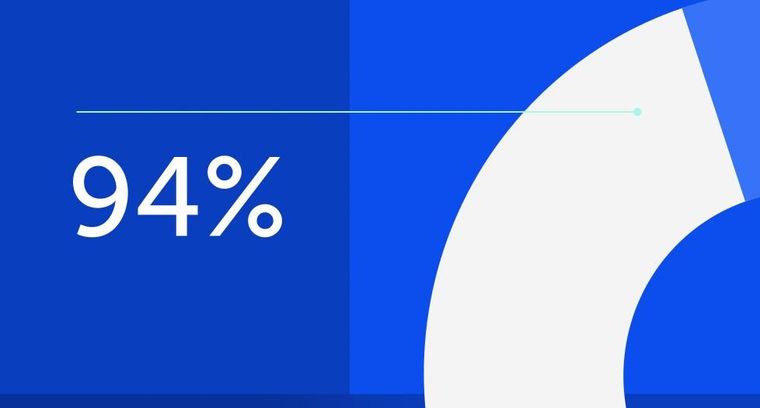
94% of researchers rate our articles as excellent or good
Learn more about the work of our research integrity team to safeguard the quality of each article we publish.
Find out more
ORIGINAL RESEARCH article
Front. Organ. Psychol., 09 April 2025
Sec. Performance and Development
Volume 3 - 2025 | https://doi.org/10.3389/forgp.2025.1500016
Introduction: To create training data for AI systems, it is necessary to manually assign correct labels to a large number of objects; this task is often performed by crowdsourcing. This task is usually divided into a certain number of smaller and more manageable segments, and workers work on them one after the other. In this study, assuming the above task, we investigated whether the deliverable evaluation feedback and provision of additional rewards contribute to the improvement of workers' motivation, that is, the persistence of the tasks and performance.
Method: We conducted a user experiment on a real crowdsourcing service platform. This provided first and second round of tasks, which ask workers input correct labels to a flower species. We developed an experimental system that assessed the work products of the first-round task performed by a worker and presented the results to the worker. Six hundred forty-five workers participated in this experiment. They were divided into high and low performing groups according to their first-round scores (correct answer ratio). The workers' performance and task continuation ratio under the high and low performance group and with and without evaluation feedback and additional rewards were compared.
Results: We found that the presentation of deliverable evaluations increased the task continuation rate of high-quality workers, but did not contribute to an increase in the task performance (correct answer rate) for either type of worker. The providing additional rewards reduced workers' task continuation rate, and the amount of reduction was larger for low-quality workers than that for high-quality workers. However, it largely increased the low-quality worker's task performance. Although not statistically significant, the low-quality worker's task performance of the second round was highest for those who were shown both feedback and additional rewards.
Discussion: It was found that rewards positively affected worker motivation in previous studies. This is inconsistent with the results of our study. One possible reason is that previous studies have examined workers' future engagements on different tasks, whereas our study examined workers' successive tackles on the almost same task. In conclusion, it is better to offer both feedback and additional rewards when the quality of the deliverables is a priority, and to give only feedback when the quantity of deliverables is a priority.
In recent years, crowdsourcing services are being used by companies to diversify work styles and improve production efficiency. In crowdsourcing, workers are recruited via the Internet, which makes recruitment easier than the conventional means, such as flyers and job magazines (Howe, 2006). In addition, workers can work anytime and from anywhere, therefore, they have the flexibility to work during their spare time. However, since the work provider (hereinafter, “crowdsourcer”) cannot directly monitor workers, it is impossible to know whether they are diligently working on their tasks. Therefore, it is necessary for crowdsourcers to devise ways to ensure this. In addition, crowdsourcing services must introduce a system that can guarantee the quality of deliverables (Kashima et al., 2016; Soliman and Tuunainen, 2015).
In particular, when collecting training data for machine learning, it is necessary to have people assign correct labels to the examples such as photos or sounds. For such tasks, it is desirable to have workers work on many tasks while improving the quality of tasks they perform. However, many crowdsourcers cannot ensure that workers work consistently on similar or subsequent tasks after their initial participation (Rockmann and Ballinger, 2017; Yang et al., 2008). If a participant does not work on several microtasks, the effort to break down tasks into microtasks and post them on the crowdsourcing platform will be wasted. Therefore, it is necessary to encourage continuous worker participation, not only to obtain large volumes of participation in similar tasks in the short term but also to maintain the total volume of efforts in the crowdsourcing community in the long term (Sun et al., 2015). Consequently, both academia and industry are looking at ways to encourage workers to continue participating on similar or subsequent tasks (Liu and Liu, 2019; Sun et al., 2012). However, there is a trade-off between the quality and quantity of deliverables. On the one hand, the quantity of deliverables will decrease as the evaluation criteria are raised to ensure reliability of the quality of deliverables. On the other hand, if the deliverables are not evaluated and payment to workers is high, the quantity of deliverables will increase, but their quality will decrease. Therefore, it is an extremely difficult problem to maintain a certain level of quality while ensuring the quantity of deliverables.
General methods to accomplish this include devising the task and its work environment. Specifically, making the task socially meaningful rather than for profit (Rogstadius et al., 2011; Cappa et al., 2019), or making it seem like a game so that workers can enjoy while working (Hong et al., 2013; Goncalves et al., 2014; Feng et al., 2018; Morschheuser et al., 2019; Uhlgren et al., 2024) are some examples. Other possible approaches include eliminating the participation of workers who do not satisfy the necessary conditions before performing the task (Matsubara and Wang, 2014; Allahbakhsh et al., 2013), and eliminating those who complete the task in an extremely short time by measuring the time taken to perform the task (Cosley et al., 2005). Furthermore, it is possible to set effective rewards that lead to incentives for the tasks performed by workers (Watts and Mason, 2009; Ho et al., 2015; Yin et al., 2013; Feng et al., 2018; Cappa et al., 2019), provide evaluation feedback on the deliverables (Feng et al., 2018), and inform workers about the evaluation criteria to encourage them to take their work more seriously (Dow et al., 2012). Another way is to evaluate workers' abilities in advance by testing and distribute tasks according to workers' abilities (Tauchmann et al., 2020).
Some studies have shown that explaining the objectives or social significance of the project improves the worker's motivation. For examples, showing the project's technical features has been found to lead to sustained participation (Jackson et al., 2015), and showing social significance of the task has been found to increase the number of participants (Cappa et al., 2019). The relationship between task instructions and worker participation intentions or motivations has also been investigated. For examples, the type of task instructions (i.e., unbounded, suggestive, and prohibitive) has been found to affect the creativity of participants (Chaffois et al., 2015). Yin et al. (2022) examined how requirement-oriented and reward-oriented strategies in task instructions affect worker participation and found that the usage of restrictive words affect the number of participants.
Some studies have combined the methods described above to increase workers' motivation to engage in tasks. Feng et al. (2018) studied the effects of rewards for deliverables and feedback on motivation to participate in tasks for 295 workers in a crowdsourcing service. Furthermore, they examined whether the four intrinsic motivations (self-presentation, self-efficacy, social bonding, and playfulness) in the crowdsourcing context have a mediating effect on workers' motivation. The results confirmed the mediating effects of intrinsic motivations of self-presentation, self-efficacy, and playfulness on the effect of rewards and feedback on respondents' willingness to participate.
Using a public database of crowdsourcing tasks, Cappa et al. (2019) collected data related to “crowdsourcing invention activities” campaigns conducted between 2007 and 2014. The data were used to investigate the impact of financial rewards and social significance explanation on the number of project participants. Regression analysis revealed that financial rewards had a significant trend on the number of participants, while social significance explanation had a significant impact on the number of participants.
While the aforementioned studies examined whether task devices affect worker motivation, some studies have investigated the psychological elements that constitute worker motivation in crowdsourcing. According to the self-determination theory (SDT), two types of motivation impact human behavior: intrinsic and extrinsic motivation (Ryan and Deci, 2000). Participants of collaborative work are also motivated in two different ways (extrinsic one and intrinsic one; Wasko and Faraj, 2005; Antikainen et al., 2010). Soliman and Tuunainen (2015) investigated the extrinsic and intrinsic motivations that influence the use of crowdsourcing services. It showed that intrinsic motivation consisted of curiosity, enjoyment, and altruism, while extrinsic motivation consisted of financial rewards, skill development, future employment, and publicity. Kaufmann et al. (2011) investigated which of intrinsic and extrinsic motivation was high among crowdsourcing service workers. The survey results showed that immediate rewards, which are extrinsic motivations, were high. Among the intrinsic motivators, enjoyment-based motivation tended to be higher than the others.
Huang et al. (2020) conducted a questionnaire survey on crowdsourcing to investigate whether workers intended to continue working on tasks from the same crowdsourcer. They investigated whether the flexibility and enjoyment of previous tasks, as well as trust in the crowdsourcer, affected the worker's intention to continue working. The results showed that enjoyment of the previous task and trust in the crowdsourcer had positive effects on task continuation.
Thus, studies investigating the factors that correlate with workers' motivation to engage in tasks and task persistence have mainly been conducted using questionnaires. The results of these questionnaire-based studies will be more reliable in terms of causality if they are supported by the results from psychological experiments. Therefore, in this study, we used a psychological experiment to test whether evaluation feedback on deliverables and additional rewards based on it increases workers' persistence in participating in tasks and improves the quality of tasks they perform.
We targeted tasks that require a large number of identical inputs and responses, such as the task of collecting training data for machine learning. In this simple repetitive input/response task, the crowdsourcer can divide the tasks into a certain number of smaller and more manageable segments (microtasks) and request the workers in crowdsourcing services to complete them (Deng et al., 2016). We developed an experimental system that assessed the work products of the first task performed by a worker and presented the results to the worker (feedback). While feedback in crowdsourcing services typically includes numeric reviews and textual comments by crowdsourcers (Feng et al., 2018; Jian et al., 2019), in this study, the correct answer rate, which can be systematically calculated from responses and immediately presented to the worker, was used. By allowing workers to check the evaluation of their work products, it is expected that if the evaluation is good, the worker will be more motivated for the task. Moreover, we paid additional rewards only if the evaluation was good because workers were expected to be more motivated when they knew that their rewards would change depending on their effort.
We examined the effects of the abovementioned system by categorizing workers into two groups: high-quality and low-quality workers. We prepared a task that could be worked on twice in succession and grouped workers according to their performance on the first task: high-quality workers were those with high correct answer ratio (hereinafter “score”) and low-quality workers were those with low scores. For high-quality workers, presentation of work product evaluations (feedback) and additional rewards were expected to positively affect their motivation because those who work sincerely are likely to feel more satisfied with their work when they know that their efforts are evaluated fairly. However, for low-quality workers it may negatively affect their motivation because they do not work honestly, and when they know that the crowdsourcer is carefully evaluating their task, they may think that they cannot complete the task with ease, even if they continue to work on it. However, it is also possible that some workers, even those who were of low-quality, change their motivation and try to work on the task with integrity when they know that their work products are being evaluated. Therefore, the following eight hypotheses were formulated:
Hypothesis 1-1: High-quality workers will continue with the tasks when they receive feedback.
Hypothesis 1-2: High-quality workers will continue with the tasks when they receive rewards in addition to feedback.
Hypothesis 1-3: High-quality workers will achieve a higher score on the second task when feedback on the first task is provided.
Hypothesis 1-4: High-quality workers will achieve a higher score on the second task when they receive rewards in addition to feedback.
Hypothesis 2-1: Low-quality workers are less likely to continue with the tasks when they receive feedback.
Hypothesis 2-2: Low-quality workers are less likely to continue with the tasks when they receive rewards in addition to feedback.
Hypothesis 2-3: Low-quality workers will achieve a higher score on the second task when feedback for the first task is provided.
Hypothesis 2-4: Low-quality workers will achieve a higher score on the second task when they receive rewards in addition to feedback.
In this study, an experiment was conducted on an actual crowdsourcing service with people who typically undertake crowdsourcing jobs. Among the crowdsourcing services available in Japan, we chose “CrowdWorks,” as it had the largest number of workers in Japan. We developed an experimental system designed to collect training data for machine learning (hereinafter referred to as the “experimental system”). The experimental system was implemented in PHP. There exist three versions (conditions) in the developed system: Condition (1) Without feedback (FB) and without additional reward (AR), Condition (2) with FB and without AR, and Condition (3) with FB and with AR.
The task prepared for the experiment was to identify the type of a flower image displayed on a screen. Specifically, the image of a flower either of the species “halcyon” (scientific name: “erigeron philadelphicus”) or of “daisy” (scientific name: “erigeron annuus”) was displayed, and workers needed to guess which of the two types of flowers was displayed (Figure 1). Because it is not easy to distinguish between these two types of flowers, an explanation of how to distinguish between them was always displayed below the question. Twenty questions were asked in succession to identify flower types and the task was presented twice. After completing the first task, the workers were allowed to complete the second task at their discretion. That is, they could end the experiment after completing the first task or proceed to the second task.
To test our hypothesis, we prepared the following three conditions:
(1) Feedback (percentage of correct answers) was not provided and no additional reward was given.
(2) Feedback (percentage of correct answers) was provided but no additional reward was given.
(3) Feedback (percentage of correct answers) was provided and additional rewards were paid to those with a high score (correct answer ratio).
By comparing conditions (1) and (2), we could clarify the relationship between feedback and task performance (i.e., the score in the second task), and between feedback and continuation rate (i.e., the ratio of workers who proceeded to the second task after completing the first task). Comparing (2) and (3) allowed us to determine whether there was a difference in task performance or continuation rate based on an additional reward when feedback was provided.
The first and second tasks consisted of 20 questions each. The score (correct answer ratio) was calculated by dividing the number of correct answers by 20 and presenting it as a percentage to the workers (Figure 2). To test the hypotheses, it was necessary to establish a threshold score to distinguish between high- and low-quality workers. Thus, we asked 13 students in our laboratory to complete the task. The results are shown in Figure 3. Based on these results, we adopted a correct answer ratio of 80% as the threshold for distinguishing between high- and low-quality workers. In the actual experiment, workers who answered the first task correctly at least 80% of the time were considered high-quality workers, and others were considered low-quality workers. All workers were paid a flat rate of 50 yen per task for the first and second tasks. In experimental condition (3), the additional reward for high-quality workers was 25 yen. It was paid to them through a dummy task on the crowdsourcing service that the authorized workers could access and obtain money by inputting a password.
In a crowdsourcing experiment, workers who are demotivated or who have worked on a task in a random manner can be eliminated by a simple rule (Matsubara and Wang, 2014; Allahbakhsh et al., 2013; Cosley et al., 2005), and this experiment was conducted with these eliminated workers as well. The participants were asked about their birth year before starting the task and their zodiac sign (sexagenary cycle traditionally used in East Asia, represented by the name of an animal in Japan) after completing the task. In addition, an image that was clearly incorrect (an image of a large lily flower) was inserted in the middle of the task (hereafter referred to as a dummy question). The usual number of choices for an answer was two, however, for the dummy question, three choices were given by adding the “neither” option. Therefore, 21 questions were presented to the participants. The experimental system also measured the start and end times of the task and calculated the time required to answer. Therefore, workers whose birth year and zodiac sign did not match, who answered anything other than “neither” to the dummy question, and whose took < 60 s to answer were considered unreliable (these criteria were called filtering rules) and were excluded from the experimental results. Here, 60 s was considered because it indicated the time in which it was physically impossible to answer the question (i.e., to input all the answers in the Web forms). A total of 300 workers were recruited for each experiment. They were recruited under the guise of participating in a plant image determination task. The experiment was also conducted under their consent to participate in the task. This study was reviewed and approved by the Research Ethics Review Committee of the authors' institution.
Table 1 shows the numbers of participants (workers) in each experimental condition, those excluded as unreliable by the filtering rule, and those who remained. The results showed that few workers provided unreliable answers. We focused on workers' score (correct answer ratio). First, a Kolmogorov-Smirnov test was performed on the scores for each experimental condition (first and second tasks) and no normality was found. The average scores of the first and second tasks in experimental conditions (1)–(3) are shown in Table 2 (second and third columns). The mean score in the first task combined with conditions (1)–(3) was 0.76, and that in the second task was 0.83. The mean score was higher in the second task than that in the first task under every experimental condition. Because a worker could perform both the tasks, their corresponding scores were used. Wilcoxon signed-rank tests were conducted for workers performing both tasks, and significant differences were found with p = 9.99e-13, 1.34e-11, and 2.65e-07 (all < 0.05) for experimental conditions (1), (2), and (3), respectively.
Table 2. Average score in the first and second tasks and continuation rate of all workers in each experimental condition.
Although not a hypothesis of the study, we first examined whether feedback and additional rewards affected continuation rates and scores for all participants including both high-quality workers and low-quality workers. In detail, we checked whether the difference between the scores in the first and second tasks differed depending on the experimental condition. A Kruskal-Wallis test was conducted, yielding a p = 0.69 (>0.05). The Wilcoxon rank sum test with Bonferroni's adjustment showed no significant difference, with p = 1.00, 1.00, 1.00 (all >0.05), for experimental conditions (1) and (2), (2) and (3), and (1) and (3), respectively. Therefore, there was no difference in the scores depending on the experimental conditions.
The continuation rates for experimental conditions (1) and (3) are shown in Table 2 (fourth column). We statistically tested whether continuation rate differed depending on the experimental conditions. A chi-square test of the experimental conditions showed a significant difference, with a p-value of 4.84e-6 (< 0.05). The Cramer's coefficient of association was 0.17. Residual analysis revealed that the adjusted standardized residuals for experimental conditions (1), (2), and (3) were 2.32, 2.73, and −4.94, respectively (all absolute values >1.96), indicating significant differences. This indicated that the experimental condition (3) with feedback and additional rewards had a lower continuation rate than other conditions. Experimental condition (2) with feedback but no additional reward and experimental condition (1) with neither feedback nor additional reward had higher continuation rates than experimental condition (3). However, there was no difference between them in the continuation rates. This suggested that feedback was ineffective in increasing the overall continuation rate.
Next, we examined whether task continuation rate and score on the second task differed depending on the quality of the workers. Specifically, we calculated the average score (correct answer ratio) and continuation rate for the high- and low-quality groups. The results for the high- and low-quality workers are shown in Tables 3, 4, respectively.
Table 3. Average score in the first and second tasks and continuation rate of high-quality workers in each experimental condition.
Table 4. Average score in the first and second tasks and continuation rate of low-quality workers in each experimental condition.
We statistically examined whether the continuation rate differed between the experimental conditions for high- and low-quality workers. First, we focused on high-quality workers. A chi-square test was conducted on experimental condition and task continuation, and a significant difference was found with a p = 0.017 (< 0.05). The Cramer's coefficient of association was 0.14. Further residual analysis revealed that the adjusted standardized residuals for experimental conditions (1), (2), and (3) were 0.86, 2.06, and −2.72, respectively (judgment condition |stdres| >1.96), indicating significant differences. Thus, we found that experimental condition (3) with additional reward had a lower continuation rate than experimental condition (2) without it. Therefore, Hypothesis 1-2 was not supported. The continuation rate was higher in experimental condition (2), in which feedback was provided, than in experimental condition (1), in which no feedback was provided. Thus, Hypothesis 1-1 was supported. This suggested that feedback succeeded in maintaining the motivation of high-quality workers to some extent. In other words, workers with high judgment ability or motivation might have been able to maintain their motivation by learning about their own performance.
Regarding low-quality workers, A chi-square test on experimental condition and task continuation showed a significant difference, p = 5.98e-5 (< 0.05). The Cramer's coefficient of association was 0.22. Further residual analysis revealed that the adjusted standardized residuals for experimental conditions (1), (2), and (3) were 2.37, 1.93, and −4.40, respectively (judgment condition |stdres| >1.96), indicating significant differences. Thus, we found that experimental condition (3) with additional reward had a lower continuation rate than the other conditions. Thus, Hypothesis 2-2 was supported. It was likely that low-quality workers, in other words, workers with poor judgment ability or low motivation, lost the motivation to continue working on the task even when additional rewards were offered. Experimental condition (1), with neither additional reward nor feedback, had the highest continuation rate. In experimental condition (2), in which only feedback was provided, no association was found, and Hypothesis 2-1 was not supported.
Next, we focused on scores (correct answer ratio). Those of the high-quality workers showed little change, with the mean of the first and second task scores being approximately 0.86. To confirm this, Wilcoxon signed-rank tests were conducted on the first and second task scores of high-quality workers who proceeded to the second task. The p-values were 0.60, 1.00, and 0.43 (all >0.05) for conditions (1), (2), and (3), respectively, and no significant differences were found. Additionally, we checked whether the difference between the second and first task scores depended on experimental conditions. A Kruskal-Wallis test was performed which showed a p = 0.76 (>0.05). A Wilcoxon rank sum test with Bonferroni adjustment revealed no significant differences with p = 1.00, 1.00, 1.00 (all >0.05) for experimental conditions (1) and (2), (2) and (3), and (1) and (3), respectively. Therefore, Hypotheses 1-3 and 1-4 were not supported.
In contrast, low-quality workers' second task scores were higher than their first task scores in all experimental conditions. Wilcoxon signed-rank tests revealed significant differences in the experimental conditions (1), (2), and (3) with p = 4.49e-15, 1.11e-14, and 1.07e-5 (all < 0.05), respectively. We tested whether the difference between the second and first task scores depended on experimental conditions. A Kruskal-Wallis test was performed with a p = 0.14 (>0.05). A Wilcoxon rank sum test with Bonferroni's adjustment revealed no significant differences with p = 1.00, 0.20, 0.26 > 0.05 for the experimental conditions (1) and (2), (2) and (3), and (1) and (3), respectively.
Moreover, we checked whether the second task score differed according to experimental conditions. The Kruskal-Wallis test was performed with a p-value of 0.0033 (< 0.05). The Wilcoxon rank sum test with Bonferroni's adjustment revealed partially significant differences with p = 1.00, 0.039, and 0.0026 (p < 0.05 for the decision condition) for the experimental conditions (1) and (2), (2) and (3), and (1) and (3), respectively. Thus, the highest score was obtained in the experimental condition (3) (with feedback and additional rewards). Thus, Hypothesis 2-4 was partially supported. That is, although we were not able to increase the scores of workers individually, we were able to maintain an overall high score for the second task, as some workers did not proceed to the second task.
Surprisingly, the second task scores of the low-quality workers were almost identical to those of high-quality workers. This may be due to the fact that those who did not work hard for the first time worked hard the second time to receive the additional reward, and that only the low-quality workers who were confident about receiving the additional reward in the second time proceeded to the second task. In experimental condition (2) (with feedback and no additional reward), the increase in scores was much smaller than in experimental condition (3). Therefore, Hypothesis 2-3 was not supported. It is likely that low-quality workers were not motivated by feedback on work product evaluations alone.
An analysis of all workers showed that additional rewards reduced the task continuation, and an analysis of high- and low-quality workers showed that providing feedback on work product evaluations contributed to a higher continuation rate for high-quality workers. However, it had little effect on the continuation rate of low-quality workers. Feedback did not improve the second task score for either high- or low-quality workers. Although additional reward largely reduced the continuation rate for low-quality workers, it also slightly reduced that for high-quality workers. Although the additional rewards did not improve individual scores among low-quality workers, high scores were maintained for those who proceeded to the second task.
Previous studies on motivation for crowdsourcing task efforts (Feng et al., 2018; Cappa et al., 2019; Kaufmann et al., 2011) found that rewards positively affect worker motivation. This is inconsistent with the results of this study which showed that additional rewards did not lead to higher continuation rates. One reason for this is that the previous studies involved working on different tasks (one-shot tasks independent of each other), whereas our study involved working on the next task immediately (a task with almost the same content as the first one). The decision to work on the task was not a significant cognitive burden, and rewards might not have positively affected worker motivation. Another possibility is that the tasks used in the experiment were microtasks aimed at acquiring machine learning training data; therefore, the base and additional reward (half of the base reward) amounts were small (Of course, the amount of compensation they receive for their working hours is not low). It is possible that this small amount did not motivate workers to work diligently on the second task and receive additional rewards.
Regarding the motivation behind each worker's quality, additional rewards did not increase the continuation rate of high-quality workers. It is possible that rewards are not the only motivating factors for high-quality workers. The additional reward may have provided them with a certain degree of satisfaction and discouraged them from continuing to work on the task. This can be explained using the attribution process (Kelley and Michela, 1980), from the field of social psychology, which mention that people infer why events in the real world, including their own actions, occurred. The factors affecting human behavior can be largely divided into internal and external factors (Weiner, 1974). The target event in this study needed the user's own effort to guess the name of the flower. In our experimental task, the internal factors might include the user's original pleasures and enjoyment of engaging in the crowdsourcing task (Deng and Joshi, 2016; Ye and Kankanhalli, 2017) and personal growth achievement obtained by finishing the task (Deng and Joshi, 2016; Feller et al., 2012), and the external factor might be the reward that can be obtained by working on the task (Taylor and Joshi, 2019). Generally, monetary rewards are used as incentives in exchange of contributions to crowdsourcing tasks (Hann et al., 2013; Khern-am-nuai et al., 2018). In crowdsourcing services, it is a common practice to get paid on task completion; therefore, the standard amount of payment is not a strong external factor. In fact, the rewards for the tasks in our experiment were not higher than those for other tasks in crowdsourcing. The task was divided into microtasks and a standard payment was set considering the actual working hours. It seems that high-quality workers began this task with the intention of taking it seriously. In other words, they were motivated by internal factors. However, the additional reward after working on the task may have changed the cause of their action from an internal to an external factor of obtaining the reward. It has been shown in a study on young children's play (Lepper et al., 1973) that switching attribution from internal to external factors results in a loss of motivation for the task, and the results of the present study seem to be consistent with this. Cappa et al. (2019) showed that financial and social rewards (explanation of social benefit) were able to attract more participation, indicating that both extrinsic and intrinsic motivation should be utilized to increase the number of participants and contributions. They also suggested that methods of reward that negatively affect intrinsic motivation should be avoided. Based on the above discussion, it is necessary to consider how to reward high-quality workers without damaging their motivation.
This study had some limitations. We set up a simple task as the experiment to judge whether an example was true or false, assuming the acquisition of training data for machine learning. Our experimental results show that feedback on performance evaluation increases the continuation rate of high-quality workers, and that additional rewards increase the overall performance of low-quality workers. We cannot confirm if our experimental results are applicable to other creative tasks, such as creating a tagline for a product or composing a theme song. We are also uncertain if our results can be applied to tasks that require logical thinking to make judgments. The feedback in this experiment was simply the number of correct answers out of 20 questions, and the percentage of correct answers. If emotional expressions are added to feedback, the continuation rate may change. It has been found that a person's altruistic behavior is influenced by empathy for the other person (Batson et al., 1981). Expressions of empathy and emotional praise for the efforts of the worker may influence task continuation. It has also been found that workers committed to the crowdsourcing community are more likely to voluntarily engage in tasks (Ghosh et al., 2012). It would be good to indicate how much of the deliverables by the worker contributed to the target community (e.g., the discipline of biology or environmental studies, if the task was to identify flower species used in the experiment). Future work should conduct similar experiments on a variety of tasks and investigate the effects of different types of feedback, including emotional feedback. In particular, emotional appeals may influence workers' internal motivation. For example, simply showing troubles that a researcher faces may motivate workers to participate in the task. What kind of appeals should be included in task instruction is an issue for future research.
In conclusion, this study examined whether the presentation of evaluations of work products (feedback) and additional rewards in crowdsourcing services can encourage workers to continue working on tasks and improve the quality of deliverables. We developed an experimental system that can be changed with or without feedback and additional rewards, and conducted an experiment on a real crowdsourcing service using this system. When only feedback was provided, the task continuation rate increased for high-quality workers. However, for low-quality workers, the task continuation rate could not be reduced. Feedback did not contribute to an increase in the correct answer rate for either type of worker. Although the presence of both feedback and additional rewards reduced workers' task continuation rate, the amount of reduction was larger for low-quality workers than that for high-quality workers. Furthermore, it significantly increased the low-quality worker's task score. Although not statistically significant, the second score was highest for those who were shown both feedback and additional rewards.
These results are not simple, but they suggest that for businesses ordering tasks for crowdsourcing, it is better to offer both feedback and additional rewards when the quality of the deliverables is a priority, and to give only feedback when the quantity of deliverables is a priority. In the future, we aim to improve the quality of the work products of the subsequent task of low-quality workers without decreasing the continuation rate of high-quality workers by devising the reward method and messages in the feedback.
The raw data supporting the conclusions of this article will be made available by the authors, without undue reservation.
The studies involving humans were approved by the Research Ethics Review Committee of “Behavioral Studies on Human Subjects” at Kwansei Gakuin University. The studies were conducted in accordance with the local legislation and institutional requirements. Written informed consent for participation was not required from the participants or the participants' legal guardians/next of kin because the experiment was conducted online.
YH: Writing – original draft, Writing – review & editing, Conceptualization, Data curation, Formal analysis, Funding acquisition, Investigation, Methodology, Project administration, Resources, Software, Supervision, Validation, Visualization. HI: Data curation, Investigation, Writing – review & editing.
The author(s) declare that financial support was received for the research and/or publication of this article. This work was supported by JSPS KAKENHI Grant Number JP19K12242 and JP23K28194, and was partially supported by JST CREST Grant Number JPMJCR20D4.
The authors declare that the research was conducted in the absence of any commercial or financial relationships that could be construed as a potential conflict of interest.
The author(s) declare that no Gen AI was used in the creation of this manuscript.
All claims expressed in this article are solely those of the authors and do not necessarily represent those of their affiliated organizations, or those of the publisher, the editors and the reviewers. Any product that may be evaluated in this article, or claim that may be made by its manufacturer, is not guaranteed or endorsed by the publisher.
Allahbakhsh, M., Benatallah, B., Ignjatovic, A., Motahari-Nezhad, H. R., Bertino, E., and Dustdar, S. (2013). Quality control in crowdsourcing systems: issues and directions. IEEE Internet Comput. 17, 76–81. doi: 10.1109/MIC.2013.20
Antikainen, M., Mäkipää, M., and Ahonen, M. (2010). Motivating and supporting collaboration in open innovation. Eur. J. Innov. Manag. 13, 100–119. doi: 10.1108/14601061011013258
Batson, C. D., Duncan, B. D., Ackerman, P., Buckley, T., and Birch, K. (1981). Is empathic emotion a source of altruistic motivation? J. Pers. Soc. Psychol. 40, 290–302. doi: 10.1037/0022-3514.40.2.290
Cappa, F., Rosso, F., and Hayes, D. (2019). Monetary and social rewards for crowdsourcing. Sustainability 11, 2834. doi: 10.3390/su11102834
Chaffois, C., Gillier, T., Belkhouja, M., and Roth, Y. (2015). “How task instructions impact the creativity of designers and ordinary participants in online idea generation,” in Proceedings of the 22nd Innovation Product Development Management Conference (IPDMC) (Lyon: HAL).
Cosley, D., Frankowski, D., Kiesler, S., Terveen, L., and Riedl, J. (2005). “How oversight improves member-maintained communities,” in Proceedings of the SIGCHI Conference on Human Factors in Computing Systems (CHI '05) (New York, NY: ACM). doi: 10.1145/1054972.1054975
Deng, X., Joshi, K. D., and Galliers, R. D. (2016). The duality of empowerment and marginalization in microtask crowdsourcing: giving voice to the less powerful through value sensitive design. MIS Q. 40, 279–302. doi: 10.25300/MISQ/2016/40.2.01
Deng, X. N., and Joshi, K. D. (2016). Why individuals participate in micro-task crowdsourcing work environment: revealing crowdworkers' perceptions. J. Assoc. Inf. Syst. 17, 711–736. doi: 10.17705/1jais.00441
Dow, S., Kulkarni, A. P., Klemmer, S. R., and Hartmann, B. (2012). “Shepherding the crowd yields better work,” in Proceedings of the ACM Conference on Computer Supported Cooperative Work (CSCW '12) (New York, NY: ACM) 1013–1022. doi: 10.1145/2145204.2145355
Feller, J., Finnegan, P., Hayes, J., and O'Reilly, P. (2012). ‘Orchestrating' sustainable crowdsourcing: a characterisation of solver brokerages. J. Strateg. Inf. Syst. 21, 216–232. doi: 10.1016/j.jsis.2012.03.002
Feng, Y., Ye, H. J., Yu, Y., Yang, C., and Cui, T. (2018). Gamification artifacts and crowdsourcing participation: examining the mediating role of intrinsic motivations. Comput. Hum. Behav. 81, 124–136. doi: 10.1016/j.chb.2017.12.018
Ghosh, R., Reio, J. T. G., and Haynes, R. K. (2012). Mentoring and organizational citizenship behavior: estimating the mediating effects of organization-based self-esteem and affective commitment. Hum. Resour. Dev. Q. 23, 41–63. doi: 10.1002/hrdq.21121
Goncalves, J., Hosio, S., Ferreira, D., and Vassilis, K. (2014). “Game of words: tagging places through crowdsourcing on public displays,” Proceedings of the 2014 ACM Conference on Designing Interactive Systems (DIS '14), ACM, 705–714. doi: 10.1145/2598510.2598514
Hann, I.-H., Roberts, J. A., and Slaughter, S. A. (2013). All are not equal: an examination of the economic returns to different forms of participation in open source software communities. Inf. Syst. Res. 24, 520–538. doi: 10.1287/isre.2013.0474
Ho, C.-J., Slivkins, A., Suri, S., and Vaughanet, J. W. (2015). “Incentivizing high quality crowdwork,” Proceedings of the 24th International Conference on World Wide Web (WWW '15), International World Wide Web Conferences Steering Committee, Republic and Canton of Geneva, CHE (New York, NY: ACM), 419–429. doi: 10.1145/2736277.2741102
Hong, Y., Kwak, H., Baek, Y., and Moon, S. (2013). “Tower of babel: a crowdsourcing game building sentiment lexicons for resource-scarce languages,” in Proceedings of the ACM 22nd International Conference on World Wide Web (WWW '13) (New York, NY: ACM), 549–556. doi: 10.1145/2487788.2487993
Howe, J. (2006). The Rise of Crowdsourcing. Wired. Available online at: http://www.wired.com/wired/archive/14.06/crowds_pr.html (accessed June 1, 2006).
Huang, L., Xie, G., Blenkinsopp, J., Huang, R., and Bin, H. (2020). Crowdsourcing for sustainable urban logistics: exploring the factors influencing crowd workers' participative behavior. Sustainability 12, 3091. doi: 10.3390/su12083091
Jackson, C., Østerlund, C. S., Mugar, G., Hassman, K. D. V., and Crowston, K. (2015). “Motivations for sustained participation in crowdsourcing: case studies of citizen science on the role of talk,” in Proceedings of the 48th Hawaii International Conference on System Sciences (New York, NY: IEEE), 1624–1634. doi: 10.1109/HICSS.2015.196
Jian, L., Yang, S., Ba, S., Lu, L., and Jiang, C. (2019). Managing the crowds: the effect of prize guarantees and in-process feedback on participation in crowdsourcing contests. MIS Q. 43, 97–112. doi: 10.25300/MISQ/2019/13649
Kaufmann, N., Schulze, T., and Veit, D. (2011). “More than fun and money. Worker motivation in crowdsourcing: a study on mechanical turk,” in Proceedings of the Seventeenth Americas Conference on Information Systems (AMCIS '11) (Atlanta, GA: AIS).
Kelley, H. H., and Michela, J. L. (1980). Attribution theory and research. Annu. Rev. Psychol. 31, 457–501. doi: 10.1146/annurev.ps.31.020180.002325
Khern-am-nuai, W., Kannan, K., and Ghasemkhani, H. (2018). Extrinsic vs. intrinsic rewards for contributing reviews in an online platform. Inf. Syst. Res. 29, 871–892. doi: 10.1287/isre.2017.0750
Lepper, M. R., Greene, D., and Nisbett, R. E. (1973). Undermining children's intrinsic interest with extrinsic reward: a test of “overjustification” hypothesis. J. Pers. Soc. Psychol. 28, 129–137. doi: 10.1037/h0035519
Liu, Y., and Liu, Y. (2019). The effect of workers' justice perception on continuance participation intention in the crowdsourcing market. Internet Res. 29, 1485–1508. doi: 10.1108/INTR-02-2018-0060
Matsubara, S., and Wang, M. (2014). Preventing participation of insincere workers in crowdsourcing by using pay-for-performance payments. IEICE Trans. Inf. Syst. E97D, 2415–2422. doi: 10.1587/transinf.2013EDP7441
Morschheuser, B., Hamari, J., and Maedche, A. (2019). Cooperation or competition–When do people contribute more? A field experiment on gamification of crowdsourcing. Int. J. Hum.-Comput. Stud. 127, 7–24. doi: 10.1016/j.ijhcs.2018.10.001
Rockmann, K. W., and Ballinger, G. A. (2017). Intrinsic motivation and organizational identification among on-demand workers. J. Appl. Psychol. 102, 1305–1316. doi: 10.1037/apl0000224
Rogstadius, J., Kostakos, V., Kittur, A., Smus, B., Laredo, J., and Vukovic, M. (2011). An assessment of intrinsic and extrinsic motivation on task performance in crowdsourcing markets. Proc. 5th Int. AAAI Conf. Web Soc. Media 5, 321–328. doi: 10.1609/icwsm.v5i1.14105
Ryan, R. M., and Deci, E. L. (2000). Self-determination theory and the facilitation of intrinsic motivation, social development, and well-being. Am. Psychol. 55, 68–78. doi: 10.1037/0003-066X.55.1.68
Soliman, W., and Tuunainen, V. K. (2015). Understanding continued use of crowdsourcing systems: an interpretive study. J. Theor. Appl. Electron. Commer. Res. 10, 1–18. doi: 10.4067/S0718-18762015000100002
Sun, Y., Fang, Y., and Lim, K. H. (2012). Understanding sustained participation in transactional virtual communities. Decis. Support Syst. 53, 12–22. doi: 10.1016/j.dss.2011.10.006
Sun, Y., Wang, N., Yin, C., and Zhang, X. (2015). Understanding the relationships between motivators and effort in crowdsourcing marketplaces: a nonlinear analysis. Int. J. Inf. Manag. 35, 267–276. doi: 10.1016/j.ijinfomgt.2015.01.009
Tauchmann, C., Daxenberger, J., and Mieskes, M. (2020). “The influence of input data complexity on crowdsourcing quality,” in Proceedings of the 25th International Conference on Intelligent User Interfaces Companion (IUI '20) (New York, NY: ACM), 71–72. doi: 10.1145/3379336.3381499
Taylor, J., and Joshi, K. D. (2019). Joining the crowd: the career anchors of information technology workers participating in crowdsourcing. Inf. Syst. J. 29, 641–673. doi: 10.1111/isj.12225
Uhlgren, V.-V., Laato, S., Hamari, J., and Nummenmaa, T. (2024). “Gamification to motivate the crowdsourcing of dynamic nature data: a field experiment in northern Europe,” Proceedings of the 27th International Academic Mindtrek Conference (Mindtrek'24) (New York, NY: ACM), 230–234. doi: 10.1145/3681716.3689440
Wasko, M. M., and Faraj, S. (2005). Why should I share? Examining social capital and knowledge contribution in electronic networks of practice. MIS Q. 29, 35–57. doi: 10.2307/25148667
Watts, D. J., and Mason, W. (2009). “Financial incentives and the ‘performance of crowds',” Proceedings of the ACM SIGKDD Workshop on Human Computation (HCOMP '09) (New York, NY: ACM), 77–85. doi: 10.1145/1600150.1600175
Weiner, B. (1974). Achievement Motivation and Attribution Theory. New York, NY: General Learning Press.
Yang, J., Adamic, L. A., and Ackerman, M. S. (2008). “Crowdsourcing and knowledge sharing: strategic user behavior on TaskCN,” in Proceedings of the 9th ACM Conference on Electronic Commerce (EC'08) (New York, NY: ACM), 246–255. doi: 10.1145/1386790.1386829
Ye, H. J., and Kankanhalli, A. (2017). Solvers' participation in crowdsourcing platforms: examining the impacts of trust, and benefit and cost factors. J. Strateg. Inf. Syst. 26, 101–117. doi: 10.1016/j.jsis.2017.02.001
Yin, M., Chen, Y., and Sun, Y.-A. (2013). “The effects of performance-contingent financial incentives in online labor markets,” Proceedings of the 27th AAAI Conference on Artificial Intelligence (AAAI '13) (Washington, DC: AAAI), 1191–1197. doi: 10.1609/aaai.v27i1.8461
Keywords: crowdsourcing, deliverable assessment, evaluation feedback, additional reward, worker motivation, task continuation, task performance
Citation: Hijikata Y and Ishizaki H (2025) Influence of deliverable evaluation feedback and additional reward on worker's motivation in crowdsourcing services. Front. Organ. Psychol. 3:1500016. doi: 10.3389/forgp.2025.1500016
Received: 29 September 2024; Accepted: 18 March 2025;
Published: 09 April 2025.
Edited by:
Ron Landis, Wilbur O. and Ann Powers College of Business, Clemson University, United StatesReviewed by:
Hale Erden, Final International University, CyprusCopyright © 2025 Hijikata and Ishizaki. This is an open-access article distributed under the terms of the Creative Commons Attribution License (CC BY). The use, distribution or reproduction in other forums is permitted, provided the original author(s) and the copyright owner(s) are credited and that the original publication in this journal is cited, in accordance with accepted academic practice. No use, distribution or reproduction is permitted which does not comply with these terms.
*Correspondence: Yoshinori Hijikata, Y29udGFjdEBzb2MtcmVzZWFyY2gub3Jn
Disclaimer: All claims expressed in this article are solely those of the authors and do not necessarily represent those of their affiliated organizations, or those of the publisher, the editors and the reviewers. Any product that may be evaluated in this article or claim that may be made by its manufacturer is not guaranteed or endorsed by the publisher.
Research integrity at Frontiers
Learn more about the work of our research integrity team to safeguard the quality of each article we publish.