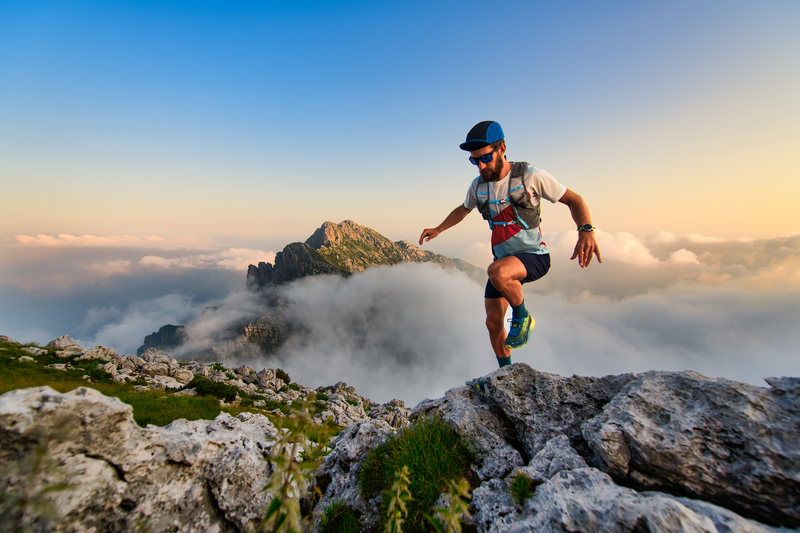
94% of researchers rate our articles as excellent or good
Learn more about the work of our research integrity team to safeguard the quality of each article we publish.
Find out more
ORIGINAL RESEARCH article
Front. Oncol.
Sec. Cancer Imaging and Image-directed Interventions
Volume 15 - 2025 | doi: 10.3389/fonc.2025.1530144
This article is part of the Research Topic Current Challenges and Future Perspectives in Neuro-Oncological Imaging View all articles
The final, formatted version of the article will be published soon.
You have multiple emails registered with Frontiers:
Please enter your email address:
If you already have an account, please login
You don't have a Frontiers account ? You can register here
Objectives To develop and validate a multiparametric prognostic model, incorporating Dynamic Contrast -Enhanced (DCE) quantitative parameters and multi-modality Radiomics features, for the accurate identification of isocitrate dehydrogenase 1 (IDH1) mutation status from glioma patients. Methods A total of 152 glioma patient data with confirmed IDH1 mutation status were retrospectively collected. A segmentation neural network was used to measure MRI quantitative parameters compared with the empirically oriented ROI selection. Radiomics Features, extracted from conventional MR images (T1CE, T2W, and ADC) and DCE quantitative parameter images, were combined with MRI quantitative parameters in our research to predict IDH1 mutation status. We constructed and analyzed clinical models 1 -2 (corresponding to manual and automatic MRI quantitative parameters), Radiomic feature models 1 -3 (corresponding to structural MRI, DCE, and multi-modality respectively), and a multivariable combined model. We tried different usual classifiers and selected logistic regression according to AUC. Five-fold cross-validation was applied for validation. Results Multivariable Combined Model showed the best prediction performance (AUC, 0.915; 95% CI: 0.87, 0.96) in the validation cohort. Multivariable Combined Model performed better than Clinical Model 1 and Radiomic Feature Model 1 (DeLong p all < 0.05), and Radiomic Feature Model 3 performed better than Radiomic Feature Model 1 (DeLong p < 0.05). Conclusions Compared with the conventional MRI Radiomics and Clinical models, the Multivariable Combined Model, mainly based on DCE quantitative parameters and multi-modality Radiomics features, is the most promising and deserves attention in the current study.
Keywords: Glioma, Dynamic contrast enhanced, Quantitative parameter, Radiomics, Logistic regression
Received: 19 Nov 2024; Accepted: 17 Feb 2025.
Copyright: © 2025 Ye, Cao, Zhu, Chen, Zhou, Yang, Li, Chen, Luan, Li, Tian, Sun, Shi, Zhang and Zhang. This is an open-access article distributed under the terms of the Creative Commons Attribution License (CC BY). The use, distribution or reproduction in other forums is permitted, provided the original author(s) or licensor are credited and that the original publication in this journal is cited, in accordance with accepted academic practice. No use, distribution or reproduction is permitted which does not comply with these terms.
* Correspondence:
Xin Zhang, Department of Radiology, Nanjing Drum Tower Hospital, Nanjing, China
Disclaimer: All claims expressed in this article are solely those of the authors and do not necessarily represent those of their affiliated organizations, or those of the publisher, the editors and the reviewers. Any product that may be evaluated in this article or claim that may be made by its manufacturer is not guaranteed or endorsed by the publisher.
Research integrity at Frontiers
Learn more about the work of our research integrity team to safeguard the quality of each article we publish.