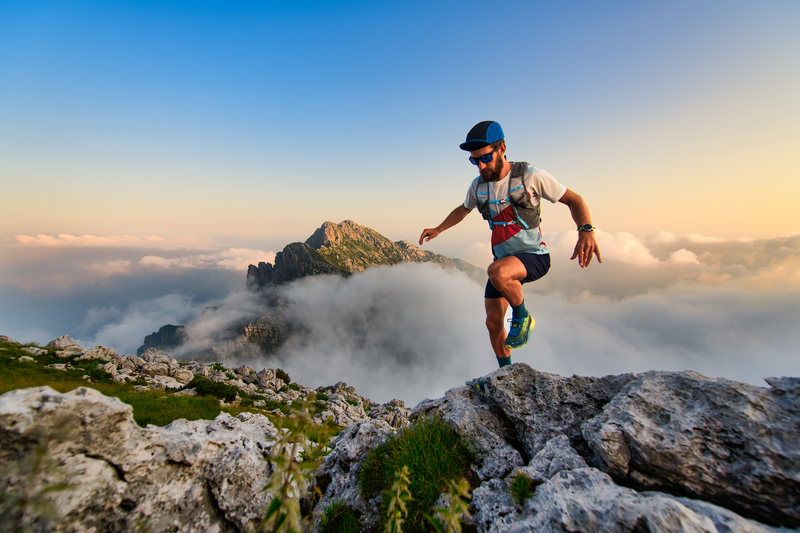
95% of researchers rate our articles as excellent or good
Learn more about the work of our research integrity team to safeguard the quality of each article we publish.
Find out more
ORIGINAL RESEARCH article
Front. Oncol. , 03 March 2025
Sec. Cancer Imaging and Image-directed Interventions
Volume 15 - 2025 | https://doi.org/10.3389/fonc.2025.1510071
Objective: To investigate the predictive value of radiomics models based on intra-tumoral ecological diversity (iTED) and temporal characteristics for assessing microvascular invasion (MVI) in patients with hepatocellular carcinoma (HCC).
Material and Methods: We retrospectively analyzed the data of 398 HCC patients who underwent dynamic contrast-enhanced MRI with Gd-EOB-DTPA (training set: 318; testing set: 80). The tumors were segmented into five distinct habitats using case-level clustering and a Gaussian mixture model was used to determine the optimal clusters based on the Bayesian information criterion to produce an iTED feature vector for each patient, which was used to assess intra-tumoral heterogeneity. Radiomics models were developed using iTED features from the arterial phase (AP), portal venous phase (PVP), and hepatobiliary phase (HBP), referred to as MiTED-AP, MiTED-PVP, and MiTED-HBP, respectively. Additionally, temporal features were derived by subtracting the PVP features from the AP features, creating a delta-radiomics model (MDelta). Conventional radiomics features were also extracted from the AP, PVP, and HBP images, resulting in three models: MCVT-AP, MCVT-PVP, and MCVT-HBP. A clinical-radiological model (CR model) was constructed, and two fusion models were generated by combining the radiomics or/and CR models using a stacking algorithm (fusion_R and fusion_CR). Model performance was evaluated using AUC, accuracy, sensitivity, and specificity.
Results: The MDelta model demonstrated higher sensitivity compared to the MCVT-AP and MCVT-PVP models. No significant differences in performance were observed across different imaging phases for either conventional radiomics (p = 0.096–0.420) or iTED features (p = 0.106–0.744). Similarly, for images from the same phase, we found no significant differences between the performance of conventional radiomics and iTED features (AP: p = 0.158; PVP: p = 0.844; HBP: p = 0.157). The fusion_R and fusion_CR models enhanced MVI discrimination, achieving AUCs of 0.823 (95% CI: 0.816–0.831) and 0.830 (95% CI: 0.824–0.835), respectively.
Conclusion: Delta radiomics features are temporal and predictive of MVI, providing additional predictive information for MVI beyond conventional AP and PVP features. The iTED features provide an alternative perspective in interpreting tumor characteristics and hold the potential to replace conventional radiomics features to some extent for MVI prediction.
Hepatocellular carcinoma (HCC) is the most common form of primary liver cancer, ranking sixth in global incidence and third in mortality rate (1). Although surgical resection and liver transplantation have been shown to be effective for HCC, the high recurrence rate remains a major factor contributing to poor overall survival (2). Microvascular invasion (MVI), characterized by the presence of cancer cell clusters within endothelial-lined vascular spaces visible under microscopy (3), is an important predictor of recurrence and reduced survival in HCC patients (4, 5) and also plays a key role in determining treatment strategies (6). Currently, MVI is typically diagnosed through the examination of postoperative surgical specimens. However, needle biopsies often have low diagnostic yields and pose a risk of tumor implantation, making the preoperative and noninvasive assessment of MVI particularly challenging.
Radiomics, which provides important insights into tumor heterogeneity and the tumor microenvironment (7), offers potential for the preoperative identification of MVI. In recent years, radiomics models based on dynamic contrast-enhanced MRI have gained attention for their potential to predict MVI (8, 9). Multi-sequence and multi-parameter radiomics models have demonstrated superior predictive capabilities compared to models based on single-sequence imaging (8). Gadolinium ethoxybenzyl diethylenetriamine pentaacetic acid-enhanced MRI (Gd-EOB-DTPA MRI) is commonly used to improve the detection and characterization of HCC in clinical practice (10–12), and it has shown utility in evaluating tumor features related to MVI (13, 14).Thus, images captured during the hepatobiliary phase (HBP) are particularly valuable for defining tumor boundaries (15).
Delta radiomics involves the evaluation of relative changes in radiomic features over time (16, 17). HCC typically exhibits arterial phase (AP) hyperenhancement followed by washout in the portal venous phase (PVP). Therefore, changes in radiomic features derived from dynamic contrast-enhanced imaging can serve as important predictive biomarkers for MVI (18). Previous studies have primarily focused on individual imaging phases, often neglecting the temporal characteristics of the tumor (19, 20). In this study, we utilized deltaradiomics by subtracting PVP features from AP features to capture temporal variations that could enhance the prediction of MVI.
Previous studies mainly extracted radiomic features from the entire tumor without adequately addressing inter-tumoral heterogeneity (21). However, we believe that radiomic habitat analysis, which uses clustering methods to identify similar voxel groupings and describe environmental habitats based on ecological and biodiversity principles (22), could provide a more detailed understanding of the heterogeneous nature of HCC. Given the highly heterogeneous nature of HCC, the quantitative characterization of distinct intra-tumoral habitats may offer valuable predictive information for MVI. In this study, we applied radiomic habitat analysis on AP, PVP, and HBP images from Gd-EOB-DTPA MRI to investigate the potential of intra-tumoral habitat characteristics in predicting MVI.
This study included 312 HCC patients from Hunan Provincial People’s Hospital/The First Affiliated Hospital of Hunan Normal University (referred to as dataset A) and 86 patients from the Third Affiliated Hospital of Sun Yat-sen University (referred to as dataset B) between February 2018 and October 2023. Institutional review board approval was obtained from each participating center. The inclusion criteria were: (1) a solitary tumor, (2) pathologically confirmed HCC following surgical resection, (3) available information on MVI status and grade, and (4) preoperative Gd-EOB-DTPA MRI conducted within two weeks prior to surgery. The exclusion criteria were: (1) macrovascular invasion, (2) prior HCC treatment before MRI (e.g., radiofrequency ablation, microwave ablation, or transcatheter arterial chemoembolization), (3) tumors larger than 10 cm in maximum diameter (as previous studies (23–25) have shown a greater likelihood of MVI in such cases), (4) inadequate MRI quality, and (5) missing pathological or clinical data. The two datasets were combined to form a total cohort of 398 patients. A randomly selected 20% of this cohort was designated as a fixed test set, and the remaining 80% of the cases were used for 5-fold cross-validation. Clinical data, including variables such as age, gender, etiology, cirrhosis, MVI status, and pathological differentiation, were extracted from electronic medical records and are summarized in Table 1.
For dataset A, MRI scans were performed using 1.5T or 3.0T MRI machines from GE (Signal Greator, Premier), Philips (Achieva, Ingenia), and Siemens (Magnetom Trio, Magnetom Prisma, Vida). For dataset B, MRI scans were conducted using 1.5T or 3.0T MRI systems from GE (Optima MR360, Signa Excite, Discovery MR750, Signa Architect), Philips (Achieva), Siemens (Magnetom Prisma), and United Imaging (uMR790). All patients underwent fat-saturated T1-weighted pre-contrast scans, followed by scans in the AP, PVP, and HBP. AP images were acquired 20-30 seconds after gadolinium contrast injection, PVP images 60-70 seconds post-injection, and HBP images were taken 20-30 minutes after contrast administration.
Two experienced radiologists, each with over ten years of experience in MRI diagnostics, independently and blindly assessed the radiological features of the tumors. A consensus was reached regarding the following six characteristics: (1) tumor size, (2) non-smooth tumor margin, (3) radiological capsules (26), (4) intratumoral artery (27), (5) arterial peritumoral enhancement (28), and (6) peritumoral hypointensity on HBP (29).
Primary tumors from the HBP images were manually delineated by a senior radiologist, Yuli Zeng, with over 15 years of experience, using the ITK-SNAP 3.4 software platform (www.itksnap.org). The AP and PVP images were then registered to the HBP images, which served as reference images. To correct for low-frequency intensity nonuniformity, N4 bias field correction (30) was applied to all images. All images were resampled to an isotropic voxel size of 1 × 1 × 1 mm3 using B-spline interpolation, while the delineated tumor masks were resampled using nearest neighbor interpolation.
For each sequence (i.e., AP, PVP, and HBP), 105 radiomics features were extracted from the original images using the PyRadiomics package (31). These features included 14 shape features and 91 texture features. Additionally, texture features were extracted using wavelet filters (HHH, HHL, HLH, HLL, LHH, LHL, LLH, LLL) and Laplacian of Gaussian filters with sigmas of 2.0, 3.0, 4.0 and 5.0, which resulted in a comprehensive set of 1197 features for each sequence.
The images of HCC patients typically exhibit AP hyperenhancement followed by washout in the PVP (16). To assess radiomic changes during dynamic contrast enhancement, the features from the AP images were compared to those from the PVP images using the following Equation 1:
Where represents the change in features between AP images and PVP images, which is time-related and predictive to MVI, represents the features extracted from AP images, and refers to the features generated from the PVP images.
Radiomics offers detailed insights into tumor phenotypes and the tumor microenvironment (32). To capture intra-tumoral heterogeneity, we performed intra-tumoral habitat partitioning in two steps: case-based clustering and subregion feature extraction. Case-based clustering was conducted independently for each tumor using the k-means algorithm with squared Euclidean distances between voxel intensities. The number of clusters was set to five due to the small tumor volumes in this study. The clustering process is performed using the in-house nnFAE software. In the subregion feature extraction step, radiomics features, including histogram, GLCM, GLRLM, NGTDM, GLSZM and GLDM, were extracted from each subregion without applying additional filters.
Subsequently, we applied a Gaussian mixture model to perform unsupervised clustering of radiomic features across all tumor habitats. The optimal number of clusters, representing the diversity of the tumor ecosystem, was determined using the Bayesian Information Criterion (BIC) (33), which generated an intra-tumoral ecological diversity (iTED) feature vector for each patient, which could then be used for further analysis. Each iTED feature reflects the optimal number of clusters corresponding to specific radiomic features. For example, the iTED_entropy feature represents the optimal number of clusters for assessing tumor heterogeneity, using traditional entropy as a metric. While conventional entropy measures the unpredictability or variability of image values, iTED_entropy quantifies the complexity of intra-tumoral heterogeneity by evaluating entropy at the cluster level. This iTED feature vector provides a novel approach to tumor characterization, potentially offering new insights into tumor behavior and structure (34).
The generation of the conventional radiomics features, delta radiomics features and iTED features is shown in Figure 1. To address potential variability in the radiomics features caused by differences in imaging protocols across the two centers, the ComBat harmonization method (35) was applied.
Figure 1. Schematic shows the workflow of the generation of conventional radiomics features, delta radiomics features and ecological diversity features. (A) Conventional radiomics features. (B) Delta radiomics features. (C) Ecological diversity features.
To preselect features with high stability, we simulated delineation perturbations based on the training cohort. Morphological operations, including dilation and erosion, were applied slice-by-slice using a circular structural element with distances of 1 mm and 2 mm. This process generated four distinct VOIs, labeled D1, D2, E1, and E2. To assess feature stability, we used the inter-class correlation coefficient (ICC) (36), classifying features as having high (ICC ≥ 0.75), moderate (0.75 > ICC ≥ 0.50), or low (ICC < 0.50) stability. Following established guidelines (37), we applied the ICC (2,1) model as defined by Shrout and Fleiss (38) and calculated the ICC using the Pingouin statistical library (https://github.com/raphaelvallat/pingouin).
ICC was calculated for all five ROIs, including the original ROI, and the dilated (1 mm and 2 mm) and eroded (1 mm and 2 mm) ROIs. Only first-order and textural features were evaluated for stability, with features having an ICC greater than 0.75 selected for the next stage of the feature selection pipeline. Shape-related features, however, were directly retained and included in the pipeline without undergoing stability evaluation.
For both conventional radiomic features and delta radiomics features, the selection process began with retaining features that showed significant differences between patients with and without MVI, as determined by the Mann–Whitney U-test. Next, we selected features that achieved an Area Under the Curve (AUC) greater than 0.60 in univariate logistic regression analysis. To further refine the feature set, the minimum redundancy and maximum relevancy (mRMR) method was applied to eliminate redundant and irrelevant features. Finally, the Least Absolute Shrinkage and Selection Operator (LASSO) algorithm was used to reduce the feature set to only the most predictive features. For conventional radiomic features, this process resulted in the selection of 18 features from AP images, 13 from PVP images, and 10 from HBP images. For delta radiomics features, 14 features were retained for model development.
For the iTED features, we first applied z-score normalization to standardize the features and removed those with minimal variance. Next, features with an AUC greater than 0.55 in univariate logistic regression were retained. The LASSO algorithm was then applied, leaving 4 features from AP images, 4 from PVP images, and 9 from HBP images for further analysis.
The details of the selected features and their corresponding ICC values are provided in Supplementary Tables S1-S7 of the Supplementary Material.
Differences in clinical and radiological characteristics between the training and test cohorts were assessed using the Mann-Whitney U test for continuous variables and the chi-square test for categorical variables. In the training dataset, five-fold cross-validation with stratified sampling was performed to ensure consistent category proportions. A random forest (RF) model was constructed to classify patients with or without MVI, and Bayesian optimization (39) was applied to fine-tune the model’s hyperparameters.
Ultimately, eight model types were constructed using (1) A clinical-radiological model (CR model) using demographic, pathological, and radiological features, (2) conventional radiomic features from AP images (MCVT-AP), (3) conventional radiomic features from PVP images (MCVT-PVP), (4) conventional radiomic features from HBP images (MCVT-HBP), (5) delta radiomics features (MDelta), (6) iTED features from AP images (MiTED-AP), (7) iTED features from PVP images (MiTED-PVP), and (8) iTED features from HBP images (MiTED-HBP). Then, we developed a fusion_R model to combine the predictions from the above seven radiomics models using a stacking algorithm (40). Furthermore, we constructed a fusion_CR model, which combines the radiomics models with the CR model. The workflow of developing the fusion model by stacking algorithm is shown in Figure 2.
Figure 2. The workflow of developing the fusion model. (A) Cross-validation predictions and test set predictions generated by the based learner. (B) The training process of fusion_R model. (C) The training process of fusion_CR model.
The performance of the models in predicting MVI was evaluated using the Area Under the Receiver Operating Characteristic Curve (AUC) with 95% confidence intervals, as well as accuracy (ACC), sensitivity, and specificity. Delong’s test was employed to compare the AUCs of different models, with statistical significance set at p < 0.05.
A total of 398 HCC patients were included in the study, with 318 patients in the training dataset (mean age 56 years; 271 males, 47 females) and 80 patients in the testing dataset (mean age 55 years; 67 males, 13 females). As shown in Table 1, the two sets were well-balanced as there were no statistically significant differences in clinical-radiological characteristics either between the training and testing sets or within each set (p = 0.104-1.000).
Univariate analysis identified one demographic factor (HBV infection), four radiological factors (tumor size, nonsmooth tumor margin, arterial peritumoral enhancement, and peritumoral hypointensity on HBP), and two pathological factors (degree of differentiation and cirrhosis, stage 4 fibrosis) as being associated with MVI in the training set. Multivariable analysis (Table 2) revealed that tumor size (OR = 1.20, 95% CI: 1.06–1.36, p < 0.001), nonsmooth tumor margin (OR = 2.84, 95% CI: 1.63–5.06, p < 0.001), and cirrhosis (stage 4 fibrosis) (OR = 2.02, 95% CI: 1.19–3.50, p = 0.01) were significant predictors of MVI and were incorporated into the clinical-radiological (CR) model. The CR model achieved an AUC of 0.784 (95% CI: 0.766–0.802) in the training dataset, 0.722 (95% CI: 0.661–0.784) in the validation dataset, and 0.677 (95% CI: 0.610–0.744) in the testing dataset.
Table 2. Stepwise multivariable logistic regression analysis for clinical and radiological variables.
The performance of models based on conventional radiomics features is presented in Table 3. The MCVT-AP model achieved an AUC of 0.723 (95% CI: 0.655–0.789) in the testing cohort, demonstrating superior diagnostic performance compared to the MCVT-PVP model (AUC = 0.672, 95% CI: 0.611–0.734) and the MCVT-HBP model (AUC = 0.620, 95% CI: 0.601–0.639). The higher signal contrast within and between tumors on AP images, due to significant enhancement, likely contributed to this improved performance. Conversely, the MCVT-HBP model exhibited lower sensitivity (0.496), likely due to the minimal signal variation observed in HCC lesions during the HBP phase. Figure 3 illustrates the mean receiver operating characteristic (ROC) curves, the probability distribution of classes, and the confusion matrices for the MCVT-AP, MCVT-PVP, and MCVT-HBP models.
Figure 3. The mean ROC curves, the probability distribution of classes and the confusion matrixes of MCVT-AP, MCVT-PVP, and MCVT-HBP model. (a–c), the mean ROC curves of MCVT-AP (a), MCVT-PVP (b), and MCVT-HBP (c) model, where the shaded region indicates 95% confidence intervals. (d–f) depict the probability distribution of classes for the MCVT-AP (d), MCVT-PVP (e), and MCVT-HBP (f) model. Presented here is the model from one of the folds that is closest to the cross-validation mean. (g-i) depict the confusion matrixes for the MCVT-AP (g), MCVT-PVP (h), and MCVT-HBP (i) model. Presented here is the model from one of the folds that is closest to the cross-validation mean.
The performance of the MDelta model is summarized in Table 4. The MDelta model achieved an AUC of 0.707 (95% CI: 0.678–0.735), outperforming the MCVT-PVP model (AUC = 0.672, 95% CI: 0.611–0.734) but falling short of the MCVT-AP model (AUC = 0.723, 95% CI: 0.655–0.789). Importantly, the MDelta model exhibited a higher sensitivity (0.672, 95% CI: 0.590–0.753) compared to the MCVT-AP model (0.635, 95% CI: 0.508–0.763) and the MCVT-PVP model (0.520, 95% CI: 0.448–0.593), suggesting that delta radiomics features, which capture time-related changes, provide valuable predictive information for MVI beyond what is offered by AP and PVP features alone.
As shown in Table 5, the MiTED-HBP model (AUC = 0.727, 95% CI: 0.706–0.749) outperformed the MiTED-AP model (AUC = 0.613, 95% CI: 0.575–0.651) and the MiTED-PVP model (AUC = 0.691, 95% CI: 0.676–0.707). Interestingly, the performance of the iTED models in different phases was the opposite of that observed in the conventional radiomics models. However, the sensitivity of the iTED models was relatively low (Sensitivity = 0.460–0.545) in the testing cohort. Representative MRI images are displayed in Figure 4. MVI-positive HCC cases were found to have a higher proportion of habitat-4 in the tumor center. Figure 5 provides a visual assessment of clustering effectiveness while emphasizing intra-tumor heterogeneity. In the figure, habitat-1 represents regions with high enhancement; habitat-2 corresponds to areas with medium to medium-high enhancement; habitat-3 includes regions with low or no enhancement; habitat-4 highlights areas of cystic degeneration and necrosis; and habitat-5 encompasses regions with medium-low enhancement.
Figure 4. Representative MRI images of two MVI-negative (a, b) as well as two MVI-positive HCCs (c, d).
Figure 5. Spatial habitats clustered by similar voxels using AP images are demonstrated for a 68-year-old male patient (a, b) and a 51-year-old male patient (c, d). The habitats are defined as follows: habitat-1, regions with high enhancement; habitat-2, regions with medium to medium-high enhancement; habitat-3, regions with low or no enhancement; habitat-4, regions of cystic degeneration and necrosis; habitat-5, regions with medium-low enhancement.
As shown in Table 6, the fusion_R model demonstrated excellent discriminatory performance, achieving an AUC of 0.823 (95% CI: 0.816–0.831) and an accuracy of 0.775 (95% CI: 0.753–0.796) in the testing cohort. The fusion_R model outperformed the base classifiers (MCVT-AP, MCVT-PVP, MCVT-HBP, MDelta, MiTED-AP, MiTED-PVP, and MiTED-HBP) across nearly all evaluation metrics in both the validation and testing cohorts. Figures 6a, b shows the ROC and precision-recall (PR) curves for the fusion_R model alongside the best-performing conventional radiomics model (MCVT-AP), delta radiomics model (MDelta), and iTED model (MiTED-HBP).
Figure 6. The receiver operating characteristic curves (ROC curves) of (a) MCVT-AP model, MDelta model, MiTED-HBP model and the fusion_R model, (c) the fusion_R and fusion_CR model. The precision–recall curves (PR curves) of (b) MCVT-AP model, MDelta model, MiTED-HBP model and the fusion_R model, (d) the fusion_R and fusion_CR model.
The performance of the fusion_R model was comparable to that of the fusion_CR model (AUC = 0.823 vs. AUC = 0.830, p = 0.718), suggesting that while clinical-radiological features had predictive value, their contribution to enhancing the radiomics-based prediction was minimal. The ROC and PR curves for the fusion_R and fusion_CR models are displayed in Figures 6c, d. Additionally, we applied sigmoid calibration to the fusion_R and fusion_CR models. However, the calibration resulted in no significant improvement in performance, with the fusion_R model showing a slight change (pre: 0.823 vs. post: 0.825) and the fusion_CR model exhibiting minimal variation (pre: 0.830 vs. post: 0.828). Supplementary Figure S1 in the Supplementary Material presents the model calibration curves both before and after calibration.
The p values of the Delong test between the different models are shown in Figure 7. The fusion model significantly improved MVI discrimination compared to every other model (p = 0.000–0.050) except the MCVT-AP model (fusion_R: p = 0.101, fusion_CR: p = 0.054). No significant differences were found in the performance of images from different phases, whether using conventional radiomics features (p = 0.096–0.420) or iTED features (p = 0.106–0.744). Additionally, for images from the same phase, there was no significant difference in performance between conventional radiomics features and iTED features (p = 0.158 for AP images, p = 0.844 for PVP images, and p = 0.157 for HBP images). These findings suggest that, although the predictive power of iTED features is not as strong as conventional radiomics features, iTED features have the potential to replace conventional radiomics features to some extent.
In this study, we developed and validated seven radiomics models (MCVT-AP, MCVT-PVP, MCVT-HBP, MDelta, MiTED-AP, MiTED-PVP, and MiTED-HBP) as well as a CR model. Additionally, two fusion models were constructed by combining radiomics models and/or the CR model using a stacking algorithm. Our findings demonstrated that both iTED features and temporal delta radiomics features exhibit substantial predictive power for MVI in HCC.
Gd-EOB-DTPA-enhanced MRI is highly effective in detecting and characterizing focal liver lesions, particularly small-size HCC. After the uptake of Gd-EOB-DTPA contrast, normal functioning hepatocytes exhibit significant enhancement, resulting in high signal intensity during the HBP. In contrast, lesions with impaired or absent hepatocyte function show varying degrees of reduced signal intensity. This marked difference in signal between tumor tissues and the surrounding liver parenchyma is more pronounced in HBP images compared to conventional contrast agents, making tumor boundaries easier to delineate (15). Additionally, key radiological features associated with MVI, such as tumor margin, capsule formation, tumor size, and peritumoral hypointensity, are more clearly visualized in HBP images (41). Our findings align with a previous study, which reported AUC and accuracy values of 0.62 and 0.64, respectively, for an HBP-based model (42).
Delta radiomics analyzes variations in imaging features at different time points, typically before and after treatment. This approach allows for the assessment of changes in features following specific steps in the patient’s care process, such as after therapy, at a particular time point, or in response to a biological event (43). HCC is predominantly supplied by the hepatic artery, which leads to distinct enhancement patterns and signal variations, particularly during the AP and PVP. These dynamic changes in imaging features can serve as strong predictors of MVI, offering additional insights beyond the static AP and PVP features. Xia et al. (18) applied delta radiomics to predict MVI using CT images, yielding AUC values of 0.76 for the internal test set and 0.72 for the external test set. Our experimental results align closely with these findings.
Recent studies have shown that tumors consist of multiple subregions or habitats, each representing clusters of tissue with similar structural, metabolic, or functional characteristics (44). In our study, we accounted for this spatial heterogeneity by dividing tumors into five habitats and extracting radiomic features from each habitat independently. The iTED feature vectors quantified intra-tumoral heterogeneity by determining the optimal number of clusters for each feature. Research has highlighted the importance of radiomic habitat analysis in evaluating MVI. For example, Zhang et al. (44) demonstrated that habitat-imaging-derived quantitative metrics from AP images were significantly associated with MVI, and a nomogram incorporating habitat-derived metrics and tumor size effectively identified MVI-positive HCC. Liu et al. (22) combined habitat modeling with a deep-learning approach based on AP, PVP, and delayed phase images to predict MVI, achieving AUC values of 0.90 in the training set and 0.86 in the validation set. Although their results slightly outperform ours (training: AUC = 0.90 vs. 0.88; validation: AUC = 0.86 vs. 0.78), our findings remain consistent with the predictive power of habitat-based radiomics.
This study demonstrated that the two fusion models significantly improved the accuracy of MVI prediction. Each of the individual models performed exceptionally well in different aspects. The MCVT-AP model, in particular, had a higher AUC value in the testing cohort, indicating stronger predictive power. However, the MiTED models displayed lower sensitivity, which suggests a higher risk of missing MVI-positive patients. In contrast, the MDelta model exhibited notable sensitivity, making it more reliable in detecting MVI-positive cases. The fusion models outperformed all other models, and the reasons for this superior performance are twofold. First, the models were developed using images from different contrast enhancement phases, with each phase providing distinct yet complementary information. Second, the use of a stacking algorithm to combine the radiomics models and/or the CR model further enhanced predictive accuracy, reduced the risk of overfitting, and minimized assumptions related to model parameters (40).
Despite these promising findings, several limitations should be acknowledged. First, being a retrospective study, this research inherently introduced certain biases, such as variations in image acquisition times during dynamic enhanced scanning and differences in imaging parameters. Second, despite being manually delineated by an experienced associate chief diagnostic physician, ROI boundaries may still be inaccurate in cases of incomplete capsules or unclear lesion edges due to subjective interpretation. To account for inter-observer variability, we simulated variability using morphological operations (dilation and erosion), though these methods are limited in capturing actual delineation discrepancies. Third, the fusion models were created by combining all radiomics models, but alternative model combinations might exist that could further enhance the models’ performance and robustness.
In conclusion, iTED features reflecting intratumoral heterogeneity and time-related delta features demonstrated strong predictive capabilities for the preoperative and non-invasive prediction of MVI. The fusion_R and fusion_CR models provided complementary strengths and exhibited superior efficacy, offering valuable assistance in personalized clinical decision-making and improving the prognosis of HCC patients.
The original contributions presented in the study are included in the article/Supplementary Material. Further inquiries can be directed to the corresponding authors.
The studies involving humans were approved by The Medical Ethics Committee of Hunan Provincial People’s Hospital. The studies were conducted in accordance with the local legislation and institutional requirements. Written informed consent for participation was not required from the participants or the participants’ legal guardians/next of kin because Patient informed consent was waived due to the retrospective nature of this study. Written informed consent was not obtained from the individual(s) for the publication of any potentially identifiable images or data included in this article because Patient informed consent was waived due to the retrospective nature of this study.
YuZ: Data curation, Writing – original draft, Writing – review & editing, Investigation. HW: Conceptualization, Formal analysis, Methodology, Visualization, Writing – original draft, Writing – review & editing. YaZ: Data curation, Writing – review & editing. CL: Funding acquisition, Validation, Writing – review & editing. DD: Funding acquisition, Writing – review & editing. YS: Software, Writing – review & editing. SS: Resources, Writing – review & editing. JQ: Data curation, Funding acquisition, Writing – review & editing. GJ: Conceptualization, Funding acquisition, Project administration, Resources, Supervision, Writing – review & editing.
The author(s) declare that financial support was received for the research, authorship, and/or publication of this article. This research was supported by Major Research plan of the National Natural Science Foundation of China (No.2022YFC2410000), National Natural Science Foundation of China (No. 82271948, No.82202129), Guangzhou Key Laboratory of Molecular Functional Imaging and Artificial Intelligence for Major Brain Diseases (No.20220102037320), Guangdong Provincial Natural Science Foundation (2017A030313841), Hospital National Natural Science Foundation Cultivation Project (2021GZRPYM06), Five-Five Project of the Third Affiliated Hospital of Sun Yat-sen University (2023WW605), and the Natural Science Foundation of Inner Mongolia Autonomous Region (No. 2024QN08063).
Author YS was employed by the company Siemens Healthineers Ltd.
The remaining authors declare that the research was conducted in the absence of any commercial or financial relationships that could be constructed as a potential conflict of interest.
The reviewer HT declared a shared parent affiliation with the author(s) YZ, CL, JQ to the handling editor at the time of review.
The author(s) declare that no Generative AI was used in the creation of this manuscript.
All claims expressed in this article are solely those of the authors and do not necessarily represent those of their affiliated organizations, or those of the publisher, the editors and the reviewers. Any product that may be evaluated in this article, or claim that may be made by its manufacturer, is not guaranteed or endorsed by the publisher.
The Supplementary Material for this article can be found online at: https://www.frontiersin.org/articles/10.3389/fonc.2025.1510071/full#supplementary-material
1. Sung H, Ferlay J, Siegel RL, Laversanne M, Soerjomataram I, Jemal A, et al. Global Cancer Statistics 2020: GLOBOCAN estimates of incidence and mortality worldwide for 36 cancers in 185 countries. CA Cancer J Clin. (2021) 71:209–49. doi: 10.3322/caac.21660
2. Forner A, Reig M, Bruix J. Hepatocellular carcinoma. Lancet. (2018) 391:1301–14. doi: 10.1016/S0140-6736(18)30010-2
3. Rodríguez-Perálvarez M, Luong TV, Andreana L, Meyer T, Dhillon AP, Burroughs AK. A systematic review of microvascular invasion in hepatocellular carcinoma: diagnostic and prognostic variability. Ann Surg Oncol. (2013) 20:325–39. doi: 10.1245/s10434-012-2513-1
4. Chan A, Zhong J, Berhane S, Toyoda H, Cucchetti A, Shi K, et al. Development of pre and post-operative models to predict early recurrence of hepatocellular carcinoma after surgical resection. J Hepatol. (2018) 69:1284–93. doi: 10.1016/j.jhep.2018.08.027
5. Chen ZH, Zhang XP, Feng JK, Li LQ, Zhang F, Hu YR, et al. Actual long-term survival in hepatocellular carcinoma patients with microvascular invasion: a multicenter study from China. Hepatol Int. (2021) 15:642–50. doi: 10.1007/s12072-021-10174-x
6. Sheng X, Ji Y, Ren GP, Lu CL, Yun JP, Chen LH, et al. A standardized pathological proposal for evaluating microvascular invasion of hepatocellular carcinoma: a multicenter study by LCPGC. Hepatol Int. (2020) 14:1034–47. doi: 10.1007/s12072-020-10111-4
7. Lambin P, Leijenaar R, Deist TM, Peerlings J, de Jong E, van Timmeren J, et al. Radiomics: the bridge between medical imaging and personalized medicine. Nat Rev Clin Oncol. (2017) 14:749–62. doi: 10.1038/nrclinonc.2017.141
8. Chong HH, Yang L, Sheng RF, Yu YL, Wu DJ, Rao SX, et al. Multi-scale and multi-parametric radiomics of gadoxetate disodium-enhanced MRI predicts microvascular invasion and outcome in patients with solitary hepatocellular carcinoma ≤ 5cm. Eur Radiol. (2021) 31:4824–38. doi: 10.1007/s00330-020-07601-2
9. Yang J, Dong X, Jin S, Wang S, Wang Y, Zhang L, et al. Radiomics model of dynamic Contrast-Enhanced MRI for evaluating vessels encapsulating tumor clusters and microvascular invasion in hepatocellular carcinoma. Acad Radiol. (2025) 32(1):146-56. doi: 10.1016/j.acra.2024.07.007
10. Ahn SS, Kim MJ, Lim JS, Hong HS, Chung YE, Choi JY. Added value of gadoxetic acid-enhanced hepatobiliary phase MR imaging in the diagnosis of hepatocellular carcinoma. Radiology. (2010) 255:459–66. doi: 10.1148/radiol.10091388
11. Jhaveri K, Cleary S, Audet P, Balaa F, Bhayana D, Burak K, et al. Consensus statements from a multidisciplinary expert panel on the utilization and application of a liver-specific MRI contrast agent (gadoxetic acid). AJR Am J Roentgenol. (2015) 204:498–509. doi: 10.2214/AJR.13.12399
12. Li J, Wang J, Lei L, Yuan G, He S. The diagnostic performance of gadoxetic acid disodium-enhanced magnetic resonance imaging and contrast-enhanced multi-detector computed tomography in detecting hepatocellular carcinoma: a meta-analysis of eight prospective studies. Eur Radiol. (2019) 29:6519–28. doi: 10.1007/s00330-019-06294-6
13. Lee S, Kim SH, Lee JE, Sinn DH, Park CK. Preoperative gadoxetic acid-enhanced MRI for predicting microvascular invasion in patients with single hepatocellular carcinoma. J Hepatol. (2017) 67:526–34. doi: 10.1016/j.jhep.2017.04.024
14. Zech CJ, Ba-Ssalamah A, Berg T, Chandarana H, Chau GY, Grazioli L, et al. Consensus report from the 8th international forum for liver magnetic resonance imaging. Eur Radiol. (2020) 30:370–82. doi: 10.1007/s00330-019-06369-4
15. Feng ST, Jia Y, Liao B, Huang B, Zhou Q, Li X, et al. Preoperative prediction of microvascular invasion in hepatocellular cancer: a radiomics model using Gd-EOB-DTPA-enhanced MRI. Eur Radiol. (2019) 29:4648–59. doi: 10.1007/s00330-018-5935-8
16. Khorrami M, Prasanna P, Gupta A, Patil P, Velu PD, Thawani R, et al. Changes in CT radiomic features associated with lymphocyte distribution predict overall survival and response to immunotherapy in non-small cell lung cancer. Cancer Immunol Res. (2020) 8:108–19. doi: 10.1158/2326-6066.CIR-19-0476
17. Mokrane FZ, Lu L, Vavasseur A, Otal P, Peron JM, Luk L, et al. Radiomics machine-learning signature for diagnosis of hepatocellular carcinoma in cirrhotic patients with indeterminate liver nodules. Eur Radiol. (2020) 30:558–70. doi: 10.1007/s00330-019-06347-w
18. Xia TY, Zhou ZH, Meng XP, Zha JH, Yu Q, Wang WL, et al. Predicting microvascular invasion in hepatocellular carcinoma using CT-based radiomics model. Radiology. (2023) 307:e222729. doi: 10.1148/radiol.222729
19. Yang Y, Fan W, Gu T, Yu L, Chen H, Lv Y, et al. Radiomic features of multi-ROI and multi-phase MRI for the prediction of microvascular invasion in solitary hepatocellular carcinoma. Front Oncol. (2021) 11:756216. doi: 10.3389/fonc.2021.756216
20. Nebbia G, Zhang Q, Arefan D, Zhao X, Wu S. Pre-operative microvascular invasion prediction using multi-parametric liver MRI radiomics. J Digit Imaging. (2020) 33:1376–86. doi: 10.1007/s10278-020-00353-x
21. Zhang HD, Li XM, Zhang YH, Hu F, Tan L, Wang F, et al. Evaluation of preoperative microvascular invasion in hepatocellular carcinoma through multidimensional parameter combination modeling based on Gd-EOB-DTPA MRI. JClin Transl Hepatol. (2023) 11:350–9. doi: 10.14218/JCTH.2021.00546
22. Liu HF, Wang M, Lu YJ, Wang Q, Lu Y, Xing F, et al. CEMRI-based quantification of intratumoral heterogeneity for predicting aggressive characteristics of hepatocellular carcinoma using habitata nalysis: comparison and combination of deep learning. Acad Radiol. (2024) 31:2346–55. doi: 10.1016/j.acra.2023.11.024
23. Eguchi S, Takatsuki M, Hidaka M, Soyama A, Tomonaga T, Muraoka I, et al. Predictor for histological microvascular invasion of hepatocellular carcinoma: a lesson from 229 consecutive cases of curative liver resection. World J Surg. (2010) 34:1034–8. doi: 10.1007/s00268-010-0424-5
24. Kaibori M, Ishizaki M, Matsui K, Kwon AH. Predictors of microvascular invasion before hepatectomy for hepatocellular carcinoma. J Surg Oncol. (2010) 102:462–8. doi: 10.1002/jso.21631
25. Kim BK, Han KH, Park YN, Park MS, Kim KS, Choi JS, et al. Prediction of microvascular invasion before curative resection of hepatocellular carcinoma. J Surg Oncol. (2008) 97:246–52. doi: 10.1002/jso.20953
26. Reginelli A, Vanzulli A, Sgrazzutti C, Caschera L, Serra N, Raucci A, et al. Vascular microinvasion from hepatocellular carcinoma: CT findings and pathologic correlation for the best therapeutic strategies. Med Oncol. (2017) 34:93. doi: 10.1007/s12032-017-0949-7
27. Banerjee S, Wang DS, Kim HJ, Sirlin CB, Chan MG, Korn RL, et al. A computed tomography radiogenomic biomarker predicts microvascular invasion and clinical outcomes in hepatocellular carcinoma. Hepatology. (2015) 62:792–800. doi: 10.1002/hep.27877
28. Renzulli M, Brocchi S, Cucchetti A, Mazzotti F, Mosconi C, Sportoletti C, et al. Can current preoperative imaging be used to detect microvascular invasion of hepatocellular carcinoma. radiology. (2016) 279:432–42. doi: 10.1148/radiol.2015150998
29. Kim KA, Kim MJ, Jeon HM, Kim KS, Choi JS, Ahn SH, et al. Prediction of microvascular invasion of hepatocellular carcinoma: usefulness of peritumoral hypointensity seen on gadoxetate disodium-enhanced hepatobiliary phase images. JMagn Reson Imaging. (2012) 35:629–34. doi: 10.1002/jmri.22876
30. Tustison NJ, Avants BB, Cook PA, Zheng Y, Egan A, Yushkevich PA, et al. N4ITK: improved N3 bias correction. IEEE Trans Med Imaging. (2010) 29:1310–20. doi: 10.1109/TMI.2010.2046908
31. van Griethuysen J, Fedorov A, Parmar C, Hosny A, Aucoin N, Narayan V, et al. Computational radiomics system to decode the radiographic phenotype. Cancer Res. (2017) 77:e104–104e107. doi: 10.1158/0008-5472.CAN-17-0339
32. Wu J, Mayer AT, Li R. Integrated imaging and molecular analysis to decipher tumor microenvironment in the era of immunotherapy. Semin Cancer Biol. (2022) 84:310–28. doi: 10.1016/j.semcancer.2020.12.005
33. Natrajan R, Sailem H, Mardakheh FK, Arias Garcia M, Tape CJ, Dowsett M, et al. Microenvironmental heterogeneity parallels breast cancer progression: A histology-genomic integration analysis. PloS Med. (2016) 13:e1001961. doi: 10.1371/journal.pmed.1001961
34. Shi Z, Huang X, Cheng Z, Xu Z, Lin H, Liu C, et al. MRI-based quantification of intratumoral heterogeneity for predicting treatment response to neoadjuvant chemotherapy in breast cancer. Radiology. (2023) 308:e222830. doi: 10.1148/radiol.222830
35. Johnson WE, Li C, Rabinovic A. Adjusting batch effects in microarray expression data using empirical Bayes methods. Biostatistics. (2007) 8:118–27. doi: 10.1093/biostatistics/kxj037
36. Bartko JJ. The intraclass correlation coefficient as a measure of reliability. Psychol Rep. (1966) 19:3–11. doi: 10.2466/pr0.1966.19.1.3
37. Koo TK, Li MY. A guideline of selecting and reporting intraclass correlation coefficients for reliability research. J Chiropr Med. (2016) 15:155–63. doi: 10.1016/j.jcm.2016.02.012
38. Shrout PE, Fleiss JL. Intraclass correlations: uses in assessing rater reliability. Psychol Bull. (1979) 86:420–8. doi: 10.1037//0033-2909.86.2.420
39. Shahriari B, Swersky K, Wang Z, Adams RP, De Freitas N. Taking the human out of the loop: a review of bayesian optimization. Proc IEEE. (2015) 104:148–75. doi: 10.1109/JPROC.2015.2494218
40. Naimi AI, Balzer LB. Stacked generalization: an introduction to super learning. Eur J Epidemiol. (2018) 33:459–64. doi: 10.1007/s10654-018-0390-z
41. Ariizumi S, Kitagawa K, Kotera Y, Takahashi Y, Katagiri S, Kuwatsuru R, et al. A non-smooth tumor margin in the hepatobiliary phase of gadoxetic acid disodium (Gd-EOB-DTPA)-enhanced magnetic resonance imaging predicts microscopic portal vein invasion, intrahepatic metastasis, and early recurrence after hepatectomy in patients with hepatocellular carcinoma. J Hepatobil Pancreat Sci. (2011) 18:575–85. doi: 10.1007/s00534-010-0369-y
42. Qu C, Wang Q, Li C, Xie Q, Cai P, Yan X, et al. A radiomics model based on Gd-EOB-DTPA-Enhanced MRI for the prediction of microvascular invasion in solitary hepatocellular carcinoma ≤ 5 cm. Front Oncol. (2022) 12:831795. doi: 10.3389/fonc.2022.831795
43. Nardone V, Reginelli A, Grassi R, Boldrini L, Vacca G, D’Ippolito E, et al. Delta radiomics: a systematic review. Radiol Med. (2021) 126:1571–83. doi: 10.1007/s11547-021-01436-7
Keywords: intra-tumoral heterogeneity, temporal features, microvascular invasion, radiomics, ensemble learning
Citation: Zeng Y, Wu H, Zhu Y, Li C, Du D, Song Y, Su S, Qin J and Jiang G (2025) MRI-based intra-tumoral ecological diversity features and temporal characteristics for predicting microvascular invasion in hepatocellular carcinoma. Front. Oncol. 15:1510071. doi: 10.3389/fonc.2025.1510071
Received: 18 October 2024; Accepted: 10 February 2025;
Published: 03 March 2025.
Edited by:
Sharon R. Pine, University of Colorado Anschutz Medical Campus, United StatesReviewed by:
Hailin Tang, Sun Yat-sen University Cancer Center (SYSUCC), ChinaCopyright © 2025 Zeng, Wu, Zhu, Li, Du, Song, Su, Qin and Jiang. This is an open-access article distributed under the terms of the Creative Commons Attribution License (CC BY). The use, distribution or reproduction in other forums is permitted, provided the original author(s) and the copyright owner(s) are credited and that the original publication in this journal is cited, in accordance with accepted academic practice. No use, distribution or reproduction is permitted which does not comply with these terms.
*Correspondence: Jie Qin, cWluamllQG1haWwuc3lzdS5lZHUuY24=; Guihua Jiang, MTM4Mjg0NzIyMDFAMTYzLmNvbQ==
†These authors have contributed equally to this work
Disclaimer: All claims expressed in this article are solely those of the authors and do not necessarily represent those of their affiliated organizations, or those of the publisher, the editors and the reviewers. Any product that may be evaluated in this article or claim that may be made by its manufacturer is not guaranteed or endorsed by the publisher.
Research integrity at Frontiers
Learn more about the work of our research integrity team to safeguard the quality of each article we publish.