic), age, and sex, for a total of 153 items. VAS values were normalized by dividing each VAS value by the maximum VAS value for each patient, as in previous studies (Katayama et al., 2011; Katayama et al., 2014; Maeda-Minami et al., 2019; Maeda-Minami et al., 2020).
We have already reported the confounding relationship between the deficiency–excess and cold–heat patterns (Maeda-Minami et al., 2020). In our data, there were approximately nine times as many patients with deficiency and cold patterns as patients with deficiency and heat patterns and approximately twice as many patients with excess and heat patterns as patients with excess and cold patterns. Our data had both high-frequency and low-frequency classes, which influenced this relationship. When creating prediction models using imbalanced data, it is generally suggested to sample the training data according to either the high-frequency or low-frequency class (Fujiwara et al., 2020; He and Garcia, 2009). Our previous study found that random undersampling to match the low-frequency class, rather than extracting based on the overall data ratio, led to better prediction models with key items closely aligning with the ICD-11TM1 definition (Maeda-Minami et al., 2020).
Following the method of previous research, the training data were randomly sampled from the following 12 pattern patients with a balance of 10 cases each; deficiency-cold pattern, deficiency-moderate (heat/cold) pattern, deficiency-heat pattern, deficiency-tangled heat/cold pattern, medium-cold pattern, medium-moderate (heat/cold) pattern, medium-heat pattern, medium-tangled heat/cold pattern, excess-cold pattern, excess-moderate (heat/cold) pattern, excess-heat pattern, and excess-tangled heat/cold pattern (Table 2). The number of patients in the excess-moderate (heat/cold) pattern was less than 10, so bootstrap resampling was performed. We sampled a total of 120 cases from 12 patterns and divided them into 60 cases each to train classification models for the cold pattern or not and heat pattern or not. Based on our previous results, this was considered sufficient for training data. The remaining data other than the training data were used as test data.
The cold pattern and heat pattern models were created separately for this study to predict the four cold–heat patterns (Figure 1). In ICD-11, the tangled heat/cold pattern is described as having both cold and heat patterns, while the moderate (heat/cold) pattern is described as having neither a cold pattern nor a heat pattern (World Health Organization, 2023). The cold pattern prediction model and the heat pattern prediction model were constructed by the random forest classification algorithm using the training data.
At the stage of creating the cold pattern prediction model, data diagnosed by Kampo specialists as the cold pattern or tangled heat/cold pattern were used as 1/true, while data diagnosed as the heat pattern or moderate (heat/cold) pattern were used as 0/false for training. Similarly, data diagnosed by Kampo specialists as the heat pattern or tangled heat/cold pattern were used as 1/true, while data diagnosed as the cold pattern or moderate (heat/cold) pattern were used as 0/false for the heat pattern prediction model.
Both prediction models are used to calculate a vote value in the range of 0–1 for each test data (Figure 1). In the cold pattern prediction model, the calculated vote value of 0.5 or more was judged as a cold pattern or tangled heat/cold pattern and that of less than 0.5 as a heat pattern or moderate (heat/cold) pattern. In the heat pattern prediction model, a calculated vote value of 0.5 or higher was considered a heat pattern or tangled heat/cold pattern, and a calculated vote value of less than 0.5 was considered a cold pattern or moderate (heat/cold) pattern.
Based on the results of the cold pattern prediction model and the heat pattern prediction model, we determined the four cold–heat patterns as the cold pattern, moderate (heat/cold) pattern, heat pattern, and tangled heat/cold pattern for each of the test data. We calculated the accuracy (percent agreement) between the ground truth and the patterns predicted by the prediction models. We also calculated specific metrics used in multi-class classification, macro-recall, precision, and F1-score.
The 30 most important items for the cold pattern prediction model and the heat pattern prediction model were selected. Moreover, before finalizing the random forest, we repeated random samplings to create the models multiple times and confirmed that it generally resulted in similar accuracy and important explanatory items each time.
All analyses were performed using R4.2.2 (R Foundation for Statistical Computing, Vienna, Austria). R’s random forests package was used (Breiman et al., 2008).
The number of patients registered at the six institutions was 925 at Chiba University, 791 at Iizuka Hospital, 781 at Keio University, 501 at University of Toyama, 424 at Kameda General Hospital, and 59 at Jichi Medical University (Figure 2). The number of eligible patients was 133 at Chiba University, 151 at Iizuka Hospital, 224 at Keio University, 65 at University of Toyama, 46 at Kameda General Hospital, and 3 at Jichi Medical University. The patient backgrounds used in the prediction model are shown in Table 3. The cross table on the deficiency–excess pattern and cold–heat pattern diagnosed by the physicians is shown in Table 2.
The accuracy of the cold pattern prediction model was 96.7%, and the accuracy of the heat pattern prediction model was 96.7%. The accuracy, macro-recall, precision, and F1-score by the combination of the two prediction models were 96.7%, 93.2%, 85.6%, and 88.5%, respectively (Table 4).
The important items in the cold pattern prediction model include cold hypersensitivity in legs, cold intolerance, cold hypersensitivity in hands, general cold hypersensitivity, and blood pressure. The important items in the heat pattern prediction model include heat hypersensitivity in face, easy to sweat, heat intolerance, night sweats, and hot flashes. These items are included in the definitions of the cold–heat pattern in the ICD-11TM1 (Table 5).
We developed prediction models on four cold–heat patterns including the tangled heat/cold and moderate (heat/cold) patterns at six institutions specializing in Kampo in Japan. First, we constructed a cold pattern prediction model and a heat pattern prediction model and then combined them to predict the four cold–heat patterns. Even though the model was validated by the selected patients’ data, the accuracy was over 90%, showing high concordance with the physician’s diagnosis. The high accuracy suggests that the combination of two prediction models is likely to be a successful method for predicting the cold–heat pattern.
In the present study, we combined the two prediction models to predict a physician’s cold–heat pattern diagnosis, meaning both models have to predict the diagnosis correctly to reach a correct conclusion. There was no case of both models predicting the wrong diagnosis (i.e., predicted as a moderate (heat/cold) pattern for cases with a tangled heat/cold pattern by specialist physicians). Most of the misclassified cases occurred because one of the prediction models failed to identify a cold or heat pattern in patients diagnosed by physicians as having such patterns. In only two cases, one of the two prediction models incorrectly identified a cold or heat pattern in patients who were diagnosed by physicians as having no such pattern. In both cases, our model concluded a tangled heat/cold pattern, while the specialists diagnosed them as cold or heat.
The prediction model was constructed based on the results of the patient questionnaires, but we also found that the accuracy of the prediction model for the deficiency–excess pattern increased when the results of abdominal strength diagnosed by the physician were added as an explanatory variable (Maeda-Minami et al., 2019; Maeda-Minami et al., 2020). By adding the results of the physician’s examination, for example, complexion and limb temperature, according to the ICD-11TM1 definition of cold/heat patterns, as an explanatory variable may enable the construction of a model with high accuracy even when using patients whose patterns did not match the patterns diagnosed by the physician based on the patient’s symptoms. However, since one of the purposes of our study is to create a diagnostic aid tool that will allow physicians who do not specialize in Kampo to easily make an appropriate diagnosis and select a Kampo medicine, we did not incorporate the results of the physician’s examination into our study.
This study has several limitations. The prediction models were constructed excluding patients whose patterns diagnosed by the physician did not agree with subjective symptoms, and the accuracy was approximately 10% to these patients (data not shown). Further research is needed to use predictive models in daily clinical practice. To improve the predictive model that can also be applied to the patients excluded in this study, we plan to apply the current model in real clinical settings, examine which types of patients it misclassifies, and incorporate explanatory variables that can be measured even by non-specialists in Kampo medicine. These results cannot be applied to those under 20 years of age because those under 20 years of age were excluded from this study. Similarly, patients with 19 or fewer symptoms are excluded from the present study due to the difficulty of predicting their pattern diagnosis with our data according to our prior studies for the deficiency–excess pattern. In our previous study, the most important items that contributed to the prediction of the cold–heat pattern in the prediction model was patients’ symptoms, so it was difficult to predict it accurately for patients with few symptoms. Since this study was limited to institutions in Japan that specialize in Kampo, it is unclear whether the results can be applied to other traditional East Asian medicine. Additionally, external validation was not conducted in this study even though we used the data from multiple facilities in various regions of Japan for model construction.
We developed a prediction model on cold–heat patterns with high accuracy, macro-recall, precision, and F1-score with data from patients whose subjective cold/heat-related symptoms matched the cold–heat pattern diagnosis by the physician.
The data that support the findings of this study are not publicly available due to their containing information that could compromise the privacy of research participants but are available from the corresponding author TY upon reasonable request.
The studies involving human participants were reviewed and approved by the appropriate institutional review boards at Keio University, Chiba University, University of Toyama, Jichi Medical University, Iizuka Hospital, and Kameda Medical Center. The studies were conducted in accordance with the local legislation and institutional requirements. The participants provided their written informed consent to participate in this study.
AM-M: conceptualization, formal analysis, writing–original draft, software, and visualization. TY: conceptualization, data curation, funding acquisition, project administration, supervision, validation, and writing–original draft. KK: methodology, supervision, and writing–review and editing. YH: data curation and writing–review and editing. HH: data curation and writing–review and editing. YS: data curation and writing–review and editing. TN (7th author): data curation and writing–review and editing. ET: data curation and writing–review and editing. KM: data curation and writing–review and editing. SHM: data curation and writing–review and editing. RY: methodology, supervision, and writing–review and editing. SI: methodology, supervision, and writing–review and editing. SAM: methodology, supervision, and writing–review and editing. HM: methodology, supervision, and writing–review and editing. KU: writing–review and editing and supervision. TN (16th author): funding acquisition, writing–review and editing, data curation, and supervision. KF: funding acquisition and writing–review and editing. KW: funding acquisition, project administration, and writing–review and editing.
The author(s) declare that financial support was received for the research, authorship, and/or publication of this article. This work was supported by a Grant-in-Aid for Research on Propulsion Study of Clinical Research from the Ministry of Health, Labor and Welfare in building the questionnaire and data collection. An article processing charge and English editing fee for this article were paid by the Japan Agency for Medical Research and Development (JP23lk0310096 and JP24lk0310096). The funding source was not involved in the interpretation of data, writing of the report, and the decision to submit the article for publication.
The authors declare that the research was conducted in the absence of any commercial or financial relationships that could be construed as a potential conflict of interest.
The author(s) declared that they were an editorial board member of Frontiers, at the time of submission. This had no impact on the peer review process and the final decision.
All claims expressed in this article are solely those of the authors and do not necessarily represent those of their affiliated organizations, or those of the publisher, the editors, and the reviewers. Any product that may be evaluated in this article, or claim that may be made by its manufacturer, is not guaranteed or endorsed by the publisher.
The Supplementary Material for this article can be found online at: https://www.frontiersin.org/articles/10.3389/fphar.2024.1412593/full#supplementary-material
Breiman, L., Cutler, A., Liaw, A., and Wiener, M. (2008). Breiman and cutler’s random forests for classification and regression. Package ‘randomForest’. Available at: https://cran.r-project.org/web/packages/randomForest/randomForest.pdf (Accessed October 31, 2018).
Fujiwara, K., Huang, Y., Hori, K., Nishioji, K., Kobayashi, M., Kamaguchi, M., et al. (2020). Over- and under-sampling approach for extremely imbalanced and small minority data problem in Health record analysis. Front. Public. Health 8, 178. doi:10.3389/fpubh.2020.00178
He, H., and Garcia, E. A. (2009). Learning from imbalanced data. IEEE Trans. Knowl. Data Eng. 21, 1041–4347. doi:10.1109/TKDE.2008.239
Katayama, K., Yamaguchi, R., Imoto, S., Matsuura, K., Watanabe, K., and Miyano, S. (2011). Clustering for visual analogue scale data in symbolic data analysis. Procedia Comput. Sci. 6, 370–374. doi:10.1016/j.procs.2011.08.068
Katayama, K., Yamaguchi, R., Imoto, S., Watanabe, K., and Miyano, S. (2014). Analysis of questionnaire for traditional medicine and development of decision support system. Evid. Based. Complement. Altern. Med. 2014, 974139. doi:10.1155/2014/974139
Lee, B. J., Lee, J. C., Nam, J., and Kim, J. Y. (2018a). Prediction of cold and heat patterns using anthropometric measures based on machine learning. Chin. J. Integr. Med. 24, 16–23. doi:10.1007/s11655-016-2641-8
Lee, J., Han, S. Y., Hwang, M., Bae, N., and Chae, H. (2018b). Biopsychological and pathophysiological features of cold-heat subgroup of sasang typology with sasang digestive function inventory, sasang personality questionnaire and body mass index. Integr. Med. Res. 7 (1), 68–76. doi:10.1016/j.imr.2017.09.005
Maeda-Minami, A., Ihara, K., Yoshino, T., Horiba, Y., Mimura, M., and Watanabe, K. (2022). A prediction model of qi stagnation: a prospective observational study referring to two existing models. Comput. Biol. Med. 146, 105619. doi:10.1016/j.compbiomed.2022.105619
Maeda-Minami, A., Yoshino, T., Horiba, Y., Nakamura, T., and Watanabe, K. (2021). Inter-rater reliability of kampo diagnosis for chronic Diseases. J. Altern. Complement. Med. 27 (7), 613–616. doi:10.1089/acm.2020.0298
Maeda-Minami, A., Yoshino, T., Katayama, K., Horiba, Y., Hikiami, H., Shimada, Y., et al. (2019). Prediction of deficiency-excess pattern in Japanese Kampo medicine: multi-centre data collection. Complement. Ther. Med. 45, 228–233. doi:10.1016/j.ctim.2019.07.003
Maeda-Minami, A., Yoshino, T., Katayama, K., Horiba, Y., Hikiami, H., Shimada, Y., et al. (2020). Discrimination of prediction models between cold-heat and deficiency-excess patterns. Complement. Ther. Med. 49, 102353. doi:10.1016/j.ctim.2020.102353
Moschik, E. C., Mercado, C., Yoshino, T., Matsuura, K., and Watanabe, K. (2012). Usage and attitudes of physicians in Japan concerning traditional Japanese medicine (kampo medicine): a descriptive evaluation of a representative questionnaire-based survey. Complement. Altern. Med. 2012, 139818. doi:10.1155/2012/139818
World Health Organization (2023). ICD-11 beta draft. Available at: https://icd.who.int/dev11 (Accessed September 18, 2024).
Keywords: Kampo medicine, the International Classification of Diseases, tangled heat/cold pattern, moderate (heat/cold) pattern, prediction model
Citation: Maeda-Minami A, Yoshino T, Katayama K, Horiba Y, Hikiami H, Shimada Y, Namiki T, Tahara E, Minamizawa K, Muramatsu S-I, Yamaguchi R, Imoto S, Miyano S, Mima H, Uneda K, Nogami T, Fukunaga K and Watanabe K (2024) Machine learning model for predicting the cold–heat pattern in Kampo medicine: a multicenter prospective observational study. Front. Pharmacol. 15:1412593. doi: 10.3389/fphar.2024.1412593
Received: 05 April 2024; Accepted: 30 September 2024;
Published: 25 October 2024.
Edited by:
Hui Hui Zhao, Beijing University of Chinese Medicine, ChinaReviewed by:
Caihong Zeng, Nanjing University School of Medicine, ChinaCopyright © 2024 Maeda-Minami, Yoshino, Katayama, Horiba, Hikiami, Shimada, Namiki, Tahara, Minamizawa, Muramatsu, Yamaguchi, Imoto, Miyano, Mima, Uneda, Nogami, Fukunaga and Watanabe. This is an open-access article distributed under the terms of the Creative Commons Attribution License (CC BY). The use, distribution or reproduction in other forums is permitted, provided the original author(s) and the copyright owner(s) are credited and that the original publication in this journal is cited, in accordance with accepted academic practice. No use, distribution or reproduction is permitted which does not comply with these terms.
*Correspondence: Tetsuhiro Yoshino, dGV0dGEyMTNAa2Vpby5qcA==
†ORCID: Ayako Maeda-Minami, orcid.org/0000-0003-1361-4514; Tetsuhiro Yoshino, orcid.org/0000-0001-6172-1926; Kotoe Katayama, orcid.org/0000-0002-3966-1231; Yuko Horiba, orcid.org/0000-0003-3260-6755; Takao Namiki, orcid.org/0000-0003-1047-7999; Eiichi Tahara, orcid.org/0009-0001-7063-7563; Kiyoshi Minamizawa, orcid.org/0000-0001-8881-4137; Shinichi Muramatsu, orcid.org/0000-0002-3185-7790; Rui Yamaguchi, orcid.org/0000-0003-1224-227X; Seiya Imoto, orcid.org/0000-0002-2989-308X; Satoru Miyano, orcid.org/0000-0002-1753-6616; Hideki Mima, orcid.org/0000-0003-4127-6161; Kazushi Uneda, orcid.org/0000-0002-9660-142X; Tatsuya Nogami, orcid.org/0000-0002-9724-4356; Koichi Fukunaga, orcid.org/0000-0001-7460-1990; Kenji Watanabe, orcid.org/0000-0002-5823-6441
Disclaimer: All claims expressed in this article are solely those of the authors and do not necessarily represent those of their affiliated organizations, or those of the publisher, the editors and the reviewers. Any product that may be evaluated in this article or claim that may be made by its manufacturer is not guaranteed or endorsed by the publisher.
Research integrity at Frontiers
Learn more about the work of our research integrity team to safeguard the quality of each article we publish.