- 1School of Traditional Chinese Pharmacy, China Pharmaceutical University, Nanjing, China
- 2Faculty of Life Science, Anhui Medical University, Hefei, China
- 3Department Biochemistry and Developmental Biology, University of Helsinki, Helsinki, Finland
- 4Research Program in Systems Oncology, Faculty of Medicine, University of Helsinki, Helsinki, Finland
Traditional Chinese Medicine (TCM) has been used for thousands of years to treat human diseases. Recently, many databases have been devoted to studying TCM pharmacology. Most of these databases include information about the active ingredients of TCM herbs and their disease indications. These databases enable researchers to interrogate the mechanisms of action of TCM systematically. However, there is a need for comparative studies of these databases, as they are derived from various resources with different data processing methods. In this review, we provide a comprehensive analysis of the existing TCM databases. We found that the information complements each other by comparing herbs, ingredients, and herb-ingredient pairs in these databases. Therefore, data harmonization is vital to use all the available information fully. Moreover, different TCM databases may contain various annotation types for herbs or ingredients, notably for the chemical structure of ingredients, making it challenging to integrate data from them. We also highlight the latest TCM databases on symptoms or gene expressions, suggesting that using multi-omics data and advanced bioinformatics approaches may provide new insights for drug discovery in TCM. In summary, such a comparative study would help improve the understanding of data complexity that may ultimately motivate more efficient and more standardized strategies towards the digitalization of TCM.
1 Introduction
TCM has not only played a crucial role in the treatment and prevention of disease in ancient times but also is used as a valuable source of natural products in modern drug discovery (Atanasov et al., 2021; Ngo et al., 2013). At present, there are more than 8,000 TCM components in total, which have been reported to have various pharmacological effects (Wangkheirakpam et al., 2018), especially for complex diseases (Yao et al., 2021), such as obesity (Vermaak et al., 2011), nonalcoholic fatty liver disease (Yan et al., 2020), cancer (Wang et al., 2021), and diabetes (Tong et al., 2012). TCM herbs as plant-based substances for medicinal purposes typically refer to the leaves, flowers, stems, seeds, or roots of plants that may induce potential health benefits. They can be used either naturally or as preparations. TCM herbs, as one particular type of natural products, have become increasingly popular in drug discovery in recent years. There are 3,322 clinical trials registered during 1999–2021 in ClinicalTrials.gov (Zhang et al., 2019). For instance, PHY906 is based on Huang-Qin-Tang’s prescription for common gastrointestinal distress and has been studied for seven cancer types in clinical trials (Wang et al., 2011; Saif et al., 2014; Liu, 2015; Ganguly et al., 2019). ACT001 is an analog of parthenolide derivative from the shoots of feverfew (Tanacetum parthenium). It has been approved as orphan drug status by the FDA and is in phase I clinical trials for advanced glioblastoma in China (CTR20171274) and Australia (ACTRN12616000228482) (Zhang et al., 2012).
One of the main characteristics of TCM is that it considers the human body as a holistic system to achieve maximal synergistic effects and minimal side effects (Wang et al., 2012; Zhou et al., 2017; Ramsay et al., 2018). The holistic concepts proposed by the TCM theories thousands of years ago coincide with the system biology concepts in modern medicine (Bahari and Yavari, 2021). As an essential branch of system biology, network pharmacology approaches have attracted considerable attention because of their potential for understanding drug interactions in many complex diseases. Hence, system pharmacology modeling has also been widely applied in TCM to explore active ingredients or targets and to understand therapeutic mechanisms of action (Maetschke et al., 2014; Kibble et al., 2015), such as herb properties (Naghizadeh et al., 2020; Naghizadeh et al., 2021), herb combinations (Vanunu et al., 2010; Hsieh et al., 2011; Wang et al., 2021), TCM diagnosis, and symptoms (Ma et al., 2010; Xie et al., 2018). The construction of networks in TCM mainly consists of associations between five main entities, including formulae, herbs, ingredients, targets, and diseases. Based on the network’s topology, familiar patterns or important nodes can be detected by various algorithms in network analysis. Furthermore, biological pathways or gene ontology (GO) functional terms can be inferred to discover potential mechanisms of actions (MOAs) of active ingredients in TCM (Wang et al., 2021).
Thanks to the rapid development of molecular profiling technologies (Xu et al., 2021), increasing data at multiple omics levels for both herbs and ingredients were available (Guo et al., 2020). These data were curated, standardized, and stored as databases to benefit researchers with valuable resources (Xu et al., 2021). Multiple databases have been established recently, providing diverse information for TCM herbs or ingredients (Lagunin et al., 2014; Lee et al., 2019). For instance, recent reviews summarize the databases and tools currently used for TCM research (Zhang et al., 2019). However, fewer of them have compared the overlap of these databases. Furthermore, coverage of the trends of TCM databases to advance network pharmacology is limited. We first determined their overlapping herbs, ingredients, and herb-ingredient pairs based on all the available data downloaded from major TCM databases published since 2006. Secondly, we reported the developing trend of TCM databases from the perspective of network pharmacology, such as network construction and analysis, external linking databases, and absorption, distribution, metabolism, and excretion (ADME) properties. Finally, we proposed a few promising directions and approaches for improving and developing TCM databases.
2 Overview of the significant TCM databases
Here, we briefly described 14 TCM databases developed during the last two decades. These databases are under active development and, therefore, are expected to capture the recent updates in the TCM research (Figure 1).
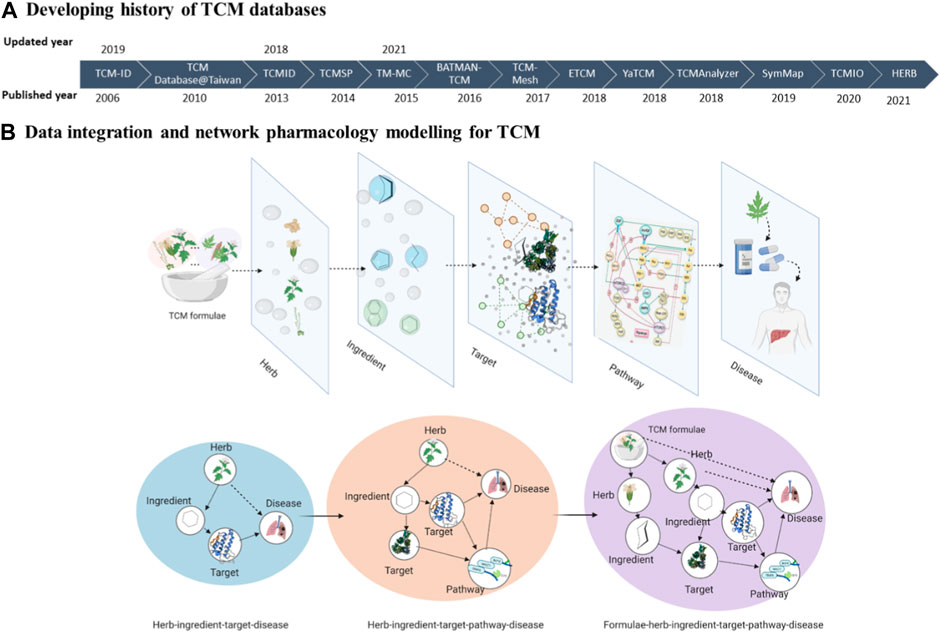
Figure 1. The schematic of this review. (A) Developing history of TCM databases. (B) Data integration and network pharmacology modelling for TCM.
2.1 TCM-ID
TCM-ID (Chen et al., 2006) (http://bidd.group/TCMID/) was initialized in 2006, including prescriptions (n = 1,588), constituent herbs (n = 1,313), herbal ingredients (n = 5,669), and their corresponding molecular information (n = 3,725). The database currently consists of 7,443 prescriptions, 2,751 herbal medicines, and 7,375 chemical ingredients. In particular, the drug-target information for the ingredients has been obtained from an in silico method named INVDOCK (Chen and Zhi, 2001) and, more recently, from experimental validation of bioactivity assays.
2.2 Database@taiwan
Database@taiwan (Chen, 2011) (http://tcm.cmu.edu.tw/) was developed in 2011 and initially contained 20,000 pure compounds and 435 TCM herbs. The number of compounds has increased to about 61,000 more recently. Although virtual screening and molecular simulation approaches are commonly used for drug discovery, their applications are rare in TCM. Therefore, Database@taiwan aimed to support virtual screening or molecular simulation with the molecular structure of ingredients in TCM.
2.3 TCMSP
TCMSP (Ru et al., 2014) (https://old.tcmsp-e.com/index.php) was published in 2012 and then updated in 2014, including 499 herbs, 29,384 ingredients, 3,311 targets, and 837 associated diseases.
TCMSP aims to establish an efficient systems pharmacology platform to integrate various information, such as pharmacochemistry, ADME properties, drug-likeness, and drug targets. In the TCMSP database, a comprehensive network between herbs–compounds–targets–diseases (H–C–T–D) was created to help illustrate the MOAs of TCM herbs, understand the rationale of TCM theory, and discover herb-derived drugs. TCMSP is also one of the first TCM databases that systematically reported ADME properties to enable the filtering of the ingredients that have poor oral absorbability and low drug-likeness.
2.4 TCMID
TCMID (Xue et al., 2013) (http://www.megabionet.org/tcmid/) integrates the data from Database@Taiwan and other databases and the literature. TCMID was updated in 2018, including 49,000 prescriptions, 8,159 herbs, 25,210 ingredients, 3,791 diseases, 6,828 drugs, and 17,521 targets. TCMID visualizes interactions between formulae, herbs, components, and their target proteins to support the network modeling.
2.5 BATMAN-TCM
BATMAN-TCM (Liu et al., 2016) (http://bionet.ncpsb.org.cn/batman-tcm/) is a bioinformatics tool for analyzing molecular mechanisms of TCM published in 2015.
BATMAN-TCM focuses on understanding the multi-component, multi-target, and multi-pathway combinational therapeutic mechanism of TCM. To explore the molecular mechanism of combinations of formulae or herbs, BATMAN-TCM provides the predicted targets for TCM ingredients. Also, BATMAN-TCM is a bioinformatics tool that performs functional analyses and visualization of targets, such as biological pathways, GO functional terms, and disease enrichment analyses.
2.6 TM-MC
TM-MC (Kim et al., 2015a) (http://informatics.kiom.re.kr/compound/) extracted 14,000 chemical compounds from 536 medicinal materials and 4,000 journal articles in MEDLINE and PubMed Central (PMC). Although many TCM databases provide diverse information, the sources of such information are seldom reported; thus, it is difficult to verify them. To solve this limitation, TM-MC aimed to construct a database to provide detailed sources of information in PubMed, PubChem, and ChemSpider for each herb-ingredient pair.
2.7 TCM-Mesh
TCM-Mesh (Zhang et al., 2017) (http://mesh.tcm.microbioinformatics.org) was published in 2017, including 6,235 herbs, 383,840 compounds, 14,298 genes, 6,204 diseases, 144,723 gene-disease associations and 3,440,231 pairs of gene interactions. TCM-Mesh was designed to integrate various resources and is intended to serve as a more comprehensive and user-friendly platform for network pharmacology analysis. In addition, TCM-Mesh provides the toxicity and side effects of ingredients, which is vital for safety assessments during the application of TCM. In total, 163,221 side effect records (1,430 ingredients and 6,123 side effects) were extracted from TOXNET (Fowler and Schnall, 2014) and SIDER (Kuhn et al., 2016).
2.8 TCMAnalyzer
TCMAnalyzer (Liu et al., 2018) (http://www.rcdd.org.cn/tcmanalyzer) was developed in 2017, covering 1,493 formulae, 618 TCM medicine, and 16,437 ingredients.
Many ingredients and their interactions with biological receptors are unknown, which makes it difficult to determine the molecular mechanisms of action. To solve this problem, TCMAnalyzer intended to identify the active ingredients, protein targets, therapeutic mechanisms, and critical structural fragments responsible for the therapeutic activities by cheminformatics and bioinformatics approaches. Compared with other TCM databases, TCMAnalyzer deepens the understanding of the structure of TCM ingredients by substructure-searching tools, similarity-searching tools, and scaffold-searching tools.
2.9 YaTCM
YaTCM (Li et al., 2018) (http://cadd.pharmacy.nankai.edu.cn/yatcm/home) was published in 2018 and contained 47,696 natural compounds, 6,220 herbs, 18,697 targets (including 3,461 therapeutic targets), 1,907 predicted targets, 390 pathways, and 1,813 prescriptions. Compared with other TCM databases, YaTCM supports unique analytical tools, including similarity and substructure searching for potential structures and identifying similar biological functions between herb pairs.
2.10 ETCM
ETCM (Xu et al., 2019) (The Encyclopedia of Traditional Chinese Medicine) (http://www.tcmip.cn/ETCM/) is a web server tool established in 2018 for the network analysis of TCM, including herbs (n = 402), formulae (n = 3,959) and ingredients (n = 7,284). ETCM has some unique characteristics. For instance, the annotation information for herbs and formulae is richer than other databases as ETCM includes not only the habitat and quality control information of herbs but also various drug-likeness information of the ingredients. ETCM also has improved functions for network analysis and visualization.
2.11 SymMap
Clinical symptoms in TCM are vital for diagnosis and treatment. To study the TCM symptoms more systematically, SymMap (Wu et al., 2019) (https://www.symmap.org/) was established in 2019 as an integrative database that maps symptoms in TCM to modern symptoms and diseases, covering 1,717 TCM symptoms, 499 herbs, 961 modern symptoms, 5,235 modern diseases, 4,302 targets, and 19,595 ingredients.
2.12 HERB
The HERB (Fang et al., 2021) database (high-throughput experiment and reference-guided database of traditional Chinese medicine) (http://herb.ac.cn/) is one of the few databases that contain transcriptomic profiles for herbs and ingredients. Established in 2020, HERB has 6,164 gene expression profiles of TCM herbs or ingredients from 1,037 high-throughput experiments. In addition, 12,933 targets and 28,212 diseases were further linked to 7,263 herbs and 49,258 ingredients by statistical inference. Moreover, the gene targets (n = 1,241) and modern disease indications (n = 494) for 473 herbs/ingredients were manually collected from 1,966 scientific references.
HERB aimed to help researchers build a high-quality pharmacology network by gene expression data, thus uncovering evidence-based associations between TCM and modern drugs. In addition, HERB also manually collects high-confidence compound-target interactions and herb-disease associations from the literature.
2.13 TCMIO
Numerous herbs or ingredients have been reported to have immunomodulatory functions and antitumor effects by targeting the immune system. However, their underlying mechanisms remain unclear. To tackle this issue, TCMIO (Liu et al., 2020) (Traditional Chinese Medicine on Immuno-Oncology, http://tcmio.xielab.net) was recently developed in 2020, including 1,493 prescriptions, 618 TCM medicine, 16,437 ingredients, and 32,847 TCM-ingredient-associations.
TCMIO was designed to explore the role of TCM in modulating the cancer immune microenvironment. Unlike other databases, TCMIO focuses only on formulae, herbs, ingredients, targets, and diseases related to immuno-oncology.
2.14 TCMSID
Traditional Chinese Medicine Simplified Integrated Database (TCMSID, https://tcm.scbdd.com/home/index/) covers 499 herbs in the Chinese pharmacopeia and 20,015 ingredients. TCMSID evaluates the structural reliability of all ingredients and their possibility of exerting pharmacological effects. In addition, the potential targets of ingredients are predicted by multiple target prediction tools.
3 Systematic comparison of TCM databases
3.1 Sizes of TCM entities
We compared the number of data points in the TCM databases for nine entities, including herbs, herbs with at least one ingredient, ingredients with structure information, ingredients with at least one target, herb-ingredient pairs, ingredient-target pairs, targets, and diseases. As shown in Figure 2, HERB has the most extensive coverage in eight of these nine entities, except for the number of targets, with 7,263 herbs, 49,258 ingredients, 12,933 targets, and 28,212 diseases. As one of the newly developed databases, HERB integrates information from the other databases, leading to a much more extensive collection of targets and diseases. Other databases, including TCMID, TCM-Mesh, and YaTCM, have a similar number of herbs (n > 6,000) as compared to the remaining databases (n < 2,000). Similarly, the top TCM databases with the largest ingredients are HERB, YaTCM, TCMID, and TCMSP (n > 30,000), while TCM-Mesh has fewer ingredients. In addition, HERB and TCMID have the most abundant herb-ingredient pairs (n > 8,000).
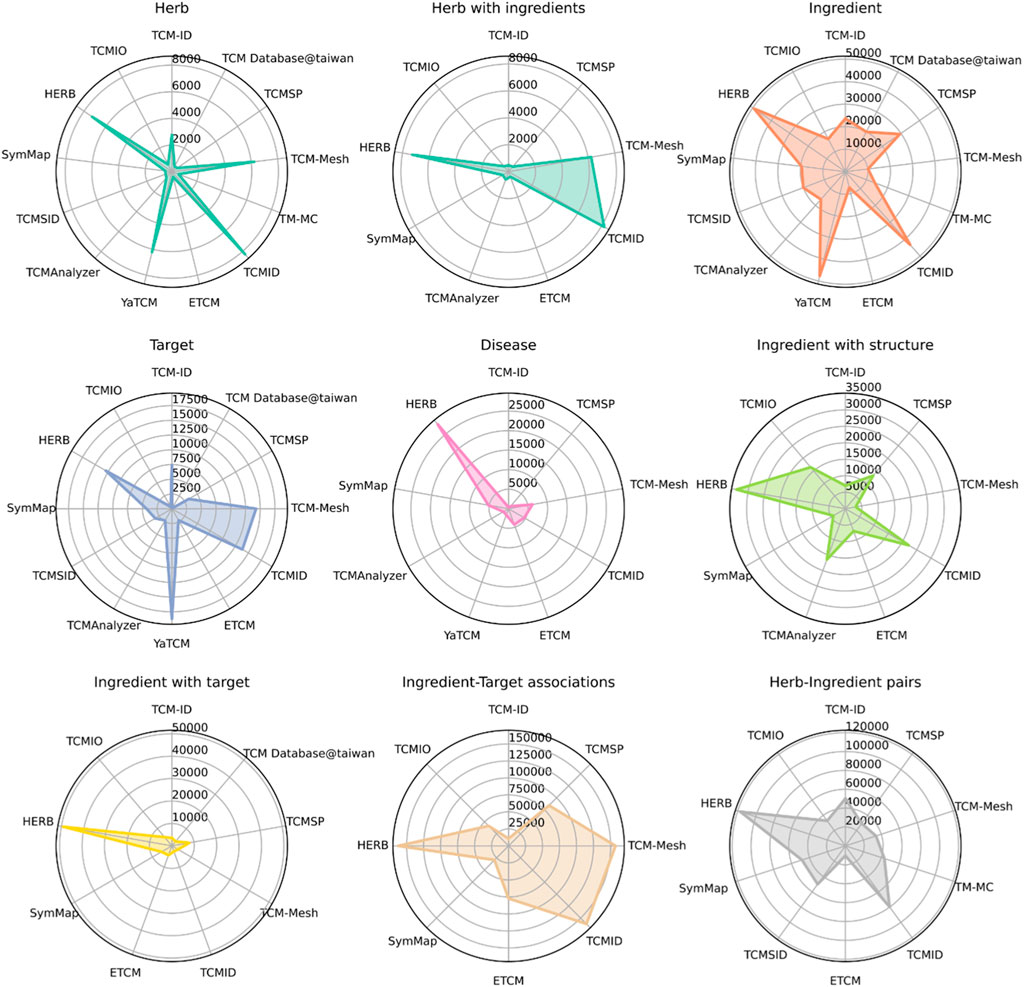
Figure 2. Summary of data sizes for multiple TCM entities, including herbs, herbs with ingredient information, ingredients, targets, diseases, ingredients with structure information, ingredients with target information, ingredient-target interactions, and herb-ingredient interactions. Note that a database does not necessarily contain all these entities’ information. Only the databases with the corresponding data entities are shown for each plot.
In brief, TCM databases have experienced a fast development in recent decades, accumulating information for ∼8,000 herbs, ∼50,000 ingredients, and ∼120,000 herb-ingredients pairs. Moreover, ∼150,000 ingredient-target associations were predicted by computational methods.
3.2 Shared herbs and ingredients
We determine the number of common herbs and ingredients to explore the overlap among the TCM databases. We matched herbs and ingredients by their Chinese names and PubChem IDs respectively, on the TCM databases for which the data can be downloaded. As shown in Figure 3A, HERB has the most unique herbs (n = 3,660), followed by TCM-ID (n = 350) and TM-MC (n = 333). There are only 78 herbs shared among nine databases, suggesting a minimal overlap. When excluding TM-MC, the overlap increases to 116 herbs. Furthermore, TCMID, TCM-Mesh, and HERB share more common herbs than the other databases (n = 1,146).
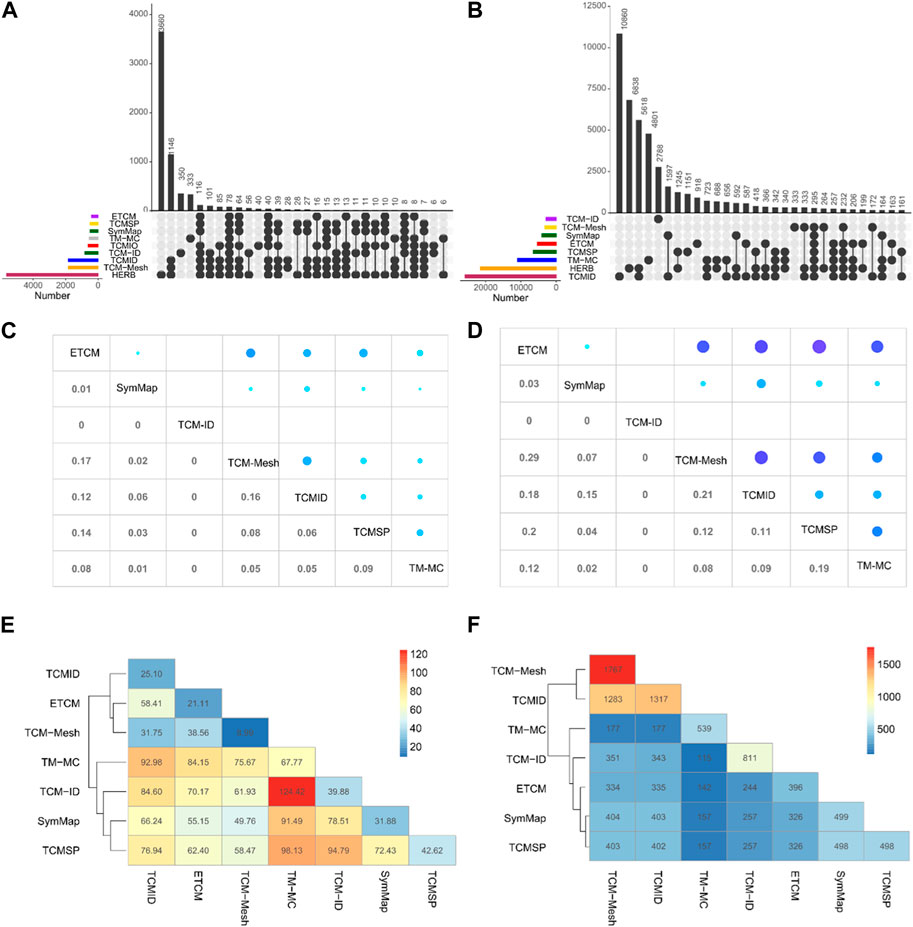
Figure 3. Overlapping of herbs and ingredients between TCM databases. Upset plot for the shared herbs (A) and ingredients (B) among the TCM databases. The color bars at the bottom left represent the number of herbs or ingredients in each TCM database, which can be further collapsed into subclasses depending on whether a herb or an ingredient exists in one or more TCM databases. The vertical bars show the number of shared herbs or ingredients for a particular subset of TCM databases, as indicated by the connected lines below the x-axis between the databases. Average Jaccard coefficients (C) and overlap rates (D) of herb-ingredient relationships between the common herbs in seven TCM databases. The average value of shared herb-ingredient relationships (E) and number of pairwise common herbs (F) between seven TCM databases.
Compared with the overlap situation in herbs, the number of overlapped ingredients between eight databases is lower, with only 295 common elements (Figure 3B). In contrast, TCM databases contain a more significant number of unique ingredients (TCMID = 10,860, HERB = 6,838, TM-MC = 4,801, TCM-ID = 2,788, TCMSP = 1,151, and, ETCM = 918). TCMID and HERB shared the most common ingredients (n = 5,618). Generally, the consistency of the herb information contained among TCM databases is higher than that for ingredients.
3.3 Shared herb-ingredient pairs
Herbal ingredients are vital for exploring the TCM mechanisms at the molecular level. Therefore, we compare the herb-ingredient pairs between the TCM databases. We consider the average overlap rate and Jaccard coefficient across all the common herbs between a given pair of databases. Namely, for a common herb, A and B represent the set of ingredients of this herb in the two databases, respectively. The overlap rate is defined as
As illustrated in Figures 3C, D, TCM-Mesh and TCMID have the maximum average Jaccard index (0.16), while TCM-Mesh and ETCM have the top average overlapped rate (0.29). ETCM has a relatively higher overlap rate with other databases, such as TCM-Mesh (0.29), TCMSP (0.20), TCMID (0.18), and TM-MC (0.12). In contrast, TCM-ID has no overlap with any of the other databases. We found that TM-MC tends to have more common herb-ingredient pairs with other TCM databases, with an average of 124.42 (Figure 3E). For example, for the 177 common herbs in TCM-ID and TM-MC, on average, 124 common herb-ingredient pairs can be identified. Furthermore, TCM-Mesh and TCMID share only 31.75 common herb-ingredient pairs, despite having 1,283 common herbs (Figure 3F). The distribution of shared herb-ingredient associations and the Jaccard index for ingredients of common herbs between TCM databases can be seen in Supplementary Figures S1, S2.
Taken together, we found a relatively low overlap of herbs and their ingredients between different databases, suggesting that a more unified knowledge base is needed to integrate these databases for further study.
3.4 Types of annotations
Annotation of TCM usually contains information about formulae, herbs, ingredients, targets, and disease indications. With the development of TCM databases, annotation types have become increasingly available for many herbs. For example, TCM database@taiwan, one of the earliest TCM databases, only contained the names of TCM herbs. After that, TCMSP, published in 2014, provided therapeutic classes of herbs and their ingredients to support more sophisticated network pharmacology analyses. More recently, TCM databases contain more annotations, such as TCM properties, meridians, disease indications, and therapeutic effects (Figure 4A).
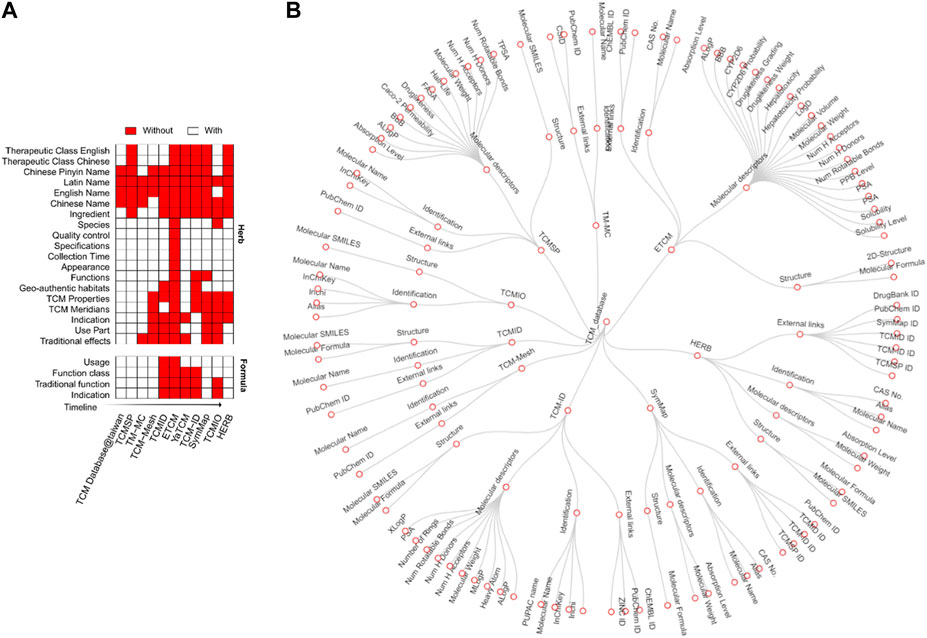
Figure 4. Types of annotations in different databases. (A) Annotation types for herbs (left) and formulae (right). In the heat map, rows are TCM databases, and columns are annotation items, shown in red when available. The databases were ordered by their publishing years from top to bottom. (B) Annotation tree for TCM ingredients. The nodes from inside to outside represent TCM databases, types of ingredient annotation, and their properties, respectively.
Another improvement is the annotation of the TCM formula, a unique concept that specifies how herbs can be combined to treat diseases. TCMID was the first database containing TCM formula information, including usage, classification, and indication. The therapeutic effects of one formula can be classified by the Western medicine system as “indication” and by the traditional medicine system as “function classes” according to their specific “traditional function.” For example, herbs with functional effects nourish the temper and replenish the heart, which belongs to the function class tonic medicine. So far, five databases are providing formulas, including TCM-ID, TCMID, YaTCM, ETCM, and TCMIO (Figure 4A). Although the complete species names are vital to avoid ambiguity in the use of herbal medicine, only the ETCM and ICMIO databases provide species classification. On the other hand, TCM-ID can link the prescription component by its Barcode ID into the Barcode of Life Data Systems (BOLD) database (Ratnasingham and Hebert, 2007). However, the DNA barcoding data was typically determined for two or three genes, which are limited in differentiating plants in the same genus. To improve the quality of the TCM databases, it is necessary to apply standardized reference resources such as Medicinal Plant Names Services (http://mpns.kew.org/mpns-portal/) or Plants of the World Online (http://www.plantsoftheworldonline.org) to reduce the ambiguity about the identities and names of the species. Furthermore, as an important quality control step, DNA sequencing of a comprehensive panel of marker genes should be provided to avoid species misidentification (Rivera et al., 2014).
An annotation tree was plotted to better illustrate the annotation of ingredients in different databases (Figure 4B). There are four main annotation types: ADME properties, external links, structure, and names. For each annotation type, there exists a different number of items. For example, SMILES, PubChem ID, and Mol2 are commonly used to represent the structure of ingredients. Physiological features such as molecular weights and solubility are generally reported for ADME properties. ADME gains increasing interest in the research of TCM as TCM is administered by decoction, which triggers complex absorption, distribution, and metabolism processes. It is known that TCM ingredients can mimic the metabolites of the human body to treat diseases (Kim et al., 2015). Currently, three databases provide ADME properties (Figure 4B), including TCMSP, YaTCM, and ETCM. For example, TCMSP provides 12 ADME properties systematically, such as oral absorbability, half-life, drug-likeness, Caco-2 permeability, blood-brain barrier, and Lipinski’s rule of five. These properties are considered to be essential for drug discovery in TCM. YaTCM focuses on 50 fundamental ADME properties, including four physicochemical descriptors and 48 ADME descriptors. ETCM reports around ten physical-chemical properties and six ADME properties, including blood-brain barrier penetration, CYP450 2D6 inhibition, hepatotoxicity, human intestinal absorption, plasma protein binding, and the quantitative estimate of drug-likeness (QED). Considering these ADME properties of ingredients in the study of network pharmacology could help to prioritize the potential compounds for drug discovery.
In summary, although the annotation for herbs and ingredients has also been improved, the ADMET properties were only found in four databases, with notable differences.
3.5 Network pharmacology modeling to explore the mechanisms of action
Protein targets of ingredients are essential for the MOAs of disease treatment in TCM (Chen et al., 2003). In TCM databases, the validated ingredient-target interactions are mainly extracted from four resources, including 1) Text mining from the literature, including TCM-ID and HERB; 2) the ChEMBL database (Gaulton et al., 2017), including TCM-ID, TCMAnalyzer, and TCMIO; 3) the STITCH database (Kuhn et al., 2008), including TCMID and TCM-Mesh; and 4) the HIT database (Ye et al., 2011), including TCMSP.
In addition to validated targets, most TCM databases provide predicted targets from computational methods (Table 1). In databases published before 2014, docking methods are commonly used. For example, TCM-ID implemented a ligand-protein inverse docking strategy called INVDOCK to search targets in the Protein Data Bank (PDB) (Chen and Zhi, 2001). Database@taiwan also predicts compound-target interactions by virtual screening with docking and molecular dynamics simulations. However, docking-based virtual screening approaches are usually demanding on proteins’ computational resources and 3D structures. Therefore, more TCM databases began to implement similarity-based target prediction models. For instance, TCMSP utilizes a SysDT model (Yu et al., 2012), and YaTCM utilizes a multi-voting chemical similarity ensemble approach (Wang et al., 2016). TCMIO relies on a balanced substructure-drug-target network-based inference [bSDTNBI (Wu et al., 2016)] approach based on heat diffusion modeling. In TCMSID, the potential targets of ingredients are predicted by metaTARFISHER (https://metatarget.scbdd.com/), a tool that provides multiple algorithms, including SwissTargetPrediction (Gfeller et al., 2014; Daina et al., 2019), SEA (Wang et al., 2016), HitPickV2 (Hamad et al., 2019), Polypharmacology Browser and Polypharmacology Browser 2 (Awale and Reymond, 2019). In contrast, HERB applies Fisher’s exact test to infer the targets directly from the manually collected 1,966 references rather than docking or similarity-based target prediction.
Many TCM databases harbor a mixture of experimentally validated and computationally predicted targets. In addition, the targets for herbs and formulae are usually considered as a union of targets from their ingredients, which is not necessarily true as their underlying target interactions are much more complex. Specific target prediction models at the TCM herb or formula levels are still in the early stages, with a few examples (Gu and Lai, 2020).
3.6 Disease-related properties
To help understand the rationale of TCM, most databases classify herbs and their disease indications inferred from the putative targets. Furthermore, the disease indications are annotated with commonly accepted standard terms. For example, the TCM-ID database has 153 functional classes, 380 disease indications, and 366 ICD-11 categories. In detail, there are 114,651 formulae-indication pairs involving 7,440 formulae and 380 indications. There are also 17,624 functions, covering 6,465 formulae and 4,629 functions. Similarly, in TCMSP, the disease information (2,387 target-disease pairs) was established by retrieving 2,387 targets and 84,260 compound-target pairs from the TTD database (Chen et al., 2002) (https://doi.org/10.1093/nar/gkp1014) and PharmGKB (Barbarino et al., 2018) (https://www.pharmgkb.org/). In contrast, the gene-disease associations in TCM-Mesh were collected from the GAD database (Becker et al., 2004). The ETCM database utilizes multiple resources, such as Phenotype Ontology (Köhler et al., 2017), Online Mendelian Inheritance in Man (OMIM) (Amberger and Hamosh, 2017), Database of gene-disease associations (DisGeNET) (Piñero et al., 2015) and ORPHANET database (Pavan et al., 2017). In YaTCM, the disease indication of formulae and herbs is based on the therapeutic phenotypes rather than their target genes. Unlike the previously mentioned databases that rely on targets for disease classification, the SymMap database aims to map TCM symptoms into disease indications directly (Xie et al., 2020). Namely, SymMap first curates 1,717 TCM symptoms of 499 herbs and then maps them to 961 symptoms in modern medicine. These current symptoms were finally linked to 5,235 diseases. As multiple levels of associations for formula, herbs, ingredients, targets, and diseases have been established, network pharmacology modeling has become a standard technique to tackle the mechanisms of action of TCM, where the KEGG pathway and GO analyses have been commonly used.
In summary, despite multiple databases that have provided ingredient-disease, herb-disease, and formulae-disease associations, many of them were inferred from computational approaches. In contrast, the disease symptom classifications are well-defined, although they differ from those used in mainstream medicine. As a result, phenotypic-based drug discovery (PDD) is a favorable strategy for finding new indications for TCM.
3.7 Interconnections of TCM databases
The relationships between these TCM databases are shown in Figure 5. TCMSP, TCM-ID, and TCMID were published before 2014 and were further utilized by other more recent databases, such as TCMAnalyzer and SymMap. HERB integrated information from the most significant number of other TCM databases, followed by ETCM and SymMap. We found that the TCM databases utilize multiple data sources that are grouped into four categories, including:
1) Target databases and tools (e.g., target prediction tools, target-target interaction, and annotation databases)
2) Compound databases and tools (e.g., compound annotation)
3) Disease databases and tools (e.g., disease annotation, disease genes, pathways, and symptom databases)
4) Others (e.g., scientific literature databases and gene expression databases)
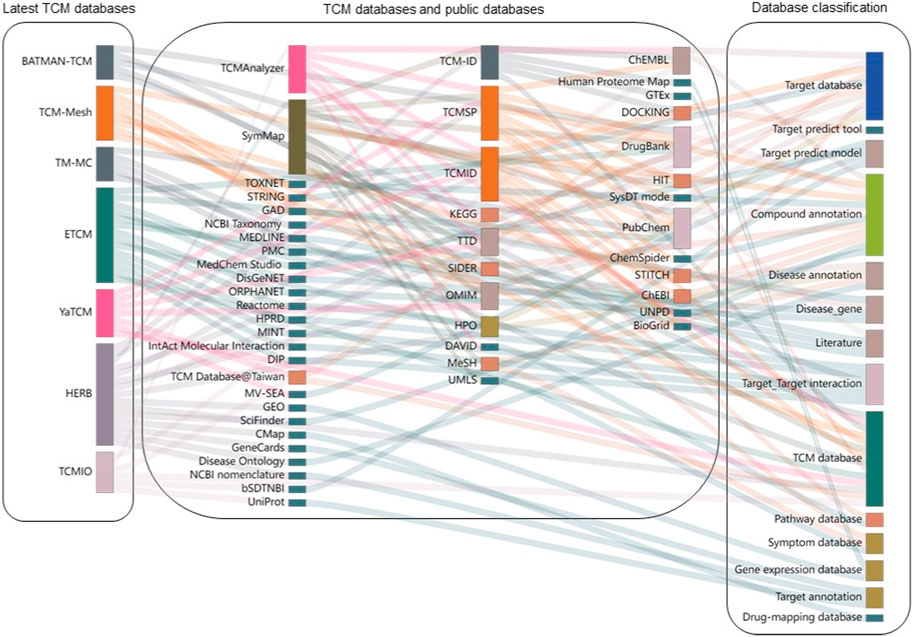
Figure 5. Interconnections of TCM databases and their data sources. Each TCM database is shown as bars on the left side, connecting to their data sources in the middle panel. These data sources are further grouped into different categories on the right side. The height of each rectangle represents the frequency with which it was linked to other databases.
Many data sources are commonly utilized in multiple TCM databases, such as PubChem, DrugBank, and ChEMBL, to annotate compounds and targets. As shown in Figure 5, the most extensively involved data source is compound annotation databases (n = 10), including PubChem and DrugBank. In addition, various target-related databases (n = 9), such as DrugBank, OMIM, and ChEMBL, are also utilized. However, there are quite a few data sources that are used by specific TCM databases. For example, Reactome (Matthews et al., 2009), HPRD (Peri et al., 2003), MINT (Zanzoni et al., 2002), DisGeNet, and GAD are only used for ETCM, while GEO (Barrett et al., 2013), CMAP (Lamb et al., 2006), and GeneCards (Safran et al., 2010) are unique resources for HERB. Therefore, it is expected that connecting TCM databases to other public medicinal databases via compound-target and target-disease associations can enhance our understanding of herbal medicine at the molecular level.
4 Discussions
The lack of information has been a limiting factor for exploring and applying TCM. With the development of computational tools, increasingly comprehensive TCM databases have been developed. To fully use all the available databases, it is essential to compare them comprehensively. Although there are several comparative studies, most of them covered TCM databases published before 2018, and little comparison about ingredients and herb-ingredient pairs has been made.
In this study, we comprehensively analyze 14 major TCM databases. We compared the recent trends of TCM data curation, including their primary functions, annotations, network analysis, and visualization tools. We searched for the herbs by their Chinese names and found that the information about their ingredients differ across different databases. We also found that these TCM databases provide ununified annotation for herbs or ingredients, especially for the structure information of the ingredients, making it challenging to integrate data from them. Furthermore, we summarized novel multi-omics and advanced bioinformatics approaches that have been applied in the study of TCM, such as symptoms or gene expressions, which may provide new insights for drug discovery from TCM. We foresee that such a comparative study would help improve the understanding of data complexity that may ultimately motivate more efficient and more standardized strategies towards the digitalization of TCM.
TCM databases have been developed rapidly. Initially, the databases contained only basic information (e.g., TCM-ID, TCMdatabse@taiwan, and TCMSP), and increasing volumes of data have been added to enable a network pharmacology visualization (e.g., TCMID and TCM-Mesh), and functional analyses (e.g., ETCM and YaTCM). A notable trend is that more specific databases, such as SymMap, have been intended for symptoms and HERB mainly for transcriptional data. In addition, the ingredient search functions are becoming more flexible and powerful. With these tools, ingredients can be searched in TCM databases through direct keywords such as herbs, SMILES, or names and structures or substructures. If two compounds are similar in structure, they usually have identical properties or biological activities (Jafari et al., 2020). Hence, a comparison of the structural similarity between TCM ingredients and known drugs is needed. Several TCM databases have provided such a functionality. For instance, YaTCM uses the likeness of KEGG (Kanehisa et al., 2017) pathways to search potential ingredients, while TCMAnalyzer is based on molecular fingerprints’ similarity. Furthermore, drug-target prediction methods are commonly used in BATMAN-TCM and TCMID.
Harmonization of terminology is critical for improving the quality of TCM databases. Among these databases, BATMAN-TCM, TM-MC, HERB, and TCMIO provide scientific binomials for plants. Particularly, TCMIO provides scientific plant names, coupled with the names of publishing authors, to avoid potential ambiguity. As shown in Figure 4, Latin names of the herbs were commonly found across the databases. However, the majority of them were adopted from Pharmacopoeia to refer to herbal substances. These pharmacopeia names were not as precise as scientific botanical nomenclature. To ensure a better standardization of herbal substances, we recommend the use of the Medicinal Plant Names Services (http://mpns.kew.org/mpns-portal/) for nomenclatural indexing and references. On the other hand, the information on used parts was found in five databases, including TCM-Mesh, TCMID, ETCM, SymMap, and TCMIO, while the location and time of herb harvesting is available only in ETCM. Furthermore, we found that these TCM databases commonly lack information on the fingerprinting protocols, such as high-performance liquid chromatography (HPLC), gas chromatography (GC), and mass spectrometry (MS). According to the Consensus statement on the Phytochemical Characterization of Medicinal Plant extract (ConPhyMP) (Heinrich et al., 2022) (https://ga-online.org/best-practice), fingerprinting protocols contain essential information to ensure the reproducibility and interpretation of herb extract characterization. The current lack of such information across the TCM databases presents a critical limitation to reusing the data for more integrative analyses. Therefore, to improve the sharing of data and resources for the TCM research community, the FAIR (Findable, Accessible, Interoperable, and Reusable) principle should be carefully followed, similar to the data curation efforts for modern medicine (Almada et al., 2020; Tanoli et al., 2022).
Recently, many studies have performed high-throughput transcriptomic profiling for ingredients, herbs, and formulas. HERB is one of the first TCM databases to provide high-throughput gene expression data for herbs and ingredients, mainly from the GEO database. The differentially expressed genes (DEGs) were obtained by comparing samples treated with ingredients or herbs and control samples. These DEGs will lead to identifying pathways that are affected by TCM. Compared with the putative targets, the pathways derived from gene expression data may be more reliable to represent the holistic effects of specific herbs or ingredients. Therefore, we foresee that the increasing availability of molecular profiling data may open opportunities for more advanced bioinformatics and machine learning approaches to tackle the complexity of TCM.
In conclusion, our study covered an extensive collection of commonly used TCM databases. Also, the developing trends in TCM databases were summarized in the aspects of their primary functions, annotations, and network analysis. More importantly, we compared their overlaps of herbs, ingredients, and herb-ingredient associations. We found that TCM databases provide different complementary sets of information, suggesting the necessity of TCM database harmonization. Our comparison of TCM databases would help to deepen the understanding of TCM databases and to integrate a diversity of data efficiently from TCM databases.
Data availability statement
The original contributions presented in the study are included in the article/Supplementary Material, further inquiries can be directed to the corresponding authors.
Author contributions
YW: Conceptualization, Formal Analysis, Methodology, Visualization, Writing–original draft, Writing–review and editing. ML: Formal Analysis, Writing–review and editing. MJ: Conceptualization, Data curation, Supervision, Writing–original draft, Writing–review and editing. JT: Conceptualization, Funding acquisition, Methodology, Project administration, Supervision, Writing–original draft, Writing–review and editing.
Funding
The author(s) declare that financial support was received for the research, authorship, and/or publication of this article. This work was supported by the European Research Council Starting Grant agreement (grant number 716063), the Academy of Finland Research Fellow funding (grant number 317680), the Helsinki Institute of Life Science Research Fellow funding, and the Program for Innovative Research Team of Jiangsu Province, Jiangsu Province Science Foundation for Youths (grant number BK202310). YW was supported by the China Scholarship Council (grant number 201706740080).
Acknowledgments
We acknowledge Kenneth P. K. Quek from the University of Helsinki Language Center for his support in language editing.
Conflict of interest
The authors declare that the research was conducted in the absence of any commercial or financial relationships that could be construed as a potential conflict of interest.
Publisher’s note
All claims expressed in this article are solely those of the authors and do not necessarily represent those of their affiliated organizations, or those of the publisher, the editors and the reviewers. Any product that may be evaluated in this article, or claim that may be made by its manufacturer, is not guaranteed or endorsed by the publisher.
Supplementary material
The Supplementary Material for this article can be found online at: https://www.frontiersin.org/articles/10.3389/fphar.2024.1303693/full#supplementary-material
SUPPLEMENTARY FIGURE S1 | The distribution of shared herb-ingredient associations of common herbs between TCM databases.
SUPPLEMENTARY FIGURE S2 | The distribution of the Jaccard index for ingredients in common herbs between TCM databases.
References
Almada, M., Midão, L., Portela, D., Dias, I., Núñez-Benjumea, F. J., Parra-Calderón, C. L., et al. (2020). Um novo paradigma em investigação em saúde: dados FAIR (localizáveis, acessíveis, interoperáveis, reutilizáveis). Acta Med. Port. 33 (12), 828–834. doi:10.20344/amp.12910
Amberger, J. S., and Hamosh, A. (2017). Searching online mendelian inheritance in man (OMIM): a knowledgebase of human genes and genetic phenotypes. Curr. Protoc. Bioinforma. 58, 1. doi:10.1002/cpbi.27
Atanasov, A. G., Zotchev, S. B., Dirsch, V. M., and Supuran, C. T. (2021). Natural products in drug discovery: advances and opportunities. Nat. Rev. Drug Discov. 20 (3), 200–216. doi:10.1038/s41573-020-00114-z
Awale, M., and Reymond, J. L. (2019). Polypharmacology browser PPB2: target prediction combining nearest neighbors with machine learning. J. Chem. Inf. Model 59 (1), 10–17. doi:10.1021/acs.jcim.8b00524
Bahari, F., and Yavari, M. (2021). Hot and cold theory: evidence in systems biology. Adv. Exp. Med. Biol. 1343, 135–160. doi:10.1007/978-3-030-80983-6_9
Barbarino, J. M., Whirl-Carrillo, M., Altman, R. B., and Klein, T. E. (2018). PharmGKB: a worldwide resource for pharmacogenomic information. Wiley Interdiscip. Rev. Syst. Biol. Med. 10 (4), e1417. doi:10.1002/wsbm.1417
Barrett, T., Wilhite, S. E., Ledoux, P., Evangelista, C., Kim, I. F., Tomashevsky, M., et al. (2013). NCBI GEO: archive for functional genomics data sets--update. Nucleic Acids Res. 41, D991–D995. doi:10.1093/nar/gks1193
Becker, K. G., Barnes, K. C., Bright, T. J., and Wang, S. A. (2004). The genetic association database. Nat. Genet. 36 (5), 431–432. doi:10.1038/ng0504-431
Chen, C. Y. (2011). TCM Database@Taiwan: the world's largest traditional Chinese medicine database for drug screening in silico. PLoS One 6 (1), e15939. doi:10.1371/journal.pone.0015939
Chen, X., Ji, Z. L., and Chen, Y. Z. (2002). TTD: therapeutic target database. Nucleic Acids Res. 30 (1), 412–415. doi:10.1093/nar/30.1.412
Chen, X., Ung, C. Y., and Chen, Y. (2003). Can an in silico drug-target search method be used to probe potential mechanisms of medicinal plant ingredients? Nat. Prod. Rep. 20 (4), 432–444. doi:10.1039/b303745b
Chen, X., Zhou, H., Liu, Y. B., Wang, J. F., Li, H., Ung, C. Y., et al. (2006). Database of traditional Chinese medicine and its application to studies of mechanism and to prescription validation. Br. J. Pharmacol. 149 (8), 1092–1103. doi:10.1038/sj.bjp.0706945
Chen, Y. Z., and Zhi, D. G. (2001). Ligand-protein inverse docking and its potential use in the computer search of protein targets of a small molecule. Proteins 43 (2), 217–226. doi:10.1002/1097-0134(20010501)43:2<217::aid-prot1032>3.0.co;2-g
Daina, A., Michielin, O., and Zoete, V. (2019). SwissTargetPrediction: updated data and new features for efficient prediction of protein targets of small molecules. Nucleic Acids Res. 47 (W1), W357–w364. doi:10.1093/nar/gkz382
Fang, S., Dong, L., Liu, L., Guo, J., Zhao, L., Zhang, J., et al. (2021). HERB: a high-throughput experiment- and reference-guided database of traditional Chinese medicine. Nucleic Acids Res. 49 (D1), D1197–d1206. doi:10.1093/nar/gkaa1063
Fowler, S., and Schnall, J. G. (2014). TOXNET: information on toxicology and environmental health. Am. J. Nurs. 114 (2), 61–63. doi:10.1097/01.NAJ.0000443783.75162.79
Ganguly, A., Frank, D., Kumar, N., Cheng, Y. C., and Chu, E. (2019). Cancer biomarkers for integrative oncology. Curr. Oncol. Rep. 21 (4), 32. doi:10.1007/s11912-019-0782-6
Gaulton, A., Hersey, A., Nowotka, M., Bento, A. P., Chambers, J., Mendez, D., et al. (2017). The ChEMBL database in 2017. Nucleic Acids Res. 45 (D1), D945–d954. doi:10.1093/nar/gkw1074
Gfeller, D., Grosdidier, A., Wirth, M., Daina, A., Michielin, O., and Zoete, V. (2014). SwissTargetPrediction: a web server for target prediction of bioactive small molecules. Nucleic Acids Res. 42, W32–W38. doi:10.1093/nar/gku293
Gu, S., and Lai, L. H. (2020). Associating 197 Chinese herbal medicine with drug targets and diseases using the similarity ensemble approach. Acta Pharmacol. Sin. 41 (3), 432–438. doi:10.1038/s41401-019-0306-9
Guo, R., Luo, X., Liu, J., Liu, L., Wang, X., and Lu, H. (2020). Omics strategies decipher therapeutic discoveries of traditional Chinese medicine against different diseases at multiple layers molecular-level. Pharmacol. Res. 152, 104627. doi:10.1016/j.phrs.2020.104627
Hamad, S., Adornetto, G., Naveja, J. J., Chavan Ravindranath, A., Raffler, J., and Campillos, M. (2019). HitPickV2: a web server to predict targets of chemical compounds. Bioinformatics 35 (7), 1239–1240. doi:10.1093/bioinformatics/bty759
Heinrich, M., Jalil, B., Abdel-Tawab, M., Echeverria, J., Kulić, Ž., McGaw, L. J., et al. (2022). Best Practice in the chemical characterisation of extracts used in pharmacological and toxicological research-The ConPhyMP-Guidelines. Front. Pharmacol. 13, 953205. doi:10.3389/fphar.2022.953205
Hsieh, H. Y., Chiu, P. H., and Wang, S. C. (2011). Epigenetics in traditional Chinese pharmacy: a bioinformatic study at pharmacopoeia scale. Evid. Based Complement. Altern. Med. 2011, 816714. doi:10.1093/ecam/neq050
Jafari, M., Wang, Y., Amiryousefi, A., and Tang, J. (2020). Unsupervised learning and multipartite network models: a promising approach for understanding traditional medicine. Front. Pharmacol. 11, 1319. doi:10.3389/fphar.2020.01319
Kanehisa, M., Furumichi, M., Tanabe, M., Sato, Y., and Morishima, K. (2017). KEGG: new perspectives on genomes, pathways, diseases and drugs. Nucleic Acids Res. 45 (D1), D353–d361. doi:10.1093/nar/gkw1092
Kibble, M., Saarinen, N., Tang, J., Wennerberg, K., Mäkelä, S., and Aittokallio, T. (2015). Network pharmacology applications to map the unexplored target space and therapeutic potential of natural products. Nat. Prod. Rep. 32 (8), 1249–1266. doi:10.1039/c5np00005j
Kim, H. U., Ryu, J. Y., Lee, J. O., and Lee, S. Y. (2015b). A systems approach to traditional oriental medicine. Nat. Biotechnol. 33 (3), 264–268. doi:10.1038/nbt.3167
Kim, S. K., Nam, S., Jang, H., Kim, A., and Lee, J. J. (2015a). TM-MC: a database of medicinal materials and chemical compounds in Northeast Asian traditional medicine. BMC Complement. Altern. Med. 15, 218. doi:10.1186/s12906-015-0758-5
Köhler, S., Vasilevsky, N. A., Engelstad, M., Foster, E., McMurry, J., Aymé, S., et al. (2017). The human phenotype ontology in 2017. Nucleic Acids Res. 45 (D1), D865–d876. doi:10.1093/nar/gkw1039
Kuhn, M., Letunic, I., Jensen, L. J., and Bork, P. (2016). The SIDER database of drugs and side effects. Nucleic Acids Res. 44 (D1), D1075–D1079. doi:10.1093/nar/gkv1075
Kuhn, M., von Mering, C., Campillos, M., Jensen, L. J., and Bork, P. (2008). STITCH: interaction networks of chemicals and proteins. Nucleic Acids Res. 36, D684–D688. doi:10.1093/nar/gkm795
Lagunin, A. A., Goel, R. K., Gawande, D. Y., Pahwa, P., Gloriozova, T. A., Dmitriev, A. V., et al. (2014). Chemo- and bioinformatics resources for in silico drug discovery from medicinal plants beyond their traditional use: a critical review. Nat. Prod. Rep. 31 (11), 1585–1611. doi:10.1039/c4np00068d
Lamb, J., Crawford, E. D., Peck, D., Modell, J. W., Blat, I. C., Wrobel, M. J., et al. (2006). The Connectivity Map: using gene-expression signatures to connect small molecules, genes, and disease. Science 313 (5795), 1929–1935. doi:10.1126/science.1132939
Lee, W. Y., Lee, C. Y., Kim, Y. S., and Kim, C. E. (2019). The methodological trends of traditional herbal medicine employing network pharmacology. Biomolecules 9 (8), 362. doi:10.3390/biom9080362
Li, B., Ma, C., Zhao, X., Hu, Z., Du, T., Xu, X., et al. (2018). YaTCM: yet another traditional Chinese medicine database for drug discovery. Comput. Struct. Biotechnol. J. 16, 600–610. doi:10.1016/j.csbj.2018.11.002
Liu, W. J. (2015). What has been overlooked on study of Chinese materia medica in the West? Chin. J. Integr. Med. 21 (7), 483–492. doi:10.1007/s11655-015-2081-x
Liu, Z., Cai, C., Du, J., Liu, B., Cui, L., Fan, X., et al. (2020). TCMIO: a comprehensive database of traditional Chinese medicine on immuno-oncology. Front. Pharmacol. 11, 439. doi:10.3389/fphar.2020.00439
Liu, Z., Du, J., Yan, X., Zhong, J., Cui, L., Lin, J., et al. (2018). TCMAnalyzer: a chemo- and bioinformatics web service for analyzing traditional Chinese medicine. J. Chem. Inf. Model 58 (3), 550–555. doi:10.1021/acs.jcim.7b00549
Liu, Z., Guo, F., Wang, Y., Li, C., Zhang, X., Li, H., et al. (2016). BATMAN-TCM: a bioinformatics analysis tool for molecular mechANism of traditional Chinese medicine. Sci. Rep. 6, 21146. doi:10.1038/srep21146
Ma, T., Tan, C., Zhang, H., Wang, M., Ding, W., and Li, S. (2010). Bridging the gap between traditional Chinese medicine and systems biology: the connection of Cold Syndrome and NEI network. Mol. Biosyst. 6 (4), 613–619. doi:10.1039/b914024g
Maetschke, S. R., Madhamshettiwar, P. B., Davis, M. J., and Ragan, M. A. (2014). Supervised, semi-supervised and unsupervised inference of gene regulatory networks. Brief. Bioinform 15 (2), 195–211. doi:10.1093/bib/bbt034
Matthews, L., Gopinath, G., Gillespie, M., Caudy, M., Croft, D., de Bono, B., et al. (2009). Reactome knowledgebase of human biological pathways and processes. Nucleic Acids Res. 37, D619–D622. doi:10.1093/nar/gkn863
Naghizadeh, A., Hamzeheian, D., Akbari, S., Mohammadi, F., Otoufat, T., Asgari, S., et al. (2020). UNaProd: a universal natural product database for materia medica of Iranian traditional medicine. Evid. Based Complement. Altern. Med. 2020, 3690781. doi:10.1155/2020/3690781
Naghizadeh, A., Salamat, M., Hamzeian, D., Akbari, S., Rezaeizadeh, H., Vaghasloo, M. A., et al. (2021). IrGO: Iranian traditional medicine General Ontology and knowledge base. J. Biomed. Semant. 12 (1), 9. doi:10.1186/s13326-021-00237-1
Ngo, L. T., Okogun, J. I., and Folk, W. R. (2013). 21st century natural product research and drug development and traditional medicines. Nat. Prod. Rep. 30 (4), 584–592. doi:10.1039/c3np20120a
Pavan, S., Rommel, K., Mateo Marquina, M. E., Höhn, S., Lanneau, V., and Rath, A. (2017). Clinical practice guidelines for rare diseases: the orphanet database. PLoS One 12 (1), e0170365. doi:10.1371/journal.pone.0170365
Peri, S., Navarro, J. D., Amanchy, R., Kristiansen, T. Z., Jonnalagadda, C. K., Surendranath, V., et al. (2003). Development of human protein reference database as an initial platform for approaching systems biology in humans. Genome Res. 13 (10), 2363–2371. doi:10.1101/gr.1680803
Piñero, J., Queralt-Rosinach, N., Bravo, À., Deu-Pons, J., Bauer-Mehren, A., Baron, M., et al. (2015). DisGeNET: a discovery platform for the dynamical exploration of human diseases and their genes. Database (Oxford) 2015, bav028. doi:10.1093/database/bav028
Ramsay, R. R., Popovic-Nikolic, M. R., Nikolic, K., Uliassi, E., and Bolognesi, M. L. (2018). A perspective on multi-target drug discovery and design for complex diseases. Clin. Transl. Med. 7 (1), 3. doi:10.1186/s40169-017-0181-2
Ratnasingham, S., and Hebert, P. D. N. (2007). bold: the Barcode of Life Data System (http://www.barcodinglife.org). Mol. Ecol. Notes 7 (3), 355–364. doi:10.1111/j.1471-8286.2007.01678.x
Rivera, D., Allkin, R., Obón, C., Alcaraz, F., Verpoorte, R., and Heinrich, M. (2014). What is in a name? The need for accurate scientific nomenclature for plants. J. Ethnopharmacol. 152 (3), 393–402. doi:10.1016/j.jep.2013.12.022
Ru, J., Li, P., Wang, J., Zhou, W., Li, B., Huang, C., et al. (2014). TCMSP: a database of systems pharmacology for drug discovery from herbal medicines. J. Cheminform 6, 13. doi:10.1186/1758-2946-6-13
Safran, M., Dalah, I., Alexander, J., Rosen, N., Iny Stein, T., Shmoish, M., et al. (2010). GeneCards Version 3: the human gene integrator. Database (Oxford) 2010, baq020. doi:10.1093/database/baq020
Saif, M. W., Li, J., Lamb, L., Kaley, K., Elligers, K., Jiang, Z., et al. (2014). First-in-human phase II trial of the botanical formulation PHY906 with capecitabine as second-line therapy in patients with advanced pancreatic cancer. Cancer Chemother. Pharmacol. 73 (2), 373–380. doi:10.1007/s00280-013-2359-7
Tanoli, Z., Aldahdooh, J., Alam, F., Wang, Y., Seemab, U., Fratelli, M., et al. (2022). Minimal information for chemosensitivity assays (MICHA): a next-generation pipeline to enable the FAIRification of drug screening experiments. Brief. Bioinform 23 (1), bbab350. doi:10.1093/bib/bbab350
Tong, X. L., Dong, L., Chen, L., and Zhen, Z. (2012). Treatment of diabetes using traditional Chinese medicine: past, present and future. Am. J. Chin. Med. 40 (5), 877–886. doi:10.1142/S0192415X12500656
Vanunu, O., Magger, O., Ruppin, E., Shlomi, T., and Sharan, R. (2010). Associating genes and protein complexes with disease via network propagation. PLoS Comput. Biol. 6 (1), e1000641. doi:10.1371/journal.pcbi.1000641
Vermaak, I., Viljoen, A. M., and Hamman, J. H. (2011). Natural products in anti-obesity therapy. Nat. Prod. Rep. 28 (9), 1493–1533. doi:10.1039/c1np00035g
Wang, E., Bussom, S., Chen, J., Quinn, C., Bedognetti, D., Lam, W., et al. (2011). Interaction of a traditional Chinese Medicine (PHY906) and CPT-11 on the inflammatory process in the tumor microenvironment. BMC Med. Genomics 4, 38. doi:10.1186/1755-8794-4-38
Wang, K., Chen, Q., Shao, Y., Yin, S., Liu, C., Liu, Y., et al. (2021a). Anticancer activities of TCM and their active components against tumor metastasis. Biomed. Pharmacother. 133, 111044. doi:10.1016/j.biopha.2020.111044
Wang, S., Hu, Y., Tan, W., Wu, X., Chen, R., Cao, J., et al. (2012). Compatibility art of traditional Chinese medicine: from the perspective of herb pairs. J. Ethnopharmacol. 143 (2), 412–423. doi:10.1016/j.jep.2012.07.033
Wang, X., Wang, Z. Y., Zheng, J. H., and Li, S. (2021c). TCM network pharmacology: a new trend towards combining computational, experimental and clinical approaches. Chin. J. Nat. Med. 19 (1), 1–11. doi:10.1016/S1875-5364(21)60001-8
Wang, Y., Yang, H., Chen, L., Jafari, M., and Tang, J. (2021b). Network-based modeling of herb combinations in traditional Chinese medicine. Brief. Bioinform 22 (5). doi:10.1093/bib/bbab106
Wang, Z., Liang, L., Yin, Z., and Lin, J. (2016). Improving chemical similarity ensemble approach in target prediction. J. Cheminform 8, 20. doi:10.1186/s13321-016-0130-x
Wangkheirakpam, S. (2018). “Chapter 2 - traditional and folk medicine as a target for drug discovery,” in Natural products and drug discovery. Editors S. C. Mandal, V. Mandal, and T. Konishi (Amsterdam, Netherlands: Elsevier), 29–56.
Wu, Y., Zhang, F., Yang, K., Fang, S., Bu, D., Li, H., et al. (2019). SymMap: an integrative database of traditional Chinese medicine enhanced by symptom mapping. Nucleic Acids Res. 47 (D1), D1110–d1117. doi:10.1093/nar/gky1021
Wu, Z., Lu, W., Wu, D., Luo, A., Bian, H., Li, J., et al. (2016). In silico prediction of chemical mechanism of action via an improved network-based inference method. Br. J. Pharmacol. 173 (23), 3372–3385. doi:10.1111/bph.13629
Xie, G., Wang, S., Zhang, H., Zhao, A., Liu, J., Ma, Y., et al. (2018). Poly-pharmacokinetic study of a multicomponent herbal medicine in healthy Chinese volunteers. Clin. Pharmacol. Ther. 103 (4), 692–702. doi:10.1002/cpt.784
Xie, Y., Feng, Y., Di Capua, A., Mak, T., Buchko, G. W., Myler, P. J., et al. (2020). A phenotarget approach for identifying an alkaloid interacting with the tuberculosis protein Rv1466. Mar. Drugs 18 (3), 149. doi:10.3390/md18030149
Xu, H., Zhang, Y., Wang, P., Zhang, J., Chen, H., Zhang, L., et al. (2021). A comprehensive review of integrative pharmacology-based investigation: a paradigm shift in traditional Chinese medicine. Acta Pharm. Sin. B 11 (6), 1379–1399. doi:10.1016/j.apsb.2021.03.024
Xu, H. Y., Zhang, Y. Q., Liu, Z. M., Chen, T., Lv, C. Y., Tang, S. H., et al. (2019). ETCM: an encyclopaedia of traditional Chinese medicine. Nucleic Acids Res. 47 (D1), D976–d982. doi:10.1093/nar/gky987
Xue, R., Fang, Z., Zhang, M., Yi, Z., Wen, C., and Shi, T. (2013). TCMID: traditional Chinese Medicine integrative database for herb molecular mechanism analysis. Nucleic Acids Res. 41, D1089–D1095. doi:10.1093/nar/gks1100
Yan, T., Yan, N., Wang, P., Xia, Y., Hao, H., Wang, G., et al. (2020). Herbal drug discovery for the treatment of nonalcoholic fatty liver disease. Acta Pharm. Sin. B 10 (1), 3–18. doi:10.1016/j.apsb.2019.11.017
Yao, C. L., Zhang, J. Q., Li, J. Y., Wei, W. L., Wu, S. F., and Guo, D. A. (2021). Traditional Chinese medicine (TCM) as a source of new anticancer drugs. Nat. Prod. Rep. 38 (9), 1618–1633. doi:10.1039/d0np00057d
Ye, H., Ye, L., Kang, H., Zhang, D., Tao, L., Tang, K., et al. (2011). HIT: linking herbal active ingredients to targets. Nucleic Acids Res. 39, D1055–D1059. doi:10.1093/nar/gkq1165
Yu, H., Chen, J., Xu, X., Li, Y., Zhao, H., Fang, Y., et al. (2012). A systematic prediction of multiple drug-target interactions from chemical, genomic, and pharmacological data. PLoS One 7 (5), e37608. doi:10.1371/journal.pone.0037608
Zanzoni, A., Montecchi-Palazzi, L., Quondam, M., Ausiello, G., Helmer-Citterich, M., and Cesareni, G. (2002). MINT: a Molecular INTeraction database. FEBS Lett. 513 (1), 135–140. doi:10.1016/s0014-5793(01)03293-8
Zhang, Q., Lu, Y., Ding, Y., Zhai, J., Ji, Q., Ma, W., et al. (2012). Guaianolide sesquiterpene lactones, a source to discover agents that selectively inhibit acute myelogenous leukemia stem and progenitor cells. J. Med. Chem. 55 (20), 8757–8769. doi:10.1021/jm301064b
Zhang, R., Zhu, X., Bai, H., and Ning, K. (2019b). Network pharmacology databases for traditional Chinese medicine: review and assessment. Front. Pharmacol. 10, 123. doi:10.3389/fphar.2019.00123
Zhang, R. Z., Yu, S. J., Bai, H., and Ning, K. (2017). TCM-Mesh: the database and analytical system for network pharmacology analysis for TCM preparations. Sci. Rep. 7 (1), 2821. doi:10.1038/s41598-017-03039-7
Zhang, X., Tian, R., Zhao, C., Birch, S., Lee, J. A., Alraek, T., et al. (2019a). The use of pattern differentiation in WHO-registered traditional Chinese medicine trials – a systematic review. Eur. J. Integr. Med. 30, 100945. doi:10.1016/j.eujim.2019.100945
Keywords: Traditional Chinese Medicine, TCM databases, network pharmacology, mechanisms of action, drug discovery
Citation: Wang Y, Liu M, Jafari M and Tang J (2024) A critical assessment of Traditional Chinese Medicine databases as a source for drug discovery. Front. Pharmacol. 15:1303693. doi: 10.3389/fphar.2024.1303693
Received: 01 October 2023; Accepted: 15 April 2024;
Published: 26 April 2024.
Edited by:
Juei-Tang Cheng, Chang Jung Christian University, TaiwanReviewed by:
Jun Liu, China Academy of Chinese Medical Sciences, ChinaBob Allkin, Royal Botanic Gardens, Kew, United Kingdom
Opeyemi Iwaloye, Federal University of Technology, Nigeria
Copyright © 2024 Wang, Liu, Jafari and Tang. This is an open-access article distributed under the terms of the Creative Commons Attribution License (CC BY). The use, distribution or reproduction in other forums is permitted, provided the original author(s) and the copyright owner(s) are credited and that the original publication in this journal is cited, in accordance with accepted academic practice. No use, distribution or reproduction is permitted which does not comply with these terms.
*Correspondence: Yinyin Wang, yinyin.wang@cpu.edu.cn; Jing Tang, jing.tang@helsinki.fi