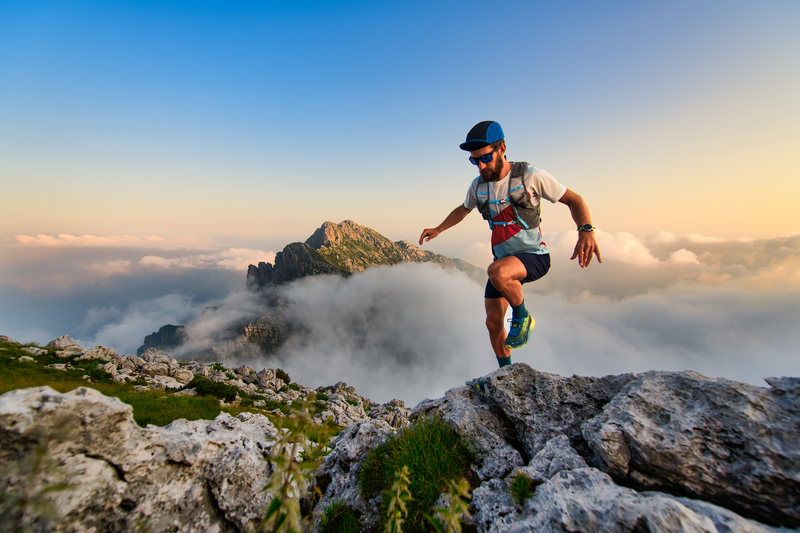
94% of researchers rate our articles as excellent or good
Learn more about the work of our research integrity team to safeguard the quality of each article we publish.
Find out more
ORIGINAL RESEARCH article
Front. Pediatr. , 24 August 2023
Sec. Neonatology
Volume 11 - 2023 | https://doi.org/10.3389/fped.2023.1252875
This article is part of the Research Topic Understanding Retinopathy of Prematurity: Update on Clinic and Pathogenesis View all 6 articles
Purpose: The purpose of this study was to investigate the quantitative retinal vascular morphological characteristics of Retinopathy of Prematurity (ROP) and Familial Exudative Vitreoretinopathy (FEVR) in the newborn by the application of a deep learning network with artificial intelligence.
Methods: Standard 130-degree fundus photographs centered on the optic disc were taken in the newborns. The deep learning network provided segmentation of the retinal vessels and the optic disc (OD). Based on the vessel segmentation, the vascular morphological characteristics, including avascular area, vessel angle, vessel density, fractal dimension (FD), and tortuosity, were automatically evaluated.
Results: 201 eyes of FEVR, 289 eyes of ROP, and 195 eyes of healthy individuals were included in this study. The deep learning system of blood vessel segmentation had a sensitivity of 72% and a specificity of 99%. The vessel angle in the FEVR group was significantly smaller than that in the normal group and ROP group (37.43 ± 5.43 vs. 39.40 ± 5.61, 39.50 ± 5.58, P = 0.001, < 0.001 respectively). The normal group had the lowest vessel density, the ROP group was in between, and the FEVR group had the highest (2.64 ± 0.85, 2.97 ± 0.92, 3.37 ± 0.88 respectively). The FD was smaller in controls than in the FEVR and ROP groups (0.984 ± 0.039, 1.018 ± 0.039 and 1.016 ± 0.044 respectively, P < 0.001). The ROP group had the most tortuous vessels, while the FEVR group had the stiffest vessels, the controls were in the middle (11.61 ± 3.17, 8.37 ± 2.33 and 7.72 ± 1.57 respectively, P < 0.001).
Conclusions: The deep learning technology used in this study has good performance in the quantitative analysis of vascular morphological characteristics in fundus photography. Vascular morphology was different in the newborns of FEVR and ROP compared to healthy individuals, which showed great clinical value for the differential diagnosis of ROP and FEVR.
Congenital eye diseases are the leading cause of vision loss and blindness in infants and young children, which could cause heavy social and economic burdens worldwide (1). Congenital retinal diseases in infants include retinopathy of prematurity (ROP), familial exudative retinopathy (FEVR), retinoblastoma, and other developmental abnormalities.
With the increasing survival rates of very preterm and very low birth weight infants, the prevalence and severity of ROP are also increasing. FEVR is an inherited retinal vascular development disease, which presented resembling ROP (2). Unlike other dysplastic diseases, infantile retinal diseases are difficult to recognize with the naked eye and often progress to an advanced stage before being detected. Since the first 4–6 months period is crucial for the development of neonatal eyes, most of the affected infants would miss the best time for treatment. Therefore, early screening and diagnosis play the key role to preventing vision loss and blindness in infants and young children. The American Academy of Pediatrics (AAP) recommended screening for all infants born before 30 weeks gestational age or with a birth weight less than 1,500 g, as well as infants with additional risk factors (3). In addition, other than screening for prematurity, screening for full-term infants is equally important.
However, the temporal periphery vascular pattern in ROP and FEVR is remarkably similar (4). In clinical practice, the differential diagnosis is commonly based on medical history and fluorescein angiography (FA) (4, 5). In the past decade, FA in infants has been increasingly utilized to visualize the infant's retina without the need for anesthesia and intravenous injections. Through FA, ROP and FEVR lesions that are difficult to distinguish on color fundus photography and indirect ophthalmoscopy could be differentiated, as the former showing discrete and homogeneous vascular-avascular junctions, while the latter typically shows bulbous vascular terminals, abnormal branching patterns, and venous-venous shunting (6). Although FA for infants has been proven to be safe and effective, it remains challenging in terms of the shortage of pediatric ophthalmologists and the difficulties of nursing infants (7).
In recent years, the development of artificial intelligence has grown rapidly with the development of computer science as well as high-performance graphics processors. Artificial intelligence (AI) is a branch of computer science that refers to the use of computers to simulate human intelligence with only minimal human intervention (8). To date, most studies have focused on computer-based systems for the identification and diagnosis of plus disease in ROP. In 2016, Worrall et al. (9) first used Convolutional Neural Networks (CNNs) that can automatically analyze image features for automatic plus disease diagnosis. Our study applied CNN and deep learning to fundus images of preterm infants for automatic plus disease diagnosis and achieved a sensitivity of 95.1% and a specificity of 97.8% (10). Ye et al. (11) proposed an automated diagnostic system for FEVR that combines deep learning and available clinical evidence. The system focused on the evaluation of retinal vascular features in Ultra-wide-field fundus images to diagnose FEVR with quantitative measurement, enabling accurate and non-invasive diagnosis, including early stages of FEVR.
Therefore, the purpose of this study was to quantitatively analyze the vascular morphological characteristics of infants with ROP and FEVR using an artificial intelligence approach, hoping to provide insights into the differential diagnosis and early intervention of these diseases.
This study adhered to the Declaration of Helsinki and received approval by the ethics review board of Zhejiang Provincial People's Hospital. All the infants presented to the ophthalmology clinic from March 2022 to January 2023 were reviewed, and infants with a clinical diagnosis of ROP (289 eyes) or FEVR (201 eyes) were included in this study. Healthy full-term infants (195 eyes) were also included as a control group.
Exclusion criteria were vitreous hemorrhage, history of retinal laser photocoagulation or vitreous injection, or any opacity of the refractive media that may affect the image quality.
All the infants underwent standard contact wide-angle fundus imaging after pupil dilatation. The collected image size was 1,600⊆1,200 pixels with a 130-degree fundus angle. Posterior fundus images centered on the optic disc were collected to avoid bias caused by different capture perspectives.
The flowchart shown in Figure 1 illustrates the data processing steps used in our study. The specific method of constructing the optic disc and vascular segmentation network is detailed in our previous study (10). First, the retinal images were subjected to optic disc segmentation using a U-Net based network (12). The optic disc center was then calculated based on the segmentation result, and the minimum enclosing circle of the optic disc was fitted. A circle is then drawn with the image center as the center and one-quarter of the image width as the radius, and the optic disc center was checked whether it falls within this circle. The images with the optic disc center located within this circle were selected for blood vessel segmentation based on a U-Net. The concentric ring region of interest (ROI) was defined with the optic disc center as the center and 2–4 times the minimum enclosing circle radius of the optic disc as the radius, in which the vascular analysis is performed, including measurements of blood vessel angle, density, tortuosity, and fractal dimension.
The vessel angle refers to the angle formed between two blood vessels that arise from a bifurcation. We used the Baker et al. (13) definition to evaluate the vessel angle, specifically computing the vessel angle at the branching points. Retinal blood vessel density was calculated as the ratio of vessel area to the area of the fundus image. The fractal dimension serves as a comprehensive measure of the intricate branching pattern exhibited by the retinal vascular tree (14). Higher values of the fractal dimension indicate a more intricate branching pattern. To evaluate the fractal dimension of the fundus vessels, we utilized the box-counting method proposed by Mainster & Martin (15) and Stosic & Stosic (16). This method allowed us to quantify the fractal dimension by analyzing the distribution of vessel segments within the retinal images. Tortuosity is a parameter employed to assess the extent of curvature exhibited by blood vessels. Greater tortuosity values indicate a higher degree of curvature in the blood vessels. Various definitions of tortuosity have been proposed (17, 18). In our study, we adopted the measurement of tortuosity known as arc length-normalized total squared curvature, initially introduced by Hart et al. (17), which was subsequently refined by Turior et al. (19). This approach allows for a more accurate evaluation of tortuosity by considering the normalized curvature of the vessel segments along their arc length.
Statistical analysis was performed using SPSS software (version 26.0, SPSS Inc, Chicago, IL, USA). All data were presented in the form of means ± standard deviations. The vascular morphological characteristics were compared between the groups using the χ2 test or one-way analysis of variance. P-values <0.05 were considered statistically significant.
The segmentation results of the optic disc and retinal blood vessels for the three groups are shown in Figure 2. The sensitivity and specificity for vascular segmentation were 0.72 and 0.99, respectively. For optic disc segmentation, the sensitivity was 0.94 and the specificity was 0.99.
Figure 2. Representative images of original, optic disc segmented and retinal blood vessels for the healthy, ROP and FEVR eyes. OD, optic disc; ROP, retinopathy of prematurity; FEVR, familial exudative vitreoretinopathy.
The difference in vessel angle was statistically significant between the three groups (P < 0.001). The vessel angle in the FEVR group was significantly smaller than that in the normal group and ROP group (37.43 ± 5.43 vs. 39.40 ± 5.61, 39.50 ± 5.58, P = 0.001, P<0.001 respectively). The vessel angle had no significant differences between ROP and the normal group (P = 0.980).
The difference in vessel density among the three groups was statistically significant, P < 0.001. The normal group had the lowest vessel density, the ROP group was in between, and the FEVR group had the highest (2.64 ± 0.85, 2.97 ± 0.92, 3.37 ± 0.88 respectively). The differences between the groups were all significantly different (all P < 0.001).
Fractal dimension was statistically different among the three groups, P < 0.001. The fractal dimension of the normal group was smaller than that of the ROP and FEVR groups (0.984 ± 0.039, 1.018 ± 0.039, 1.016 ± 0.044 respectively, both P < 0.001).
Among the three groups, the largest tortuosity appeared in the ROP group, while the FEVR group had the smallest value of tortuosity (11.61 ± 3.17, 8.37 ± 2.33, 7.72 ± 1.57 respectively, P < 0.001). Vascular morphologic parameters and intragroup comparisons among the three groups were shown in Table 1 and Figure 3, respectively.
Figure 3. Differences in morphological characteristics among healthy, ROP and FEVR groups. ROP, retinopathy of prematurity; FEVR, familial exudative vitreoretinopathy. *P < 0.05, **P < 0.01, ***P < 0.001.
In the present study, we used a deep learning system to automatically quantify and analyze the posterior retinal vascular morphology in the eyes of ROP, FEVR, and full-term newborns. We demonstrated that vessel morphological parameters (including vessel angle, vessel density, fractal dimension, and vessel tortuosity) were significantly different between the three groups of infants. We showed that ROP and FEVR are significantly different in vascular morphology and could be identified by artificial intelligence systems.
In the current study, a reduced vascular angle was found in the eyes of patients with FEVR compared to those with ROP and healthy full-term infants. We suggested that the decrease in vessel angle was possibly derived from the increased traction on the vitreoretinal interface. Lee et al. (20) analyzed the images on handheld OCT of young children with FEVR and revealed prominent retinal displacement and traction. Contraction of the strongly adherent vitreous further provoked the characteristic macular ectopia and retinal traction complications, especially seen in young patients with FEVR. In addition, Wang et al. (21) suggested that Ultra-Wide-Field OCTA could contribute to the detection of abnormalities at the temporal mid-peripheral vitreoretinal interface of patients with early stages of FEVR, which resulted in superficial retinal vascular straightening and a reduction in vessel angle. In agreement with our study results, Shao et al. (22) demonstrated a reduced angle of retinal arteries in FEVR patients in ultra-wide-field fundus imaging.
In the previous study by our team (10), it was demonstrated that due to the dilated and tortuous nature of the ROP retinal vessels, the vascular density increased with increasing severity of the plus disease. However, studies using OCTA revealed a reduction in both superficial and deep macular capillary vessel density in the eyes of patients with FEVR (23, 24). We speculated that this difference stems from the difference in measurement method and the age of the subjects.
The fractal dimension numerically measures the subtle distortions of tissue structure in pathological states and has become one of the new biomarkers in the field of neuroscience (25). In the field of ophthalmology, the fractal dimension has been used to detect early diabetic retinopathy and other retinal diseases (26). In addition, the fractal dimension has been proven to increase with the severity of diabetic retinopathy (27). Our study used the fractal dimension to quantify the complexity of retinal blood vessels in fundus imaging in infants. The results indicated that retinal vascular complexity was elevated in both FEVR and ROP infants compared to healthy full-term infants.
Traction on the temporal side of the retina in infants with FEVR causes straightening of the vessels. Hsu et al. observed straightening of the macular vasculature in OCTA imaging of patients with FEVR (24). Chen et al. (28) investigated fundus imaging in patients with asymptomatic mild FEVR and found increased vascular branching and straightening of peripheral vascular branches in 98.4% of eyes, which was also consistent with the results of our previous study (11). Vascular endothelial growth factor (VEGF) is the primary mediator of pathological angiogenesis in ROP (29). A study by Liang et al. found that elevated aqueous VEGF levels were positively correlated with ROP severity and venous tortuosity in zone Ⅰ (29). It has also been proposed that the mean and highest vascular tortuosity of fundus images of infants would be used to predict the need for treatment of ROP with a high level of sensitivity and specificity (30). Furthermore, Chen et al. suggested that vascular tortuosity following intravitreal injection of anti-VEGF for ROP is indicative of potential ROP lesion reactivation (31). Therefore, we suggested that the opposite trend of retinal vascular tortuosity in infants with FEVR and ROP indicates a different pathological process between these two diseases.
This study has several limitations. First, given the retrospective nature of this study, there are inherent limitations. Second, artificial intelligent vessel extraction has limited capability for retinal vessel extraction in severe ROP with FEVR, which may result in some selection bias. In addition, compared to adults, fundus images of infants are more difficult to capture and have poorer quality, especially in terms of low contrast, uneven illumination and thinner vessels, thus the vessel segmentation was not performed as well as in adults. We look forward to future improvements in the algorithm and performance of artificial intelligence vascular extraction for more in-depth and comprehensive analysis.
In summary, the present study applied artificial intelligence for automated vessel extraction and compared the quantified morphological characteristics of the ROP, FEVR, and healthy full-term infants in fundus images. Differences were found between the three groups in vessel angle, vessel density, fractal dimension, and vascular tortuosity. Combined with artificial intelligence's vascular extraction capabilities, these vascular morphological characteristics, which were difficult to identify with the visual eye, would allow pediatric ophthalmologists to differentially diagnose the two diseases.
The raw data supporting the conclusions of this article will be made available by the authors, without undue reservation.
This study adhered to the Declaration of Helsinki and received approval by the ethics review board of Zhejiang Provincial People’s Hospital. The participants’ legal guardian provided written informed consent to participate in this study.
XD, LC, and MS: contributed to conception and design of the study. XD: collected the images and wrote the first draft of the manuscript. KC and YC: analyzed the images and performed the statistical analysis. ZX, SZ, and LS: wrote sections of the manuscript. All authors contributed to the article and approved the submitted version.
This work was financially supported by the Natural Science Foundation of Zhejiang Province (LTGY23H120005).
The authors declare that the research was conducted in the absence of any commercial or financial relationships that could be construed as a potential conflict of interest.
All claims expressed in this article are solely those of the authors and do not necessarily represent those of their affiliated organizations, or those of the publisher, the editors and the reviewers. Any product that may be evaluated in this article, or claim that may be made by its manufacturer, is not guaranteed or endorsed by the publisher.
1. Tang H, Li N, Li Z, Zhang M, Wei M, Huang C, et al. Fundus examination of 199 851 newborns by digital imaging in China: a multicentre cross-sectional study. Br J Ophthalmol. (2018) 102(12):1742–6. doi: 10.1136/bjophthalmol-2018-312366
2. Miyakubo H, Hashimoto K, Miyakubo S. Retinal vascular pattern in familial exudative vitreoretinopathy. Ophthalmology. (1984) 91(12):1524–30. doi: 10.1016/S0161-6420(84)34119-7
3. Fierson WM; American Academy of Pediatrics Section on Ophthalmology; American Academy of Ophthalmology; American Association for Pediatric Ophthalmology and Strabismus; American Association of Certified Orthoptists. Screening examination of premature infants for retinopathy of prematurity. Pediatrics. (2018) 142(6):e20183061. doi: 10.1542/peds.2018-3061
4. John VJ, McClintic JI, Hess DJ, Berrocal AM. Retinopathy of prematurity versus familial exudative vitreoretinopathy: report on clinical and angiographic findings. Ophthalmic Surg Lasers Imaging Retina. (2016) 47(1):14–9. doi: 10.3928/23258160-20151214-02
5. Kartchner JZ, Hartnett ME. Familial exudative vitreoretinopathy presentation as persistent fetal vasculature. Am J Ophthalmol Case Rep. (2017) 6:15–7. doi: 10.1016/j.ajoc.2017.01.001
6. Kashani AH, Brown KT, Chang E, Drenser KA, Capone A, Trese MT. Diversity of retinal vascular anomalies in patients with familial exudative vitreoretinopathy. Ophthalmology. (2014) 121(11):2220–7. doi: 10.1016/j.ophtha.2014.05.029
7. Kvopka M, Chan W, Lake SR, Durkin S, Taranath D. Fundus fluorescein angiography imaging of retinopathy of prematurity in infants: a review. Surv Ophthalmol. (2023) 68(5):S0039625723000735. doi: 10.1016/j.survophthal.2023.05.004
8. Bini SA. Artificial intelligence, machine learning, deep learning, and cognitive computing: what do these terms mean and how will they impact health care? J Arthroplasty. (2018) 33(8):2358–61. doi: 10.1016/j.arth.2018.02.067
9. Worrall DE, Wilson CM, Brostow GJ. Automated retinopathy of prematurity case detection with convolutional neural networks. In: Carneiro G, Mateus D, Peter L, Bradley A, Tavares JMRS, Belagiannis V, editors. Deep learning and data labeling for medical applications. Cham: Springer International Publishing (2016). p. 68–76.
10. Mao J, Luo Y, Liu L, Lao J, Shao Y, Zhang M, et al. Automated diagnosis and quantitative analysis of plus disease in retinopathy of prematurity based on deep convolutional neural networks. Acta Ophthalmol. (2020) 98(3):e339–45. doi: 10.1111/aos.14264
11. Ye Y, Mao J, Liu L, Zhang S, Shen L, Sun M. Automatic diagnosis of familial exudative vitreoretinopathy using a fusion neural network for wide-angle retinal images. IEEE Access. (2020) 8:162–73. doi: 10.1109/ACCESS.2019.2961418
12. Ronneberger O, Fischer P, Brox T. U-Net: convolutional networks for biomedical image segmentation. In: Navab N, Hornegger J, Wells WM, Frangi AF, editors. Medical image computing and computer-assisted intervention – MICCAI 2015. Munich, Germany: Springer International Publishing (2015). p. 234–41. (Lecture Notes in Computer Science; vol. 9351).
13. Baker ML, Hand PJ, Wang JJ, Wong TY. Retinal signs and stroke: revisiting the link between the eye and brain. Stroke. (2008) 39(4):1371–9. doi: 10.1161/STROKEAHA.107.496091
14. Liew G, Wang JJ, Cheung N, Zhang YP, Hsu W, Lee ML, et al. The retinal vasculature as a fractal: methodology, reliability, and relationship to blood pressure. Ophthalmology. (2008) 115(11):1951–6.e1. doi: 10.1016/j.ophtha.2008.05.029
15. Mainster MA. The fractal properties of retinal vessels: embryological and clinical implications. Eye. (1990) 4(1):235–41. doi: 10.1038/eye.1990.33
16. Stosic T, Stosic BD. Multifractal analysis of human retinal vessels. IEEE Trans Med Imaging. (2006) 25(8):1101–7. doi: 10.1109/TMI.2006.879316
17. Hart WE, Goldbaum M, Côté B, Kube P, Nelson MR. Measurement and classification of retinal vascular tortuosity. Int J Med Inf. (1999) 53(2–3):239–52. doi: 10.1016/S1386-5056(98)00163-4
18. Smedby O, Högman N, Nilsson S, Erikson U, Olsson AG, Walldius G. Two-dimensional tortuosity of the superficial femoral artery in early atherosclerosis. J Vasc Res. (1993) 30(4):181–91. doi: 10.1159/000158993
19. Turior R, Onkaew D, Uyyanonvara B, Chutinantvarodom P. Quantification and classification of retinal vessel tortuosity. Scienceasia. (2013) 39265(39):265–77. doi: 10.2306/scienceasia1513-1874.2013.39.265
20. Lee J, El-Dairi MA, Tran-Viet D, Mangalesh S, Dandridge A, Jiramongkolchai K, et al. Longitudinal changes in the optic nerve head and retina over time in very young children with familial exudative vitreoretinopathy. Retina. (2019) 39(1):98–110. doi: 10.1097/IAE.0000000000001930
21. Wang Y, Lai Y, Zhou X, Zhang T, Sun L, Zhang Z, et al. Ultra-widefield optical coherence tomography angiography in mild familial exudative vitreoretinopathy. Retina. (2023) 43(6):932–9. doi: 10.1097/IAE.0000000000003754
22. Shao Y, Mao J, Fang Y, Chen Y, Zhang Z, Xiang Z, et al. The characteristic of optical coherence tomography angiography and retinal arteries angle in familial exudative vitreoretinopathy with inner retinal layer persistence. Curr Eye Res. (2023) 48(9):1–7. doi: 10.1080/02713683.2023.2213867
23. Koulisis N, Moysidis SN, Yonekawa Y, Dai YL, Burkemper B, Wood EH, et al. Correlating changes in the macular microvasculature and capillary network to peripheral vascular pathologic features in familial exudative vitreoretinopathy. Ophthalmology Retina. (2019) 3(7):597–606. doi: 10.1016/j.oret.2019.02.013
24. Hsu ST, Finn AP, Chen X, Ngo HT, House RJ, Toth CA, et al. Macular microvascular findings in familial exudative vitreoretinopathy on optical coherence tomography angiography. Ophthalmic Surg Lasers Imaging Retina. (2019) 50(5):322–9. doi: 10.3928/23258160-20190503-11
25. Ziukelis ET, Mak E, Dounavi ME, Su L, O’Brien JT. Fractal dimension of the brain in neurodegenerative disease and dementia: a systematic review. Ageing Res Rev. (2022) 79:101651. doi: 10.1016/j.arr.2022.101651
26. Yu S, Lakshminarayanan V. Fractal dimension and retinal pathology: a meta-analysis. Appl Sci. (2021) 11(5):2376. doi: 10.3390/app11052376
27. Hashmi S, Lopez J, Chiu B, Sarrafpour S, Gupta A, Young J. Fractal dimension analysis of OCTA images of diabetic retinopathy using circular mass-radius method. Ophthalmic Surg Lasers Imaging Retina. (2021) 52(3):116–22. doi: 10.3928/23258160-20210302-01
28. Chen C, Sun L, Li S, Huang L, Zhang T, Wang Z, et al. The spectrum of genetic mutations in patients with asymptomatic mild familial exudative vitreoretinopathy. Exp Eye Res. (2020) 192:107941. doi: 10.1016/j.exer.2020.107941
29. Chen J, Smith LEH. Retinopathy of prematurity. Angiogenesis. (2007) 10(2):133–40. doi: 10.1007/s10456-007-9066-0
30. Lertjirachai I, Stem MS, Moysidis SN, Koulisis N, Capone A, Drenser KA, et al. Vessel tortuosity cutoff values using the modified ROPtool may predict need for treatment in retinopathy of prematurity. Ophthalmic Surg Lasers Imaging Retina. (2019) 50(4):215–20. doi: 10.3928/23258160-20190401-03
Keywords: deep learning, retinopathy of prematurity, familial exudative vitreoretinopathy, vascular morphology, retina
Citation: Deng X, Chen K, Chen Y, Xiang Z, Zhang S, Shen L, Sun M and Cai L (2023) Vessels characteristics in familial exudative vitreoretinopathy and retinopathy of prematurity based on deep convolutional neural networks. Front. Pediatr. 11:1252875. doi: 10.3389/fped.2023.1252875
Received: 4 July 2023; Accepted: 8 August 2023;
Published: 24 August 2023.
Edited by:
Tang Zhu, Putian University, ChinaReviewed by:
Thiago Gonçalves dos Santos Martins, Federal University of São Paulo, Brazil© 2023 Deng, Chen, Chen, Xiang, Zhang Shen, Sun and Cai. This is an open-access article distributed under the terms of the Creative Commons Attribution License (CC BY). The use, distribution or reproduction in other forums is permitted, provided the original author(s) and the copyright owner(s) are credited and that the original publication in this journal is cited, in accordance with accepted academic practice. No use, distribution or reproduction is permitted which does not comply with these terms.
*Correspondence: Mingzhai Sun bWluZ3poYWlAdXN0Yy5lZHUuY24= Lingzhi Cai MTM0ODYxODQyMjBAMTYzLmNvbQ==
Disclaimer: All claims expressed in this article are solely those of the authors and do not necessarily represent those of their affiliated organizations, or those of the publisher, the editors and the reviewers. Any product that may be evaluated in this article or claim that may be made by its manufacturer is not guaranteed or endorsed by the publisher.
Research integrity at Frontiers
Learn more about the work of our research integrity team to safeguard the quality of each article we publish.