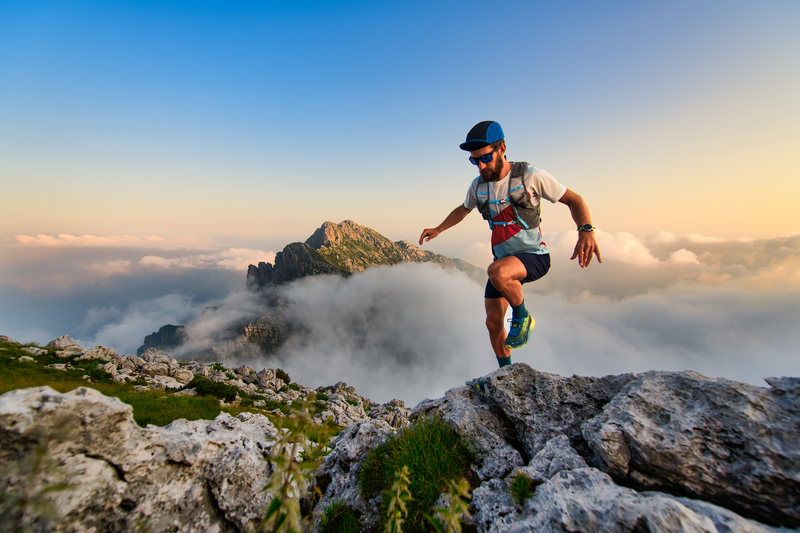
95% of researchers rate our articles as excellent or good
Learn more about the work of our research integrity team to safeguard the quality of each article we publish.
Find out more
ORIGINAL RESEARCH article
Front. Oncol. , 10 December 2024
Sec. Radiation Oncology
Volume 14 - 2024 | https://doi.org/10.3389/fonc.2024.1507806
Introduction: Following a preliminary work validating the technological feasibility of an adaptive workflow with Ethos for whole-breast cancer, this study aims to clinically evaluate the automatic segmentation generated by Ethos.
Material and methods: Twenty patients initially treated on a TrueBeam accelerator for different breast cancer indications (right/left, lumpectomy/mastectomy) were replanned using the Ethos® emulator. The adaptive workflow was performed using 5 randomly selected extended CBCTs per patient. The contours generated by artificial intelligence (AI) included both breasts, the heart, and the lungs. The target volumes, specifically the tumor bed (CTV_Boost), internal mammary chain (CTV_IMC), and clavicular nodes (CTV_Nodes), were generated through rigid propagation. The CTV_Breast corresponds to the ipsilateral breast, excluding 5mm from the skin. Two radiation oncologists independently repeated the workflow and qualitatively assessed the accuracy of the contours using a scoring system from 3 (contour to be redone) to 0 (no correction needed). Quantitative evaluation was carried out using the Dice Similarity Coefficient (DSC), Hausdorff Distance (HD), surface Dice (sDSC) and the Added Path Length (APL). The interobserver variability (IOV) between the two observers was also assessed and served as a reference. Lastly, the dosimetric impact of contour correction was evaluated. The physician-validated contours were transferred onto the plans automatically generated by Ethos in adaptive mode. The dose prescription was 52.2Gy in 18 fractions for the boost, 42.3Gy for the breast, IMC, and nodes. The CTV/PTV margin was 2mm for all volumes, except for the IMC (5mm). Dose coverage (D98%) was assessed for the CTVs, while specific parameters for organs at risk (OAR) were evaluated: mean dose and V17Gy (relative volume receiving at least 17Gy) for the ipsilateral lung, mean dose and D2cc (dose received by 2cc volume) for the heart, the contralateral lung and breast.
Results: The qualitative analysis showed that no correction or only minor corrections were needed for 98.6% of AI-generated contours and 86.7% of the target volumes. Regarding the quantitative analysis, Ethos’ contour generation outperformed inter-observer variability for all structures in terms of DSC, HD, sDSC and APL. Target volume coverage was achieved for 97.9%, 96.3%, 94.2% and 68.8% of the breast, IMC, nodes and boost CTVs, respectively. As for OARs, no significant differences in dosimetric parameters were observed.
Conclusion: This study shows high accuracy of segmentation performed by Ethos for breast cancer, except for the CTV_Boost. Contouring practices for adaptive sessions were revised following this study to improve outcomes.
Breast cancer (BC) is the most frequently diagnosed cancer and the leading cause of cancer-related death in women worldwide, according to the latest GLOBOCAN study (1). Breast-conserving surgery followed by whole breast irradiation is the current standard of care for patients with early stage BC (2). In recent years, advanced radiotherapy techniques such as Intensity Modulated Radiation Therapy (IMRT), Simultaneous Integrated Boost irradiation or deep-inspiration breath hold have played a crucial role in improving the precision of radiation delivery to tumors. These techniques effectively maximize the target dose while minimizing toxicity to normal tissues and sparing surrounding organs at risk (OAR) (3–6).
Adaptive radiotherapy (ART) is also being investigated for this indication (7). In fact, breast cancer patients have many anatomical variations: heart movement, breathing, arm position can all lead to changes in breast position and shape, as can seroma and swelling following radiation or surgery (8). The ART would therefore allow treatment margins to be reduced, and consequently the volume irradiated. Nevertheless, segmentation remains a key step in online ART (oART), as it must be as short as possible while ensuring high delineation accuracy, given the significant consequences of radiation treatment errors.
With the advent of artificial intelligence (AI) and deep learning (DL), the accuracy of auto-segmentation has improved significantly, particularly for organs at risk, and its use in routine clinical practice is now widespread (9). Various commercial software solutions are available for auto-segmentation and offer high-quality, consistent contours with comparable performance (10, 11). However, delineation of the clinical target volume (CTV) is more challenging due to factors such as physician experience, contouring techniques, and variations in delineation guidelines (12–14). As a result, manual peer review and quality assurance procedures are still recommended before clinical application (15, 16).
The Varian Ethos system (Varian Medical Systems, Palo Alto, CA, USA) uses Cone Beam Computed Tomography (CBCT)for oART and employs DL-based algorithm and structure-guided deformation for structure segmentation (17). So far, Ethos’ experience with oART for breast cancer has been limited to partial breast irradiation (18, 19) and our own technological feasibility study for whole-breast irradiation (20). To our knowledge, this is the first clinical study to evaluate the performance of Ethos auto-segmentation in breast cancer with regional lymph nodes.
This study was conducted using data from 20 patients who were treated for invasive breast cancer between November 2021 and December 2022 on a TrueBeam accelerator. Patients were included regardless of age, histological subtype, tumor grade, type of surgery (lumpectomy or mastectomy), or whether they received neoadjuvant chemotherapy. Patient characteristics are summarized in Table 1.
Table 1. Patient characteristics, including treatment side, type of surgery, and volume of breast/chest wall CTV.
Patients underwent Computed Tomography (CT) scans (GE Optima CT580, General Electric Healthcare, Waukesha, WI, USA) with a 2.5 mm slice thickness, in the supine position, free breathing, with both arms positioned above the head and supported by a personalized foam cushion.
The ESTRO consensus guidelines (21, 22) were used to delineate target volumes, breast/wall, and axillary (Berg I); subclavicular (Berg II, III) and supraclavicular (Berg IV) lymph nodes (Nodes hereafter); and internal mammary chain (IMC). Organs at risk were delineated following French RecoRad 2022 (23) recommendations using TheraPanacea software (24).
The prescribed doses for target volumes were 52.2 Gy for the tumor bed (boost) and 42.3 Gy for the breast, internal mammary chain (CTV_IMC), and clavicular lymph nodes (CTV_Nodes) over 18 fractions. CTV-PTV margins were set at 2 mm for all areas except for the IMC, where a 5 mm margin was applied. Dose constraints for the CTVs and organs at risk (OAR) are outlined in Table 2. The dose prescription, PTV margins, and dose constraints were based on the clinical trial “Adaptive radiotherapy in hypersensitive and high locoregional risk breast cancer (SAHARA-04).” (25).
The Ethos adaptive workflow for breast cancer was reproduced using a Varian Ethos emulator (v1.1, Varian Medical Systems, Palo Alto, CA). The AI generates the contours of the influencer structures (also known as organs that influence on the shape and position of the target), namely the right and left breasts (or chest walls), both lungs and the heart. The Varian’s in house AI-based algorithm uses convolutional neural networks to create the influencers (17). The contours of the target volumes are then generated by elastic or rigid registration, according to the user’s choice, to define the CTV_Boost, the CTV_IMC and the CTV_Nodes. The target propagation is based on structure-guided deformations (resulting from the influencer and bone structures generated in the previous step). Our previous study showed a better contour accuracy with rigid propagation and will be the reference propagation for this study (20). The CTV_Breast and CTV_Chestwall are derived structures from the breast and chestwall excluding the 5mm beneath the skin.
For each patient, 5 extended CBCTs performed initially for their treatment, were randomly selected in order to simulate 5 adaptive sessions. First, two radiation oncologists independently repeated the adaptive workflow and each Ethos’ auto-segmentation contour (influencers and target volumes) were reviewed. A qualitative evaluation using a physician’s rating was performed for each structure and each adaptive session (i.e 8 structures per CBCT and a total of 100 CBCTs). The scores were defined as follows:
0- No correction needed
1- Minor corrections (≤ 25% of the structure volume)
2-Major corrections (>25% of the structure volume)
3- Contour not usable
Once the score was assigned, the radiation oncologists corrected the contours online, if necessary, by comparing them with the original contours delineated on the simulation CT. A quantitative study was then carried out by comparing the automatically generated contours and the physicians’ contours using similarity metrics. The Dice similarity coefficient (DSC) and the Hausdorff distance (HD) were chosen because of their frequent use in the literature (26, 27) and their complementary properties. However, these two metrics do not correlate with the time required to edit contours. The surface Dice similarity coefficient (sDSC) (28) and the added path length (APL) (29) are more suitable for evaluating time savings (29). Unlike the DSC, which measures on the overlap between two volumes, the sDSC measures the similarity between two surfaces. The APL, on the other hand, is defined as the additional distance to overlap the two contours. The interobserver variability (IOV) between the two physicians was also assessed and served as a reference.
The automated planning process resulted in the adapted plan generated by Ethos without editing contours. When evaluating the resulting dose to the physicians’ contours, several dose-volume histogram parameters were analyzed using some dose constraints provided by the SAHARA-04 protocol and some additional relevant parameters. To study the target volume coverage (boost, breast/chestwall, IMC and CLN), the dose received by 98% of the volume (D98%) was used. For the organs at risk (OAR), the mean dose and the doses received by 2cc (D2cc) was recorded for the heart, contralateral breast and both lungs; the volume receiving 17 Gy (V17Gy) for the ipsilateral lung was evaluated too. The Mann-Whitney test was applied to assess the significant differences between the doses received by the Ethos contours and the physician contours.
Figure 1 shows the distribution of qualitative scores assigned by the two radiation oncologists to the structures generated by AI (influencers) and the CTVs produced through rigid propagation. In total, 98.6% of the influencers required no or minor adjustments, compared to 86.7% of the CTV contours. The lowest score was observed for the CTV_Boost with 22.2% of the contours considered not usable or requiring major corrections.
Quantitative results, including DSC, HD, sDSC, and APL for the Ethos contours, along with the corresponding inter-observer variability (IOV), are displayed as boxplots in Figure 2. For the influencer structures (i.e., both breasts, both lungs, and the heart), the DSC and sDSC median values exceeded 0.9, while the HD median values were below 20mm. The median APLs showed the highest values for both lungs. Additionally, autocontours consistently outperformed inter-observer variability across all metrics. For the CTVs propagated rigidly, the median DSC and sDSC were above 0.8, and the median HD was less than 10mm. The median APLs achieved the highest values for CTV_Nodes. Once again, autocontours outperformed inter-observer variability across all metrics. Figure 3 shows an example of CTV_IMC delineation comparison with the worst DSC results (DSCauto vs Med1 = 0.34; DSCauto vs Med2 = 0.44; DSVIOV = 0.56) in axial (left) and sagittal (right) slices.
Figure 2. Boxplots of DSC, HD, sDSC and APL for the structures generated by AI (left column) and the structures rigidly propagated (right column). Purple boxplots correspond to the Ethos contours while grey boxplots show the IOV results.
Figure 3. Example of CTV_IMC delineation with the worst DSC results (DSCauto vs Med1 = 0.34; DSCauto vs Med2 = 0.44; DSVIOV = 0.56) in axial (left) and sagittal (right) slices.
The comparison of dose metrics for the OARs is presented in Table 3. The dose differences between the auto-generated and physician contours were less than 0.1 Gy, except for the heart, where an increase of 0.6 Gy was observed for D2cc. However, no statistically significant difference was found.
Table 3. Comparison of dose metrics (average ± standard deviation) between auto and physicians’ contours delineated for OARs.
The results of the dosimetric evaluation of the CTVs are shown in Figure 4. The CTV coverage constraints, based on D98% > 95% for the CTV_Boost and CTV_Breast, and D98% > 90% for the CTV_IMC and CTV_Nodes, were met for 97.9% of the breast structures, 68.8% of the tumor bed structures, 96.3% of the IMC structures, and 94.2% of the CLN structures. Figure 5 presents the previous example with the lowest DSC results (Figure 4), yet the CTV_IMC coverage still meets the dosimetric constraint (D98% > 90%).
Figure 4. Percentage of sessions fulfilling the coverage constraint: D98% > 95% for CTV_Breast and CTV_Boost, D95% > 90% for CTV_IMC and CTV_Nodes.
Figure 5. Example of CTV_IMC coverage with the worst DSC results but acceptable CTV dose constraint (D98% Med1 = 96.2%; D98% Med2 = 97%; D98%Auto = 97.4%).
The aim of this study was to evaluate the clinical application of Ethos auto-segmentation for online whole breast ART and the dosimetric impact of physician corrections. While the performance of the Ethos auto-segmentation in the pelvic region is well documented (30–34), there are currently no published studies focusing on its use in whole-breast cancer.
First, the practical relevance was demonstrated by geometric similarity metrics that exceeded inter-observer variation, with most structures needing no or minor adjustment. The Ethos system generated highly accurate influencer structures, with DSC values above 0.9 and HD values below 20 mm. The highest APL was achieved for the lungs due to their large volume, and according to the Vaassen correlation (29), the lungs would require the most correction time. Regarding the structures produced through rigid propagation (i.e CTV), the DSC and HD results are satisfactory with values superior to 0.8 and below 10mm, respectively. CTV_Nodes was the structure that required more time for correction due to the highest APL. Our results are in agreement with the other DL-based segmentation algorithms (35–40). Almberg et al. (35) trained and validated their own DL-segmentation model and achieved median DSC values of 0.96, 0.98 and 0.94 for the heart, both lungs and breast, respectively. For the CTV (IMC and Nodes), their results ranged from 0.70 to 0.81, which were lower than those in our study. This discrepancy is likely due to the different methods used to generate these structures: Ethos uses rigid propagation for CTVs, whereas Almberg et al. employed DL-based segmentation.
Evaluating the dosimetric impact of contour corrections is a key step in assessing system performance, particularly in an online adaptive workflow where time constraints are critical (16). For example, although the lung structure had the highest APL median value, correction were deemed unnecessary due to their minimal dosimetric impact. In contrast, the CTV_IMC showed the largest deviation in similarity metrics relative to its volume, yet dose coverage remained unaffected, largely because a larger PTV margin of 5 mm (compared to 2 mm for other CTVs) was used to account for segmentation uncertainties. The most significant dosimetric impact was observed for CTV_Boost, with only 68.8% of the structures meeting the dose coverage requirement. Despite a standardized protocol for surgical clipping of the breast tumor bed to facilitate accurate localization of the CTV, CTV_Boost delineation in our department is performed manually and is subject to interpretation. In addition, accurate assessment of the tumor bed on CBCT images, as required by the Ethos adaptive workflow, can be challenging due to poor image quality, particularly in soft tissue. Recently, Li et al. (41) developed a contrast learning-based generative model to generate of high-quality synthetic CT from low-quality CBCT and evaluated its performance for post-breast-conserving patients. This method improved the target delineation, such as the tumor bed region. In addition, the newly commercialized CBCT technology, the HyperSight imaging solution (Varian Medical Systems), has shown superior image quality compared to previous imaging on the Ethos platform, along with HU accuracy sufficient for direct dose calculation using the acquired image data (42) and could improve the target delineation in soft tissue (43, 44). However, its performance has not yet been assessed for breast cancer.
As a result of this study, a change in contouring practice for CTV_Boost was implemented. In adaptive treatments, CTV_Boost is now defined by an automatic expansion around the surgical clips, which are more easily visible on CBCT images. Additionally, a procedural guide was created to assist radiation oncologists in managing the breast oART workflow, which includes the following guidelines:
- No correction required for influencer structures (heart, both lungs, and breasts)
- No correction needed for CTV_IMC
- Special attention should be given to the CTV, allowing for rigid movement of the structure (no deformation)
- Verify the surgical clip delineation for CTV_Boost
The primary limitation of this study was the relatively small cohort of 20 patients. Notably, no specific anatomies, such as breast expanders, which could challenge Ethos segmentation performance, were included. Additionally, the study utilized CBCT data from a TrueBeam accelerator instead of the Ethos system as whole-breast treatments were only performed on a C-Arm linac during the study period in our department. However, Cai et al. (45) demonstrated that O-ring CBCT offers equivalent or superior image quality compared to C-Arm CBCT images. Therefore, it is reasonable to assume that the contours generated by Ethos on CBCT images from both the TrueBeam and the Ethos systems are comparable, if not improved.
This study demonstrated the high accuracy of segmentation performed by Ethos for breast cancer, with the exception of the CTV_Boost. Following this study, contouring practices for adaptive sessions were revised to enhance outcomes and reduce the segmentation workload.
The original contributions presented in the study are included in the article/supplementary material. Further inquiries can be directed to the corresponding author.
JP: Conceptualization, Investigation, Methodology, Writing – original draft. FM: Data curation, Writing – review & editing. PL: Data curation, Writing – review & editing. AM: Writing – review & editing. PH: Software, Writing – review & editing. CB: Writing – review & editing. DA: Writing – review & editing. PF: Conceptualization, Methodology, Writing – review & editing.
The author(s) declare that no financial support was received for the research, authorship, and/or publication of this article.
The authors declare that the research was conducted in the absence of any commercial or financial relationships that could be construed as a potential conflict of interest.
The author(s) declared that they were an editorial board member of Frontiers, at the time of submission. This had no impact on the peer review process and the final decision.
The authors declare that no Generative AI was used in the creation of this manuscript.
All claims expressed in this article are solely those of the authors and do not necessarily represent those of their affiliated organizations, or those of the publisher, the editors and the reviewers. Any product that may be evaluated in this article, or claim that may be made by its manufacturer, is not guaranteed or endorsed by the publisher.
1. Bray F, Laversanne M, Sung H, Ferlay J, Siegel RL, Soerjomataram I, et al. Global cancer statistics 2022: GLOBOCAN estimates of incidence and mortality worldwide for 36 cancers in 185 countries. CA Cancer J Clin. (2024) 74:229–63. doi: 10.3322/caac.21834
2. Maughan KL, Lutterbie MA, Ham PS. Treatment of breast cancer. Am Fam Physician. (2010) 81:1339–46.
3. Racka I, Majewska K, Winiecki J. Three-dimensional conformal radiotherapy (3D-CRT) vs. volumetric modulated arc therapy (VMAT) in deep inspiration breath-hold (DIBH) technique in left-sided breast cancer patients-comparative analysis of dose distribution and estimation of projected secondary cancer risk. Strahlenther Onkol Organ Dtsch Rontgengesellschaft Al. (2023) 199:90–101. doi: 10.1007/s00066-022-01979-2
4. Popescu CC, Olivotto IA, Beckham WA, Ansbacher W, Zavgorodni S, Shaffer R, et al. Volumetric modulated arc therapy improves dosimetry and reduces treatment time compared to conventional intensity-modulated radiotherapy for locoregional radiotherapy of left-sided breast cancer and internal mammary nodes. Int J Radiat Oncol Biol Phys. (2010) 76:287–95. doi: 10.1016/j.ijrobp.2009.05.038
5. Osman SOS, Hol S, Poortmans PM, Essers M. Volumetric modulated arc therapy and breath-hold in image-guided locoregional left-sided breast irradiation. Radiother Oncol J Eur Soc Ther Radiol Oncol. (2014) 112:17–22. doi: 10.1016/j.radonc.2014.04.004
6. Sarkar B, Pradhan A. Planning system-dependent recommendations of intensity-modulated technique for breast radiotherapy: A literature review-based adaptation and institutional dosimetric experience from a large-volume tertiary cancer care hospital. J Med Phys. (2023) 48:221. doi: 10.4103/jmp.jmp_51_23
7. De-Colle C, Kirby A, Russell N, Shaitelman SF, Currey A, Donovan E, et al. Adaptive radiotherapy for breast cancer. Clin Transl Radiat Oncol. (2023) 39:100564. doi: 10.1016/j.ctro.2022.100564
8. Iezzi M, Cusumano D, Piccari D, Menna S, Catucci F, D’Aviero A, et al. Dosimetric impact of inter-fraction variability in the treatment of breast cancer: towards new criteria to evaluate the appropriateness of online adaptive radiotherapy. Front Oncol. (2022) 12:838039. doi: 10.3389/fonc.2022.838039
9. Erdur AC, Rusche D, Scholz D, Kiechle J, Fischer S, Llorián-Salvador Ó, et al. Deep learning for autosegmentation for radiotherapy treatment planning: State-of-the-art and novel perspectives. Strahlenther Onkol Organ Dtsch Rontgengesellschaft Al. (2024). doi: 10.1007/s00066-024-02262-2
10. Doolan PJ, Charalambous S, Roussakis Y, Leczynski A, Peratikou M, Benjamin M, et al. A clinical evaluation of the performance of five commercial artificial intelligence contouring systems for radiotherapy. Front Oncol. (2023) 13:1213068. doi: 10.3389/fonc.2023.1213068
11. Goddard L, Velten C, Tang J, Skalina KA, Boyd R, Martin W, et al. Evaluation of multiple-vendor AI autocontouring solutions. Radiat Oncol Lond Engl. (2024) 19:69. doi: 10.1186/s13014-024-02451-4
12. Struikmans H, Wárlám-Rodenhuis C, Stam T, Stapper G, Tersteeg RJHA, Bol GH, et al. Interobserver variability of clinical target volume delineation of glandular breast tissue and of boost volume in tangential breast irradiation. Radiother Oncol J Eur Soc Ther Radiol Oncol. (2005) 76:293–9. doi: 10.1016/j.radonc.2005.03.029
13. Vinod SK, Jameson MG, Min M, Holloway LC. Uncertainties in volume delineation in radiation oncology: A systematic review and recommendations for future studies. Radiother Oncol J Eur Soc Ther Radiol Oncol. (2016) 121:169–79. doi: 10.1016/j.radonc.2016.09.009
14. Song YC, Yan XN, Tang Y, Jing H, Zhang N, Zhang J, et al. Variability of target volumes and organs at risk delineation in breast cancer radiation therapy: quality assurance results of the pretrial benchmark case for the POTENTIAL trial. Pract Radiat Oncol. (2022) 12:397–408. doi: 10.1016/j.prro.2021.12.018
15. Marks LB, Adams RD, Pawlicki T, Blumberg AL, Hoopes D, Brundage MD, et al. Enhancing the role of case-oriented peer review to improve quality and safety in radiation oncology: Executive summary. Pract Radiat Oncol. (2013) 3:149–56. doi: 10.1016/j.prro.2012.11.010
16. Vandewinckele L, Claessens M, Dinkla A, Brouwer C, Crijns W, Verellen D, et al. Overview of artificial intelligence-based applications in radiotherapy: Recommendations for implementation and quality assurance. Radiother Oncol J Eur Soc Ther Radiol Oncol. (2020) 153:55–66. doi: 10.1016/j.radonc.2020.09.008
17. Archambault Y, Boylan C, Bullock D, Morgas T, Peltola J, Ruokokoski E, et al. Making on-line adaptive radiotherapy possible using artificial intelligence and machine learning for efficient daily re-planning. Med Phys Intl J. (2020).
18. Pogue JA, Cardenas CE, Harms J, Soike MH, Kole AJ, Schneider CS, et al. Benchmarking automated machine learning-enhanced planning with ethos against manual and knowledge-based planning for locally advanced lung cancer. Adv Radiat Oncol. (2023) 8:101292. doi: 10.1016/j.adro.2023.101292
19. Montalvo SK, Kim N, Nwachukwu C, Alluri P, Parsons D, Lin MH, et al. On the feasibility of improved target coverage without compromising organs at risk using online adaptive stereotactic partial breast irradiation (A-SPBI). J Appl Clin Med Phys. (2023) 24:e13813. doi: 10.1002/acm2.13813
20. Galand A, Prunaretty J, Mir N, Morel A, Bourgier C, Aillères N, et al. Feasibility study of adaptive radiotherapy with Ethos for breast cancer. Front Oncol. (2023) 13:1274082. doi: 10.3389/fonc.2023.1274082
21. Offersen BV, Boersma LJ, Kirkove C, Hol S, Aznar MC, Sola AB, et al. ESTRO consensus guideline on target volume delineation for elective radiation therapy of early stage breast cancer, version 1.1. Radiother Oncol J Eur Soc Ther Radiol Oncol. (2016) 118:205–8. doi: 10.1016/j.radonc.2015.12.027
22. Offersen BV, Boersma LJ, Kirkove C, Hol S, Aznar MC, Biete Sola A, et al. ESTRO consensus guideline on target volume delineation for elective radiation therapy of early stage breast cancer. Radiother Oncol J Eur Soc Ther Radiol Oncol. (2015) 114:3–10. doi: 10.1016/j.radonc.2014.11.030
23. Noël G, Le Fèvre C, Antoni D. Delineation of organs at risk. Cancer Radiother. (2022) 26:76–91. doi: 10.1016/j.canrad.2021.08.001
24. Ung M, Rouyar-Nicolas A, Limkin E, Petit C, Sarrade T, Carre A, et al. Improving radiotherapy workflow through implementation of delineation guidelines & AI-based annotation. Int J Radiat Oncol Biol Phys. (2020) 108:e315. doi: 10.1016/j.ijrobp.2020.07.753
25. Institut du Cancer de Montpellier - Val d’Aurelle. SAHARA-04 : adaptive radiotherapy in hypersensitive patients and high locoregional risk breast cancer with ETHOS technology (SAHARA-04). (2023). Available at: https://clinicaltrials.gov/study/NCT06053086.
26. Chao PJ, Chang L, Kang CL, Lin CH, Shieh CS, Wu JM, et al. Using deep learning models to analyze the cerebral edema complication caused by radiotherapy in patients with intracranial tumor. Sci Rep. (2022) 12:1555. doi: 10.1038/s41598-022-05455-w
27. Yu Y, Jiang H, Zhang X, Chen Y. Identifying irregular potatoes using hausdorff distance and intersection over union. Sensors. (2022) 22:5740. doi: 10.3390/s22155740
28. Nikolov S, Blackwell S, Zverovitch A, Mendes R, Livne M, De Fauw J, et al. Clinically applicable segmentation of head and neck anatomy for radiotherapy: deep learning algorithm development and validation study. J Med Internet Res. (2021) 23:e26151. doi: 10.2196/26151
29. Vaassen F, Hazelaar C, Vaniqui A, Gooding M, van der Heyden B, Canters R, et al. Evaluation of measures for assessing time-saving of automatic organ-at-risk segmentation in radiotherapy. Phys Imaging Radiat Oncol. (2020) 13:1–6. doi: 10.1016/j.phro.2019.12.001
30. Branco D, Mayadev J, Moore K, Ray X. Dosimetric and feasibility evaluation of a CBCT-based daily adaptive radiotherapy protocol for locally advanced cervical cancer. J Appl Clin Med Phys. (2023) 24:e13783. doi: 10.1002/acm2.13783
31. Åström LM, Behrens CP, Calmels L, Sjöström D, Geertsen P, Mouritsen LS, et al. Online adaptive radiotherapy of urinary bladder cancer with full re-optimization to the anatomy of the day: Initial experience and dosimetric benefits. Radiother Oncol J Eur Soc Ther Radiol Oncol. (2022) 171:37–42. doi: 10.1016/j.radonc.2022.03.014
32. Åström LM, Behrens CP, Storm KS, Sibolt P, Serup-Hansen E. Online adaptive radiotherapy of anal cancer: Normal tissue sparing, target propagation methods, and first clinical experience. Radiother Oncol J Eur Soc Ther Radiol Oncol. (2022) 176:92–8. doi: 10.1016/j.radonc.2022.09.015
33. Moazzezi M, Rose B, Kisling K, Moore KL, Ray X. Prospects for daily online adaptive radiotherapy via ethos for prostate cancer patients without nodal involvement using unedited CBCT auto-segmentation. J Appl Clin Med Phys. (2021) 22:82–93. doi: 10.1002/acm2.v22.10
34. Sibolt P, Andersson LM, Calmels L, Sjöström D, Bjelkengren U, Geertsen P, et al. Clinical implementation of artificial intelligence-driven cone-beam computed tomography-guided online adaptive radiotherapy in the pelvic region. Phys Imaging Radiat Oncol. (2021) 17:1–7. doi: 10.1016/j.phro.2020.12.004
35. Almberg SS, Lervåg C, Frengen J, Eidem M, Abramova TM, Nordstrand CS, et al. Training, validation, and clinical implementation of a deep-learning segmentation model for radiotherapy of loco-regional breast cancer. Radiother Oncol. (2022) 173:62–8. doi: 10.1016/j.radonc.2022.05.018
36. Bakx N, van der Sangen M, Theuws J, Bluemink J, Hurkmans C. Comparison of the use of a clinically implemented deep learning segmentation model with the simulated study setting for breast cancer patients receiving radiotherapy. Acta Oncol Stockh Swed. (2024) 63:477–81. doi: 10.2340/1651-226X.2024.34986
37. Byun HK, Chang JS, Choi MS, Chun J, Jung J, Jeong C, et al. Evaluation of deep learning-based autosegmentation in breast cancer radiotherapy. Radiat Oncol Lond Engl. (2021) 16:203. doi: 10.1186/s13014-021-01923-1
38. Tsui T, Podgorsak A, Roeske JC, Small W, Refaat T, Kang H. Geometric and dosimetric evaluation for breast and regional nodal auto-segmentation structures. J Appl Clin Med Phys. (2024) 25:e14461. doi: 10.1002/acm2.v25.10
39. Chung SY, Chang JS, Choi MS, Chang Y, Choi BS, Chun J, et al. Clinical feasibility of deep learning-based auto-segmentation of target volumes and organs-at-risk in breast cancer patients after breast-conserving surgery. Radiat Oncol Lond Engl. (2021) 16:44. doi: 10.1186/s13014-021-01771-z
40. Choi MS, Choi BS, Chung SY, Kim N, Chun J, Kim YB, et al. Clinical evaluation of atlas- and deep learning-based automatic segmentation of multiple organs and clinical target volumes for breast cancer. Radiother Oncol J Eur Soc Ther Radiol Oncol. (2020) 153:139–45. doi: 10.1016/j.radonc.2020.09.045
41. Li N, Zhou X, Chen S, Dai J, Wang T, Zhang C, et al. Incorporating the synthetic CT image for improving the performance of deformable image registration between planning CT and cone-beam CT. Front Oncol. (2023) 13:1127866/full. doi: 10.3389/fonc.2023.1127866/full
42. Available online at: https://varian.widen.net/s/sd5kdw7wxv/hypersight_brochure_rad11063_oct2022 (accessed October 20, 2024).
43. Duan J, Pogue JA, Boggs DH, Harms J. Enhancing precision in radiation therapy for locally advanced lung cancer: A case study of cone-beam computed tomography (CBCT)-based online adaptive techniques and the promise of hyperSightTM iterative CBCT. Cureus. (2024) 16:e66943. doi: 10.7759/cureus.66943
44. Kunnen B, van de Schoot A JAJ, Fremeijer KP, Nicolai-Koornneef EM, Offereins-van Harten K, Sluijter JH, et al. The added value of a new high-performance ring-gantry CBCT imaging system for prostate cancer patients. Radiother Oncol J Eur Soc Ther Radiol Oncol. (2024) 200:110458. doi: 10.1016/j.radonc.2024.110458
Keywords: Ethos, auto-segmentation, artificial intelligence, breast cancer, adaptive treatment
Citation: Prunaretty J, Mekki F, Laurent P-I, Morel A, Hinault P, Bourgier C, Azria D and Fenoglietto P (2024) Clinical feasibility of Ethos auto-segmentation for adaptive whole-breast cancer treatment. Front. Oncol. 14:1507806. doi: 10.3389/fonc.2024.1507806
Received: 08 October 2024; Accepted: 21 November 2024;
Published: 10 December 2024.
Edited by:
Valdir Carlos Colussi, University Hospitals Cleveland Medical Center, United StatesReviewed by:
Giuseppe Carlo Iorio, University of Turin, ItalyCopyright © 2024 Prunaretty, Mekki, Laurent, Morel, Hinault, Bourgier, Azria and Fenoglietto. This is an open-access article distributed under the terms of the Creative Commons Attribution License (CC BY). The use, distribution or reproduction in other forums is permitted, provided the original author(s) and the copyright owner(s) are credited and that the original publication in this journal is cited, in accordance with accepted academic practice. No use, distribution or reproduction is permitted which does not comply with these terms.
*Correspondence: Jessica Prunaretty, amVzc2ljYS5wcnVuYXJldHR5QGljbS51bmljYW5jZXIuZnI=
†These authors have contributed equally to this work
Disclaimer: All claims expressed in this article are solely those of the authors and do not necessarily represent those of their affiliated organizations, or those of the publisher, the editors and the reviewers. Any product that may be evaluated in this article or claim that may be made by its manufacturer is not guaranteed or endorsed by the publisher.
Research integrity at Frontiers
Learn more about the work of our research integrity team to safeguard the quality of each article we publish.