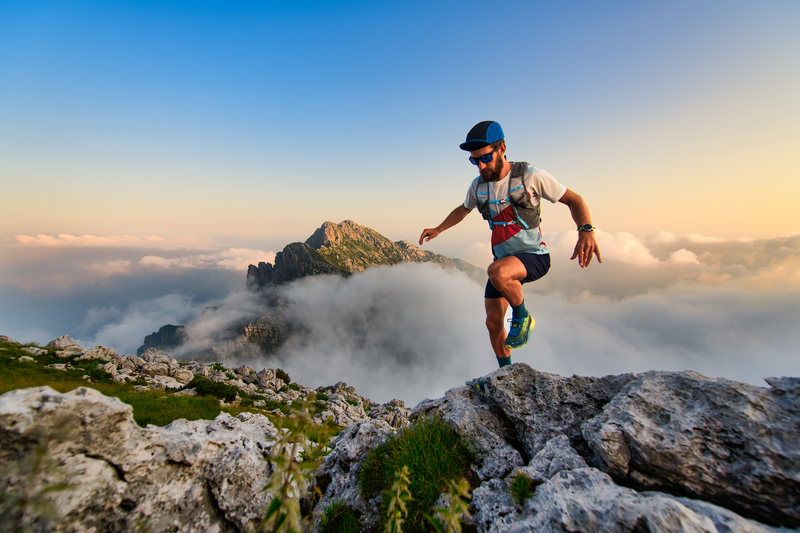
94% of researchers rate our articles as excellent or good
Learn more about the work of our research integrity team to safeguard the quality of each article we publish.
Find out more
ORIGINAL RESEARCH article
Front. Oncol.
Sec. Gastrointestinal Cancers: Hepato Pancreatic Biliary Cancers
Volume 15 - 2025 | doi: 10.3389/fonc.2025.1546940
The final, formatted version of the article will be published soon.
You have multiple emails registered with Frontiers:
Please enter your email address:
If you already have an account, please login
You don't have a Frontiers account ? You can register here
Background: This study aimed to develop and validate a predictive model integrating radiomics features and clinical variables to differentiate intrahepatic bile duct stones with cholangitis (IBDS-IL) from intrahepatic cholangiocarcinoma (ICC) preoperatively, as accurate distinction is crucial for determining appropriate treatment strategies.Methods: A total of 169 patients (97 IBDS-IL and 72 ICC) who underwent surgical resection were retrospectively analyzed. Radiomics features were extracted from ultrasound images, and clinical variables with significant differences between groups were identified. Feature selection was performed using LASSO regression and recursive feature elimination (RFE). The radiomics model, clinical model, and combined model were constructed and evaluated using the area under the curve (AUC), calibration curves, decision curve analysis (DCA), and SHAP analysis. Results: The radiomics model achieved an AUC of 0.962, and the clinical model achieved an AUC of 0.861. The combined model, integrating the Radiomics Score with clinical variables, demonstrated the highest predictive performance with an AUC of 0.988, significantly outperforming the clinical model (p < 0.05). Calibration curves showed excellent agreement between predicted and observed outcomes, and the Hosmer-Lemeshow test confirmed a good model fit (p = 0.998). DCA revealed that the combined model provided the greatest clinical benefit across a wide range of threshold probabilities. SHAP analysis identified the Radiomics Score as the most significant contributor, complemented by abdominal pain and liver atrophy. Conclusion: The combined model integrating radiomics features and clinical data offers a powerful and reliable tool for preoperative differentiation of IBDS-IL and ICC. Its superior performance and clinical interpretability highlight its potential for improving diagnostic accuracy and guiding clinical decision-making. Further validation in larger, multicenter datasets is warranted to confirm its generalizability.
Keywords: intrahepatic cholangiocarcinoma, Intrahepatic bile duct stones, Intrahepatic lithiasis, Radiomics, nomogram
Received: 17 Dec 2024; Accepted: 26 Feb 2025.
Copyright: © 2025 Qian, Huang, Dong, Xu, Chen, Zhou, Zhou, Yu and Lu. This is an open-access article distributed under the terms of the Creative Commons Attribution License (CC BY). The use, distribution or reproduction in other forums is permitted, provided the original author(s) or licensor are credited and that the original publication in this journal is cited, in accordance with accepted academic practice. No use, distribution or reproduction is permitted which does not comply with these terms.
* Correspondence:
Baochun Lu, Shaoxing People's Hospital, Shaoxing, China
Disclaimer: All claims expressed in this article are solely those of the authors and do not necessarily represent those of their affiliated organizations, or those of the publisher, the editors and the reviewers. Any product that may be evaluated in this article or claim that may be made by its manufacturer is not guaranteed or endorsed by the publisher.
Research integrity at Frontiers
Learn more about the work of our research integrity team to safeguard the quality of each article we publish.