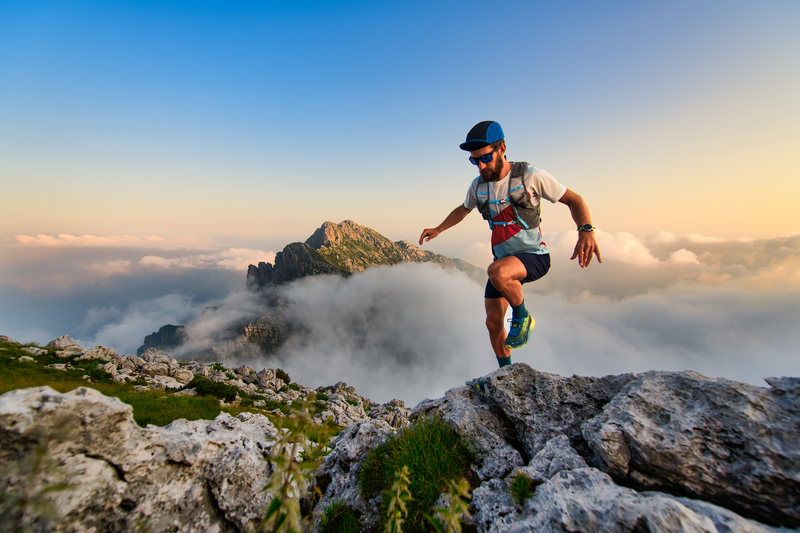
94% of researchers rate our articles as excellent or good
Learn more about the work of our research integrity team to safeguard the quality of each article we publish.
Find out more
ORIGINAL RESEARCH article
Front. Organ. Psychol. , 23 December 2024
Sec. Performance and Development
Volume 2 - 2024 | https://doi.org/10.3389/forgp.2024.1468907
Artificial intelligence (AI) is increasingly taking over leadership tasks in companies, including the provision of feedback. However, the effect of AI-driven feedback on employees and its theoretical foundations are poorly understood. We aimed to close this research gap by comparing perceptions of AI and human feedback based on construal level theory and the feedback process model. Using these theories, our objective was also to investigate the moderating role of feedback valence and the mediating effect of social distance. A 2 × 2 between-subjects design was applied to manipulate feedback source (human vs. AI) and valence (negative vs. positive) via vignettes. In a preregistered experimental study (S1) and subsequent direct replication (S2), responses from NS1 = 263 and NS2 = 449 participants were studied who completed a German online questionnaire asking for feedback acceptance, performance motivation, social distance, acceptance of the feedback source itself, and intention to seek further feedback. Regression analyses showed that AI feedback was rated as less accurate and led to lower performance motivation, acceptance of the feedback provider, and intention to seek further feedback. These effects were mediated by perceived social distance. Moreover, for feedback acceptance and performance motivation, the differences were only found for positive but not for negative feedback in the first study. This implies that AI feedback may not inherently be perceived as more negatively than human feedback as it depends on the feedback's valence. Furthermore, the mediation effects indicate that the shown negative evaluations of the AI can be explained by higher social distance and that increased social closeness to feedback providers may improve appraisals of them and of their feedback. Theoretical contributions of the studies and implications for the use of AI for providing feedback in the workplace are discussed, emphasizing the influence of effects related to construal level theory.
In some organizations, technologies using artificial intelligence (AI) are being implemented to perform tasks usually executed by managers, such as assigning tasks, terminations, and providing feedback. For instance, Uber uses AI algorithms to assign rides to drivers, give them feedback, and deactivate their accounts if they perform poorly (Wesche and Sonderegger, 2019; Zhang et al., 2023). Most likely, AI technology will play an increasingly important role in the management of organizations (Yam et al., 2022). This development is facilitated by technological advances enabling the completion of complex tasks as well as social interactions with such technologies (Harms and Han, 2019; Hubner et al., 2019; Cichor et al., 2023). Moreover, an AI system is not designed to be influenced by self-interest, mood, or stress and might therefore be perceived as less biased and subjective (Hubner et al., 2019). Also, AI technology has the potential to evaluate employee behavior more comprehensively by processing larger amounts of data than a human could (Tong et al., 2021; Tsai et al., 2022).
Despite the increasing involvement of AI systems in leadership tasks, its effects on employees are sparsely investigated in psychological science. First, AI technologies are mostly considered as subordinate tools, but rarely as superordinate counterparts performing leadership responsibilities (e.g., Wesche and Sonderegger, 2019; Tsai et al., 2022). Only recently, concepts such as algorithmic leadership (i.e., AI or algorithms take over leadership activities; Harms and Han, 2019) and automated leadership (i.e., computer agents influence human leaders purposefully; Höddinghaus et al., 2021) emerged. Previously, merely terms describing human supervisors who use information and communication technologies were established (e-leadership; Avolio et al., 2014). Second, if at all, leadership through AI is only discussed on a conceptual level and not examined empirically (e.g., Gladden, 2014; Hou and Jung, 2018). Third, it was criticized that there are no theories or models that address employees' responses to AI executing leadership activities (e.g., Lopes, 2021; Wesche and Sonderegger, 2019).
Examining feedback provision is particularly relevant for AI carrying out leadership functions. As illustrated, AI systems are already implemented in organizations to perform this function. Other leadership activities that go beyond processing information, such as inspiring employees, are currently not easily transferable to AI and require further technical advancement (Gladden, 2016). Moreover, supervisors seem to be motivated to hand over the communication of negative feedback to AI, as this is an unpleasant task (Gloor et al., 2020). At the same time, research showed that the provision of feedback by an algorithm is experienced more negatively than the execution of other leadership tasks, such as work assignment or scheduling (Lee, 2018). However, the employees' perception of feedback can have far-reaching effects on their work engagement and turnover intention (Lee, 2018). Since the prevalence of AI feedback seems not to be reflected in a positive perception thereof, it is necessary to look more closely at responses to AI feedback and identify possible factors influencing them.
Therefore, the present paper extends existing research by empirically investigating the appraisal and motivational function of AI feedback in the workplace using a theoretical foundation. We conducted an experimental vignette study with a direction replication to compare the effects of AI and human feedback on feedback acceptance and performance motivation using the feedback process model (FPM; Ilgen et al., 1979). The social distance to the feedback provider was considered as a mediator and the feedback valence as a moderator of these effects. These assumptions were based on the construal level theory (CLT; Trope and Liberman, 2010). Furthermore, we studied if the acceptance of the feedback source and the intention to seek further feedback differed between an AI and a human supervisor and if the feedback valence moderated the effect of feedback source on acceptance of feedback source and intention to seek further feedback. This is therefore one of the first investigations to compare perceptions of a message to perceptions of the message source in AI research, and likely the first in the area of AI feedback. In addition to theoretical implications, conclusions on the use of AI feedback in the workplace were derived.
The influence of the feedback source on the perceptions of the feedback was studied against the background of the CLT (Trope and Liberman, 2010). CLT posits at its core that mental representations are related to the perceived distance from targets. One dimension of the CLT's construct of psychological distance is social distance, describing how distant or distinct social objects feel to oneself (Bar-Anan et al., 2006). Referring to CLT and previous investigations (Ahn et al., 2021; Kaju, 2020), people should experience higher social distance to an AI than to a human. This could be explained by people experiencing higher familiarity with humans than AI agents due to more frequent interactions (Förster et al., 2009) and them feeling more similar to a human because of equal appearance and shared attributes (Prahl and van Swol, 2017; Ahn et al., 2021). Further, a smaller psychological distance to counterparts led to more positive attitudes toward their input (Ahn et al., 2021; Greller, 1978). Consequently, feedback from a human should be judged more positively than from an AI. As an indication that this can indeed be explained by psychological distance, Li and Sung (2021) found that a positive correlation between perceived anthropomorphization (i.e., human-likeness) and appraisal of an AI assistant was mediated by psychological distance.
Based on the FPM (Ilgen et al., 1979), the feedback source should not only influence the feedback acceptance but also the performance motivation as a crucial stage of the processing of feedback. According to the FPM, feedback acceptance is the recipients' belief that the feedback accurately reflects their performance and impacts the intended response, that is, the way they aim to react, and the goals pursued thereby (Ilgen et al., 1979). According to Kinicki et al. (2004), the latter construct represents performance motivation, which covers the employee's desire to perform better based on feedback (Fedor et al., 1989). The positive influence of feedback acceptance on performance motivation or job performance has been empirically supported (e.g., Kinicki et al., 2004; Anseel and Lievens, 2009). Therefore, a higher social distance to an AI should result in the recipient being less accepting of the feedback and thus less motivated compared to a human. Accordingly, subjects rated the quality of human feedback higher than that of AI feedback (Tong et al., 2021) and had a lower job performance with AI than human feedback or guidance (Tong et al., 2021; Lopes, 2021). Based on the theoretical explanations and empirical evidence, the following hypotheses were assumed (see preregistration at https://osf.io/bna2z):
H1: The feedback source influences the feedback acceptance and performance motivation.
H1a: Feedback provided by an AI is less accepted than feedback from a human supervisor.
H1b: Feedback provided by an AI leads to less performance motivation than feedback from a human supervisor.
H2: The effect of feedback source on feedback acceptance is mediated by psychological distance to the feedback source.
H2a: An AI is perceived to be more socially distant than a human supervisor.
H2b: Social distance negatively affects feedback acceptance.
CLT also states that psychological distance influences the construal level (CL), that is, the mental representation of objects, people, and events (Trope and Liberman, 2010). A high CL is characterized by more abstract thinking and is applied at a higher psychological distance than a low CL. This implies that people receiving feedback from an AI should apply a higher CL than people receiving feedback from a human due to their higher social distance. This was supported by Ahn et al. (2021), who found that recommendations with abstract compared to concrete content were rated more positively when presented by an AI and more negatively when delivered by a human. In line with CLT, this effect was mediated by the experienced psychological distance to the agent.
Furthermore, previous research suggested that the CL influences feedback perceptions. While lower CLs should enhance self-protection motives and thus make positive feedback more desirable, higher CLs should strengthen self-change motives and interest in negative feedback (Belding et al., 2015). Likewise, subjects with low CL preferred positive and strength-focused feedback, whereas people with high CLs were more interested in negative and weakness-focused feedback (Freitas et al., 2001). In the context of AI feedback, feedback valence has been addressed by Yam et al. (2022). They demonstrated that negative feedback from an anthropomorphized robot led to more retaliation than from a non-anthropomorphized robot. However, they did not compare negative with positive feedback nor robots with humans. Based on these findings, negative feedback should result in higher feedback acceptance and performance motivation given AI than human feedback. Contrarily, positive feedback from a human should be more accepted and motivating than from an AI due to a lower CL. Considering the supposed negative relationship between social distance and feedback perception, the difference between both feedback sources should be larger for positive than negative feedback. Taking these assumptions into account, the following hypotheses were made:
H3: Feedback valence moderates the effect of feedback source on feedback acceptance.
H3a: Negative feedback is more accepted when it is provided by an AI than a human supervisor.
H3b: Positive feedback is more accepted when it is provided by a human supervisor than an AI.
H4: Feedback valence moderates the effect of feedback source on performance motivation.
H4a: Negative feedback leads to more performance motivation when it is provided by an AI than a human supervisor.
H4b: Positive feedback leads to more performance motivation when it is provided by a human supervisor than an AI.
In addition to the effects of feedback source and valence on the feedback evaluation, the appraisal of the feedback source itself is important to consider. Specifically, the acceptance of the feedback source and the intention to seek further feedback from it should be relevant for deploying AI feedback providers. Alongside arguments of Wesche and Sonderegger (2019), the service robot acceptance model of Wirtz et al. (2018) underlines this by defining the acceptance of robots as an antecedent of their actual use. Likewise, intention to seek further feedback can be seen as equivalent to the behavioral intention construct, which determines the actual use of technologies in the technology acceptance model (Venkatesh and Bala, 2008) and unified theory of acceptance and use of technology (Venkatesh and Bala, 2008). If employees would not interact with and seek feedback from the AI feedback provider, this would harm their performance and job satisfaction (Anseel et al., 2015; De Stobbeleir et al., 2011). Therefore, the following research questions were posed:
RQ1: Do (a) the acceptance of the feedback source and (b) the intention to seek further feedback differ between an AI and a human supervisor?
RQ2: Does the feedback valence moderate the effect of feedback source on (a) acceptance of feedback source and (b) intention to seek further feedback?
Participants were recruited using platforms and mailing lists of German universities, social media, and snowball sampling (see Supplementary Table S1a; all Appendices are available on the journal's website and on the OSF platform unter https://osf.io/3uyna). Participation requirements were age of majority, proficiency in German, and no participation in the pretest, which was used to evaluate materials. As incentive, students could receive course credit.
Participants were excluded according to the preregistration (see https://osf.io/bna2z). A total of 295 subjects completed the questionnaire, containing participants who correctly answered the item asking for the feedback source and attention check (see Supplementary Table S1b for items). Ten subjects were not included who could not sufficiently empathize with the situation and 22 participants who did not perceive the feedback valence as intended (see Supplementary Table S1b for items). Analyses for H1, H2, and RQ1, for which feedback valence was initially irrelevant, were also calculated including the 22 subjects and therefore with 285 subjects. Since the results varied only slightly to the reduced sample size of 263 participants, all analyses were based on the same sample of 263 participants. In detail, 66 participants received negative and 65 positive feedback from a human, while 67 got negative and 65 positive feedback from an AI. The sample size exceeded the minimum number of 227 participants calculated with a priori power analyses using G*Power 3 (Faul et al., 2009), a power of 0.80, an alpha of 0.05, and an effect size of f2 = 0.035 (see preregistration at https://osf.io/bna2z for computations).
Most participants identified as female (65.02% female, 31.56% male, 1.52% diverse, 1.90% no indication). The 252 subjects who voluntarily indicated their age were 18 to 65 years old with 25.94 years on average (SD = 8.52). Most subjects reported being a student (49.43%), employee (35.36%), or public official (4.94%). Additional sample information is shown in Supplementary Table S1a.
The study was preregistered (see https://osf.io/bna2z9) and then conducted online using the SoSci Survey software (Leiner, 2022). Following an introduction and informed consent, subjects were shown a definition of AI to ensure that they understood what AI means. They were then randomly assigned to one of four between-subjects conditions in which they empathized with an employee receiving feedback on an important task (see AI definition and vignettes in Appendix S1). The vignettes varied regarding feedback source (human vs. AI) and valence (negative vs. positive). We developed them based on recommendations for designing vignette studies (Aguinis and Bradley, 2014) and previous AI-related research (e.g., Gaube et al., 2021; Park et al., 2021). Moreover, the four vignettes and AI definition were checked in a pretest (see pretest document at https://osf.io/3uyna).
After reading the vignette, participants completed the measures presented below and in Supplementary Table S1b. Then, they voluntarily specified their age, gender, employment status including weekly working hours and leadership responsibilities, highest academic degree, place of residence, and study access. In the end, they could register for course credit and were thanked for participating.
Social distance as subdimension of psychological distance was surveyed using an adapted version of the Inclusion of Other in the Self Scale (Aron et al., 1992). The scale consists of one item with seven images of increasingly converging circles as response options. Subjects should choose the picture that best described the perceived distance or closeness to the human or AI. Similar operationalizations proved themselves in previous research (e.g., Ahn et al., 2021; Salzmann and Grasha, 1991).
Feedback acceptance was measured with four items adapted from Tonidandel et al. (2002). The items were rated on a 7-point scale (1 = strongly disagree to 7 = strongly agree; Cronbach's α = 0.87).
Performance motivation was assessed via a German translation of three items by Fedor et al. (1989). Subjects indicated their agreement from 1 (strongly disagree) to 7 (strongly agree; Cronbach's α = 0.95).
Source acceptance was investigated by three items adapted from Höddinghaus et al. (2021). Responses were given from 1 (strongly disagree) to 7 (strongly agree; Cronbach's α = 0.92).
Intention to seek further feedback was examined by one item adapted from Zingoni (2017), which was based on Ashford (1986). It was rated from 1 (strongly disagree) to 7 (strongly agree).
Additional measures were used to investigate other mechanisms of the effects or included as control variables because studies suggested their influence on the results. These were warmth, competence, power distance, attitude toward AI, knowledge about AI, experience with AI as well as with supervisors and their feedback, affinity for technology interaction, and change seeking (see Supplementary Table S1b and additional measures for justifications and details).
Calculations were made using R (version 4.2.2; R Core Team, 2022) and its integrated development environment RStudio (version 2022.7.2.576; RStudio Team, 2022). At first, a one-tailed independent samples t-test was used as a manipulation check to compare the perceived feedback valence between the groups with negative feedback and those with positive feedback. Moreover, the subjects' average immersion in the situation was inspected as an indication of the effectiveness of manipulation.
To test the effects of feedback source described in H1a, H1b, RQ1a, and RQ1b, four simple linear regressions were calculated. All of them contained the feedback source as predictor and, depending on the effect investigated, feedback acceptance, performance motivation, source acceptance, or intention to seek further feedback as criterion. Using the PROCESS macro (Hayes, 2022), a mediation analysis was conducted for H2 to investigate the assumed mechanism of social distance. More precisely, feedback source was the independent variable, social distance the mediator, and feedback acceptance the dependent variable. For the influence of feedback valence presented in H3, H4, RQ2a, and RQ2b, four multiple linear regressions were computed. Feedback source, feedback valence, and their interaction were included as predictors and either feedback acceptance, performance motivation, source acceptance, or intention to seek further feedback as criterion.
We dummy coded the independent variables for all analyses. For feedback source, a value of 0 indicated the human and of 1 the AI. Regarding feedback valence, a value of 0 referred to negative and of 1 to positive feedback. An alpha of 0.05 was applied to the analyses as significance level. Before performing the regression analyses, their requirements and the descriptive values of the dependent variables were also checked (see Appendix S2 for requirements and Supplementary Table S5a for descriptive statistics and correlations of all variables). Pointing to relevant results of these analyses, the regression results of the effects for the hypotheses and research questions are presented below (see Appendix S3 for complete regression models).
Additional analyses such as the correction for multiple comparisons, inclusion of control variables, and further mediation models are described afterwards. As the control variables were the only items that were not mandatory, missing data only occurred when they were included. The specific sample sizes after listwise exclusion are presented together with the respective analyses.
Testing the feedback valence manipulation, feedback was perceived as more negative in the conditions with negative (M = 1.7, SD = 1.0) than in those with positive feedback (M = 5.7, SD = 1.4), t(264.77) = −28.07, p < 0.001, d = −3.26. To ensure dependable results, individuals who experienced the feedback as neutral, negative feedback as positive, and positive feedback as negative were nevertheless not considered (n = 22). Likewise, as the sample only contained participants who correctly stated the feedback source, a successful manipulation of the second independent variable was guaranteed. Also, participants were able to put themselves in the situations well (M = 5.3, SD = 1.3).
Subjects accepted feedback from the AI (M = 4.0, SD = 1.5) descriptively but not significantly less than from the human (M = 4.4, SD = 1.6), b = −0.36, t(261) = −1.85, p = 0.065, f = 0.11. H1a, which predicted lower acceptance for AI than human feedback, was therefore not supported. However, excluding influential outliers led to the effect becoming significant, p = 0.047 (see Supplementary Table S2f). Providing support for H1b, feedback from the AI (M = 3.6, SD = 1.6) motivated participants less to perform better than that from the human (M = 4.1, SD = 1.9), b = −0.48, t(261) = −2.25, p = 0.025, f = 0.14.
Since an indirect effect can occur despite a non-significant total effect, the latter should not be a prerequisite for testing mediation (Hayes, 2022; Zhao et al., 2010). Therefore, the mediation analysis for H2 was conducted although feedback source did not significantly affect feedback acceptance (see Figure 1). Supporting H2a, the AI (M = 6.3, SD = 0.9) was perceived as more socially distant than the human (M = 5.8, SD = 1.2). In addition, the negative relationship between social distance and feedback acceptance corresponded to H2b. Accounting for the impact of social distance on feedback acceptance, there was no significant effect of feedback source on feedback acceptance. The confidence interval of the indirect effect, which was based on 5,000 bootstrap samples, was completely below zero, b = −0.31, 95% CI (−0.50, −0.14). This provided evidence for a mediating influence of social distance on the effect of feedback source on feedback acceptance and thus for H2.
Figure 1. Mediation effect of social distance on the relationship between feedback source and feedback acceptance for study 1.
In line with H3 and H4, we found interaction effects of feedback source and valence on feedback acceptance, b = −0.88, t(259) = −3.21, p = 0.002, f = 0.20, and performance motivation b = −1.27, t(259) = −3.49, p < 0.001, f = 0.22. As shown in Figure 2, negative feedback resulted in slightly higher feedback acceptance and performance motivation with the AI than human. Hence, the results correspond to H3a and H4a, which assumed that negative feedback is more accepted and lead to more performance motivation when provided by an AI than a human. However, positive feedback was more accepted and had a higher motivational effect when given by the human than AI. Thereby, H3b and H4b were supported.
Figure 2. Interaction effects of feedback source and feedback valence on (A) feedback acceptance and (B) performance motivation for study 1.
Regarding RQ1, the human (M = 4.6, SD = 1.5) was more accepted than the AI feedback source (M = 3.8, SD = 1.4), b = −0.83, t(261) = −4.58, p < 0.001, f = 0.28. Likewise, subjects were more likely to ask the human (M = 4.6, SD = 1.8) for further feedback than the AI (M = 3.0, SD = 1.6), b = −1.66, t(261) = −7.83, p < 0.001, f = 0.48. Thus, RQ1a and RQ1b can be affirmed since both dependent variables differed between feedback sources.
Interaction effects of feedback source and valence were significant for source acceptance, b = −1.25, t(259) = −4.25, p < 0.001, f = 0.26, and intention to seek further feedback, b = −0.81, t(259) = −1.98, p = 0.049, f = 0.12. Figure 3 illustrates that the human was more accepted and triggered a higher intention to seek further feedback than the AI and that this effect was more pronounced for positive feedback than negative feedback. For both valence conditions, the differences between the human and AI were greater in intention to seek further feedback than in source acceptance. Due to the observed interactions, RQ2a and RQ2b can be affirmed. However, the interaction of feedback source and valence on intention to seek further feedback was not significant when outliers were excluded, p = 0.050 (see Supplementary Table S2f).
Figure 3. Interaction effects of feedback source and feedback valence on (A) source acceptance and (B) intention to seek further feedback for study 1.
Due to multiple testing, we adjusted the p-values of the effects for the hypotheses and research questions with the Benjamini-Hochberg correction (Benjamini and Hochberg, 1995), which has a higher power compared to other corrections (e.g., Aickin and Gensler, 1996; Verhoeven et al., 2005). Only the interaction of feedback source and valence on intention to seek further feedback became non-significant, p = 0.060 (see Appendix S3).
Moreover, attitude toward AI, knowledge about AI, experience with AI and feedback, affinity for technology interaction, change seeking, as well as age and gender were considered as predictors in the regressions and as covariates in the mediation. Age and gender were additionally integrated because research indicated their influence on technology acceptance and its perceived input quality (e.g., Gaube et al., 2021; Venkatesh et al., 2003). The interaction of feedback source and valence on intention to seek further feedback was no longer significant, p = 0.301. The other effects, including the indirect effect, b = −0.36, 95% CI (−0.59, −0.15), changed only marginally (see Appendix S3).
Further investigating the relationship between feedback source and feedback acceptance, warmth, competence, and power distance were each used as mediators instead of social distance. The indirect effect for warmth was significant, b = −0.79, 95% CI (−1.04, −0.55), in that the human was perceived as warmer than the AI, and warmth positively influenced feedback acceptance. However, the direct effect of feedback source on feedback acceptance was positive. For competence, no difference between feedback sources and no mediation was found, b = −0.12, 95% CI (−0.32, 0.07). Furthermore, the AI was perceived as having less power than the human, but this did not mediate the relationship between feedback source and feedback acceptance, b = 0.07, 95% CI (−0.12, 0.27). The detailed results are shown in Supplementary Figures S6a–c.
Social distance was also tested as mediator of feedback source on performance motivation, source acceptance, and intention to seek further feedback. A negative mediation was evident for performance motivation, b = −0.31, 95% CI (−0.50, −0.15), source acceptance, b = −0.26, 95% CI (−0.42, −0.12), and intention to seek further feedback, b = −0.17, 95% CI (−0.31, −0.06) (see Supplementary Figures S6d–f).
Regarding the FPM, it was lastly tested whether the observed effect of feedback source on performance motivation was mediated by feedback acceptance. Although feedback acceptance significantly and positively influenced performance motivation, this was not confirmed, b = −0.25, 95% CI (−0.52, 0.02) (see Supplementary Figure S6g).
First, results showed that feedback from an AI motivated less to perform better than feedback from a human but was accepted to the same extent when outliers were not excluded. Still, social distance mediated the effect of feedback source on feedback acceptance in that the AI was perceived as more socially distant than the human, and that social distance negatively related to feedback acceptance. Second, while positive feedback was more accepted and resulted in higher performance motivation from a human than an AI, the effects slightly reversed for negative feedback. Third, the AI feedback provider itself was less accepted and caused a lower intention to seek further feedback than the human. Both effects were also moderated by feedback valence, being smaller for negative than positive feedback. However, when influential outliers were excluded, moderation was no longer significant for intention to seek further feedback. To test the generalizability of the results, a direct replication was conducted with employees only, who may better empathize with feedback situations due to their professional experience.
An online platform of a German university for employees was used to recruit participants (see Supplementary Table S1a). The participation requirements, incentive, and ethical considerations were the same as in the first study.
Five hundred thirty subjects finished the questionnaire and correctly responded to the feedback source and attention check item. Three participants were omitted because their response time was more than two standard deviations shorter than the mean. In addition, 23 subjects were not included who did not sufficiently immerse themselves in the situation and 55 participants who perceived the feedback valence differently. Again, including these 55 participants in the analyses for H1, H2, and RQ1 only changed the effects marginally. Thus, all results were calculated based on responses of 449 subjects. Specifically, 114 participants got negative and 122 positive feedback from a human, whereas 116 got negative and 97 positive feedback from an AI. The sample size was larger than the needed number of 227 subjects already computed for the first study (see preregistration at https://osf.io/bna2z for computations).
Participants mostly assigned themselves to the female gender (68.15% female, 31.18% male, 0.67% no indication) and indicated ages from 19 to 49 years with an average of 26.13 years (SD = 4.63). Most subjects stated being an employee (69.04%) or student (22.49%) due to their dual role as employee and student. However, as the university used for recruitment only offers part-time studies accompanying work and 99.11% of the participants reported accessing the study via the university platform, we could be sure that almost all subjects were employed. Supplementary Table S1a presents further information on the sample.
The procedure, measures, and data analyses were consistent with the first study. Also, internal consistencies of the main dependent variables were similar to those of the first study (Cronbach's α = 0.90 for feedback acceptance, α = 0.95 for performance motivation, and α = 0.93 for source acceptance). Reliabilities of the additional measures are described in Appendix S1.
Requirements for regression analyses were mostly fulfilled, and subsequent calculations did not alter the results decisively (see Appendix S2). With reference to important results of these computations, the effects for the hypotheses and research questions are described below (see Appendix S4 for complete models). Supplementary Table S5b presents the descriptive statistics and correlations of all variables.
Regarding the feedback valence manipulation, feedback was rated as more negative in the negative feedback conditions (M = 1.9, SD = 1.1) than in the positive feedback ones (M = 5.7, SD = 1.3), t(508.18) = −36.29, p < 0.001, d = −3.16. Also, a successful manipulation of the feedback source was realized by only considering participants who correctly indicated the source. Furthermore, subjects immersed themselves in the situation well (M = 5.0, SD = 1.3).
Contrary to the first study, H1a was also supported without excluding outliers in that participants accepted feedback from the AI (M = 3.9, SD = 1.6) less than from the human (M = 4.3, SD = 1.5), b = −0.47, t(447) = −3.24, p = 0.001, f = 0.15. Likewise, feedback from the AI (M = 3.7, SD = 1.6) motivated participants less than from the human (M = 4.2, SD = 1.6), b = −0.49, t(447) = −3.27, p = 0.001, f = 0.15. Consequently, support was provided for H1b as in the first study.
The mediation analysis of H2 is illustrated in Figure 4. In line with H2a, the AI (M = 6.0, SD = 1.3) was perceived as more socially distant than the human (M = 5.7, SD = 1.4). Furthermore, social distance correlated negatively with feedback acceptance as H2b assumed. Different from the first study, AI feedback was less accepted than human feedback when the effect of social distance on feedback acceptance was considered. However, this was only the case when outliers were not excluded, p = 0.061 (see Supplementary Table S2f). Support for the mediation of social distance on the relationship between feedback source and feedback acceptance was again given by the indirect effect, b = −0.19, 95% CI (−0.34, −0.05).
Figure 4. Mediation effect of social distance on the relationship between feedback source and feedback acceptance for study 2.
Opposed to the first study, significant interaction effects of feedback source and valence on feedback acceptance and performance motivation (H3 and H4) were not found, b = 0.07, t(445) = 0.39, p = 0.699, f = 0.02 for feedback acceptance and b = −0.01, t(445) = −0.03, p = 0.974, f = 0.00 for performance motivation. Figure 5 visualizes that negative feedback led to higher feedback acceptance and performance motivation when provided from the human than AI. Thus, H3a and H4a were not supported because they implicated that negative feedback should be more accepted and result in more performance motivation when received from an AI than a human. Yet, the fact that positive feedback from the human was also more accepted and motivated to a higher extent than that by the AI, was in line with H3b and H4b.
Figure 5. Interaction effects of feedback source and feedback valence on (A) feedback acceptance and (B) performance motivation for study 2.
Participants accepted the human (M = 4.7, SD = 1.4) to a higher extent than the AI feedback source (M = 3.7, SD = 1.5), b = −0.94, t(447) = −7.02, p < 0.001, f = 0.33. They were also more likely to request more feedback from the human (M = 5.0, SD = 1.6) than the AI (M = 3.1, SD = 1.7), b = −1.90, t(447) = −12.26, p < 0.001, f = 0.58. As in the first study, RQ1a and RQ1b can thus be affirmed because source acceptance and intention to seek further feedback varied between feedback sources.
However, no interactions of feedback source and valence were found for source acceptance, b = −0.12, t(445) = −0.58, p = 0.560, f = 0.03, and intention to seek further feedback, b = −0.08, t(445) = −0.26, p = 0.799, f = 0.01. Figure 6 shows that negative and positive feedback from the human received higher ratings than that from the AI, particularly for intention to seek further feedback. Contrary to the first study, RQ2a and RQ2b can therefore not be answered in the affirmative since interactions between feedback source and valence were not evident.
Figure 6. Interaction effects of feedback source and feedback valence on (A) source acceptance and (B) intention to seek further feedback for study 2.
The Benjamini-Hochberg correction (Benjamini and Hochberg, 1995) altered the p-values only marginally (see Appendix S4). Furthermore, the same control and demographic variables as in the first study were integrated into the analyses, resulting in minor changes of the relevant effects (see Appendix S4). Likewise, all additional mediation analyses showed results comparable to the first study (see Appendix S6). However, feedback acceptance mediated the effect of feedback source on performance motivation significantly, b = −0.29, 95% CI (−0.46, −0.11).
The second study showed that feedback from an AI was accepted and motivated less than that from a human. As in the first study, social distance mediated the relationship between feedback source and feedback acceptance. Likewise, positive feedback from a human was more accepted and led to higher performance motivation than that from an AI. However, similar differences between feedback sources were also shown for negative feedback and no interaction effects between feedback source and valence occurred. Results resembled the first study in that the AI feedback provider was less accepted and triggered a lower intention to seek further feedback than the human. Nevertheless, these effects were not moderated by feedback valence.
The present research investigated how feedback by an AI is perceived compared to that from a human supervisor and how the feedback valence influences this experience. Across two studies, AI compared to human feedback led to lower performance motivation, acceptance of the feedback provider, and intention to seek further feedback. Additionally, it was less accepted in the second study and after excluding outliers, also in the first study. All these effects were mediated by perceived social distance. For feedback acceptance and performance motivation, differences between the human and AI were no longer present in case of negative compared to positive feedback in the first study. Since the findings partly support and partly undermine the theoretical framework based on CLT and the FPM, the question of possible explanations arises.
The lower acceptance of AI than human feedback was consistent with our expectations and previous findings (e.g., Tong et al., 2021; Edwards et al., 2021). The results showed the assumed algorithm aversion (i.e., the preference for human over algorithmic input; De Cremer, 2019) and suggest that the high quality of AI messages (Tong et al., 2021; Tsai et al., 2022) seems to not yet have reached people's awareness. Moreover, the mediation of the relationship between feedback source and acceptance by social distance supports the theoretical derivation based on CLT. As in prior studies, mediation coefficients revealed an influence of feedback source on social distance (Ahn et al., 2021; Li and Sung, 2021) and a negative relationship between social distance and feedback acceptance (Ahn et al., 2021). Overall, we found an indirect-only mediation providing evidence for the theoretical framework and absence of omitted mediators (Zhao et al., 2010).
Alongside social distance, prior research suggests other mechanisms influencing the relationship between feedback source and acceptance. First, according to the FPM, the expertise of the feedback provider is an antecedent of feedback acceptance (Ilgen et al., 1979). This was also underpinned for algorithmic predictions (Kaju, 2020). Although work evaluation should require human skills (Lee, 2018), feedback sources were not judged to be differentially competent in our studies and due to no mediating influence, competence does not seem to have contributed decisively to the feedback accuracy ratings. Still, the less reliable competence measurement calls for further investigation. Second, results support that an AI is perceived as less warm and powerful than a human (Caić et al., 2020), which might drive evaluations of AI inputs and feedback responses (Ilgen et al., 1979; Zhu and Chang, 2020). Yet, power distance may be neglected as a mechanism because of no mediating influence on feedback acceptance. Concerning warmth, a suppressor effect emerged in that the direct in contrast to the total effect reversed and in the first study, also became significant (MacKinnon et al., 2000). Thus, the indirect effect should not be taken as evidence for mediation. Social distance therefore seems to be the most crucial driver for the acceptance of AI feedback compared to warmth, power distance, and competence. This underpins the applicability of CLT-related effects to AI feedback and presents CLT as a new and promising research perspective.
Congruent with feedback acceptance, the lower motivational effect of AI than human feedback reflects findings in which human feedback providers induced higher performance than AI feedback providers (Tong et al., 2021; Lopes, 2021). This effect was small but may still have emerged because the leader's social and emotional intelligence is particularly relevant for employees' motivation (Njoroge and Yazdanifard, 2014). However, such intelligence is commonly not ascribed to AI (Gladden, 2016). In our studies, social distance should have been influenced by, but not limited to emotional and social evaluations of the AI (Liviatan et al., 2008), thus necessitating additional research. Nevertheless, the mediation of performance motivation effects by social distance emphasizes the importance of the latter for responses to AI feedback.
In sum, the findings partly support the FPM. On the one hand, feedback acceptance and performance motivation, or rather the intended response construct of the model, were positively related according to the theorized feedback process and previous findings (e.g., Kinicki et al., 2004; Ilgen et al., 1979). In addition, feedback source influenced feedback acceptance and performance motivation. On the other hand, also based on Tong et al. (2021), feedback acceptance was expected to mediate the effect of feedback source on performance motivation. This was shown in the second study but not in the first study. However, the latter results may be due to omitted mediators of the model which should intermediate between feedback acceptance and intended response (Ilgen et al., 1979; Zhao et al., 2010). Based on our studies, the FPM can hence be deemed to be largely applicable to feedback delivery through AI.
Regarding feedback valence, the results underpin that negative feedback is less accepted and rather dismissed than positive feedback (e.g., Anseel and Lievens, 2006; Elder et al., 2022). Moreover, as expected due to stronger self-protection motives by a human, positive feedback from a human induced higher feedback acceptance and performance motivation than from an AI. Yet, only the first study pointed to the assumption of stronger self-change motives by AI, showing interactions between feedback source and valence and slightly higher ratings on both dependent variables for AI than human feedback when it was negative. Drawing on CLT, this could reflect the small impact of feedback source on social distance, which could have triggered only slightly different CLs and motives. Given that self-protection motives are usually more salient than self-change motives (Anseel and Lievens, 2006), a larger difference in CL between feedback sources might have been needed to find the effect. This assumption may be supported by the fact that the difference in social distance between the feedback sources was greater in the first study than in the second study and that an interaction effect was accordingly only found in the former. Yet, the influence of subjects' CL and motives has not been directly measured and thus no clear evidence for this CLT-based mechanism can be provided.
In line with research suggesting that people dislike AI that takes on roles such as being an employee (Akdim et al., 2021), source acceptance and intention to seek further feedback were lower for an AI than a human supervisor. Since these effects were larger than those for feedback acceptance and performance motivation, results indicate that one should distinguish between the perception of a single feedback message from an AI and of the AI in the role of a feedback provider. Similarly, social distance had a smaller mediating effect especially on intention to seek further feedback. Possibly, thinking of an AI as one's feedback provider and future feedback from it rendered concerns such as displacement and monitoring fears more salient and thus led to stronger effects than evaluating one feedback message (Tong et al., 2021; Akdim et al., 2021). Consequently, effects of AI feedback should not only be distinguished in terms of message- and role-accentuating evaluations but may also have differently weighted mechanisms.
Source acceptance was similarly influenced by feedback valence as were feedback acceptance and performance motivation in the first study. However, intention to seek further feedback was in both studies clearly higher for positive and negative feedback when the feedback was received from a human rather than an AI. On the one hand, this reflects that intention to seek further feedback showed a higher tendency toward algorithm aversion (De Cremer, 2019). One reason might be that intention to seek further feedback was the most behavior-related variable in that it asked for a proactive behavior toward the AI. Correspondingly, research lately showed that perceptions of and behavioral intentions toward algorithms should be distinguished (Renier et al., 2021). On the other hand, the non-existent moderating impact of feedback valence on intention to seek further feedback is consistent with the low mediating influence of social distance on it. Referring to CLT, participants' CLs and motives thus could have played a subordinate role in their responses. Nevertheless, it can be seen as support for CLT that the strength of the mediation corresponded to the magnitude of the moderation effect for all dependent variables.
From the results, practical implications can be derived for the implementation of AI feedback in the workplace. While positive feedback from a human should be more accepted by employees and motivate them more to perform better than feedback from an AI, such differences may be less pronounced for negative feedback. These effects should be largely independent of employees' attitudes, experiences, and characteristics, but may be dependent on different perceptions of social distance between humans and AI. Consequently, the results suggest that an AI might take over the unpleasant task of giving negative feedback (Gloor et al., 2020) rather than providing positive feedback. The pleasant task of giving positive feedback should be performed by a human to achieve high levels of acceptance, motivation, and likely performance (Ilgen et al., 1979; Kinicki et al., 2004). Still, it should be tested whether the moderation by feedback valence changes based on the perceived social distance and whether an AI providing both negative and positive feedback may overall result in lower accuracy perceptions and performance motivation as the results propose. In addition, difficulties should emerge in accepting the AI in the role of a feedback provider and asking for its feedback, which could impede implementing such AI systems and negatively affect employee performance (Anseel et al., 2015).
The studies further suggest that reducing the perceived social distance to the feedback provider should improve recipients' evaluations of it and its feedback. Hence, developers of AI systems that are used for providing feedback should consider aspects that convey feelings of social closeness, for instance, by a human-like appearance and natural sounding voice (Ahn et al., 2021; Li and Sung, 2021). In addition, companies could raise employees' familiarity with the AI by giving them the opportunity to experience the technology before and during its deployment, for example, through providing information and trial sessions (Akdim et al., 2021; Hein et al., 2023). However, an appropriate acceptance level should be aimed at and not blind trust, in which possible errors of the AI are not taken into account (Gaube et al., 2023). Nevertheless, perceptions of AI and human feedback should be modifiable by the perceived social distance of employees.
One limitation is that we used hypothetical scenarios, which can elicit different responses than natural settings (Aguinis and Bradley, 2014). To counteract this, materials were pretested and subjects excluded who poorly empathized with the situation. Moreover, a vignette study was chosen because it provides high internal validity (Aguinis and Bradley, 2014). Stronger effects should emerge in a real setting, since the feedback could then impact the recipients' self-image (Yam et al., 2022). Still, a field study should be conducted to examine whether comparable effects emerge. In addition, the cross-sectional design can be criticized since previous research suggested that aversion to AI technologies should decrease as people gain experience with them (Tong et al., 2021; Akdim et al., 2021) and understand their functioning (Hein et al., 2023). Yet, our aim was to examine initial perceptions of AI feedback and not intrapersonal changes over time, but the results can inform future research on this topic.
For external validity, it is questionable whether the predominantly German sample affected the results since AI is less prevalent in Western than Eastern countries (Yam et al., 2022). Moreover, the same feedback message might be perceived differently due to other cultural customs (Gladden, 2014). Future research should involve samples from further cultures to investigate the findings' generalizability. One might also query the transferability to other feedback contents and AI technologies. We kept the feedback and description of the AI low in detail to examine general effects of feedback source and valence. A question that now arises is thus whether a more detailed explanation of the feedback would lead to more positive perceptions of AI feedback, as implied by Gaube et al. (2023). Here, the content (what aspects) and presentation of the explanation (how aspects; Hein et al., 2023) could evoke different effects, which is why both should be varied to understand factors influencing responses to AI feedback and derive recommendations for its implementation. For example, information on the accuracy of the feedback may influence recipients' perceptions (Cecil et al., 2024). Accordingly, it could be tested whether an anthropomorphization of the AI also produces more favorable responses to feedback and not only to recommendations (Ahn et al., 2021).
Last, although the study yields insights into mechanisms of the effects and applicability of the FPM and CLT to AI feedback, it does not provide clear evidence for it. Specifically, the influence of feedback source on subjects' CL and motives was not explicitly surveyed and competence was measured with low reliability even though all scales were carefully selected. Subsequent studies could also examine other phases of the FPM which were beyond the scope of this investigation. Future research should address these issues by integrating alternative measures and further exploring the theory underlying responses to AI feedback.
This study is one of the first to investigate effects of AI feedback in the workplace. It was found that not only the perception of positive and negative feedback may be distinguished, but also that of single AI feedback messages as opposed to that of the AI in the role of a feedback provider. Namely, the AI itself was experienced more negatively than its individual message. Moreover, the FPM and CLT were found to be largely applicable to AI feedback, identifying a reduction in perceived social distance to the AI as a potential way to improve recipients' feedback evaluations. Future research should further investigate the effects of AI feedback on employees and its theoretical background to provide recommendations for the increasing implementation of AI feedback in the workplace.
The datasets presented in this study can be found in online repositories. The names of the repository/repositories and accession number(s) can be found below: the databases, codebooks, and analysis scripts of the pretest, first and second study are available on the OSF platform under https://osf.io/3uyna. The appendices and a description of the pretest and its results can also be accessed via the link.
Ethical review and approval was not required for the study on human participants in accordance with the local legislation and institutional requirements. The participants provided their written informed consent to participate in this study.
IH: Conceptualization, Data curation, Formal analysis, Investigation, Methodology, Project administration, Validation, Visualization, Writing – original draft, Writing – review & editing. JC: Writing – review & editing. EL: Conceptualization, Funding acquisition, Methodology, Supervision, Writing – review & editing.
The author(s) declare financial support was received for the research, authorship, and/or publication of this article. The research was funded by a grant from the VolkswagenStiftung (grant number: 98525). In addition, the publication fees are subsidized by the LMU Munich Library. The funders did not play any role in the study design and collection, analysis, and interpretation of the results.
Many thanks to Annabel Jünke (Institute of Management and Organization Science, Technical University Braunschweig) for reviewing the study and proofreading the written paper. This paper was previously publish as a preprint (Hein et al., 2024).
The authors declare that the research was conducted in the absence of any commercial or financial relationships that could be construed as a potential conflict of interest.
All claims expressed in this article are solely those of the authors and do not necessarily represent those of their affiliated organizations, or those of the publisher, the editors and the reviewers. Any product that may be evaluated in this article, or claim that may be made by its manufacturer, is not guaranteed or endorsed by the publisher.
The Supplementary Material for this article can be found online at: https://www.frontiersin.org/articles/10.3389/forgp.2024.1468907/full#supplementary-material
Aguinis, H., and Bradley, K. J. (2014). Best practice recommendations for designing and implementing experimental vignette methodology studies. Organ. Res. Methods 17, 351–371. doi: 10.1177/1094428114547952
Ahn, J., Kim, J., and Sung, Y. (2021). AI-powered recommendations: the roles of perceived similarity and psychological distance on persuasion. Int. J. Advert. 40, 1366–1384. doi: 10.1080/02650487.2021.1982529
Aickin, M., and Gensler, H. (1996). Adjusting for multiple testing when reporting research results: The Bonferroni vs Holm methods. Am. J. Public Health 86, 726–728. doi: 10.2105/AJPH.86.5.726
Akdim, K., Belanche, D., and Flavián, M. (2021). Attitudes toward service robots: analyses of explicit and implicit attitudes based on anthropomorphism and construal level theory. Int. J. Contemp. Hosp. Manag. 35, 2816–2837. doi: 10.1108/IJCHM-12-2020-1406
Anseel, F., Beatty, A. S., Shen, W., Lievens, F., and Sackett, P. R. (2015). How are we doing after 30 years? A meta-analytic review of the antecedents and outcomes of feedback-seeking behavior. J. Manag. 41, 318–348. doi: 10.1177/0149206313484521
Anseel, F., and Lievens, F. (2006). Certainty as a moderator of feedback reactions? A test of the strength of the self-verification motive. J. Occup. Org. Psychol. 79, 533–551. doi: 10.1348/096317905X71462
Anseel, F., and Lievens, F. (2009). The mediating role of feedback acceptance in the relationship between feedback and attitudinal and performance outcomes. Int. J. Select. Assess. 17, 362–376. doi: 10.1111/j.1468-2389.2009.00479.x
Aron, A., Aron, E. N., and Smollan, D. (1992). Inclusion of other in the self scale and the structure of interpersonal closeness. J. Pers. Soc. Psychol. 63, 596–612. doi: 10.1037/0022-3514.63.4.596
Ashford, S. J. (1986). Feedback-seeking in individual adaptation: a resource perspective. Acad. Manag. J. 29, 465–487. doi: 10.2307/256219
Avolio, B. J., Sosik, J. J., Kahai, S. S., and Baker, B. (2014). E-leadership: re-examining transformations in leadership source and transmission. Leadersh. Q. 25, 105–131. doi: 10.1016/j.leaqua.2013.11.003
Bar-Anan, Y., Liberman, N., and Trope, Y. (2006). The association between psychological distance and construal level: evidence from an implicit association test. J. Exp. Psychol. 135, 609–622. doi: 10.1037/0096-3445.135.4.609
Belding, J. N., Naufel, K. Z., and Fujita, K. (2015). Using high-level construal and perceptions of changeability to promote self-change over self-protection motives in response to negative feedback. Pers. Soc. Psychol. Bull. 41, 822–838. doi: 10.1177/0146167215580776
Benjamini, Y., and Hochberg, Y. (1995). Controlling the false discovery rate: a practical and powerful approach to multiple testing. J. R. Stat. Soc. 57, 289–300. doi: 10.1111/j.2517-6161.1995.tb02031.x
Caić, M., Avelino, J., Mahr, D., Odekerken-Schröder, G., and Bernardino, A. (2020). Robotic versus human coaches for active aging: an automated social presence perspective. Int. J. Soc. Robot. 12, 867–882. doi: 10.1007/s12369-018-0507-2
Cecil, J., Lermer, E., Hudecek, M. F. C., Sauer, J., and Gaube, S. (2024). Explainability does not mitigate the negative impact of incorrect AI advice in a personnel selection task. Sci. Rep. 14:9736. doi: 10.1038/s41598-024-60220-5
Cichor, J. E., Hubner-Benz, S., Benz, T., Emmerling, F., and Peus, C. (2023). Robot leadership-investigating human perceptions and reactions towards social robots showing leadership behaviors. PLoS ONE 18:e0281786. doi: 10.1371/journal.pone.0281786
De Cremer, D. (2019). Leading artificial intelligence at work: a matter of facilitating human-algorithm cocreation. J. Leader. Stud. 13, 81–83. doi: 10.1002/jls.21637
De Stobbeleir, K. E. M., Ashford, S. J., and Buyens, D. (2011). Self-regulation of creativity at work: the role of feedback-seeking behavior in creative performance. Acad. Manag. J. 54, 811–831. doi: 10.5465/amj.2011.64870144
Edwards, C., Edwards, A., Albrehi, F., and Spence, P. (2021). Interpersonal impressions of a social robot versus human in the context of performance evaluations. Commun. Educ. 70, 165–182. doi: 10.1080/03634523.2020.1802495
Elder, J., Davis, T., and Hughes, B. L. (2022). Learning about the self: motives for coherence and positivity constrain learning from self-relevant social feedback. Psychol. Sci. 33, 629–647. doi: 10.1177/09567976211045934
Faul, F., Erdfelder, E., Buchner, A., and Lang, A.-G. (2009). Statistical power analyses using G*Power 3.1: tests for correlation and regression analyses. Behav. Res. Methods 41, 1149–1160. doi: 10.3758/BRM.41.4.1149
Fedor, D. B., Eder, R. W., and Buckley, M. (1989). The contributory effects of supervisor intentions on subordinate feedback responses. Organ. Behav. Hum. Decis. Process. 44, 396–414. doi: 10.1016/0749-5978(89)90016-2
Förster, J., Liberman, N., and Shapira, O. (2009). Preparing for novel versus familiar events: shifts in global and local processing. J. Exp. Psychol. 138, 383–399. doi: 10.1037/a0015748
Freitas, A. L., Salovey, P., and Liberman, N. (2001). Abstract and concrete self-evaluative goals. J. Pers. Soc. Psychol. 80, 410–424. doi: 10.1037/0022-3514.80.3.410
Gaube, S., Suresh, H., Raue, M., Lermer, E., Koch, T. K., Hudecek, M. F. C., et al. (2023). Non-task expert physicians benefit from correct explainable AI advice when reviewing X-rays. Sci. Rep. 13:1383. doi: 10.1038/s41598-023-28633-w
Gaube, S., Suresh, H., Raue, M., Merritt, A., Berkowitz, S. J., Lermer, E., et al. (2021). Do as AI say: susceptibility in deployment of clinical decision-aids. npj Dig. Med. 4:31. doi: 10.1038/s41746-021-00385-9
Gladden, M. E. (2014). The social robot as ‘charismatic leader': a phenomenology of human submission to nonhuman power. Soc. Robots Fut. Soc. Relat. 273, 329–339. doi: 10.3233/978-1-61499-480-0-329
Gladden, M. E. (2016). Posthuman management: creating effective organizations in an age of social robotics, ubiquitous AI, human augmentation, and virtual worlds. 2nd Edn. Indianapolis, IN: Defragmenter Media.
Gloor, J. L., Howe, L., De Cremer, D., and Yam, K. C. (2020). The funny thing about robot leadership. Alphen aan den Rijn: Kluwer Law International.
Greller, M. M. (1978). The nature of subordinate participation in the appraisal interview. Acad. Manag. J. 21, 646–658. doi: 10.2307/255705
Harms, P. D., and Han, G. (2019). Algorithmic leadership: the future is now. J. Leader. Stud. 12, 74–75. doi: 10.1002/jls.21615
Hayes, A. F. (2022). Introduction to mediation, moderation, and conditional process analysis: a regression-based approach. 3rd Edn. New York, NY; London: The Guilford Press.
Hein, I., Cecil, J., and Lermer, E. (2024). Acceptance and motivational effect of AI-driven feedback in the workplace: an experimental study with direct replication. OSF. 1–34. doi: 10.31219/osf.io/uczaw
Hein, I., Diefenbach, S., and Ullrich, D. (2023). Designing for technology transparency – transparency cues and user experience. Usability Prof. 23, 1–5. doi: 10.18420/muc2023-up-448
Höddinghaus, M., Sondern, D., and Hertel, G. (2021). The automation of leadership functions: would people trust decision algorithms? Comput. Human Behav. 116:106635. doi: 10.1016/j.chb.2020.106635
Hou, Y. T.-Y., and Jung, M. F. (2018). Robots in power. Proc. Longit. Hum. Robot Teaming 2018, 325–338. Available at: https://interplay.infosci.cornell.edu/assets/papers/power.pdf
Hubner, S., Benz, T., and Peus, C. (2019). Chancen und Herausforderungen beim Einsatz von Robotern in Führungsrollen. Pers. Q. 71, 28–34. Available at: https://hdl.handle.net/10863/15545
Ilgen, D. R., Fisher, C. D., and Taylor, M. S. (1979). Consequences of individual feedback on behavior in organizations. J. Appl. Psychol. 64, 349–371. doi: 10.1037/0021-9010.64.4.349
Kaju, A. (2020). Technologically yours: how new technologies affect psychological distance (dissertation). University of Toronto. Available at: https://hdl.handle.net/1807/100922 (accessed December 7, 2022).
Kinicki, A. J., Prussia, G. E., Wu, B. J., and McKee-Ryan, F. M. (2004). A covariance structure analysis of employees' response to performance feedback. J. Appl. Psychol. 89, 1057–1069. doi: 10.1037/0021-9010.89.6.1057
Lee, M. K. (2018). Understanding perception of algorithmic decisions: fairness, trust, and emotion in response to algorithmic management. Big Data Soc. 5, 1–16. doi: 10.1177/2053951718756684
Li, X., and Sung, Y. (2021). Anthropomorphism brings us closer: the mediating role of psychological distance in user–AI assistant interactions. Comput. Human Behav. 118:106680. doi: 10.1016/j.chb.2021.106680
Liviatan, I., Trope, Y., and Liberman, N. (2008). Interpersonal similarity as a social distance dimension: implications for perception of others' actions. J. Exp. Soc. Psychol. 44, 1256–1269. doi: 10.1016/j.jesp.2008.04.007
Lopes, S. (2021). Towards a socio cognitive perspective of presenteeism, leadership and the rise of robotic interventions in the workplace (dissertation). University of Lisbon. Available at: http://hdl.handle.net/10071/25643 (accessed December 12, 2022).
MacKinnon, D. P., Krull, J. L., and Lockwood, C. M. (2000). Equivalence of the mediation, confounding and suppression effect. Prev. Sci. 1, 173–181. doi: 10.1023/A:1026595011371
Njoroge, C. N., and Yazdanifard, R. (2014). The impact of social and emotional intelligence on employee motivation in a multigenerational workplace. Glob. J. Manag. Bus. Res. 14, 31–36. Available at: https://www.proquest.com/scholarly-journals/impact-social-emotional-intelligence-on-employee/docview/1552838299/se-2
Park, H., Ahn, D., Hosanagar, K., and Lee, J. (2021). “Human-AI interaction in human resource management: understanding why employees resist algorithmic evaluation at workplaces and how to mitigate burdens,” in CHI '21: Proceedings of the 2021 CHI Conference on Human Factors in Computing Systems (New York, NY: ACM), 1–15.
Prahl, A., and van Swol, L. (2017). Understanding algorithm aversion: when is advice from automation discounted? J. Forecast. 36, 691–702. doi: 10.1002/for.2464
R Core Team (2022). R: a language and environment for statistical computing. Version 4.2.2 [software]. Vienna: The R Foundation.
Renier, L. A., Schmid Mast, M., and Bekbergenova, A. (2021). To err is human, not algorithmic – robust reactions to erring algorithms. Comput. Human Behav. 124:106879. doi: 10.1016/j.chb.2021.106879
RStudio Team (2022). RStudio: integrated development environment for R. Version 2022.7.2.576 [software]. Boston, MA: Posit.
Salzmann, J., and Grasha, A. F. (1991). Psychological size and psychological distance in manager-subordinate relationships. J. Soc. Psychol. 131, 629–646. doi: 10.1080/00224545.1991.9924647
Tong, S., Jia, N., Luo, X., and Fang, Z. (2021). The janus face of artificial intelligence feedback: deployment versus disclosure effects on employee performance. Strat. Manag. J. 42, 1600–1631. doi: 10.1002/smj.3322
Tonidandel, S., Quiñones, M. A., and Adams, A. A. (2002). Computer-adaptive testing: the impact of test characteristics on perceived performance and test takers' reactions. J. Appl. Psychol. 87, 320–332. doi: 10.1037/0021-9010.87.2.320
Trope, Y., and Liberman, N. (2010). Construal-level theory of psychological distance. Psychol. Rev. 117, 440–463. doi: 10.1037/a0018963
Tsai, C.-Y., Marshall, J. D., Choudhury, A., Serban, A., Tsung-Yu Hou, Y., Jung, M. F., et al. (2022). Human-robot collaboration: a multilevel and integrated leadership framework. Leadersh. Q. 33:101594. doi: 10.1016/j.leaqua.2021.101594
Venkatesh, V., and Bala, H. (2008). Technology acceptance model 3 and a research agenda on interventions. Decis. Sci. 39, 273–315. doi: 10.1111/j.1540-5915.2008.00192.x
Venkatesh, V., Morris, M. G., Davis, G. B., and Davis, F. D. (2003). User acceptance of information technology: toward a unified view. MIS Q. 27, 425–478. doi: 10.2307/30036540
Verhoeven, K. J., Simonsen, K. L., and McIntyre, L. M. (2005). Implementing false discovery rate control: increasing your power. Oikos 108, 643–647. doi: 10.1111/j.0030-1299.2005.13727.x
Wesche, J. S., and Sonderegger, A. (2019). When computers take the lead: the automation of leadership. Comput. Human Behav. 101, 197–209. doi: 10.1016/j.chb.2019.07.027
Wirtz, J., Patterson, P. G., Kunz, W. H., Gruber, T., Lu, V. N., Paluch, S., et al. (2018). Brave new world: service robots in the frontline. J. Serv. Manag. 29, 907–931. doi: 10.1108/JOSM-04-2018-0119
Yam, K. C., Goh, E.-Y., Fehr, R., Lee, R., Soh, H., and Gray, K. (2022). When your boss is a robot: workers are more spiteful to robot supervisors that seem more human. J. Exp. Soc. Psychol. 102:104360. doi: 10.1016/j.jesp.2022.104360
Zhang, L., Yang, J., Zhang, Y., and Xu, G. (2023). Gig worker's perceived algorithmic management, stress appraisal, and destructive deviant behavior. PLoS ONE 18:e0294074. doi: 10.1371/journal.pone.0294074
Zhao, X., Lynch, J. G., and Chen, Q. (2010). Reconsidering Baron and Kenny: myths and truths about mediation analysis. J. Cons. Res. 37, 197–206. doi: 10.1086/651257
Zhu, D. H., and Chang, Y. P. (2020). Robot with humanoid hands cooks food better? Int. J. Contemp. Hosp. Manag. 32, 1367–1383. doi: 10.1108/IJCHM-10-2019-0904
Keywords: artificial intelligence, leadership, automated leadership, feedback, construal level theory, feedback process model
Citation: Hein I, Cecil J and Lermer E (2024) Acceptance and motivational effect of AI-driven feedback in the workplace: an experimental study with direct replication. Front. Organ. Psychol. 2:1468907. doi: 10.3389/forgp.2024.1468907
Received: 20 August 2024; Accepted: 06 December 2024;
Published: 23 December 2024.
Edited by:
Osman Titrek, Sakarya University, TürkiyeReviewed by:
MeiPeng Low, Tunku Abdul Rahman University, MalaysiaCopyright © 2024 Hein, Cecil and Lermer. This is an open-access article distributed under the terms of the Creative Commons Attribution License (CC BY). The use, distribution or reproduction in other forums is permitted, provided the original author(s) and the copyright owner(s) are credited and that the original publication in this journal is cited, in accordance with accepted academic practice. No use, distribution or reproduction is permitted which does not comply with these terms.
*Correspondence: Ilka Hein, aWxrYS5oZWluQHBzeS5sbXUuZGU=
Disclaimer: All claims expressed in this article are solely those of the authors and do not necessarily represent those of their affiliated organizations, or those of the publisher, the editors and the reviewers. Any product that may be evaluated in this article or claim that may be made by its manufacturer is not guaranteed or endorsed by the publisher.
Research integrity at Frontiers
Learn more about the work of our research integrity team to safeguard the quality of each article we publish.