- 1Department of Anesthesiology, First Hospital of Jilin University, Changchun, China
- 2First Hospital of Jilin University, Changchun, China
Background: Gastric cancer is one of the most important malignancies with poor prognosis. Ferroptosis and cuproptosis are newly discovered metal-dependent types of programmed cell death, which may directly affect the outcome of gastric cancer. Long noncoding RNAs (lncRNAs) can affect the prognosis of cancer with stable structures, which could be potential prognostic prediction factors for gastric cancer.
Methods: Differentially expressed metal-dependent programmed cell death (PCD)-related lncRNAs were identified with DESeq2 and Pearson’s correlation analysis. Through GO and KEGG analyses and GSEA , we identified the potential effects of metal-dependent PCD-related lncRNAs on prognosis. Using Cox regression analysis with the LASSO method, we constructed a 12-lncRNA prognostic signature model. Also, we evaluated the prognostic efficiency with Kaplan–Meier (K-M) survival curve, receiver operating characteristic (ROC) curve, and decision curve analysis (DCA) methods. The sensitivities for antitumor drugs were then predicted with the pRRophetic method. Also, we discuss Chinese patent medicines and plant extracts that could induce metal-dependent programmed cell death.
Results: We constructed a metal-dependent PCD-related lncRNA-gene co-expression network. Also, a metal-dependent PCD-related gastric cancer prognostic signature model including 12 lncRNAs was constructed. The K-M survival curve revealed a poor prognosis in the high-risk group. ROC curve analysis shows that the AUC of our model is 0.766, which is better than that of other published models. Moreover, the half-maximum inhibitory concentration (IC50) for dasatinib, lapatinib, sunitinib, cytarabine, saracatinib, and vinorelbine was much lower among the high-risk group.
Conclusion: Our 12 metal-dependent PCD-related lncRNA prognostic signature model may improve the OS prediction for gastric cancer. The antitumor drug sensitivity analysis results may also be helpful for individualized chemotherapy regimen design.
Introduction
Gastric cancer is one of the most important malignancies worldwide, resulting in unhealthy dietary habits, increasing social burden, and helicobacter pylori infection. Further, 1,089,103 new cases and 768,793 death cases occurred in 2020 (Sung et al., 2021). Although surgical methods and adjuvant therapeutic technologies have improved rapidly, the outcome of gastric cancer patients has not improved. Especially when progressed to an advanced status, the 5-year overall survival (OS) rates remained lower than 20 percent (Ferlay et al., 2019; Tan, 2019). At present, prognostic evaluation is referred to the tumor node metastasis (TNM) classification standard. But the drug response and individual variation would also affect the outcome of gastric cancer, due to the tumor mutation burden (TMB) (Chen et al., 2022; Lee et al., 2022) and drug-resistant status (Wang et al., 2022a; Blange et al., 2022; Zhong et al., 2022). Therefore, it is urgently needed to identify novel powerful prognostic markers and to predict the drug sensitivity status for gastric cancer.
Ferroptosis and cuproptosis are newly discovered metal-dependent types of programmed cell death (PCD) distinct from apoptosis and autophagy (Dixon et al., 2012; Tang et al., 2019; Wang et al., 2022b). Through the accumulation of reactive oxygen species (ROC), ferroptosis controls cell death by the dysregulation of glutathione peroxidase activities (Li et al., 2020). Meanwhile, cuproptosis is a copper-triggered modality of mitochondrial cell death, which may be also mediated by lipoylated TCA cycle proteins (Cobine and Brady, 2022; Li et al., 2022; Tang et al., 2022). Recent studies have shown that the aberrant expression of ferroptosis- and cuproptosis-related genes are critical risk factors that directly affect the prognosis of cancer patients (Xie et al., 2016; Roemhild et al., 2021). Moreover, long noncoding RNAs (lncRNAs) are special endogenic functional molecules, which regulate the metabolic progress of gastric cancer cells (Ransohoff et al., 2018). The aberrant expression of lncRNAs regulates the functions of ferroptosis- and cuproptosis-related genes (Mao et al., 2018; Wang et al., 2019; Wu et al., 2020; Zhang et al., 2022a), which are potential prognostic biomarker pools (Statello et al., 2021).
In our study, we collected the RNA-seq, clinicopathological, survival, and simple nucleotide variation data of stomach adenocarcinoma (STAD) from The Cancer Genome Atlas (TCGA) database. Also, we screened the differentially expressed (DE) genes and lncRNAs. Then, we constructed the metal-dependent PCD-related lncRNA-gene co-expression network. GO and KEGG analyses and GSEA showed that these lncRNAs affect the patients’ prognosis status. A 12-lncRNA signature model for gastric cancer prognostic evaluation was built based on metal-dependent PCD-related lncRNAs. Receiver operating characteristic (ROC) curve analysis shows that the AUC value is 0.766. Also, drug sensitivity analysis showed that the half-maximum inhibitory concentration (IC50) for dasatinib, lapatinib, sunitinib, cytarabine, saracatinib, and vinorelbine was much lower among the high-risk group. Therefore, the prognostic evaluation model constructed in our study may improve the prognostic prediction for gastric cancer patients.
Materials and methods
Data collection
We collected the open RNA-seq data, clinicopathological data, OS information, and simple nucleotide variation data for stomach adenocarcinoma (STAD) and para-carcinoma tissues from The Cancer Genome Atlas (TCGA) database (https://cancergenome.nih.gov/) (Hutter and Zenklusen, 2018). Totally, transcriptome data for 434 tissues (387 STAD tissues and 47 normal tissues from 387 patient samples) were downloaded. Then, in order to annotate the RNA-seq data, we also collected the TCGA annotation information version 22 from the GENCODE database (https://www.gencodegenes.org/) (Frankish et al., 2019). The ferroptosis-related gene list was downloaded from the FerrDb database (http://www.zhounan.org/ferrdb/), and the cuproptosis-related gene list was collected among all published reports (Zhou and Bao, 2020) (detailed in Supplementary Table S1). In addition, the gene sets used for gene set enrichment analysis (GSEA) were collected from MSigDB (http://www.gsea-msigdb.org/gsea/index.jsp) (Subramanian et al., 2005).
Identification of metal-dependent programmed cell death-related differentially expressed lncRNAs
“DESeq2” R package was used to identify the DE lncRNAs and DE genes under R version 4.0.4. Moreover, lncRNAs or genes with the threshold values |log2(FoldChange)| > 1 and p-value < 0.05 were defined as DE. Then, we used Pearson’s correlation analysis to identify the co-expression relationships, and lncRNAs with threshold values |cor| > 0.7 and p-value < 0.05 were defined as co-expressed.
Construction for the metal-dependent programmed cell death-related lncRNA-gene co-expression networks
Utilizing Cytoscape version 3.8.3, we visualized the metal-dependent programmed cell death lncRNA-gene co-expression network. Also, the edge widths represent Pearson’s correlation coefficient. The node sizes represent the betweenness centrality for each molecule.
Functional analysis of the metal-dependent programmed cell death-related lncRNA-gene co-expression networks
The “clusterProfiler” R package was used to identify the functions of the co-expression networks involved under R version 4.0.4. We performed Gene Ontology (GO) annotation to identify the functions for each gene and Kyoto Encyclopedia of Genes and Genomes (KEGG) pathway enrichment analysis to identify the significantly involved metabolism pathways, with default parameters. Then, we performed gene set enrichment analysis (GSEA) with the gene set “h.all.v7.5.1.entrez.gmt” downloaded from MSigDB.
Construction of a metal-dependent programmed cell death-related lncRNA prognostic model
The “survival” and “glmnet” R packages were used to perform Cox regression with the least absolute shrinkage and selection operator (LASSO) algorithm and multivariate Cox regression analysis under R version 4.0.4. Then, utilizing the prognostic model, risk scores were calculated with the formula
Nomogram and calibration analysis
The “rms” R package was used to construct the nomogram in order to predict the 1-, 3-, and 5-year OS rates of gastric cancer patients under R version 4.0.4. “rmda” R package was then used to build the calibration curve and evaluate the consistency between the OS rates predicted by the nomogram and the actually observed OS rates (Zhang and Kattan, 2017).
Survival and reactive oxygen species analysis
The “survival” and “survminer” R packages were used to perform the Kaplan–Meier (K-M) survival curve analysis on the OS rates under R version 4.0.4. Also, the “survivalROC” R package was also used for ROC analysis.
Tumor mutation burden analysis
Tumor mutation burden (TMB) is the total exonic mutation count per megabase of tumor DNA. Using homemade Perl script, we calculated the tumor mutation burden (TMB) scores for each STAD sample. The difference in TMB scores between the high-risk and low-risk group was calculated with R version 4.0.4.
Immune functions and immunotherapy prediction
The “limma,” “GSVA,” “GSEABase,” and “ggpubr” R packages were used to evaluate the immune escape functions and immunotherapy difference between the high-risk and low-risk groups, which were divided according to the predicted results of PCD-related lncRNA under R version 4.0.4.
Drug sensitivity prediction
The “pRRophetic” R package (Geeleher et al., 2014) was used to predict the clinical drug sensitivities for antitumor drugs under R version 4.0.4. The correlation between risk scores and half-maximum inhibitory concentration (IC50) was evaluated. Also, the difference in drug response for high-risk and low-risk groups was exhibited with the bar plot.
Results
Identification of metal-dependent programmed cell death-related differentially expressed lncRNAs
Following the workflow shown in Supplementary Figure S1, we totally identified 4466 DE genes (2125 upregulated and 2341 downregulated), as well as 3391 DE lncRNAs (2394 upregulated and 997 downregulated), detailed in Supplementary Table S2. Among these genes, 84 DE metal-dependent PCD-related genes were identified (23 upregulated and 61 downregulated, detailed in Supplementary Table S3). Through Pearson’s correlation analysis (|cor| ≥ 0.7 and p-value < 0.05), we identified 271 metal-dependent PCD-related DE lncRNAs among gastric cancer tissues (Supplementary Table S4).
In order to identify the potential effects of metal-dependent PCD-related lncRNAs on gastric cancer outcomes, we built a lncRNAs-genes co-expression network (Figure 1A, detailed in Supplementary Table S4). GO annotation shows that the genes involved in this network played important roles in “GO:0010038 response to metal ion,” “GO:0006979 response to oxidative stress,” and “GO:0072593 reactive oxygen species metabolic process” (Figure 1B, detailed in Supplementary Table S5). Also, the “HIF-1 signaling pathway,” “FoxO signaling pathway,” and “ErbB signaling pathway” were significantly enriched with the KEGG pathway enrichment analysis (Figure 1C, detailed in Supplementary Table S6). Moreover, GSEA showed “HALLMARK E2F targets” which was also significantly identified (Figure 1D). All these results mentioned earlier indicated that the metal-dependent PCD-related lncRNAs would regulate the prognosis of gastric cancer by controlling the activities of oncogenic pathways, which could be potential prognostic prediction features.
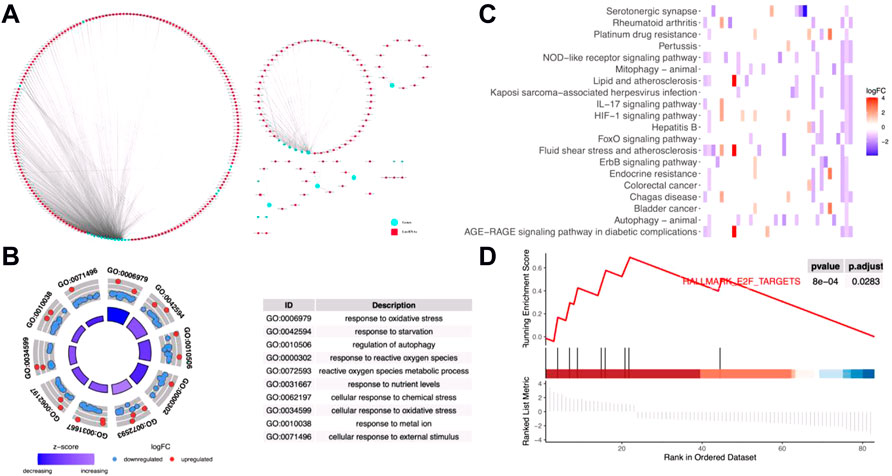
FIGURE 1. Functions of metal-dependent programmed cell death-related genes. (A) lncRNAs-mRNAs co-expression network. Blue circles represent differentially expressed ferroptosis- and cuproptosis-related genes among gastric cancer tissues. Red squares represent co-expressed lncRNAs with relative coefficient over 0.7. (B–D) Gene Ontology (GO) annotation (B) and Kyoto Encyclopedia of Genes and Genomes (KEGG) enrichment analyses (C) and gene set enrichment analysis (GSEA) (D) were performed among genes involved in the co-expression network.
Construction of metal-dependent programmed cell death-related prognostic prediction lncRNA signatures
Then, based on the expression levels of the 271 DE metal-dependent PCD-related lncRNAs and OS information, a gastric cancer prognostic prediction model was identified by Cox regression analysis with the LASSO method (Figure 2 and Supplementary Figure S2 for univariated Cox regression analysis). In this model, 12 metal-dependent PCD-related lncRNA signatures were involved in gastric cancer prognostic evaluation (Table 1), including seven prognostic risk factors (ENSG00000230387.2, ENSG00000233262.1, ENSG00000241111.1, ENSG00000248279.4, ENSG00000248356.1, ENSG00000249807.1, and ENSG00000250303.3) and four prognostic protective factors (ENSG00000221819.5, ENSG00000239265.4, ENSG00000265194.1, and ENSG00000266957.1).
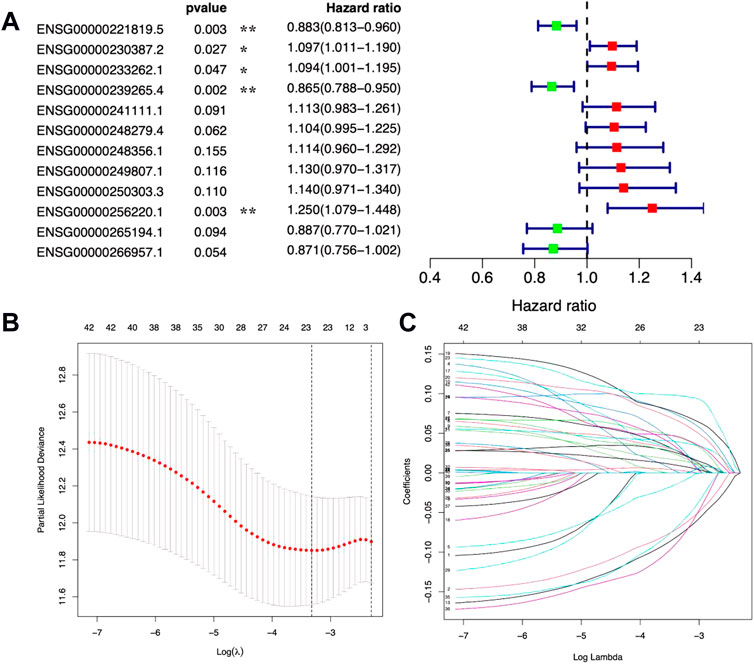
FIGURE 2. Prognostic values of 12 metal-dependent programmed cell death-related lncRNAs. * p-value < 0.05 and ** p-value < 0.01. (A) Forest plot for prognostic values. (B,C) Cv-fit (B) and lambda (C) values for the prognostic prediction model.
Prognostic evaluation of the 12 metal-dependent programmed cell death-related lncRNA signatures
Based on the expression levels of 12 metal-dependent PCD-related lncRNA signatures, we calculated the risk score for each sample among the “training,” “testing,” and “all samples” groups (Figure 3A). Also, the patients were divided into high-risk (risk scores higher than the median) and low-risk (risk scores lower than the median) groups. The risk score distributions for each patient were shown in Figures 3B,C, which showed a worse survival status in the high-risk group.
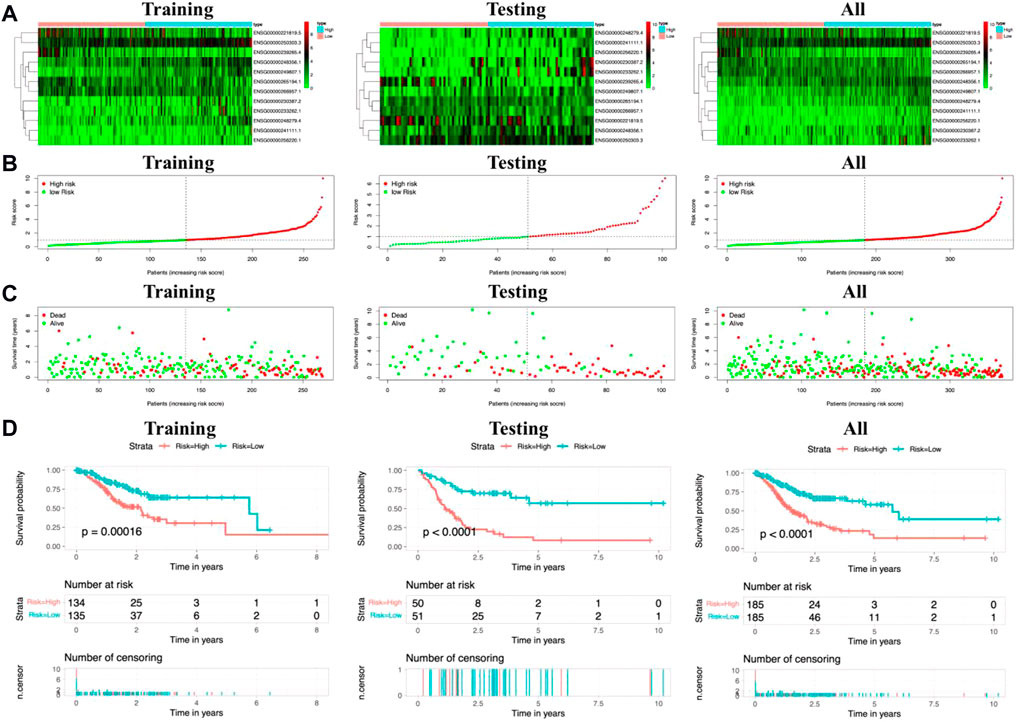
FIGURE 3. Risk scores and survival evaluation for the prognostic prediction model. (A–C) Prognostic signature signal heatmaps (A), risk score distributions (B), and overall survival (OS) status distributions (C) for “training,” “testing,” and “all sample” groups. (D) Kaplan–Meier (K-M) survival curves for patients separated into the high-risk and low-risk groups.
In the meantime, the prognostic effectiveness of these 12 lncRNA signatures was evaluated with K-M survival analysis. The OS rates for the high-risk group were significantly poorer than those of the low-risk group (Figure 3D, p-value < 0.0001). Using ROC curve analysis, the AUC value in the training group is 0.766 (Figure 4A), 0.672 in the testing group (Figure 4B), and 0.704 in all samples (Figure 4C). Then, in order to evaluate the independence of the 12 lncRNA prognostic signatures, we performed univariate and multivariate Cox regression analysis. The results of which indicated that the prognostic prediction model constructed in our work was an independent prognostic evaluation factor (HR = 1.493, 95% CI = 1.339–1.665, p-value < 0.001, Figures 4D,E). Additionally, the relationships between the 12 lncRNA signatures and clinicopathological features are shown with a heatmap in Figure 4F.
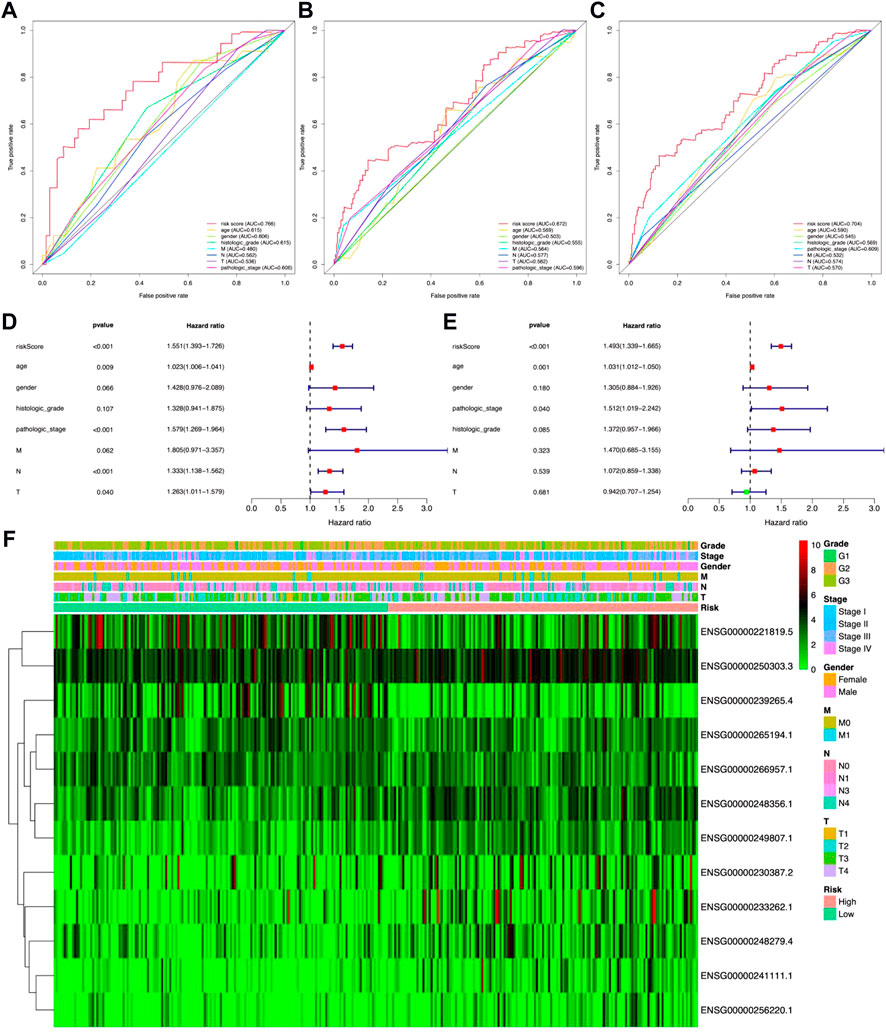
FIGURE 4. Identification ability and independence evaluation for the prognostic prediction model. (A–C) Receiver operating characteristic (ROC) curve analysis for the prognostic model based on 12 metal-dependent programmed cell death-related lncRNAs. (D,E) Forest plot for the overall survival (OS) prognostic values through univariate Cox regression analysis (D) and multivariate Cox regression analysis (E). (F) Heatmap for the relationship between risk scores and clinical phenotypes of patients.
Construction and evaluation of the metal-dependent programmed cell death-related lncRNA-based prognostic nomogram
In order to evaluate the potential clinical practicality of the 12 metal-dependent PCD-related lncRNA signatures, a nomogram was constructed with the risk scores and the clinicopathological features for gastric cancer patients. As shown in Figure 5A, we observed that the higher the risk score calculated, the poorer the prognosis predicted. Also, the range of risk scores could cover the 1-, 3-, and 5-year overall survival rates. The DCA curve shows better clinical practicality for gastric cancer patient prognostic prediction (Figure 5B). Then, the calibration curve showed relatively good fits for the 1- (Figure 5C), 3- (Figure 5D), and 5-year OS prediction (Figure 5E).
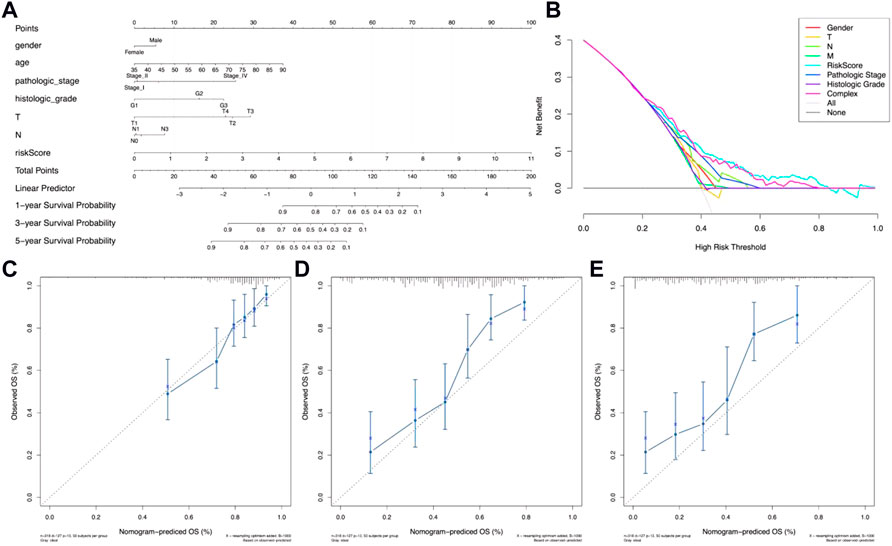
FIGURE 5. Nomogram of OS prediction of gastric cancer patients. (A) Nomogram for risk scores and clinical pathological factors. (B) Decision curve analysis (DCA) of the clinical practicality evaluation of the nomogram. (C–E) Calibration curve analysis of the 1-year (C), 3-year (D), and 5-year (E) survival prediction accuracy.
Tumor mutation burden, immune functions, and drug sensitivity prediction
TMB, immune function, and drug sensitivity might also affect the outcome of gastric cancer, which may impact the prognostic prediction of the 12 lncRNA signatures. In order to evaluate the effect of TMB, we calculated the TMB scores for each gastric cancer patient and observed no significant difference between high-risk and low-risk groups (Figure 6A). The K-M survival analysis results showed that patients with high TMB scores exhibited poorer OS status (Figure 6B). Also, a combination of TMB scores may improve the OS prediction for gastric cancer (Figure 6C).
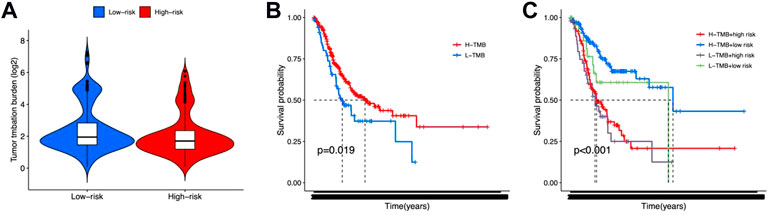
FIGURE 6. Tumor mutation burden for high-risk and low-risk groups. (A) Violin plot for tumor mutation burden showed no significant difference between high-risk and low-risk groups. (B,C) K-M survival curves shown the survival status of whether or not to combine the tumor mutation burden factors.
Tumor Immune Dysfunction and Exclusion (TIDE) was then performed to evaluate immune functions and immunotherapy prediction. We observed that “APC co-stimulation,” “CCR,” “MHC class I,” “parainflammation,” and “type I IFN response” were significantly different between high-risk and low-risk groups (Figure 7A). But the TIDE scores between these two groups showed no difference (Figure 7B).
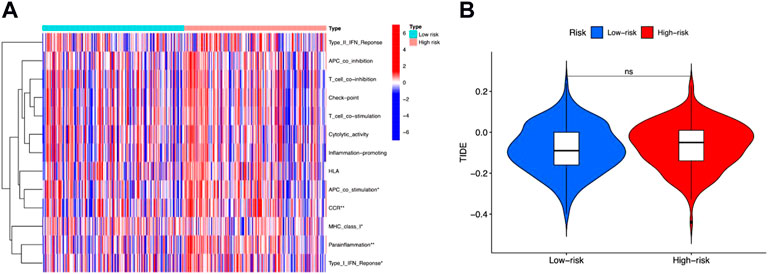
FIGURE 7. Immune functions and immunotherapy prediction. (A) Immune function correlation heatmap. (B) Immunotherapy prediction shown no significant difference between high-risk and low-risk groups.
Finally, we also predicted drug sensitivities for clinical antigastric cancer drugs. Results in Figure 8 show that the IC50 for dasatinib (Figure 8A), lapatinib (Figure 8B), sunitinib (Figure 8C), cytarabine (Figure 8D), and saracatinib (Figure 8E) was negatively correlated with risk scores. Also, the IC50 was remarkably lower among high-risk groups, which means that these antitumor drugs would be more sensitive for high-risk gastric cancer patients. However, IC50 for vinorelbine (Figure 8F), OSI-027 (Figure 8G), CP724714 (Figure 8H), EX-527 (Figure 8I), and FH535 (Figure 8J) was positively correlated with risk scores, which were also significantly higher in high-risk groups. In addition, the sensitivities for other antitumor drugs are shown in Supplementary Figures S3, S4. Although these results still need further clinical verification, these predictions may also be helpful for gastric cancer prognostic evaluation and individualized chemotherapy regimen design.
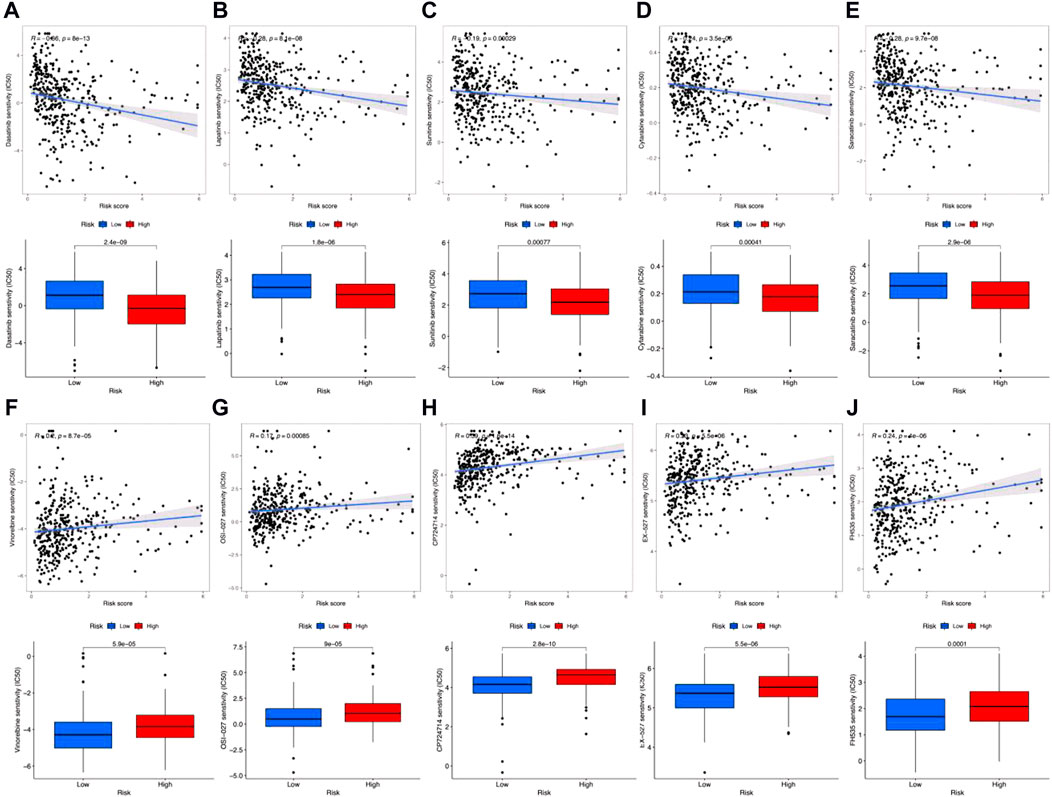
FIGURE 8. Clinically used antigastric cancer drug sensitivity prediction. (A–J) Correlation coefficients (up) and difference (down) between IC50 and risk scores among dasatinib (A), lapatinib (B), sunitinib (C), cytarabine (D), saracatinib (E), vinorelbine (F), OSI-027 (G), CP724714 (H), EX-527 (I), and FH535 (J).
Discussion
Ferroptosis and cuproptosis are newly identified metal-dependent types of programmed cell death distinct from apoptosis, autophagy, and pyroptosis (Dixon et al., 2012; Tang et al., 2022). Ferroptosis is driven by iron-triggered peroxidation while cuproptosis is a copper-dependent modality of mitochondrial cell death (Doll et al., 2017; Cobine and Brady, 2022; Li et al., 2022). Recent studies have shown that
Both ferroptosis and cuproptosis played important roles in the generation and outcome of solid tumors. Through promoting the location of DPP4 in a nonenzymatically active nucleus, TP53 could inhibit ferroptosis and promote the growth of colorectal cancer cells (Xie et al., 2017). In gastric cancer, apatinib exhibits its antitumor activity by inducing the ferroptosis process through lipid peroxidation (Zhao et al., 2021). Also, some studies showed the expression of ferroptosis- and cuproptosis-related genes and lncRNA-exhibited tumor prognostic prediction values. Pan et al. (2021) showed that the AUC value for 17 ferroptosis-related lncRNAs was 0.751 in gastric cancer. The cuproptosis-related risk score would predict the outcome of hepatocellular carcinoma (HCC) with an AUC value of 0.72 (Zhang et al., 2022b), and Zhang et al. (2022a) also constructed a cuproptosis-related lncRNA signature for HCC with a higher AUC value 0.739. But the cuproptosis-related genes and lncRNAs are still not used for gastric cancer prognostic prediction. In our study, both ferroptosis- and cuproptosis-related genes and lncRNAs were involved in the construction of the gastric cancer prognostic prediction model. We have identified 12 lncRNA prognostic signatures for gastric cancer outcome status evaluation. ROC curve analysis shows the AUC value for this model reached 0.766 in our study, which is higher than other published signatures. The model by Pan et al. (2021) model showed a high AUC value of 0.751. Chen et al. (2021) obtained the 0.736 AUC value using autophagy-related genes. In addition to this, the AUC of the model by Jiang et al. (2021) was 0.654 at 1 year, 0.657 at 3 years, and 0.733 at 5 years. In addition, the AUC values for much more related works were no more than 0.7 (Hu et al., 2019; Yu et al., 2019; Qi et al., 2020). Therefore, these results indicate that the 12 metal-dependent programmed cell death lncRNA signatures constructed in our study improved the prognostic evaluation effectiveness for gastric cancer.
Antitumor drug sensitivities would also determine the outcome of gastric cancer. For instance, as reported, the small molecular targeted drug CP724714 may decrease the IC50 and drug resistance index of gastric cancer to cisplatin (Huang et al., 2016). Dasatinib is an efficient gastric cancer inhibitor, which targets SRC family kinases (Montenegro et al., 2020). EX-527 is a specific inhibitor to SIRT1, which would reintroduce the chemotherapeutic sensitivity (Zhu et al., 2012). FH535 combined with taxol can enhance the invasion inhibitory effect on gastric cancer (Liu et al., 2018). Lapatinib is a kind of tyrosine kinase inhibitor that significantly decreases cell viability and migration of gastric cancer cells, which can also induce cell apoptosis suffering G0/G1 arrest (Yi et al., 2021). R8-modified vinorelbine combined with schisandrin B liposomes can significantly inhibit gastric cancer metastasis by downregulating VEGF, VE-Cad, HIF-1a, PI3K, MMP-2, and FAK (Li et al., 2021). Sunitinib is a multitargeted tyrosine kinase inhibitor, which can enhance the cytotoxicity of vincristine, adriamycin, and cisplatin on multidrug resistant gastric cancer cells (Zhang and Wang, 2013; Hojo et al., 2022). Saracatinib is an Src inhibitor that suppresses gastric cancer invasion and migration, which can also enhance the antitumor effects of lapatinib (Bertotti et al., 2010; Nam et al., 2013; Yamaguchi et al., 2014). Saracatinib also has a synergistic effect with trastuzumab on antigastric cancer (Han et al., 2014). Moreover, OSI-027 can enhance oxaliplatin-induced cell apoptosis and inhibit multidrug resistance to gastric cancer (Xu et al., 2021). In our study, we predicted IC50 for dasatinib, lapatinib, sunitinib, cytarabine, and saracatinib were negatively correlated with risk scores. These results demonstrated that these antitumor drugs would be more sensitive in the high-risk group, which may be more suitable for clinical usage toward patients in the high-risk group. Meanwhile, IC50 for vinorelbine, OSI-027, CP724714, EX-527, and FH535 was positively correlated with risk scores, which demonstrated these drugs may be unsuitable.
Some studies have shown that Chinese patent medicines and plant extracts can also induce apoptosis through ferroptosis and cuproptosis. Liu et al. (2022) found that disulfiram (DSF)/Cu elevated the generation of reactive oxygen species (ROS), and apoptosis was induced in a ROS-dependent manner. Song et al. (2022) showed that Yiqi Huayu decoction can induce ferroptosis in GC by affecting the JAK2-STAT3 pathway and the expression of ACSL4. Guan et al. (2020) found that tanshinone IIA could suppress the proliferation of gastric cancer via inducing p53 upregulation-mediated ferroptosis. Other studies have found that salidroside can inhibit the growth of gastric cancer and induce apoptosis through the PI3K/Akt/mTOR pathway (Rong et al., 2020). Therefore, traditional Chinese medicine and plant extracts also play an important role in the treatment of gastric cancer. Some studies have also shown that TCM extracts can fight cancer by promoting programmed cell apoptosis. Tanshinone IIA (Tan-IIA) was extracted from Danshen (Salviae miltiorrhizae Radix). Su (2018) found that Tan-IIA inhibited human gastric cancer AGS cells; one of the molecular mechanisms may be through decreasing the protein expression of VEGFR and HER2, then blocking the Ras/Raf/MEK/ERK pathway to induce the activation of PARP and caspase-3 to induce apoptosis. ’It is worth mentioning that Ni et al. (2022) and Guan et al. (2020) pointed out that Tanshinone IIA inhibited the stemness of gastric cancer cells partly by inducing ferroptosis. Chen et al. (2017) showed that the tuber of Amorphophallus konjac could increase cell apoptosis and induce cell cycle arrest. TuAKe could also promote autophagy. Moreover, toosendanin (TSN) is a triterpenoid derived from the bark of Melia toosendanin Sieb. Zhou et al. (2018) found that TSN suppressed cell viability, inhibited cell proliferation by causing G1/S arrest, and induced caspase-dependent apoptosis in AGS and HGC-27 cells. In addition, macrocalyxin C is a Chinese herb-derived diterpenoid compound that has been postulated to possess anticancer characteristics. Dang et al. (2020) showed that macrocalyxin C may halt the proliferation of gastric malignancies through alteration of cell invasion, apoptosis, progression through the cell cycle, and cell growth.
All in all, we screened differentially expressed metal-dependent programmed cell death-related lncRNAs and constructed a 12-lncRNA prognostic prediction model for gastric cancer, the prognostic power of which was better than that of other models. We observed that tumor mutation burden and immunotherapy effect have no difference between the high-risk and low-risk groups. Also, antitumor drug sensitivity analysis results may also be helpful for individualized chemotherapy regimen design.
Data availability statement
The datasets presented in this study can be found in online repositories. The names of the repository/repositories and accession number(s) can be found in the article/Supplementary Material.
Author contributions
ZL designed this work. XS collected the data and built the gastric cancer prognostic prediction model. LH performed the GO and KEGG, analyses and GSEA. XS, LH, and YZ evaluated the prediction abilities. QG performed the drug sensitivity prediction. XS prepared the manuscript, and all authors have verified the final version of the manuscript.
Funding
This work was supported by the National Natural Science Foundation of China (grant no. 82072206).
Acknowledgments
The authors thank all editors and reviewers for their efforts.
Conflict of interest
The authors declare that the research was conducted in the absence of any commercial or financial relationships that could be construed as a potential conflict of interest.
Publisher’s note
All claims expressed in this article are solely those of the authors and do not necessarily represent those of their affiliated organizations, or those of the publisher, the editors, and the reviewers. Any product that may be evaluated in this article, or claim that may be made by its manufacturer, is not guaranteed or endorsed by the publisher.
Supplementary material
The Supplementary Material for this article can be found online at: https://www.frontiersin.org/articles/10.3389/fphar.2022.1039499/full#supplementary-material
Supplementary Figure S1 | Flowchart for this work.
Supplementary Figure S2 | Forest plot for the prognostic values of the metal-dependent programmed cell death-related lncRNA signatures through univariate Cox regression analysis.
Supplementary Figure S3 | Correlation coefficients between IC50 and risk scores for other antitumor drugs.
Supplementary Figure S4 | Difference of IC50 between high-risk and low-risk groups for other antitumor drugs.
References
Bertotti, A., Bracco, C., Girolami, F., Torti, D., Gastaldi, S., Galimi, F., et al. (2010). Inhibition of Src impairs the growth of met-addicted gastric tumors. Clin. Cancer Res. 16 (15), 3933–3943. doi:10.1158/1078-0432.CCR-10-0106
Blange, D., Stroes, C. I., Derks, S., Bijlsma, M. F., and van Laarhoven, H. W. M. (2022). Resistance mechanisms to HER2-targeted therapy in gastroesophageal adenocarcinoma: A systematic review. Cancer Treat. Rev. 108, 102418. doi:10.1016/j.ctrv.2022.102418
Chen, X., Yuan, L. Q., Li, L. J., Lv, Y., Chen, P. F., and Pan, L. (2017). Suppression of gastric cancer by extract from the tuber of amorphophallus konjac via induction of apoptosis and autophagy. Oncol. Rep. 38 (2), 1051–1058. doi:10.3892/or.2017.5747
Chen, L., Ma, G., Wang, P., Dong, Y., Liu, Y., Zhao, Z., et al. (2021). Establishment and verification of prognostic model for gastric cancer based on autophagy-related genes. Am. J. Cancer Res. 11 (4), 1335–1346.
Chen, C., Chen, Y., Jin, X., Ding, Y., Jiang, J., Wang, H., et al. (2022). Identification of tumor mutation burden, microsatellite instability, and somatic copy number alteration derived nine gene signatures to predict clinical outcomes in STAD. Front. Mol. Biosci. 9, 793403. doi:10.3389/fmolb.2022.793403
Cobine, P. A., and Brady, D. C. (2022). Cuproptosis: Cellular and molecular mechanisms underlying copper-induced cell death. Mol. Cell 82 (10), 1786–1787. doi:10.1016/j.molcel.2022.05.001
Dang, Y., Liu, T., Yan, J., Reinhardt, J. D., Yin, C., Ye, F., et al. (2020). Gastric cancer proliferation and invasion is reduced by macrocalyxin C via activation of the miR-212-3p/Sox6 Pathway. Cell. Signal. 66, 109430. doi:10.1016/j.cellsig.2019.109430
Dixon, S. J., Lemberg, K. M., Lamprecht, M. R., Skouta, R., Zaitsev, E. M., Gleason, C. E., et al. (2012). Ferroptosis: an iron-dependent form of nonapoptotic cell death. Cell 149 (5), 1060–1072. doi:10.1016/j.cell.2012.03.042
Doll, S., Proneth, B., Tyurina, Y. Y., Panzilius, E., Kobayashi, S., Ingold, I., et al. (2017). ACSL4 dictates ferroptosis sensitivity by shaping cellular lipid composition. Nat. Chem. Biol. 13 (1), 91–98. doi:10.1038/nchembio.2239
Ferlay, J., Colombet, M., Soerjomataram, I., Mathers, C., Parkin, D. M., Pineros, M., et al. (2019). Estimating the global cancer incidence and mortality in 2018: GLOBOCAN sources and methods. Int. J. Cancer 144 (8), 1941–1953. doi:10.1002/ijc.31937
Frankish, A., Diekhans, M., Ferreira, A. M., Johnson, R., Jungreis, I., Loveland, J., et al. (2019). GENCODE reference annotation for the human and mouse genomes. Nucleic Acids Res. 47 (D1), D766. doi:10.1093/nar/gky955
Geeleher, P., Cox, N., and Huang, R. S. (2014). pRRophetic: an R package for prediction of clinical chemotherapeutic response from tumor gene expression levels. PLoS One 9 (9), e107468. doi:10.1371/journal.pone.0107468
Guan, Z., Chen, J., Li, X., and Dong, N. (2020). Tanshinone IIA induces ferroptosis in gastric cancer cells through p53-mediated SLC7A11 down-regulation. Biosci. Rep. 40 (8), BSR20201807. doi:10.1042/BSR20201807
Han, S., Meng, Y., Tong, Q., Li, G., Zhang, X., Chen, Y., et al. (2014). The ErbB2-targeting antibody trastuzumab and the small-molecule SRC inhibitor saracatinib synergistically inhibit ErbB2-overexpressing gastric cancer. MAbs 6 (2), 403–408. doi:10.4161/mabs.27443
Hojo, Y., Kishi, S., Mori, S., Fujiwara-Tani, R., Sasaki, T., Fujii, K., et al. (2022). Sunitinib and pterostilbene combination treatment exerts antitumor effects in gastric cancer via suppression of PDZD8. Int. J. Mol. Sci. 23 (7), 4002. doi:10.3390/ijms23074002
Hu, B. L., Xie, M. Z., Li, K. Z., Li, J. L., Gui, Y. C., and Xu, J. W. (2019). Genome-wide analysis to identify a novel distant metastasis-related gene signature predicting survival in patients with gastric cancer. Biomed. Pharmacother. 117, 109159. doi:10.1016/j.biopha.2019.109159
Huang, D., Duan, H., Huang, H., Tong, X., Han, Y., Ru, G., et al. (2016). Cisplatin resistance in gastric cancer cells is associated with HER2 upregulation-induced epithelial-mesenchymal transition. Sci. Rep. 6, 20502. doi:10.1038/srep20502
Hutter, C., and Zenklusen, J. C. (2018). The cancer genome Atlas: Creating lasting value beyond its data. Cell 173 (2), 283–285. doi:10.1016/j.cell.2018.03.042
Jiang, X., Yan, Q., Xie, L., Xu, S., Jiang, K., Huang, J., et al. (2021). Construction and validation of a ferroptosis-related prognostic model for gastric cancer. J. Oncol. 2021, 6635526. doi:10.1155/2021/6635526
Lee, K. W., Van Cutsem, E., Bang, Y. J., Fuchs, C. S., Kudaba, I., Garrido, M., et al. (2022). Association of tumor mutational burden with efficacy of Pembrolizumab{plus minus}Chemotherapy as first-line therapy for gastric cancer in the phase III KEYNOTE-062 study. Clin. Cancer Res. 28, 3489. doi:10.1158/1078-0432.CCR-22-0121
Li, J., Cao, F., Yin, H. L., Huang, Z. J., Lin, Z. T., Mao, N., et al. (2020). Ferroptosis: Past, present and future. Cell Death Dis. 11 (2), 88. doi:10.1038/s41419-020-2298-2
Li, X. Y., Shi, L. X., Yao, X. M., Jing, M., Li, Q. Q., Wang, Y. L., et al. (2021). Functional vinorelbine plus schisandrin B liposomes destroying tumor metastasis in treatment of gastric cancer. Drug Dev. Ind. Pharm. 47 (1), 100–112. doi:10.1080/03639045.2020.1862169
Li, S. R., Bu, L. L., and Cai, L. (2022). Cuproptosis: lipoylated TCA cycle proteins-mediated novel cell death pathway. Signal Transduct. Target. Ther. 7 (1), 158. doi:10.1038/s41392-022-01014-x
Liu, X., Du, P., Han, L., Zhang, A., Jiang, K., and Zhang, Q. (2018). Effects of miR-200a and FH535 combined with taxol on proliferation and invasion of gastric cancer. Pathol. Res. Pract. 214 (3), 442–449. doi:10.1016/j.prp.2017.12.004
Liu, Y., Guan, X., Wang, M., Wang, N., Chen, Y., Li, B., et al. (2022). Disulfiram/Copper induces antitumor activity against gastric cancer via the ROS/MAPK and NPL4 pathways. Bioengineered 13 (3), 6579–6589. doi:10.1080/21655979.2022.2038434
Mao, C., Wang, X., Liu, Y., Wang, M., Yan, B., Jiang, Y., et al. (2018). A G3BP1-interacting lncRNA promotes ferroptosis and apoptosis in cancer via nuclear sequestration of p53. Cancer Res. 78 (13), 3484–3496. doi:10.1158/0008-5472.CAN-17-3454
Montenegro, R. C., Howarth, A., Ceroni, A., Fedele, V., Farran, B., Mesquita, F. P., et al. (2020). Identification of molecular targets for the targeted treatment of gastric cancer using dasatinib. Oncotarget 11 (5), 535–549. doi:10.18632/oncotarget.27462
Nam, H. J., Im, S. A., Oh, D. Y., Elvin, P., Kim, H. P., Yoon, Y. K., et al. (2013). Antitumor activity of saracatinib (AZD0530), a c-Src/Abl kinase inhibitor, alone or in combination with chemotherapeutic agents in gastric cancer. Mol. Cancer Ther. 12 (1), 16–26. doi:10.1158/1535-7163.MCT-12-0109
Ni, H., Ruan, G., Sun, C., Yang, X., Miao, Z., Li, J., et al. (2022). Tanshinone IIA inhibits gastric cancer cell stemness through inducing ferroptosis. Environ. Toxicol. 37 (2), 192–200. doi:10.1002/tox.23388
Pan, J., Zhang, X., Fang, X., and Xin, Z. (2021). Construction on of a ferroptosis-related lncRNA-based model to improve the prognostic evaluation of gastric cancer patients based on bioinformatics. Front. Genet. 12, 739470. doi:10.3389/fgene.2021.739470
Qi, M., Yu, B., Yu, H., and Li, F. (2020). Integrated analysis of a ceRNA network reveals potential prognostic lncRNAs in gastric cancer. Cancer Med. 9 (5), 1798–1817. doi:10.1002/cam4.2760
Ransohoff, J. D., Wei, Y., and Khavari, P. A. (2018). The functions and unique features of long intergenic non-coding RNA. Nat. Rev. Mol. Cell Biol. 19 (3), 143–157. doi:10.1038/nrm.2017.104
Roemhild, K., von Maltzahn, F., Weiskirchen, R., Knuchel, R., von Stillfried, S., and Lammers, T. (2021). Iron metabolism: Pathophysiology and pharmacology. Trends Pharmacol. Sci. 42 (8), 640–656. doi:10.1016/j.tips.2021.05.001
Rong, L., Li, Z., Leng, X., Li, H., Ma, Y., Chen, Y., et al. (2020). Salidroside induces apoptosis and protective autophagy in human gastric cancer AGS cells through the PI3K/Akt/mTOR pathway. Biomed. Pharmacother. 122, 109726. doi:10.1016/j.biopha.2019.109726
Song, S., Wen, F., Gu, S., Gu, P., Huang, W., Ruan, S., et al. (2022). Network pharmacology study and experimental validation of Yiqi Huayu decoction inducing ferroptosis in gastric cancer. Front. Oncol. 12, 820059. doi:10.3389/fonc.2022.820059
Statello, L., Guo, C. J., Chen, L. L., and Huarte, M. (2021). Gene regulation by long non-coding RNAs and its biological functions. Nat. Rev. Mol. Cell Biol. 22 (2), 96–118. doi:10.1038/s41580-020-00315-9
Su, C. C. (2018). Tanshinone IIA inhibits gastric carcinoma AGS cells by decreasing the protein expression of VEGFR and blocking Ras/Raf/MEK/ERK pathway. Int. J. Mol. Med. 41 (4), 2389–2396. doi:10.3892/ijmm.2018.3407
Subramanian, A., Tamayo, P., Mootha, V. K., Mukherjee, S., Ebert, B. L., Gillette, M. A., et al. (2005). Gene set enrichment analysis: a knowledge-based approach for interpreting genome-wide expression profiles. Proc. Natl. Acad. Sci. U. S. A. 102 (43), 15545–15550. doi:10.1073/pnas.0506580102
Sung, H., Ferlay, J., Siegel, R. L., Laversanne, M., Soerjomataram, I., Jemal, A., et al. (2021). Global cancer statistics 2020: GLOBOCAN estimates of incidence and mortality worldwide for 36 cancers in 185 countries. Ca. Cancer J. Clin. 71 (3), 209–249. doi:10.3322/caac.21660
Tan, Z. (2019). Recent advances in the surgical treatment of advanced gastric cancer: A review. Med. Sci. Monit. 25, 3537–3541. doi:10.12659/MSM.916475
Tang, D., Kang, R., Berghe, T. V., Vandenabeele, P., and Kroemer, G. (2019). The molecular machinery of regulated cell death. Cell Res. 29 (5), 347–364. doi:10.1038/s41422-019-0164-5
Tang, D., Chen, X., and Kroemer, G. (2022). Cuproptosis: a copper-triggered modality of mitochondrial cell death. Cell Res. 32 (5), 417–418. doi:10.1038/s41422-022-00653-7
Wang, M., Mao, C., Ouyang, L., Liu, Y., Lai, W., Liu, N., et al. (2019). Long noncoding RNA LINC00336 inhibits ferroptosis in lung cancer by functioning as a competing endogenous RNA. Cell Death Differ. 26 (11), 2329–2343. doi:10.1038/s41418-019-0304-y
Wang, X., Zhang, J., Cao, G., Hua, J., Shan, G., and Lin, W. (2022). Emerging roles of circular RNAs in gastric cancer metastasis and drug resistance. J. Exp. Clin. Cancer Res. 41 (1), 218. doi:10.1186/s13046-022-02432-z
Wang, Y., Zhang, L., and Zhou, F. (2022). Cuproptosis: a new form of programmed cell death. Cell. Mol. Immunol. 19 (8), 867–868. doi:10.1038/s41423-022-00866-1
Wu, Y., Zhang, S., Gong, X., Tam, S., Xiao, D., Liu, S., et al. (2020). The epigenetic regulators and metabolic changes in ferroptosis-associated cancer progression. Mol. Cancer 19 (1), 39. doi:10.1186/s12943-020-01157-x
Xie, Y., Hou, W., Song, X., Yu, Y., Huang, J., Sun, X., et al. (2016). Ferroptosis: Process and function. Cell Death Differ. 23 (3), 369–379. doi:10.1038/cdd.2015.158
Xie, Y., Zhu, S., Song, X., Sun, X., Fan, Y., Liu, J., et al. (2017). The tumor suppressor p53 limits ferroptosis by blocking DPP4 activity. Cell Rep. 20 (7), 1692–1704. doi:10.1016/j.celrep.2017.07.055
Xu, E., Zhu, H., Wang, F., Miao, J., Du, S., Zheng, C., et al. (2021). OSI-027 alleviates oxaliplatin chemoresistance in gastric cancer cells by suppressing P-gp induction. Curr. Mol. Med. 21 (10), 922–930. doi:10.2174/1566524020666201120113538
Yamaguchi, H., Takanashi, M., Yoshida, N., Ito, Y., Kamata, R., Fukami, K., et al. (2014). Saracatinib impairs the peritoneal dissemination of diffuse-type gastric carcinoma cells resistant to Met and fibroblast growth factor receptor inhibitors. Cancer Sci. 105 (5), 528–536. doi:10.1111/cas.12387
Yi, H., Li, Z., Liu, X., Dai, S., and Li, S. (2021). Therapeutic mechanism of lapatinib combined with sulforaphane on gastric cancer. Evid. Based. Complement. Altern. Med. 2021, 9933274. doi:10.1155/2021/9933274
Yu, C., Hao, X., Zhang, S., Hu, W., Li, J., Sun, J., et al. (2019). Characterization of the prognostic values of the NDRG family in gastric cancer. Ther. Adv. Gastroenterol. 12, 1756284819858507. doi:10.1177/1756284819858507
Zhang, Z., and Kattan, M. W. (2017). Drawing nomograms with R: applications to categorical outcome and survival data. Ann. Transl. Med. 5 (10), 211. doi:10.21037/atm.2017.04.01
Zhang, Y., and Wang, Q. (2013). Sunitinib reverse multidrug resistance in gastric cancer cells by modulating Stat3 and inhibiting P-gp function. Cell biochem. Biophys. 67 (2), 575–581. doi:10.1007/s12013-013-9544-5
Zhang, G., Sun, J., and Zhang, X. (2022). A novel Cuproptosis-related LncRNA signature to predict prognosis in hepatocellular carcinoma. Sci. Rep. 12 (1), 11325. doi:10.1038/s41598-022-15251-1
Zhang, Z., Zeng, X., Wu, Y., Liu, Y., Zhang, X., and Song, Z. (2022). Cuproptosis-related risk score predicts prognosis and characterizes the tumor microenvironment in hepatocellular carcinoma. Front. Immunol. 13, 925618. doi:10.3389/fimmu.2022.925618
Zhao, L., Peng, Y., He, S., Li, R., Wang, Z., Huang, J., et al. (2021). Apatinib induced ferroptosis by lipid peroxidation in gastric cancer. Gastric Cancer 24 (3), 642–654. doi:10.1007/s10120-021-01159-8
Zhong, T., Zhang, W., Guo, H., Pan, X., Chen, X., He, Q., et al. (2022). The regulatory and modulatory roles of TRP family channels in malignant tumors and relevant therapeutic strategies. Acta Pharm. Sin. B 12 (4), 1761–1780. doi:10.1016/j.apsb.2021.11.001
Zhou, N., and Bao, J. (2020). FerrDb: a manually curated resource for regulators and markers of ferroptosis and ferroptosis-disease associations. Database (Oxford) 2020, baaa021. doi:10.1093/database/baaa021
Zhou, Q., Wu, X., Wen, C., Wang, H., Wang, H., Liu, H., et al. (2018). Toosendanin induces caspase-dependent apoptosis through the p38 MAPK pathway in human gastric cancer cells. Biochem. Biophys. Res. Commun. 505 (1), 261–266. doi:10.1016/j.bbrc.2018.09.093
Keywords: gastric cancer, prognostic prediction, natural drug sensitivity, metal-dependent programmed cell death, immune sensitivity
Citation: Song X, Hou L, Zhao Y, Guan Q and Li Z (2022) Metal-dependent programmed cell death-related lncRNA prognostic signatures and natural drug sensitivity prediction for gastric cancer. Front. Pharmacol. 13:1039499. doi: 10.3389/fphar.2022.1039499
Received: 08 September 2022; Accepted: 05 October 2022;
Published: 21 October 2022.
Edited by:
Yulin Dai, Changchun University of Chinese Medicine, ChinaCopyright © 2022 Song, Hou, Zhao, Guan and Li. This is an open-access article distributed under the terms of the Creative Commons Attribution License (CC BY). The use, distribution or reproduction in other forums is permitted, provided the original author(s) and the copyright owner(s) are credited and that the original publication in this journal is cited, in accordance with accepted academic practice. No use, distribution or reproduction is permitted which does not comply with these terms.
*Correspondence: Zhiwen Li, lizhiwen@jlu.edu.cn