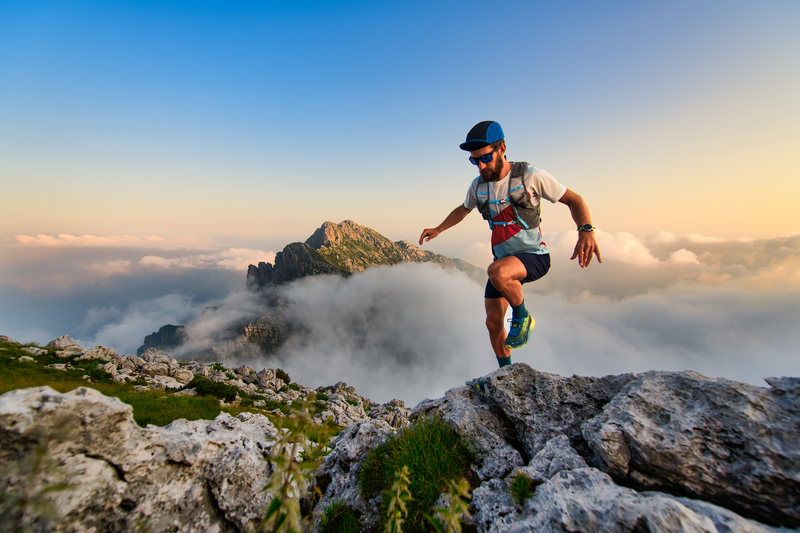
94% of researchers rate our articles as excellent or good
Learn more about the work of our research integrity team to safeguard the quality of each article we publish.
Find out more
ORIGINAL RESEARCH article
Front. Oncol.
Sec. Pharmacology of Anti-Cancer Drugs
Volume 15 - 2025 | doi: 10.3389/fonc.2025.1570647
This article is part of the Research Topic Demystifying Fundamental Mechanisms of anticancer drug resistance in Gastrointestinal Oncology View all articles
The final, formatted version of the article will be published soon.
You have multiple emails registered with Frontiers:
Please enter your email address:
If you already have an account, please login
You don't have a Frontiers account ? You can register here
The tumor microenvironment (TME) plays a critical role in the development, progression, and clinical outcomes of hepatocellular carcinoma (HCC).Despite the critical role of natural killer (NK) cells in tumor immunity, there is limited research on their status within the tumor microenvironment of HCC. In this study, single-cell RNA sequencing (scRNA-seq) analysis of HCC datasets was performed to identify potential biomarkers and investigate the involvement of natural killer (NK) cells in the TME.Methods: Single-cell RNA sequencing (scRNA-seq) data were extracted from the GSE149614 dataset and processed for quality control using the "Seurat" package.HCC subtypes from the TCGA dataset were classified through consensus clustering based on differentially expressed genes (DEGs). Weighted gene co-expression network analysis (WGCNA) was employed to construct co-expression networks.Furthermore, univariate and multivariate Cox regression analyses were conducted to identify variables linked to overall survival. The single-sample gene set enrichment analysis (ssGSEA) was used to analyze immune cells and the screened genes.Result: A total of 715 DEGs from GSE149614 and 864 DEGs from TCGA were identified, with 25 overlapping DEGs found between the two datasets. A prognostic risk score model based on two genes was then established. Significant differences in immune cell infiltration were observed between high-risk and low-risk groups.Immunohistochemistry showed that HRG expression was decreased in HCC compared to normal tissues, whereas TUBA1B expression was elevated in HCC.Our study identified a two-gene prognostic signature based on NK cell markers and highlighted their role in the TME, which may offer novel insights in immunotherapy strategies. Additionally, we developed an accurate and reliable prognostic model, combining clinical factors to aid clinicians in decision-making.
Keywords: Hepatocellular Carcinoma, Natural kill cell, Tumor Microenvironment, nomogram, prognosis, TUBA1B, single-cell
Received: 04 Feb 2025; Accepted: 04 Mar 2025.
Copyright: © 2025 Li, Liu, Zhang, Yin, Yuan, Xie and Ding. This is an open-access article distributed under the terms of the Creative Commons Attribution License (CC BY). The use, distribution or reproduction in other forums is permitted, provided the original author(s) or licensor are credited and that the original publication in this journal is cited, in accordance with accepted academic practice. No use, distribution or reproduction is permitted which does not comply with these terms.
* Correspondence:
Xiaoxue Yuan, Beijing Ditan Hospital, Capital Medical University, Beijing, China
Wen Xie, Beijing Ditan Hospital, Capital Medical University, Beijing, China
Xiaoyan Ding, Beijing Ditan Hospital, Capital Medical University, Beijing, China
Disclaimer: All claims expressed in this article are solely those of the authors and do not necessarily represent those of their affiliated organizations, or those of the publisher, the editors and the reviewers. Any product that may be evaluated in this article or claim that may be made by its manufacturer is not guaranteed or endorsed by the publisher.
Research integrity at Frontiers
Learn more about the work of our research integrity team to safeguard the quality of each article we publish.