- 1Department of Thyroid and Breast Surgery, Jinjiang Municipal Hospital (Shanghai Sixth People’s Hospital Fujian), Quanzhou, Fujian, China
- 2Department of Mechanical Engineering, Division of Biomedical Engineering, University of Saskatchewan, Saskatoon, SK, Canada
- 3Department of Computer Science, Central South University, Changsha, Hunan, China
Background: Accurate segmentation of thyroid nodules in ultrasound imaging remains a significant challenge in medical diagnostics, primarily due to edge blurring and substantial variability in nodule size. These challenges directly affect the precision of thyroid disorder diagnoses, which are crucial for metabolic and hormonal regulation.
Methods: This study proposes a novel segmentation approach utilizing a Swin U-Net architecture enhanced with a self-attention mechanism. The model integrates residual and multiscale convolutional structures in the encoder path, with long skip connections feeding into an attention module to improve edge preservation and feature extraction. The decoder path employs these refined features to achieve precise segmentation. Comparative evaluations were conducted against traditional models, including U-Net and DeepLabv3+.
Results: The Swin U-Net model demonstrated superior performance, achieving an average Dice Similarity Coefficient (DSC) of 0.78, surpassing baseline models such as U-Net and DeepLabv3+. The incorporation of residual and multiscale convolutional structures, along with the use of long skip connections, effectively addressed issues of edge blurring and nodule size variability. These advancements resulted in significant improvements in segmentation accuracy, highlighting the model’s potential for addressing the inherent challenges of thyroid ultrasound imaging.
Conclusion: The enhanced Swin U-Net architecture exhibits notable improvements in the robustness and accuracy of thyroid nodule segmentation, offering considerable potential for clinical applications in thyroid disorder diagnosis. While the study acknowledges dataset size limitations, the findings demonstrate the effectiveness of the proposed approach. This method represents a significant step toward more reliable and precise diagnostics in thyroid disease management, with potential implications for enhanced patient outcomes in clinical practice.
1 Introduction
The prevalence of thyroid nodules, a potential indicator of thyroid cancer, has risen in recent years, as noted by Haugen et al. (1). Ultrasound, a simple, convenient, cost-effective, and rapid imaging technique, has become the preferred clinical tool for detecting thyroid nodules. Accurate determination of the size, shape, and contour of thyroid nodules is critical for differentiating between benign and malignant cases. As a result, achieving fully automated and highly precise segmentation of thyroid nodules in ultrasound images is of paramount clinical significance.
Currently, thyroid nodule segmentation methods are categorized into four main approaches: active contour models, region-based methods, and three types of deep learning techniques. Active contour models are commonly employed for the detection of thyroid nodules in ultrasound images, as demonstrated by the works of Maroulis et al. (2), Savelonas et al. (3), and Wong et al. (4). To provide comprehensive patient care, it is essential to understand the physiological implications of thyroid nodules and their detection through ultrasound imaging. Thyroid nodules can trigger physiological responses, including alterations in thyroid hormone levels and disruptions in metabolic processes. Such changes may stem from the potential association between nodules and thyroid cancer, which can impact endocrine function and metabolic regulation. Additionally, medical imaging procedures like ultrasound may induce physiological stress responses, such as fluctuations in blood pressure and heart rate, particularly in patients unfamiliar with the procedure.
Addressing these physiological responses is vital for patient well-being. Effective communication and emotional support play a pivotal role in mitigating patient anxiety and maintaining homeostasis during the diagnostic process. Moreover, precise and timely segmentation of thyroid nodules in ultrasound images can alleviate patient distress by enabling early diagnosis and facilitating appropriate treatment. By recognizing and addressing the physiological dimensions of thyroid nodule detection and diagnosis, healthcare providers can enhance patient well-being and optimize clinical outcomes. Figure 1 illustrates the process of thyroid nodule detection and segmentation, highlighting key techniques from initial ultrasound detection to advanced deep learning methods and their physiological implications.
Traditional methods for medical image segmentation, such as those relying on boundary energy functions, often struggle with the complexity of irregular edges, particularly in cases involving infiltrative or malignant thyroid nodules. These methods typically require the pre-setting of an initial contour, which can result in suboptimal segmentation in areas with irregular tissue boundaries. Variability in gray-level distributions between tissue regions further complicates the segmentation process, even when gray levels are uniform within a single tissue type. In thyroid ultrasound images, the subtle gray-level differences between distinct tissue regions exacerbate these issues, underscoring the need for more advanced methods that leverage prior shape and positional information.
Thyroid nodules, which are abnormal growths in the thyroid gland, can arise due to a variety of factors. Among the known causes are iodine deficiency, genetic mutations, environmental exposure to radiation, and hormonal imbalances. These factors contribute to the formation of both benign and malignant nodules, which may vary in size, shape, and tissue characteristics. The complexity and clinical importance of thyroid nodule detection and classification in ultrasound images highlight the need for precise segmentation methods. Accurate segmentation can aid in distinguishing malignant nodules from benign ones, guiding treatment decisions and improving patient outcomes. This context further emphasizes the significance of developing advanced segmentation models to enhance the precision of thyroid nodule detection.
Recent advancements in deep learning, particularly with architectures like U-Net, have made significant strides in medical image segmentation by automating the process without manual intervention. However, these methods still encounter challenges in distributing information uniformly across spatial locations and channels, leading to computational redundancy that hinders both model training speed and segmentation accuracy. The integration of attention mechanisms has shown promising results in improving efficiency and performance, allowing the model to focus on relevant features while reducing unnecessary computations. A key challenge in deep learning-based segmentation is the need for large, labeled training datasets, which are often time-consuming and difficult to obtain. To address this, Chen et al. (5) propose an enhanced U-Net model for thyroid nodule segmentation, building upon the works of Ma et al. (6) and Gulame et al. (7). Their model incorporates a Swin U-Net backbone with multi-scale convolution modules, improving segmentation precision across a wide range of nodule sizes. Additionally, they enhance the segmentation process with spatial and channel attention mechanisms within the skip connections, allowing the model to preserve critical edge information. The use of self-attention mechanisms further refines the model’s ability to focus on relevant features at different scales, optimizing segmentation performance.
In this work, we introduce an innovative integration of self-attention mechanisms within the Swin U-Net architecture to address the specific challenges associated with thyroid nodule segmentation. This approach enhances feature extraction by prioritizing salient regions of interest, effectively overcoming challenges such as blurred edges and variations in nodule size. Our method significantly advances the capabilities of traditional models like BCDU-Net, offering superior segmentation accuracy, particularly for thyroid nodules of varying sizes. The combined strengths of Swin U-Net’s backbone, multi-scale convolution, and self-attention mechanisms contribute to the high accuracy and robustness of our model, representing a significant advancement in medical image segmentation.
2 Related work
Researchers have proposed an active contour model by Chan and Vese (8) for segmenting thyroid nodules based on the Active Contour Without Edges (ACWE) model. Savelonas et al. (2) introduce the Variable Background Active Contour (VBAC) model, which outperforms ACWE in segmenting thyroid nodule ultrasound images with uneven background distribution. While VBAC is effective at segmenting hypoechoic nodules, it struggles with non-hypoechoic nodules. In the work by Savelonas et al. (3), the Joint Echogenicity-Texture (JET) model is presented as an extension of the VBAC model, incorporating information on regional pixel intensity and texture feature distribution. This enhancement improves segmentation performance in thyroid nodule ultrasound images, particularly outperforming the VBAC model for isoechoic thyroid nodules. However, it faces challenges in distinguishing large blood vessels from thyroid nodules.
In the literature, Wong et al. (4) introduced a method for segmenting thyroid nodule ultrasound images that combines the Active Contour Without Edges (ACWE) model with the Region-Scalable Fitting (RSF) energy model (9). While this approach yields commendable segmentation results, it necessitates the presetting of an initial contour. The implementation process is relatively simple, but the time-consuming segmentation iterations and the need for an initial contour reduce its efficiency. Furthermore, segmentation results vary significantly across different thyroid nodules due to their individual differences.
Simultaneously, researchers have introduced region-based methods for thyroid nodule ultrasound image segmentation. A thyroid nodule hyper-image segmentation method is presented by Zhao et al. in (10), utilizing a normalized model. By incorporating homomorphic filtering and anisotropic diffusion operations, this method reduces image noise while maintaining crucial edge details. However, it has limitations in terms of generality and applicability across different cases. Another region-based approach, utilizing radial gradients and the Variance-Reduction Statistics (VRS) algorithm, is introduced by Zhu et al. in (11). In this method, radiologists manually mark the long and short axes, as well as the center points of the nodules. The VRS algorithm is then used to determine where the nodule’s radial line intersects its edge, after which adjacent points are selected and connected to outline the nodule. B-spline methods are employed to refine the segmentation accuracy. However, the requirement for manual intervention by radiologists introduces inefficiencies in the process. Existing research on region-based methods highlights the importance of abundant prior information to achieve more precise segmentation results.
Deep learning algorithms significantly improve the accuracy and automation of image segmentation compared to traditional approaches. A U-Net network with a residual structure and attention gate mechanism is presented by Wang et al. in (12), which enhances segmentation compared to the conventional U-Net network. However, it is limited when applied to thyroid nodule ultrasound images with low contrast and struggles to segment all nodule regions when multiple nodules are present in the image. For thyroid nodule ultrasound image segmentation, a semi-supervised neural network with an attention mechanism is proposed by Wu et al. in (13). This network leverages weakly annotated classification data and a limited amount of fully annotated segmentation data, yielding commendable results, although its generalization ability still needs improvement. A spatial pyramid pooling model is introduced by Oktay et al. in (14), integrated with the codec path to enhance context information capture. While this model delivers favorable segmentation results, it requires a lengthy training period. A network framework based on Mask R-CNN with multi-task processing capability is devised by Huang et al. in (15), enabling simultaneous detection, segmentation, and classification of thyroid nodules. However, the model shows suboptimal segmentation performance for small nodules. Compared to the two traditional segmentation approaches mentioned above, deep learning-based approaches exhibit substantial advancements in algorithmic automation and adaptability. In recent years, attention mechanisms have been incorporated into deep learning for image segmentation. According to Oktay et al. (15), U-Net integrates the attention mechanism, where a weight map extracted through deep convolution is utilized to guide and supervise shallow convolution. This optimization restricts activation to the targeted segmentation region while decreasing the activation of the background, resulting in improved segmentation outcomes. In the work by Fu et al. (16), an attention module that combines both channel and spatial aspects is introduced. With fewer parameters than mainstream networks, this module can seamlessly integrate into such networks, significantly improving classification and detection accuracy.
A dual attention network is presented by Fu et al. in (17), utilizing spatial and channel attention modules to extract context information within a channel and to identify dependencies between channels. In the context of medical ultrasound image segmentation, Lee et al. propose a model that incorporates a boundary preservation module in (18). This model generates a weight map from boundary key points, which enhances the network’s focus on the target boundary area, ensuring that the segmentation results closely align with expert-defined gold standards for shape and contour. According to Zhong et al. in (19), the semantic segmentation task can be divided into two subtasks: pixel prediction and pixel grouping. They also introduce the Squeeze-and-Attention (SA) module, which learns multi-scale spatial features and non-local features, optimizing segmentation results. This module is seamlessly integrated into mainstream segmentation models. By incorporating attention mechanisms, network models achieve enhanced segmentation results, contributing to greater accuracy and effectiveness in image segmentation tasks.
3 Methodology
Using a Swin-U-Net model, a variant of the U-Net architecture enhanced with Swin Transformer layers, we propose a novel approach for thyroid nodule segmentation. The primary aim of this study is to accurately segment thyroid nodules, which exhibit varying sizes and indistinct boundaries. Figure 2 illustrates how the Swin Transformer components are integrated into the traditional U-Net framework. The Swin-U-Net model consists of three key components: the encoding path, the Swin Transformer layers (serving as attention modules), and the decoding path. In the encoding phase, hierarchical features are extracted from the input image, facilitating a precise representation of nodule characteristics. By incorporating the Swin Transformer architecture, the attention modules capture long-range dependencies and contextual information, significantly enhancing the model’s ability to discern intricate details crucial for thyroid nodule segmentation.
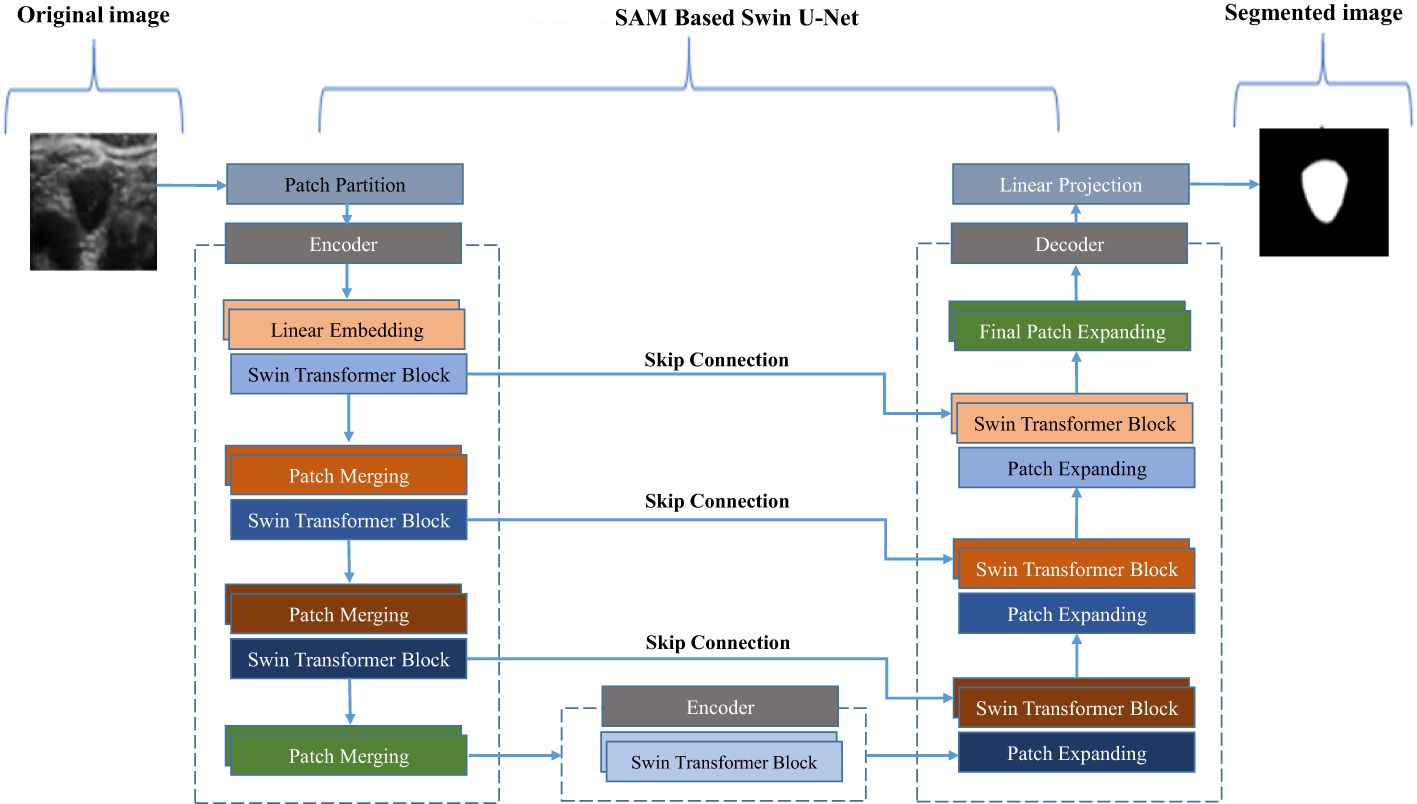
Figure 2. Deep convolutional neural network model based on residual multi-scale convolution and attention mechanism.
Simultaneously, the Swin Transformer attention modules capture and preserve critical information across multiple scales during the down-sampling process within the encoding path. This information is efficiently passed to the corresponding levels in the decoder, thereby enriching the feature representation for the subsequent up-sampling process. The attention mechanism of the Swin Transformer enables the model to better understand spatial relationships within the nodule region. In the decoding path, the combined feature tensor is up-sampled, and the decoder leverages the enriched information from the attention modules to accurately reconstruct the segmented thyroid nodule masks. To further optimize segmentation, the model uses the dice loss function, ensuring alignment between the predicted masks and ground truth labels.
3.1 Ultrasound image acquisition protocol
To ensure high-quality and accurate thyroid ultrasound images for the segmentation task, a standardized ultrasound image acquisition protocol was followed. The following key aspects were considered during the image acquisition process:
a) Anatomical positioning of the transducer
The sonographer placed the transducer on the patient’s neck, typically over the thyroid gland region. The transducer was positioned in such a way that it allowed for optimal visualization of the thyroid lobes, isthmus, and any nodules present. A linear transducer with a high frequency was used to capture detailed images of the thyroid tissue. The sonographer adjusted the angle of the transducer to ensure that both the anterior and lateral aspects of the thyroid gland were clearly visualized.
b) Patient posture and head position
The patient was instructed to lie supine with their neck slightly extended. The head was positioned in a neutral alignment or slightly tilted backward to facilitate better access to the thyroid region. This positioning ensured that the thyroid gland was in the optimal imaging plane for accurate visualization. The patient’s comfort was prioritized throughout the scan to minimize movement and artifacts during image acquisition.
c) Scanning technique
The sonographer used both longitudinal and transverse sweeps to capture comprehensive images of the thyroid gland. The longitudinal sweep provided a vertical view of the gland, enabling the assessment of the thyroid’s length, height, and depth. The transverse sweep allowed for a cross-sectional view, facilitating the identification of nodules and the evaluation of their size, shape, and boundaries. The sonographer also employed slight adjustments to the transducer pressure to optimize image quality and ensure consistent contact with the skin.
d) Specifications of the ultrasound machine and transducer
The ultrasound images were acquired using a GE Logiq E9 ultrasound machine equipped with a GE 12L-RS linear array transducer. The transducer used has a frequency range of 7-12 MHz, which is suitable for high-resolution imaging of soft tissue structures like the thyroid. The image resolution provided by the machine was 1024x768 pixels, ensuring clear and detailed visualizations of the thyroid nodules.
3.2 Segmentation through encoder-decoder architecture
In this study, both training and testing are performed using the proposed Swin-U-Net-based self-attention mechanism architecture for anchor-free object detection, specifically focusing on thyroid nodules. The model follows a structured approach, beginning with an annotated image being input into the encoder-decoder architecture. This framework extracts essential features and gradually reconstructs the segmented image.
Once the image is processed through the encoder-decoder model, it is further refined using a self-attention mechanism. This mechanism enhances the model’s capability by capturing high-level features and contextual information, essential for the accurate detection of thyroid nodules. The self-attention mechanism works by selectively focusing on the most relevant spatial relationships within the image, enhancing the precision of nodule segmentation. This process is accomplished through the use of convolutional and pooling layers, which extract and pool features at multiple scales, contributing to improved segmentation performance. By combining the strengths of Swin-U-Net’s architecture and self-attention mechanisms, the model achieves robust object detection and segmentation, even in challenging thyroid ultrasound images with variable nodule sizes and subtle edge differences.
In this study, an annotated image is processed through a Swin U-Net-based self-attention mechanism architecture, designed specifically for anchor-free object detection, particularly for thyroid nodule analysis. The input image first traverses the encoder path of the Swin U-Net, where features are captured and organized hierarchically. The encoder utilizes a series of convolutional and pooling layers to efficiently extract essential features, enabling the model to identify hierarchical representations of the input image. After passing through the encoder, the processed image enters the self-attention mechanism, a critical phase where the model captures long-range dependencies and contextual information. By employing convolutional layers, the self-attention mechanism refines and emphasizes significant features, dynamically considering the importance of different regions in the image. This allows the model to focus on specific details, which is particularly valuable in detecting intricate structures such as thyroid nodules.
Following the self-attention mechanism, the enhanced image progresses through the Swin U-Net decoding path. The decoder is responsible for reconstructing the spatial information and generating the final segmentation output.
The decoder refines the features of the input image through the use of up-sampling and convolutional layers. As a result, a segmented output is created that identifies and highlights thyroid nodules. These layers progressively reduce the spatial dimensions while capturing diverse visual patterns using learned filters. Mathematically, this is represented in Equations 1 and 2, where represents the feature maps in the encoder and represents the pooled feature maps.
Max pooling is a commonly used downsampling technique in convolutional neural networks (CNNs), designed to reduce the spatial dimensions of feature maps while retaining essential information. After each convolutional layer, the feature maps are activated using Rectified Linear Units (ReLUs), which introduce nonlinearity and enhance the model’s ability to capture complex patterns. Subsequently, max pooling is applied to further decrease the spatial resolution of the feature maps, ensuring that only the most significant features are preserved while irrelevant ones are discarded. This downsampling operation reduces the dimensionality of the data, enabling the network to focus on higher-level representations.
As the spatial dimensions of the feature maps decrease, the number of feature maps typically increases, allowing the network to capture more abstract representations of the input data. The processed feature maps are then passed through the decoder to restore the spatial information lost during the downsampling process. In the case of the Swin U-Net architecture, this reconstruction is achieved through a combination of upsampling layers and convolutional operations, which progressively recover the original resolution of the input image.
During upsampling, skip connections play a crucial role. At each stage, feature maps from the corresponding encoder path are concatenated with those in the decoder. These skip connections integrate both local and global information, which is vital for improving the accuracy of object localization and segmentation. By utilizing these connections, the decoder can access low-level features from the encoder, ensuring that fine-grained details are preserved and enhancing segmentation precision. This process allows the network to maintain accurate object boundaries and effectively recover spatial information, ultimately leading to more precise segmentation of thyroid nodules or other medical imaging tasks.
A mathematical representation of the aforementioned concept is represented by Equation 3. where represents the up-sampled feature maps in the decoder.
The convolutional layers of the decoder play a crucial role in enhancing feature maps, emphasizing intricate details, and improving segmentation accuracy. By incorporating ReLU activation, the network effectively models complex relationships and patterns. During the upsampling process, spatial resolution is restored while simultaneously reducing the dimensionality of feature maps, resulting in a segmentation map that aligns closely with the original input image. Furthermore, the integration of skip connections or residual connections facilitates direct pathways between corresponding layers in the encoder and decoder paths. This architecture allows for the transfer of low-level details from the encoder to the decoder, thereby enhancing detection accuracy. The mathematical representation of this concept is provided in Equation 4, where represents the concatenated feature maps, represents the encoder, and represents the up-sampling operation.
The network adeptly combines high-level information with detailed spatial nuances by concatenating encoder feature maps with up-sampled feature maps in the decoder, thereby achieving precise localization and detection. The incorporation of skip connections effectively mitigates information loss during the down-sampling process, facilitating enhanced information flow and, consequently, improved performance in segmentation tasks. In the context of Swin U-Net-based Self-Attention Mechanism (SAM) models, which utilize an encoder-decoder architecture, the detection process is typically finalized with a 1 1 convolutional layer. This layer is subsequently followed by an activation function, such as sigmoid or SoftMax, to produce the final segmentation map. This structured approach ensures that both coarse and fine details are preserved, leading to superior segmentation outcomes.
The purpose of this layer is to generate the ultimate segmentation map, assigning probabilities or class labels to every pixel. The use of a 1 1 convolutional layer facilitates the aggregation of information, enabling the network to comprehend intricate relationships and integrate features across different scales, which contributes to the precise segmentation of data. As a result, pixel-level probabilities or class labels are derived using the activation function, whether it is sigmoid or SoftMax. The Equations 5 and 6 show this, where represents the feature maps in the decoder, represents the predicted segmentation map, denotes convolutional operations, represents the ReLU activation function, and represent learned weights and biases, respectively.
3.3 Role of self attention module
In this methodology, training data optimization is accomplished through phased image enhancements. Initially, the original image undergoes random width and length adjustments within a factor range of 0.7 to 1.3, followed by conversion into a 256 256 grayscale format. Afterward, an HSV transformation is applied, shifting the image from within the HSV region to areas outside it, enhancing both the image quality and the model’s resilience. The hue channel is randomly adjusted with an amplitude of 0.1, while the saturation and value channels experience random variations with an amplitude of 0.5.
This technique is especially important for handling medium-resolution remote sensing images, which often span large areas with multiple acquisitions. The color variations in these images, caused by the temporal gaps between acquisitions, necessitate effective image enhancement during Swin-U-Net network training. By leveraging improved HSV conversion techniques, the model’s performance is strengthened across diverse regions, ensuring more efficient use of the training data. This advanced approach significantly enhances the model’s robustness, particularly in dealing with medium-resolution remote sensing images that exhibit color inconsistencies due to time intervals between acquisitions.
A CNN feature extraction block known as a Swin Transformer is responsible for handling this task. Through the patch partition procedure, the input image undergoes a reduction of one-fourth in both length and width, as well as sixteen times its reduction in channel. Due to the fact that the Swin Transformer considers a 4x4 image element as its minimum structural unit, this is the case. A Swin Transformer block consists of two distinct modules, which differ from the conventional Multi-Head Self-Attention (MSA) module found in VIT (Figure 3). There is a Shifted Window-Based module called MSA (SW-MSA) and a Consistent Window-Based module called MSA (W-MSA) within the Swin Transformer block. A 2-layer Multilayer Perceptron (MLP) with Gaussian Error Linear Unit nonlinearity (GELU) follows. Each MSA module and MLP is preceded by a Layer Norm (LN) layer, and each module is followed by a residual connection. Equations 7–11 outline the mathematical calculations for these procedures.
Where is the output features of the module and is the output features of the MLP module, where represents the number of blocks.
where denote the query, key, and value matrices.2 and represent the number of patches in a window and the dimension of the query or key, respectively. And, the values in are taken from the bias matrix . Attention is a mechanism that allows the model to focus on certain parts of the input data more selectively. It computes a weighted sum of the value matrix based on the similarity between the query matrix and the key matrix . SoftMax is a mathematical function used to convert a vector of real values into a probability distribution, ensuring that the attention weights sum to 1, allowing the model to assign varying degrees of importance to different inputs.
4 Experiment and analysis
4.1 Parameter settings and experimental data
Our experimental dataset consists of 600 thyroid nodule ultrasound images, carefully curated from multiple hospitals to ensure diversity and robustness. Each image is paired with expert-labeled contours to ensure high annotation accuracy. The original ultrasound images, which have dimensions of 500 × 300 pixels, were resized uniformly to 256 × 256 pixels after anonymizing any privacy-sensitive information. The dataset was randomly divided into training, validation, and test sets in an 8:1:1 ratio, ensuring balanced representation across all subsets. The network architecture was configured with the following parameters: an input image size of 256 × 256, a batch size of 8, and an initial learning rate of 0.001. The Adaptive Moment Estimation (Adam) optimizer was chosen for its efficiency in handling sparse gradients, and the Dice loss function was employed to prioritize overlap accuracy in segmentation tasks. Training was conducted for a maximum of 150 iterations, utilizing an adaptive learning rate strategy that halved the rate if the validation loss failed to improve over 10 consecutive iterations.
The hardware environment included a 2.30 GHz Intel(R) Xeon(R) CPU and an NVIDIA Tesla P-100 GPU, providing high computational performance. The software setup comprised Python 3.7 running on an Ubuntu 18.04 operating system, with hybrid frameworks based on TensorFlow and Keras for efficient implementation and experimentation. To enhance transparency, we provide demographic details of the patients included in the dataset, along with information about the imaging conditions. The dataset reflects a diverse age and gender distribution typical of thyroid nodule cases. Standardized ultrasound imaging protocols were followed to ensure uniformity in capturing the nodules, minimizing variability that could affect segmentation accuracy.
Extensive experimentation guided the selection of hyperparameters, striking a balance between mitigating overfitting and ensuring the model’s generalization ability. After initial tests, the learning rate was fine-tuned to 0.0001 to optimize convergence, and the batch size was increased to 16 to maximize GPU memory efficiency while maintaining training stability. This configuration was critical for preventing oscillation or divergence during training, ensuring steady gradient updates and improved model performance. The design and setup reflect a deliberate optimization of computational resources, model performance, and dataset integrity, establishing a robust foundation for the proposed segmentation framework.
4.2 Evaluation matrix
To compare the segmentation (20) accuracy of the proposed method to benchmark models such as Swin U-Net (21), ADeepLabv3+ (22), BCDU-Net (23), U-Net++ (24), AU-Net (15), and other methodologies which incorporate the Squeeze and Excitation (SE) module (25), we conducted experiments. The segmentation analysis is based on the Dice Similarity Coefficient (DSC), Intersection over Union (IoU), Hausdorff distance, False Positive Rate (FPR), and False Negative Rate (FNR) (26). Below are the specific definitions of these metrics. The DSC and Intersection over Union (IOU) of two regions, A and B, are defined as follows:
The Hausdorff distance, represented by , is defined as follows:
where
Here, and are denoted as:
Where is the number of false positives within the pixel classification outcome, while is the number of false negatives within the pixel classification outcome.
4.3 Experimental results
Figure 4 provides a comparative visualization of segmentation results for four ultrasound images (Samples 1, 2, 3, and 4) using various network architectures. Figure 4A presents the original ultrasound images, and Figure 4B displays the expert-annotated gold standard as a reference. Figures 4C–I showcase the segmentation results from U-Net, DeepLabv3+, BCDU-Net, U-Net++, AU-Net, AU-Net with the Squeeze-and-Excitation (SE) module, and the proposed Swin U-Net-based method, respectively. Highlighted regions in the outlined boxes indicate areas of discrepancy between the expert annotations and the segmentation outputs, emphasizing the comparative performance of each method in addressing challenges such as edge blurring and size variability of thyroid nodules.
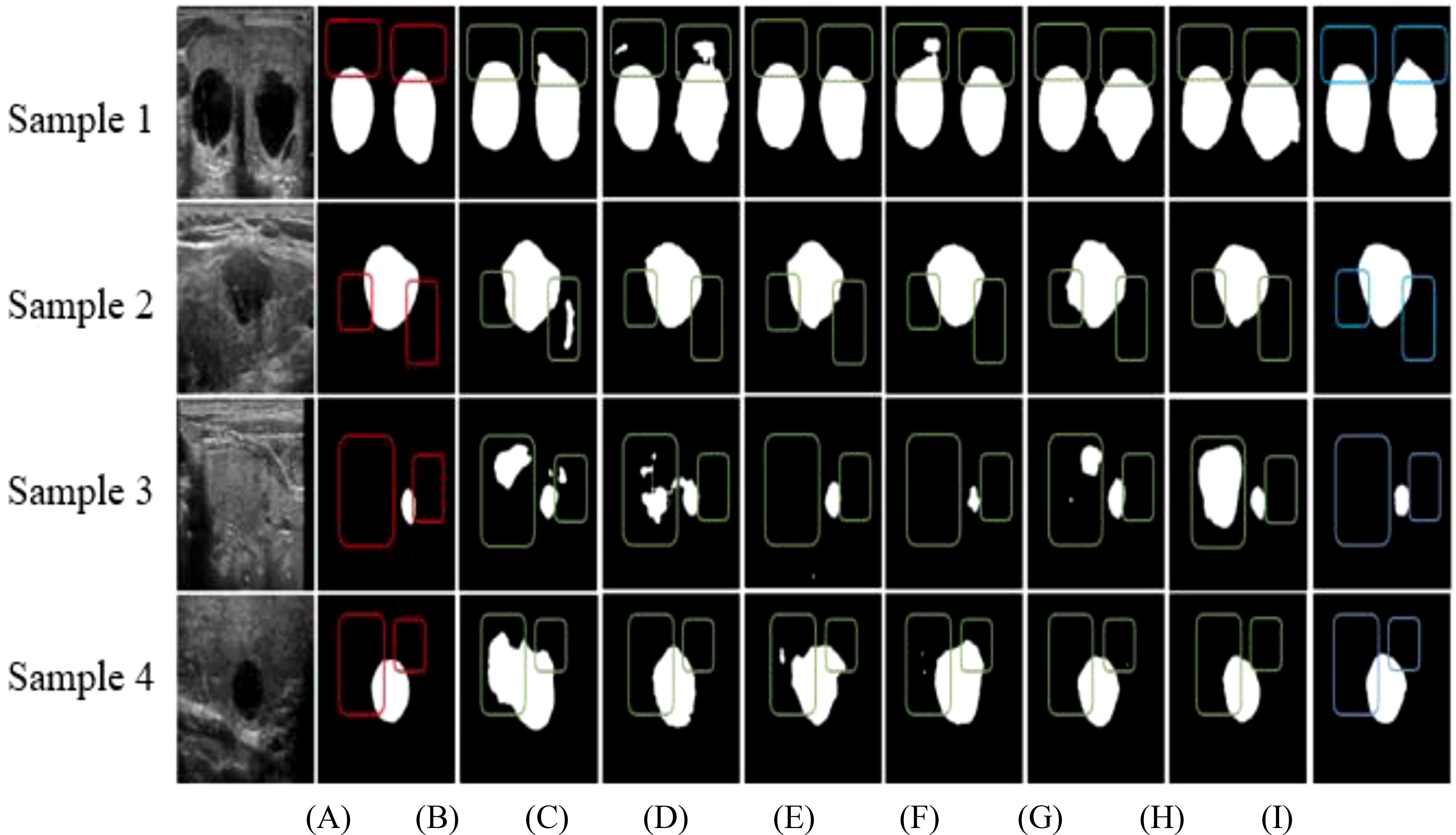
Figure 4. Comparison of segmentation results of different networks based on (A) Original data, (B) Gold standard, (C) U-Net, (D) DeepLabv3+, (E) BCDU-Net, (F) U-Net++, (G) AU-Net, (H) AU-Net+SE, and (I) Our method.
As illustrated in Figures 4C, D, G, H, over-segmentation is a prominent issue in the results produced by U-Net, DeepLabv3+, AU-Net with the Squeeze-and-Excitation (SE) module, and U-Net with the SE module. In these cases, certain regions are misclassified, diverging notably from the expert-labeled contours. This challenge is especially pronounced in the segmentation of small nodules. Figures 4E, F reveal that BCDU-Net and U-Net++ also face segmentation difficulties, although BCDU-Net demonstrates better performance with small nodules. Conversely, U-Net++ shows under-segmentation tendencies in these instances. Figure 4I highlights the superior performance of the proposed method, where the edge contours closely align with the expert-marked boundaries. The segmentation maintains accurate aspect ratios and shapes, both of which are critical for subsequent medical diagnoses. These results underscore the effectiveness of the proposed method compared to baseline models.
In convolutional neural networks, shallow layers typically extract edge details, while deeper layers capture abstract semantic features. However, increasing network depth often leads to the loss of essential shallow edge information. To address this, the proposed method employs an attention module to connect shallow encoder features to their corresponding decoder levels. This module assigns greater weights to edge details in the shallow features while suppressing irrelevant or noisy information, ensuring that critical edge details are retained. Given the wide size variability of thyroid nodules, traditional architectures like U-Net, BCDU-Net, U-Net++, and AU-Net, which rely on a fixed 3×3 convolutional kernel, often exhibit insufficient receptive fields for objects of varying dimensions. The proposed method overcomes this limitation by incorporating a multi-scale convolution module in both the encoder and decoder stages. This module concatenates outputs from convolutions of varying kernel sizes across multiple channels, thereby enhancing the network’s ability to handle diverse nodule sizes.
Our evaluation demonstrates that the Swin U-Net model consistently outperformed other architectures across multiple metrics, including Dice coefficient, Intersection over Union (IoU), and sensitivity. Specifically, the proposed model achieved a Dice coefficient of 0.78, significantly surpassing the baseline models. This indicates its superior capability in addressing challenges such as blurred edges and size variability in thyroid nodule segmentation. A detailed comparison of these metrics is presented in Table 1, showcasing the significant performance improvements achieved by our multi-scale approach on the test set.
As indicated in Table 1, the proposed method demonstrates superior performance overall. Compared to the original U-Net model, the segmentation results of the proposed method show an approximate 16% improvement in Dice Similarity Coefficient (DCS) and a 19% improvement in Intersection over Union (IOU). Furthermore, the proposed approach significantly enhances edge contour preservation, as evidenced by the reduced Hausdorff distance, highlighting the effectiveness of the attention module in maintaining boundary integrity. Figure 5 illustrates the performance metrics of various models across five criteria.
In Figure 1, the grouped bar chart displays the values for each metric across the different methods, highlighting the comparative strengths and weaknesses of each model.
The False Positive Rate (FPR) is notably reduced with the proposed method, while the False Negative Rate (FNR) is slightly less favorable compared to U-Net. This is likely because U-Net tends to over-segment, producing larger segmentation areas and thus reducing the likelihood of under-segmentation. Overall, the proposed model outperforms other algorithms in all key performance metrics.
4.4 Visualization and analysis of attention modules
This paper presents visualization experiments on two attention mechanisms to further elucidate their roles in the segmentation process. Figure 6 showcases the spatial attention weight maps for four attention modules. Specifically: (A) depicts the original ultrasound image, (B) shows the expert-annotated gold standard segmentation, and (C) through (F) illustrate the visualization results of the weight maps corresponding to the four attention modules. These visualizations highlight the contributions of each module in refining feature extraction and enhancing the accuracy of thyroid nodule segmentation.
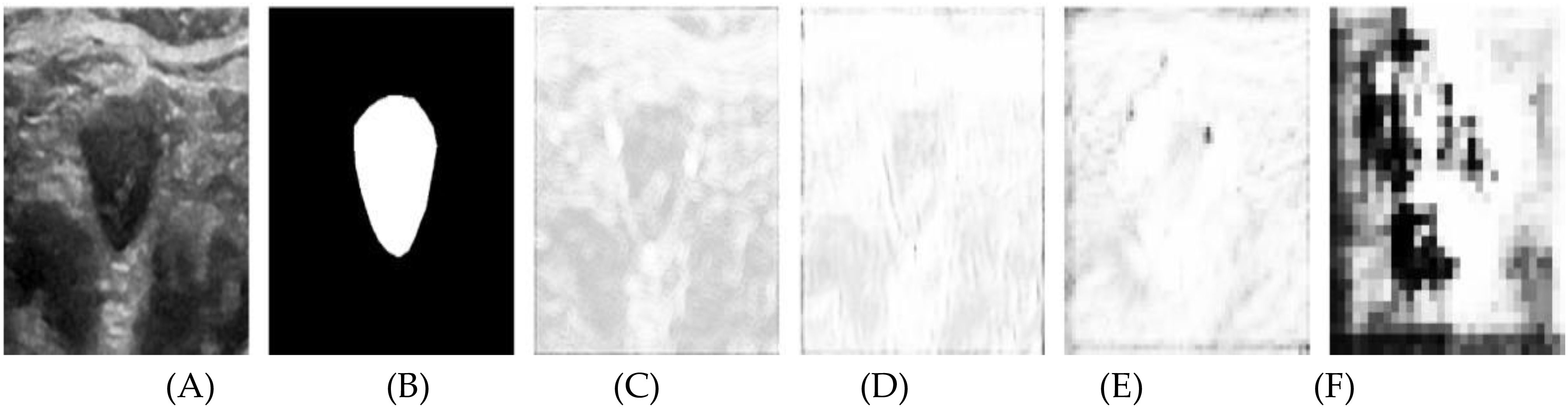
Figure 6. Results after visualization of the spatial attention weight map, including (A) the original image, (B) the labeled map, and (C-F) levels 1 through 4, respectively.
In the figure, brighter regions represent weights close to 1, indicating that spatial information in these areas significantly influences the segmentation outcome. In contrast, darker regions signify weights near zero, meaning the spatial information in these areas contributes minimally to the segmentation. Without a spatial attention module, the weight map would appear uniformly white, implying equal weighting across all spatial positions and a uniform reliance on spatial information from each pixel.
In Figure 6C, the brighter contour edges of the nodule indicate areas of high significance, although several background regions also exhibit notable brightness. Figure 6D shows a broader distribution of high brightness across various positions, except within the nodule’s interior and parts of the background. Figures 6E, F illustrate the increasing abstraction of the weight map as the network hierarchy deepens. Notably, the nodule’s edge consistently remains bright, while the background becomes progressively darker.
The attention module assigns greater weight to the contour information of the nodule edge within the feature tensor extracted from the shallow encoder path. At shallow network levels, the smaller receptive field limits the model’s ability to capture the global context, resulting in an overemphasis on certain background spatial information. As the network deepens, the spatial attention module expands its receptive field, enhancing its ability to distinguish between the foreground and background. Consequently, spatial information outside the target area becomes less pronounced, leading to improved focus on the nodule edges and more accurate segmentation.
The segmentation advancements achieved by the Swin U-Net model hold significant clinical potential. Accurate boundary detection, as demonstrated in Figure 6, is crucial for reliable area measurement, which is a key diagnostic parameter in assessing thyroid nodules. Improved segmentation precision can enhance the detection of subtle morphological changes in nodules, supporting early diagnosis and tailored treatment strategies.
Furthermore, robust segmentation of diverse nodule types, regardless of edge blurring or size variability, reduces diagnostic inconsistencies and enhances reliability in clinical workflows. These improvements translate into better patient outcomes by enabling timely and precise interventions. From a broader perspective, the societal benefits of this approach include optimized healthcare resources and improved quality of life for patients through early detection and effective management of thyroid disorders.
These results underscore the model’s capability to address critical challenges in thyroid ultrasound imaging and its potential to transform diagnostic practices, aligning with the broader goals of advancing medical imaging technologies for improved healthcare outcomes.
In Figure 7, the segmentation process is further detailed through the visualization of several channels from the output feature tensor. Figure 7A displays the original ultrasound image, while Figure 7B shows the corresponding labeled image. Figures 7C–E illustrate the visualization of representative channels from the topmost attention module of the Swin U-Net architecture (as outlined in Figure 1). The respective channel weights are indicated below each subfigure, offering insights into their contributions to the segmentation process. From these subfigures, we observe distinct patterns: channel 6 is assigned a lower weight, reflecting its extraction of largely irrelevant information, which complicates the identification of useful nodule contours. Conversely, channels 13 and 39 receive higher weights, as they effectively capture critical features, particularly the nodule’s edge. These channels highlight abstract representations that distinguish the nodule from the background, underscoring the self-attention module’s capacity to prioritize relevant features during the learning process. The attention maps derived from the self-attention mechanism provide valuable insights into the areas of the image that contribute most significantly to the segmentation. As illustrated in Figure 7, the model excels in identifying nodule boundaries, even when edge blurring is present. This ability to localize critical regions underpins the superior performance of the proposed Swin U-Net model compared to existing state-of-the-art methods, as evidenced by the study by Wang et al. (27).
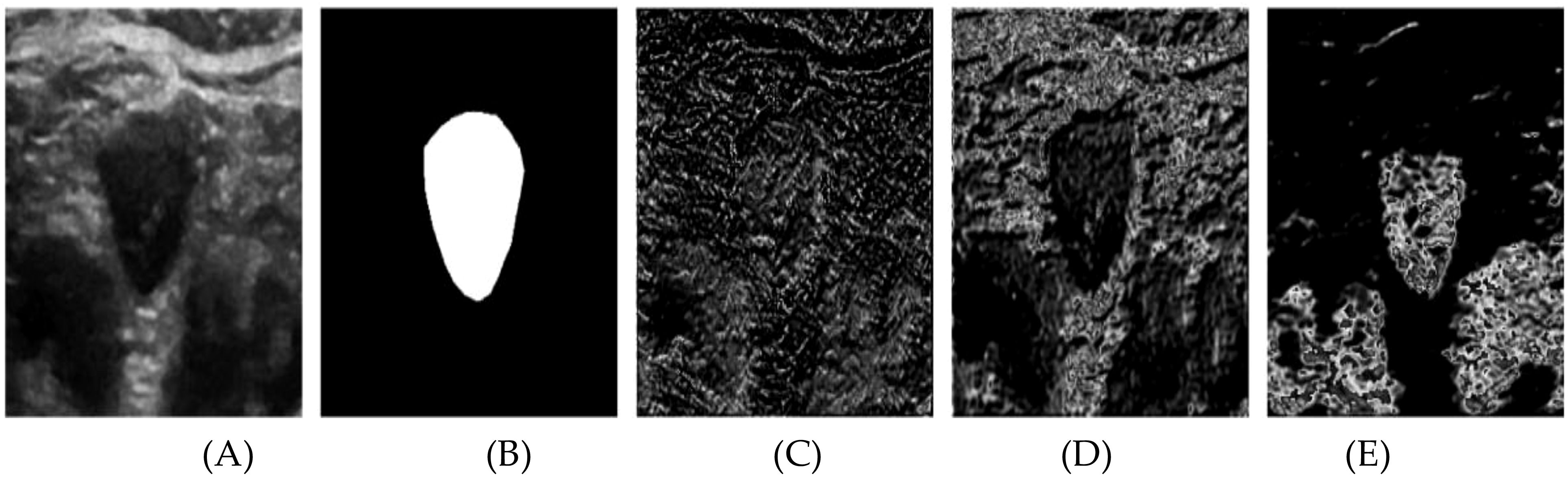
Figure 7. The channel attention module outputs the results after different channel visualizations of the feature tensor. (A) original image, (B) Labeled map (C) Channel 6Weight 0.5226 (D) Channel 13 Weight 1 (E) Channel 39 Weight 1.
The reduced clarity observed in Figure 6 compared to Figure 7 stems from the differing roles of the spatial and channel attention modules. In Figure 6, the spatial attention maps—particularly from the shallower network layers—tend to emphasize irrelevant background regions due to their limited receptive field. As the network deepens, the representations become more abstract, further diminishing visual clarity. In contrast, Figure 7 visualizes the channel attention mechanism, which selectively enhances feature channels with higher relevance, effectively filtering out background noise. This enables the model to emphasize key areas, such as nodule boundaries, resulting in more refined visualizations. This distinction underscores the importance of channel selection in optimizing segmentation performance. Although segmentation alone does not directly diagnose thyroid nodules, it plays a critical role in providing accurate size measurements, which are essential for assessing the growth and potential malignancy of the nodules. Accurate segmentation can aid clinicians in making informed decisions about the need for further investigation, monitoring, or intervention, enhancing the overall diagnostic and treatment process.
The integration of advanced neural network architectures and optimization techniques has significantly influenced various domains, including image processing, biomedical engineering, and manufacturing systems. Ding et al. (28) highlighted the application of signal processing and machine learning for medical imaging, demonstrating advancements in computational accuracy. Similarly, Szegedy et al. (29) introduced Inception-v4 and Inception-ResNet architectures, emphasizing the impact of residual connections on deep learning, thereby improving training efficiency and generalization. Kingma and Ba (30) proposed the Adam optimization algorithm, which has become a foundational tool for stochastic optimization in neural networks. In manufacturing systems, Du et al. (31) explored quality improvements in multistage production processes through comprehensive modeling and analysis. Furthermore, Lu et al. (32) developed ThyroidNet, a specialized deep learning framework for accurate localization and classification of thyroid nodules, showcasing the practical applications of deep learning in medical diagnostics.
The assignment of channel weights plays a crucial role in improving segmentation accuracy. By selectively enhancing the contributions of relevant channels (e.g., channels 13 and 39) and suppressing irrelevant ones (e.g., channel 6), the model focuses on critical features such as boundary delineation and texture variations. This weighted emphasis allows the Swin U-Net model to more effectively differentiate thyroid nodules from surrounding tissues, improving segmentation accuracy and robustness. The self-attention mechanism facilitates this process by dynamically evaluating the relevance of each channel and prioritizing those with higher significance. This results in more accurate segmentation outputs, as the model efficiently suppresses noise and irrelevant information while enhancing key diagnostic features. To evaluate the performance of the Swin U-Net model, we employed robust metrics, including the Dice Similarity Coefficient (DSC) and Intersection over Union (IoU): DSC quantifies the overlap between the predicted segmentation and the ground truth, ranging from 0 (no overlap) to 1 (perfect overlap). A high DSC reflects the model’s ability to reliably identify and delineate thyroid nodules, which is crucial for accurate diagnosis and treatment planning. IoU measures the precision of segmentation by assessing the ratio of the intersection to the union of the predicted and ground truth regions. Higher IoU values indicate reduced false positives and false negatives, leading to more reliable segmentations in clinical settings.
Several future research directions can enhance its performance. These include integrating real-time data collection using mobile and wearable ultrasound devices to capture live data, which could diversify the training dataset and enable continuous learning. Additional data augmentation techniques and synthetic data generation could improve model generalization, while integrating multi-modal imaging (e.g., CT, MRI) could enhance segmentation accuracy. Optimizing the model for real-time clinical deployment through techniques like model pruning and edge-computing is crucial for immediate feedback in resource-constrained environments. Addressing interpretability challenges in medical applications through techniques like Grad-CAM can improve clinician trust. Future research should also focus on overcoming challenges in dataset collection and annotation by collaborating with hospitals for more diverse datasets and using semi-supervised learning. To address data imbalance, techniques like oversampling minority classes or using class-weighted loss functions could be explored. Finally, improving real-time ultrasound data acquisition through robust preprocessing pipelines and efficient segmentation methods is essential for clinical applicability.
To improve segmentation accuracy and increase DSC values, future work can focus on integrating attention mechanisms, multi-scale feature fusion, and transformer-based architectures like the Swin Transformer. Enhancing data diversity through augmentation techniques, utilizing specialized loss functions, and applying post-processing methods such as CRFs can refine results. Expanding the training dataset and exploring ensemble learning methods could further enhance model performance and robustness.
5 Conclusion
In this paper, we present a novel segmentation approach tailored for thyroid nodule ultrasound images, leveraging the advanced capabilities of a Self-Attention Mechanism-based Swin U-Net architecture. The proposed method integrates cutting-edge techniques to significantly enhance segmentation performance. Specifically, the U-Net backbone is optimized with residual structures and multiscale convolution, enabling efficient feature extraction across multiple scales. This enhancement is crucial for capturing the intricate and complex characteristics of thyroid nodules accurately.
To further improve segmentation precision, a self-attention mechanism is employed, allowing the model to focus on critical features while effectively addressing challenges such as nodule size variability and edge blurring—common issues in ultrasound imaging. Experimental evaluations reveal that the proposed method outperforms existing algorithms, achieving superior accuracy across nodules of varying sizes and characteristics.
Building on these promising results, we envision the potential adaptation of our segmentation framework to other medical imaging tasks. Future work will explore its application to diverse imaging modalities, including magnetic resonance imaging (MRI) and computed tomography (CT). To facilitate real-time clinical implementation, we aim to optimize the network by reducing its parameter count, enhancing computational efficiency without compromising performance. Additionally, we plan to incorporate auxiliary features and leverage diverse datasets to further improve the model’s robustness and generalizability.
Data availability statement
The original contributions presented in the study are included in the article/supplementary material. Further inquiries can be directed to the corresponding author.
Ethics statement
The studies involving humans were approved by the Institutional Ethics Committee of the Department of Thyroid and Breast Surgery, Jinjiang Municipal Hospital, Quanzhou, Fujian Province. The study was conducted in compliance with ethical standards and in accordance with local legislation and institutional requirements. Prior to the commencement of the experiment, ethical approval was obtained to ensure the protection of participants’ rights and well-being, following the hospital’s guidelines and regulations. The participants provided their written informed consent to participate in this study. The studies were conducted in accordance with the local legislation and institutional requirements. The participants provided their written informed consent to participate in this study.
Author contributions
CY: Conceptualization, Formal analysis, Funding acquisition, Writing – review & editing. MA: Conceptualization, Formal analysis, Methodology, Writing – review & editing. MR: Conceptualization, Data curation, Methodology, Writing – original draft, Writing – review & editing. PU: Data curation, Project administration, Validation, Conceptualization, Investigation, Writing – original draft, Writing – review & editing. KC: Data curation, Formal analysis, Methodology, Project administration, Resources, Investigation, Writing – original draft, Writing – review & editing. HH: Conceptualization, Formal analysis, Writing – review & editing. YX: Conceptualization, Formal analysis, Writing – review & editing.
Funding
The author(s) declare that no financial support was received for the research, authorship, and/or publication of this article.
Acknowledgments
The authors would like to express their heartfelt gratitude to the members of the research group, whose invaluable contributions made this paper possible. Their efforts and support throughout the research process have significantly enhanced the quality of this work and shaped its direction. We are deeply appreciative of their insights and expertise, which have improved this study in countless ways.
Conflict of interest
The authors declare that the research was conducted in the absence of any commercial or financial relationships that could be construed as a potential conflict of interest.
Publisher’s note
All claims expressed in this article are solely those of the authors and do not necessarily represent those of their affiliated organizations, or those of the publisher, the editors and the reviewers. Any product that may be evaluated in this article, or claim that may be made by its manufacturer, is not guaranteed or endorsed by the publisher.
References
1. HaugenBryan R, AlexanderErik K, BibleKeith C, DohertyGerard M, MandelSusan J, NikiforovYuri E, et al. 2015 American Thyroid Association management guidelines for adult patients with thyroid nodules and differentiated thyroid cancer: the American Thyroid Association guidelines task force on thyroid nodules and differentiated thyroid cancer. Thyroid. (2016). doi: 10.1089/thy.2015.0020
2. Maroulis DE, Savelonas MA, Iakovidis DK, Karkanis SA, Dimitropoulos N. Variable background active contour model for computer-aided delineation of nodules in thyroid ultrasound images. IEEE Trans Inf Technol Biomedicine. (2007) 11:537–43. doi: 10.1109/TITB.2006.890018
3. Savelonas MA, Iakovidis DK, Legakis I, Maroulis D. Active contours guided by echogenicity and texture for delineation of thyroid nodules in ultrasound images. IEEE Trans Inf Technol Biomedicine. (2008) 13:519–27. doi: 10.1109/TITB.2008.2007192
4. Wong KK, Zhang A, Yang K, Wu S, Ghista DN. GCW-U-Net segmentation of cardiac magnetic resonance images for evaluation of left atrial enlargement. Comput Methods Programs Biomedicine. (2022) 221:106915. doi: 10.1016/j.cmpb.2022.106915
5. Junying C, You H, Li K. A review of thyroid gland segmentation and thyroid nodule segmentation methods for medical ultrasound images. Comput Methods programs biomedicine. (2020) 185:105329. doi: 10.1016/j.cmpb.2020.105329
6. Jinlian M, Wu F, Jiang T, Zhao Q, Kong D. Ultrasound image-based thyroid nodule automatic segmentation using convolutional neural networks. Int J Comput assisted Radiol Surg. (2017) 12:1895–910. doi: 10.1007/s11548-017-1649-7
7. Gulame MB, Dixit VV, Suresh M. Thyroid nodules segmentation methods in clinical ultrasound images: a review. Materials Today: Proc. (2021) 45:2270–6.
8. Chan TF, Vese LA. Active contours without edges. IEEE Trans image Process. (2001) 10:266–77. doi: 10.1109/83.902291
9. Li C, Kao CY, Gore JC, Ding Z. Minimization of region-scalable fitting energy for image segmentation. IEEE Trans image Process. (2008) 17:1940–9. doi: 10.1109/TIP.2008.2002304
10. Zhao M, Wei Y, Lu Y, Wong KK. A novel U-Net approach to segment the cardiac chamber in magnetic resonance images with ghost artifacts. Comput Methods Programs Biomedicine. (2020) 196:105623. doi: 10.1016/j.cmpb.2020.105623
11. Zhu X, Wei Y, Lu Y, Zhao M, Yang K, Wu S, et al. Comparative analysis of active contour and convolutional neural network in rapid left-ventricle volume quantification using echocardiographic imaging. Comput Methods Programs Biomedicine. (2021) 199:105914. doi: 10.1016/j.cmpb.2020.105914
12. Wang J, Zhang R, Wei X, Li X, Yu M, Zhu J, et al. (2019). An attention-based semi-supervised neural network for thyroid nodules segmentation, in: 2019 IEEE International Conference on Bioinformatics and Biomedicine (BIBM), . pp. 871–6. IEEE.
13. Wu Y, Shen X, Bu F, Tian J. Ultrasound image segmentation method for thyroid nodules using ASPP fusion features. IEEE Access. (2020) 8:172457–66. doi: 10.1109/Access.6287639
14. Abdolali F, Kapur J, Jaremko JL, Noga M, Hareendranathan AR, Punithakumar K. Automated thyroid nodule detection from ultrasound imaging using deep convolutional neural networks. Comput Biol Med. (2020) 122:103871. doi: 10.1016/j.compbiomed.2020.103871
15. Oktay O, Schlemper J, Folgoc LL, Lee M, Heinrich M, Misawa K, et al. Attention u-net: Learning where to look for the pancreas. arXiv preprint arXiv:1804.03999. (2018).
16. Wong KK, Sun Z, Tu J, Worthley SG, Mazumdar J, Abbott D. Medical image diagnostics based on computer-aided flow analysis using magnetic resonance images. Computerized Med Imaging Graphics. (2012) 36:527–41. doi: 10.1016/j.compmedimag.2012.04.003
17. Fu J, Liu J, Tian H, Li Y, Bao Y, Fang Z, et al. (2019). Dual attention network for scene segmentation, in: Proceedings of the IEEE/CVF conference on computer vision and pattern recognition, pp. 3146–54.
18. Lee HJ, Kim JU, Lee S, Kim HG, Ro YM. (2020). Structure boundary preserving segmentation for medical image with ambiguous boundary, in: Proceedings of the IEEE/CVF conference on computer vision and pattern recognition, pp. 4817–26.
19. Zhong Z, Lin ZQ, Bidart R, Hu X, Daya IB, Li Z, et al. Squeeze-and-attention networks for semantic segmentation, in: Proceedings of the IEEE/CVF conference on computer vision and pattern recognition, (2020), pp. 13065–74.
20. Taisheng Z, Chipusu K, Zhu Y, Li M, Ibrahim UM, Huang J. Differential evolutionary optimization fuzzy entropy for gland segmentation based on breast mammography imaging. J Radiat Res Appl Sci. (2024) 17:100966.
21. Ronneberger O, Fischer P, Brox T. U-net: Convolutional networks for biomedical image segmentation, in: Medical Image Computing and Computer-Assisted Intervention–MICCAI 2015: 18th International Conference, Munich, Germany, October 5-9, 2015, Springer International Publishing. (2015). Vol. 18. pp. 234–41.
22. Azad R, Asadi-Aghbolaghi M, Fathy M, Escalera S. Attention deeplabv3+: Multi-level context attention mechanism for skin lesion segmentation, in: European conference on computer vision, Cham: IEEE Publishing. (2020). pp. 251–66.
23. Azad R, Asadi-Aghbolaghi M, Fathy M, Escalera S. Bi-directional ConvLSTM U-Net with densley connected convolutions, in: Proceedings of the IEEE/CVF international conference on computer vision workshops, Springer International Publishing. (2019).
24. Zhou Z, Rahman Siddiquee MM, Tajbakhsh N, Liang J. U-Net++: A nested u-net architecture for medical image segmentation, in: Deep Learning in Medical Image Analysis and Multimodal Learning for Clinical Decision Support: 4th International Workshop, DLMIA 2018, and 8th International Workshop, ML-CDS 2018, Held in Conjunction with MICCAI 2018, September 20, 2018. pp. 3–11. Granada, Spain: Springer International Publishing. (2018).
25. Wong KK, Fortino G, Abbott D. Deep learning-based cardiovascular image diagnosis: a promising challenge. Future Generation Comput Syst. (2020) 110:802–11. doi: 10.1016/j.future.2019.09.047
26. Wong KK. Cybernetical Intelligence: Engineering Cybernetics with Machine Intelligence. John Wiley & Sons (2023).
27. Wang K, Li G, Du S, Xi L, Xia T. State space modelling of variation propagation in multistage machining processes for variable stiffness structure workpieces. Int J Production Res. (2021) 59:4033–52. doi: 10.1080/00207543.2020.1757173
28. Ding J, Huang Z, Shi M, Ning C. 2019 12th International Congress on Image and Signal Processing, BioMedical Engineering and Informatics (CISP-BMEI). IEEE (2019). p. 1–5.
29. Szegedy C, Ioffe S, Vanhoucke V, Alemi A. Inception-v4, inception-resnet and the impact of residual connections on learning, in: Proceedings of the AAAI conference on artificial intelligence, Vol. 31. CMPB journal reference. (2017).
30. Kingma DP, Ba J. Adam: A method for stochastic optimization. arXiv preprint arXiv:1412.6980. (2014).
31. Du S, Xu R, Li L. Modeling and analysis of multiproduct multistage manufacturing system for quality improvement. IEEE Transaction Systems Man Cybernetics: Syst. (2018) 48:801–20. doi: 10.1109/TSMC.2016.2614766
Keywords: Swin U-Net, image segmentation, deep learning, image dataset, thyroid, ultrasound images
Citation: Yang C, Ashraf MA, Riaz M, Umwanzavugaye P, Chipusu K, Huang H and Xu Y (2025) Improving diagnostic precision in thyroid nodule segmentation from ultrasound images with a self-attention mechanism-based Swin U-Net model. Front. Oncol. 15:1456563. doi: 10.3389/fonc.2025.1456563
Received: 03 July 2024; Accepted: 02 January 2025;
Published: 06 February 2025.
Edited by:
Aleksandar Vakanski, University of Idaho, United StatesCopyright © 2025 Yang, Ashraf, Riaz, Umwanzavugaye, Chipusu, Huang and Xu. This is an open-access article distributed under the terms of the Creative Commons Attribution License (CC BY). The use, distribution or reproduction in other forums is permitted, provided the original author(s) and the copyright owner(s) are credited and that the original publication in this journal is cited, in accordance with accepted academic practice. No use, distribution or reproduction is permitted which does not comply with these terms.
*Correspondence: Yueqin Xu, eXVlcWluLnh1QG9jaWJlLmNvbQ==