- 1Department of Neurosurgery, Mater Olbia Hospital, Olbia, Italy
- 2Institute of Neurosurgery, Fondazione Policlinico Universitario A. Gemelli IRCCS, Catholic University, Rome, Italy
- 3Neurosurgery Unit, Head-Neck and NeuroScience Department, University Hospital of Udine, Udine, Italy
- 4Advanced Radiodiagnostics Centre, Unità Operativa Semplice Dipartimentale (UOSD) Neuroradiology, Fondazione Policlinico Universitario Agostino Gemelli IRCSS, Rome, Italy
- 5Department of Neuroradiology, Azienda Sanitaria Universitaria Friuli Centrale (ASUFC) “Santa Maria Della Misericordia”, Udine, Italy
- 6Department of Radiology, Radiation Oncology and Hematology, Fondazione Policlinico Universitario Agostino Gemelli IRCSS, Rome, Italy
- 7Medical Physics Unit, Mater Olbia Hospital, Olbia, Italy
- 8Institute of Neurosurgery, Fondazione Policlinico Universitario Agostino Gemelli IRCSS, Rome, Italy
Purpose: Patients diagnosed with High Grade Gliomas (HGG) generally tend to have a relatively negative prognosis with a high risk of early tumor recurrence (TR) after post-operative radio-chemotherapy. The assessment of the pre-operative risk of early versus delayed TR can be crucial to develop a personalized surgical approach. The purpose of this article is to predict TR using MRI radiomic analysis.
Methods: Data were retrospectively collected from a database. A total of 248 patients were included based on the availability of 6-month TR results: 188 were used to train the model, the others to externally validate it. After manual segmentation of the tumor, Radiomic features were extracted and different machine learning models were implemented considering a combination of T1 and T2 weighted MR sequences. Receiver Operating Characteristic (ROC) curve was calculated with relative model performance metrics (accuracy, sensitivity, specificity, positive predictive value (PPV) and negative predictive value (NPV)) at the best threshold based on the Youden Index.
Results: Models performance were evaluated based on test set results. The best model resulted to be the XGBoost, with an area under ROC curve of 0.72 (95% CI: 0.56 - 0.87). At the best threshold, the model exhibits 0.75 (95% CI: 0.63 - 0.75) as accuracy, 0.62 (95% CI: 0.38 - 0.83) as sensitivity 0.80 (95% CI: 0.66 - 0.89 as specificity, 0.53 (95% CI: 0.31 - 0.73) as PPV, 0.88 (95% CI: 0.72 - 0.94) as NPV.
Conclusion: MRI radiomic analysis represents a powerful tool to predict late HGG recurrence, which can be useful to plan personalized surgical treatments and to offer pertinent patient pre-operative counseling.
Introduction
Despite recent advances in surgical technology and genetic discoveries, patients diagnosed with High Grade Gliomas (HGG), which is considered as grade 4, generally have a dismal prognosis with high risk of early tumor recurrence (TR) after post-operative radio-chemotherapy (1–4).
Compelling evidence, based on objective tumoral volume analysis, supports the role of the extent of resection (EOR) in HGG patients as the first step of patients management (5, 6).
Recent technological innovations have increased the safety of surgical resection, while expanding surgical options and indications for HGG surgical treatment (7, 8): several techniques can be currently used during the surgical procedure, such as intraoperative ultrasound (iUS), cortical mapping, sodium fluorescein (9) and 5-ALA fluorescence, with the aim of fostering higher rates of total resection and so increasing the survival chance (10–19).
Surgical treatment, however, can be rarely considered as radical, due to the infiltrating tumor nature, its multifocal presentation, and ill-defined tumor margins.
Although the Stupp protocol was introduced as post-operative standard treatment more than 15 years ago, alternative recent approaches have not been developed so far, and the 5-year survival has not significantly changed in these last decades (20).
Unfortunately, the infiltrative growth, the rapid proliferative rate of malignant cells and the appearance of treatment-resistant cell clones shortly after initial therapy tend to recur within 2 cm of resection margins, independently by the initial EOR exhibited by the patient (21). In this challenging setting, assessment of pre-operative risk of early versus delayed TR assumes a crucial role to develop a personalized surgical approach (with respect to surgery versus biopsy).
A presurgical identification of HGG patients with high risk of recurrence after 6 months from surgery may have several advantages (22): first of all, a more aggressive surgical resection may be pursued in patients with low risk of TR, planning the use of all intra-operative tools and strategies that allow a maximal safe resection. Furthermore, deep genetic sequences may be considered to assist clinicians during postsurgical decision-making involving patients with high risk of TR.
In addition, detection of early TR risk should encourage efforts to better understand the role of early intensified bridging therapies for HGG between surgery and postsurgical treatments (23).
Radiomics is a field of medical imaging that focuses on the extraction and advanced analysis of large amounts of quantitative features from medical images called “radiomic features”. The goal of radiomics is to convert medical images into high-dimensional data that can be analyzed to uncover underlying patterns related to disease characteristics, prognosis, and treatment response (24).
The aim of this study is to develop a Machine Learning (ML) model based on radiomics features extracted from MRI images able to stratify the risk of TR (within 6 months) in newly diagnosed HGG and support clinicians in the decision-making process.
Materials and methods
Patient population and image data
This retrospective study was focused on patients affected by high grade glioma, which is defined as grade 4, enrolled in two Institutions: Fondazione Policlinico Universitario Agostino Gemelli IRCCS in Rome (FPG) and Santa Maria della Misericordia in Udine (SMM).
The experimental protocol adopted in this study was approved by the Ethical Committee of Policlinico Universitario Agostino Gemelli IRCCS.
The informed consent was obtained for all the patients included in the study.
Patients from FPG were treated from January 2016 to December 2019, patients from SMM from November 2014 to June 2020. Tumor grading was defined considering the 2021 WHO staging (25).
Inclusion criteria were: age >/= 18 years; no previous surgery; no preoperative chemo- or radiotherapy; at least 6 months of follow up; objective evaluation of preoperative tumor volume on MRI images in DICOM format based on post-contrast T1-weighted MRI sequences and T2-weighted MRI sequences; objective estimation of EOR on post-contrast T1-weighted MRI sequences; revision of histopathological specimens by using the new 2021 WHO Classification of Tumors of the Central Nervous System; MGMT promoter methylation and IDH1/IDH2 mutation status assessment.
All the patients were subjected to the following therapeutic approach: one month before the surgical procedure, all the patients were scanned with an MR acquisition, which was carried out using the GE and Philips scanners 1.5 T in Rome and the Siemens scanner 1.5 T in Udine.
Patients with the following criteria were excluded from the study: diagnostic images blurred or some of required sequences missing.
As for surgery, all the patients underwent the following procedure: the surgical technique was carried out with an intraoperative protocol that involved the use of contrast enhancement ultrasounds (CEUS) and the fluorescence of 5-aminolevulinic acid (5-ALA). Neuro-navigation system was used in all cases, while the intra-operative neurophysiological monitoring was performed in all cases of proximity of the tumor to the cortico-spinal tract. Awake surgery was selected for patients that presented HGG in the dominant hemisphere, close to the inferior front-occipital fasciculus (IFOF) or the superior longitudinal fasciculus (SLF). In a subgroup of selected patient (young age, tumor not close to ventricular system) Carmustine Wafers (CWs) were implanted after surgical tumor removal and intra-operative pathological confirmation of HGG. CWs were not utilized when tumor removal required the creation of a large opening of ventricle and/or the basal cistern.
All methods were performed in accordance with the national guidelines and regulations and in accordance with the Declaration of Helsinki.
Image acquisition
All examinations were performed using a 1.5 T MR scanner (Siemens Aera in Udine and GE—Optima mr450 and Philips-Ingenia in Rome) with an eight-channel head coil. All study protocols included axial T2-weighted TSE/FSE images with a slice thickness between 4 and 5 mm and post-contrast volumetric T1-weighted images MPRAGE/FSPGR/WATS with a slice thickness between 1 and 1.2 mm. Imaging parameters are described in Table 1.
Image pre-processing and radiomic features extraction
Presurgical MRI performed on 1.5T scanners in FPG and SMM of HGG were analyzed by three neuroradiologists who assessed image quality, excluding patients with images degraded by artifacts or who did not present axial 3D T1 weighted post contrast and axial 2D T2 weighted. Manual segmentation of the tumoral areas was performed by one Neuroradiologist with the software “3D Slicer image computing platform” with ROIs drawn separately on T1 and T2W images as follows: on axial 3D T1 weighted contrast images post-contrast, the ROI on the “enhancing” component of the tumor was delineated, while on axial 2D T2 ROIs were outlined on the solid component of the tumor and the infiltrative one, excluding the frankly edematous areas (with higher signal in T2) (Figure 1). Before any analysis, the program anonymizes any DICOM.
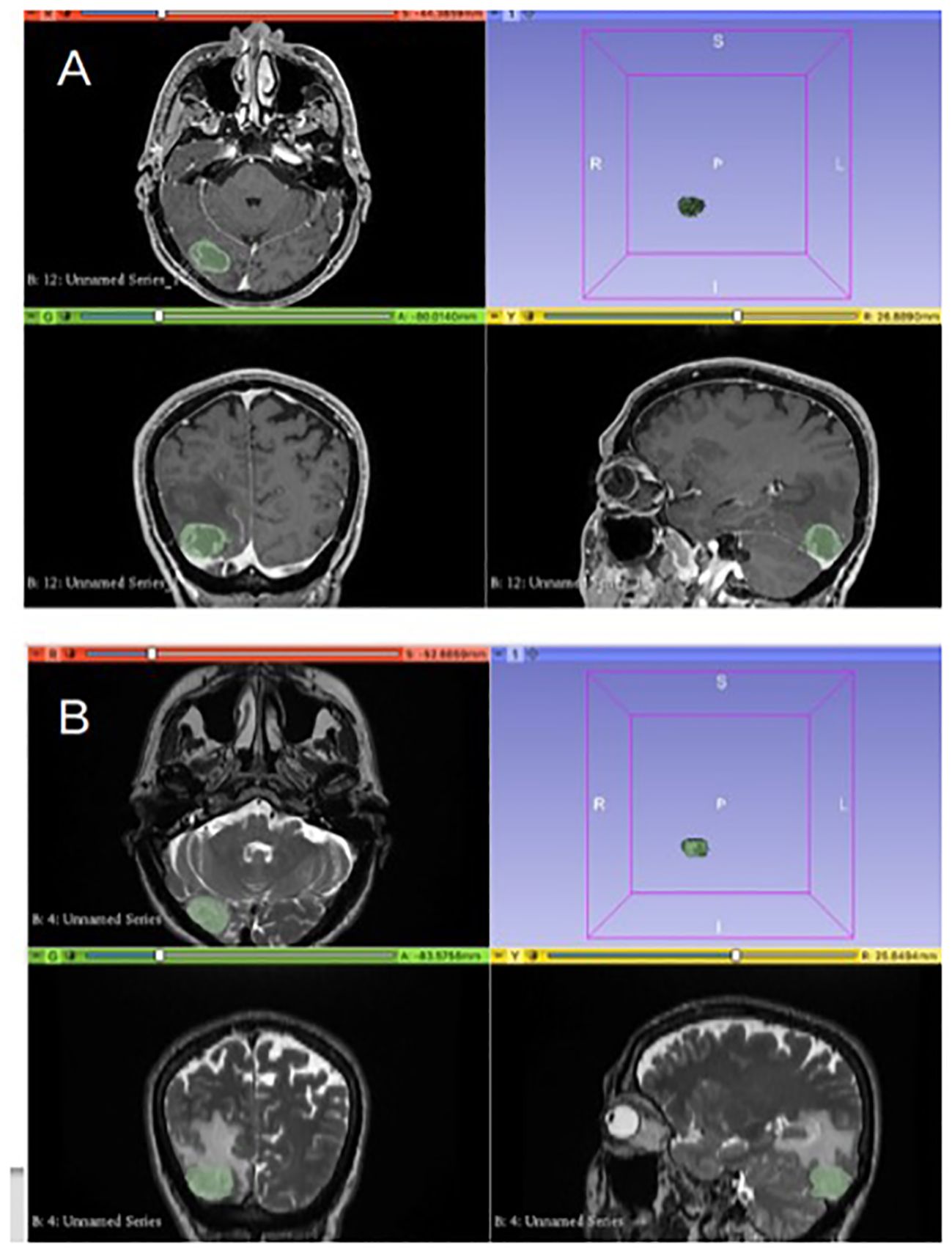
Figure 1. Segmentation of the tumoral areas: in image (A), delineation of the ROI on 3D T1 W post contrast images on the “enhancing” component of the tumor. In image (B), the ROI on axial 2D T2w images is delineated for the solid and infiltrative components of the tumor.
Image pre-processing and radiomic analyses were performed using Python 3.7.
Image pre-processing was performed via N4 bias field correction to correct low frequency intensity non uniformity and subject-specific Z-score normalization to normalize MR intensity scales and allow inter-patients comparison.
For each patient, a total of 100 radiomic features (26) were extracted from the specific ROI defined in each pre-processed MR sequence. Features were extracted in their 3D version using PyRadiomics (27).
Extracted features belonged to three families: morphology-based (14), first order (18) and second order (68). For the second order features we used a bin width discretization strategy with a bin width value of 25. Morphology-based features capture geometric characteristics of the ROI. First order features describe statistical properties of the grey level histogram, offering global metrics for the distribution of the grey levels within the ROI. Second order features provide localized measures of grey level distribution within the ROI.
Radiomic features selection and modeling
The training set included the patients enrolled in Rome, while the test set included the patients enrolled in Udine. We decided to follow this approach to develop a prediction model using data from one hospital and test its generalizability on data from a different hospital, for external testing to pursue a TRIPOD 3 approach (36).
Each radiomic feature was normalized using the z-score in the training set, and the z-score parameters of the training set were used to normalize the features in the test set.
After features extraction, feature selection and radiomics modeling was performed on the training set only. The reproducibility of radiomic features was evaluated with respect to the MRI scanner manufacturer using the t-test. Features that resulted not reproducible (p-value < 0.05) were excluded from the following analysis.
Further feature selection methods were applied to prevent overfitting, reduce linear correlations among variables and obtain a minimal and stable set of radiomic features. These methods included the univariate analysis with the Wilcoxon-Mann-Whitney statistical test (WMW, significance level of 0.05) and the computation of the Pearson correlation coefficient (PCC) with the exclusion of features that were highly correlated with any other feature (PCC > 0.9).
Different machine learning models, namely regularized logistic regression, random forest, XGBoost and Support Vector Machine (SVM) were trained on the selected radiomic features extracted from axial 3D T1w and axial 2D T2w.
The predictive models aimed to address a binary classification problem for the prediction of the 6-months progression free survival: class 0 denoted patients without 6-months TR, class 1 denoted patients with 6-months TR.
For each machine learning model, hyper-parameters tuning was performed with a grid search strategy using 3-fold cross-validation (28).
The developed models were externally evaluated on the testing set in terms of discriminative ability and predictive performance. In particular, the area under the curve (AUC) of the receiver operating characteristics (ROC) curve was calculated, and the model performance metrics based on the classification matrix were computed at the best cut-off threshold, identified by maximizing the Youden Index calculated on the training set. The metrics investigated were accurancy, sensitvity, specificity, positive and negative predictive values (PPV and NPV). 95% confidence intervals (CI) of AUC and classification metrics were computed according to bootstrap (29) and Jeffreys (30) methods, respectively.
Results
Patients population
When performing radiomic analysis and modeling, the initial dataset consisting in 273 patients affected by HGG of first diagnosis and undergone respective surgery followed by Stupp protocol, was shrunk to 248 patients on the basis of the availability of the 6-month TR outcome.
Data were split into train (approx. 75%) and test (approx. 25%) sets resulting in 188 patients from Rome and 60 patients from Udine, respectively.
Demographic and clinicopathological characteristics of the included patients are reported in Table 2.
In the training set 109 patients were classified as class 0 and 79 patients as class 1. In the test set, 44 patients belonged to class 0 and 16 to class 1 (Figure 2).
Radiomic features selection
Features selection was applied to features extracted from T1w and T2w sequences.
As for T1w sequence, the t-test evaluating features stability with respect to the manufacturer shrank the number of features from 100 to 58, further reduced by the WMW test and PCC which led to four stable relevant features, reported in Table 3 with their corresponding WMW test p-values.
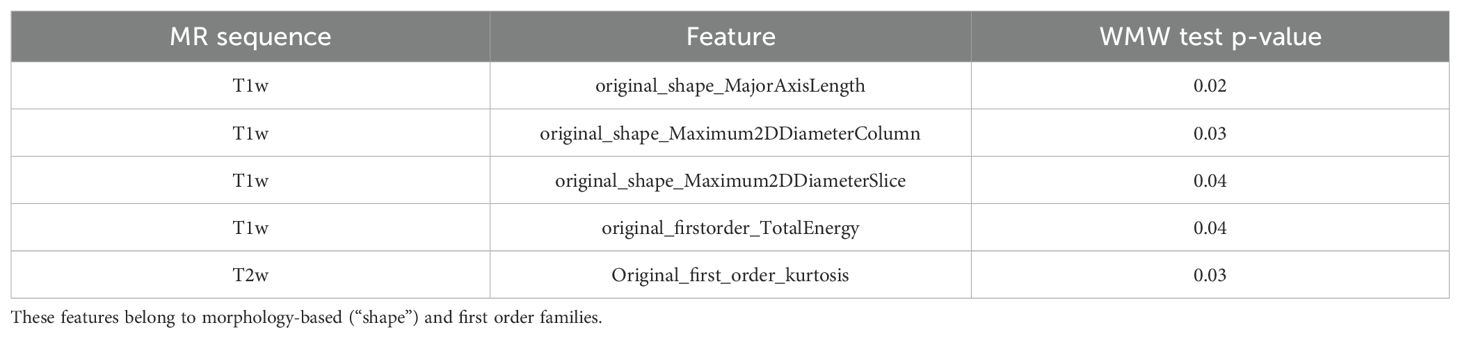
Table 3. Relevant features resulting from features selection performed on T1w and T2w sequences and corresponding p-values and used for radiomics modeling.
As for T2w sequence, 85 features out of 100 showed stability with respect to the manufacturer but only one of them was significant at univariate analysis, as reported in Table 3.
Thus, Table 3 included all the features used for the radiomics modeling.
Boxplots of the selected features showing stability with respect to the outcome are shown in Figure 3, with corresponding p-values resulting from the WMW test.
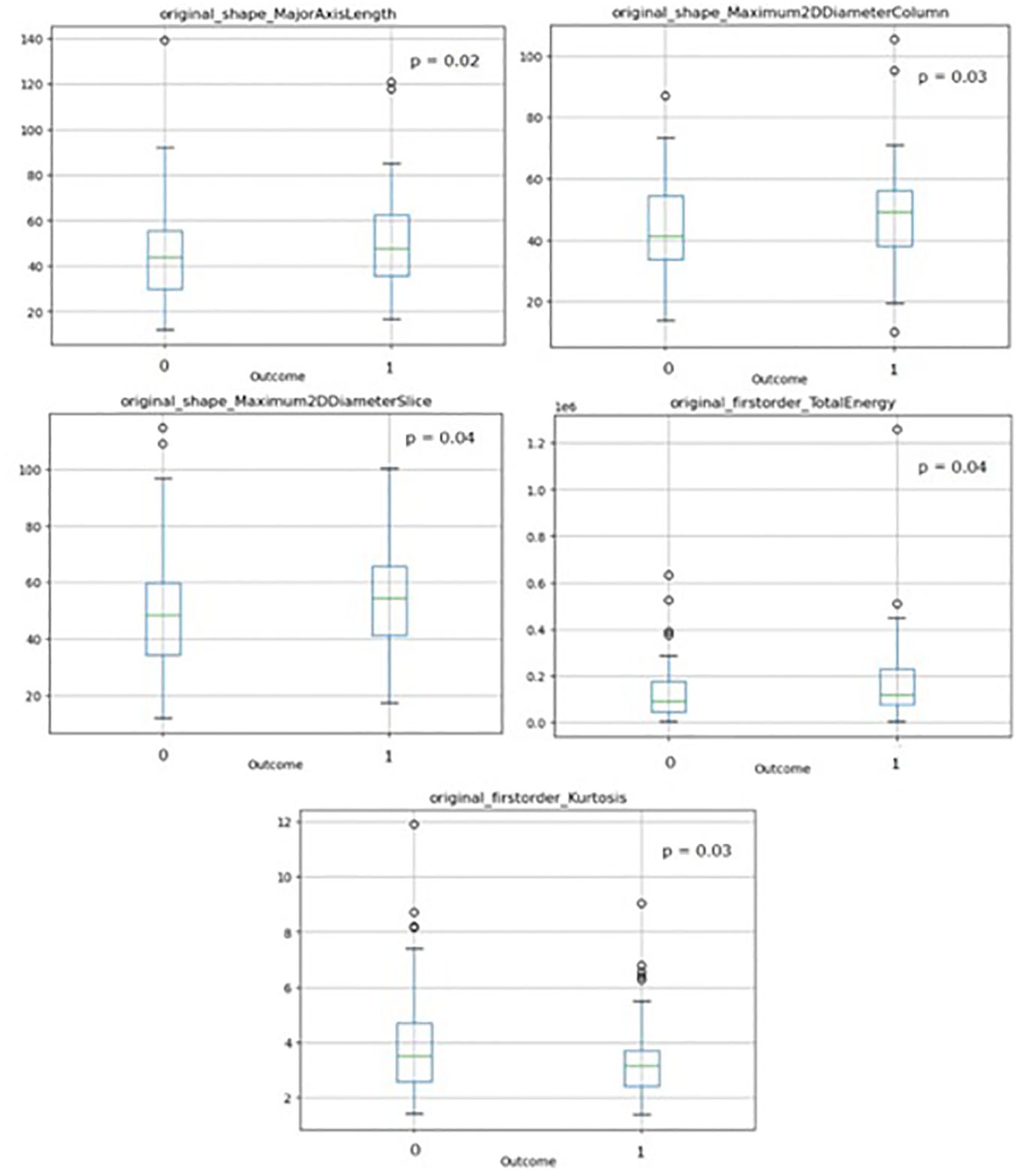
Figure 3. Boxplots of the selected radiomics features used for radiomics modeling showing stability with respect to the outcome with corresponding p-values resulting from the WMW test. (‘1’=patients with 6-months TR, ‘0’=patients without 6-months TR).
Modeling
A combined T1w&T2w (T1wT2w) modeling method was implemented, consisting in grouping the T1wT2w relevant features in a unique input dataset (see Table 3).
The cross-correlation matrix of the T1wT2w significant features is reported in Figure 4.
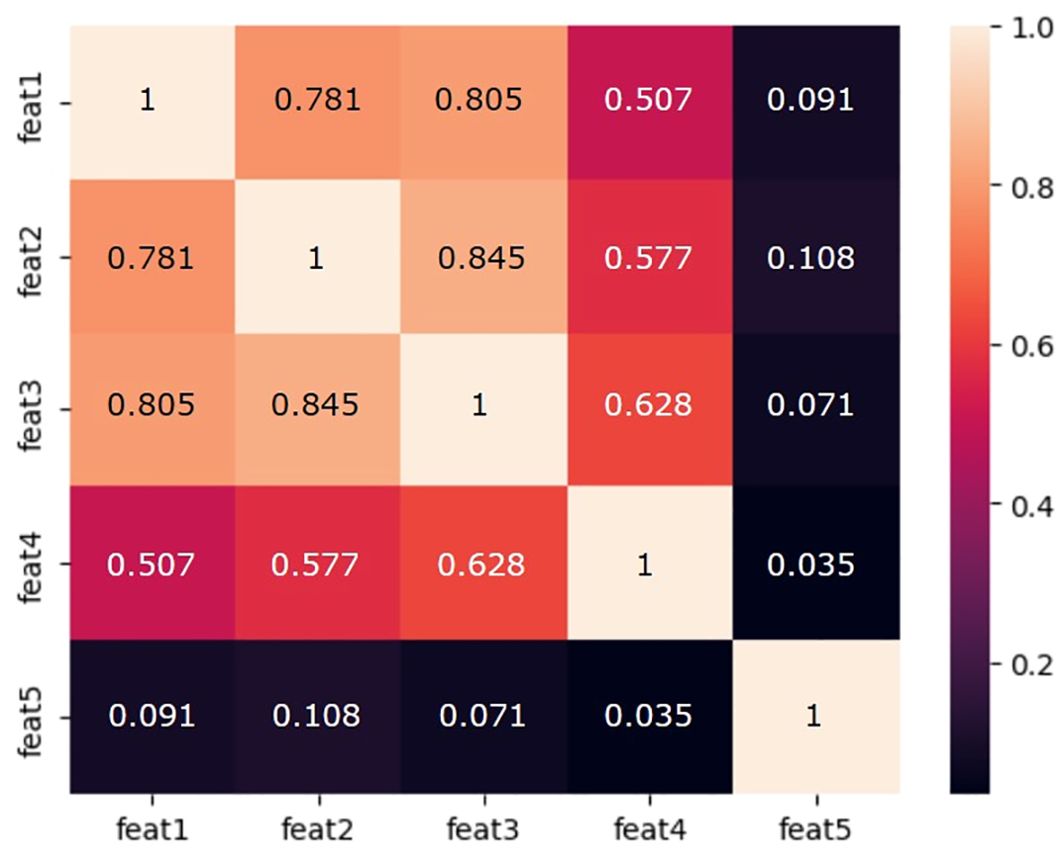
Figure 4. Cross-correlation matrix of the T1wT2w significant features used for radiomics modeling. feat1: original_shape_MajorAxisLength; feat2: original_shape_Maximum2DDiameterColumn; feat3: original_shape_Maximum2DDiameterSlice; feat4: original_firstorder_TotalEnergy; feat5: Original_first_order_kurtosis.
Table 4 reports the discriminative and predictive performance metrics for all the implemented ML models trained on T1w and T2w for both training and validation sets.
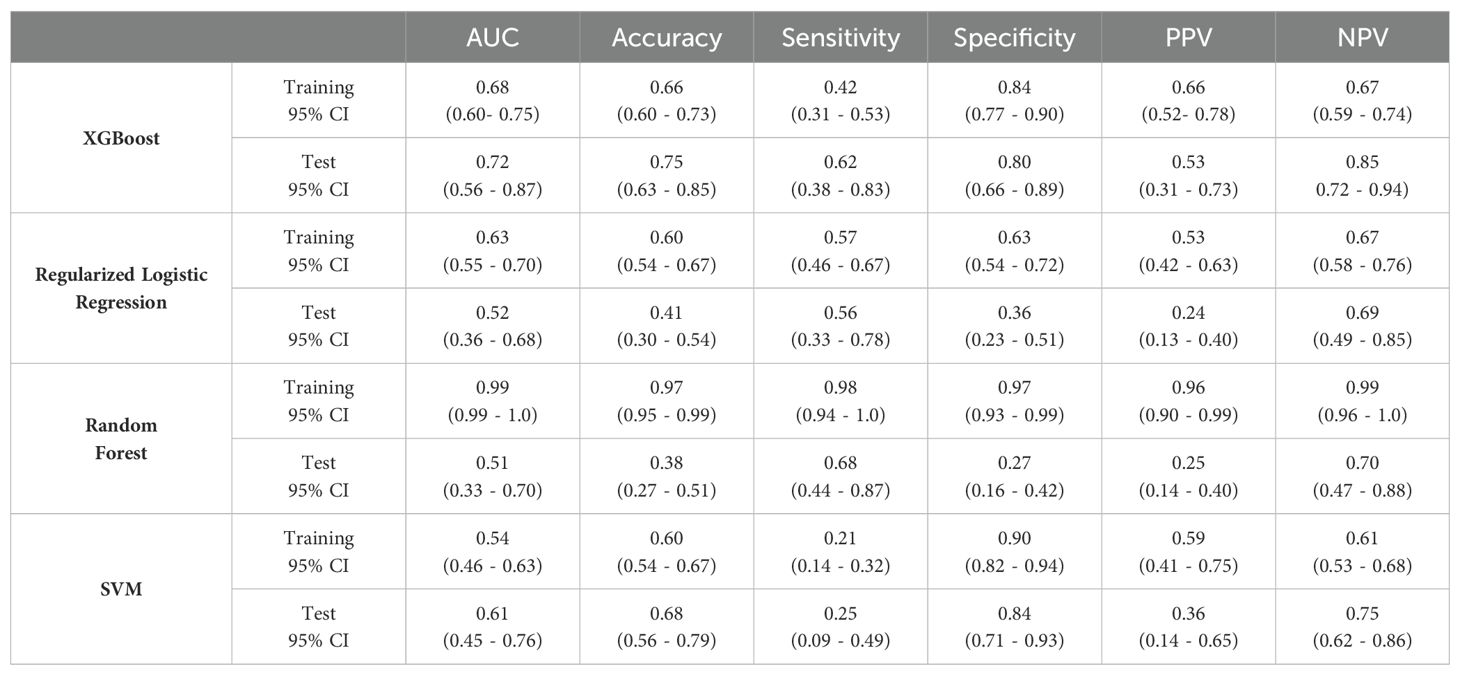
Table 4. Predictive performance metrics for ML models trained on T1w and T2w for training and validation sets.
Based on the metrics evaluated on the test set, the best model resulted to be the XGBoost, with a test set AUC of 0.72 and 95% CI of 0.56 - 0.87, and an accuracy of 0.75 with a 95% CI of 0.63 - 0.85. This model obtained high values for the specificity equal to 0.80 (95% CI: 0.66 - 0.89), and NPV equal to 0.85 (95% CI: 0.72 – 0.94).
The other models showed the problem of model over-fitting to the training data (i.e. Random Forest) or poor model performance for the training and test data (i.e. regularized logistic regression and SVM).
Discussion
Machine learning approach
Today MRI has a primary role in diagnosis, planning and monitoring of HGG patients: clinicians typically use brain MRI to evaluate radiological HGG features such as size, location, edema and enhancement characteristics. MRI features are today not sufficient to predict the risk of recurrence in HGG (31, 32):for this reason, there is a huge need to assess additional imaging biomarkers via computational methods (33, 34).
In the last years, a radiology-based approach focusing on prognosis prediction has gained an important burst fostered by the fast development of advanced computational tools able to manage a significant amount of MRI and clinical data.
Radiomics has recently emerged as a powerful data-driven approach that can offer insights into clinically relevant questions related to diagnosis, prediction, prognosis, as well as treatment response assessment (35).
In this investigation radiomic analysis and modeling were performed with the aim to select the most significant and robust MRI features able to predict which patients affected by HGG at first diagnosis would develop progression within 6 months or later. Prediction performance resulting from training was also externally tested following a TRIPOD 3 study approach, according to the original TRIPOD guidelines (36). Clustering was not accounted for in this study limiting model predictive performance and generalizability (48, 49). Future work may be conducted to evaluate the presence of potential cluster effects.
The 6-months TR time-point was chosen as cut-off value to discriminate patient with early TR from those with later TR for two main clinical reasons: it matches the standard timing of post-operative radiological assessment after conventional postsurgical treatments and it makes homogenous the study populations before the non-standardized rescue therapies at tumor recurrence.
Thus, detecting patients with low risk of TR after 6 months from surgery encourage neurosurgeons to extent tumor resection with the aim of exploiting the most modern intraoperative tools and strategies that allow a maximal safe resection.
We found that, among all features’ families, morphological features show most of the predictive power and that smaller features values decrease the probability to observe 6-month tumor recurrence in patients.
The best model resulted to be the XGBoost (eXtreme Gradient Boosting), an optimized distributed gradient boosting library designed to be highly efficient, flexible and portable. It implements ML algorithms under the Gradient Boosting framework, providing distributed gradient-boosted decision tree (GBDT). Boosting is an ensemble learning method that combines a set of weak base estimators into a strong learner to minimize training errors. For these reasons, XGBoost is considered to be one of the leading ML libraries for regression, classification and ranking problems.
Modeling results are reported in Table 3: the developed XGBoost showed a high value of specificity, which reflects the model ability in identifying patients with lower risk to experience tumor recurrence within 6 months and that might undergo a more aggressive surgical resection.
High values of specificity were observed in training (84%) and test set (80%). Features selection applied to this study highlighted that morphological features hold most of the predictive power in discriminating patients with positive and negative outcome. Although it is hard to find a direct biological interpretation of these findings, it is worth mentioning that radiomic features are not relevant if considered only “per se”. It is actually important to relate them to the model context and take linear and non-linear interactions between variables into account. In this light, a possible explanation can be given assuming that changes in morphological features might reflect tissues structural alterations (e.g. shape, volume etc…) and be more related to tumor developments. On the other hand, it is also important to ensure that selected features describing the model are actually stable, non-redundant and independent from noise or other non-relevant variables.
Pre-operative estimation of HGG biological behavior could help clinicians in detecting cases that could benefit from a maximal safe resection (e.g. HGG patients with pre-operative estimated of low risk of early TR).
On the other hand, pre-operative estimation of high risk of early TR (especially in elderly patients) could drive the choice to biopsy rather than surgery (37).
In addition, for patients with higher risk of TR, multiple tissue samplings should be extracted in order to investigate ad hoc target therapies related to lesions’ high spatial heterogeneity (38).
Our results are aligned with the study performed by Li et al. (39) with AUC of 0.70 in the training set for the prediction of disease progression at 6 months that used radiomics features extracted from multiple MRI sequences (T2 and FLAIR).
Other studies using smaller sample sizes developed MRI-based radiomics models for the prediction of the progression-free survival in patients with glioblastoma obtaining similar results. Choi et al. (40) obtained an integrated time-dependent AUC of 0.62, while Bathla et al. (41) achieved a C-index up to 0.64.
This investigation presents several limitations. First of all, this was a retrospective study including only two centers: further studies with more heterogeneous datasets would be an interesting point for future development.
Radiomic features reproducibility can be strongly affected by image acquisition parameters and scan protocols, which can vary widely across and within institutions. We took this heterogeneity into account and in order to evaluate features robustness among different centers, we performed a t-test analysis with respect to MRI scanners manufacturers. This allowed to exclude unstable features. In this light, to minimize data variability, harmonization methods might be introduced, as proposed by several research groups which focused on different modified ComBat algorithms (42–46). Although being very powerful, radiomic analysis offers no insights toward biological interpretation of the achieved findings and this study also shows this limitation: many efforts have been made in these recent years to reintroduce biological meaning into radiomics. However, some recent studies also suggest that biological correlation with radiomic features is not mandatory (47).
In addition, considering the integration of features and clinical variables in a clinical-radiomic model could help improving models’ performance in predicting 6-months TR.
Lastly, we did not assess the overall survival rate for this cohort. Data regarding selection criteria adopted at TR to plan the salvage treatment were not available. Each patient underwent an individualized management at TR, so we have not developed standardized protocols for treatments at TR. With our sample size, we did not have the statistical power to tease out the survival rate for patients undergoing different treatments. A future multi-center prospective study of HGG recurrence will be necessary to properly assess survival rate according to the salvage treatment adopted at TR.
The high incidence of early TR should encourage efforts to better understand the role of early intensified bridging therapies for HGG between surgery and postsurgical treatments.
In conclusion, the methodology adopted in this investigation is extremely time-consuming and makes it unsuitable for clinical daily implementation. The next step in this field, beyond increasing accuracy and simplifying the workflow, will be the development of an open source, easily scalable and efficient artificial intelligence algorithm requiring simple or null external intervention from physicians.
Conclusions
A thorough and reliable ML-model based on combined T1w&T2w sequences to detect the lower risk of TR in newly diagnosed HGG was trained and validated on external cohort.
Our results confirm the potential role of pre-operative MRI analysis beyond the classical anatomical and morphological parameters. MRI radiomic analysis represents a powerful tool to predict early HGG recurrence, to plan personalized surgical treatment and to offer patients pre-operative counseling. In the future, a prospective multicenter study with a larger sample size is needed in order to validate our results, to optimize prediction models for clinical practice, and to overcome the intrinsic limitations of retrospective studies met so far.
Data availability statement
The original contributions presented in the study are included in the article/supplementary material. Further inquiries can be directed to the corresponding author.
Ethics statement
The studies involving humans were approved by Ethical Committee of Policlinico Universitario Agostino Gemelli IRCCS. The studies were conducted in accordance with the local legislation and institutional requirements. The participants provided their written informed consent to participate in this study.
Author contributions
FP: Writing – original draft, Writing – review & editing. TI: Writing – original draft, Writing – review & editing. RR: Writing – original draft, Writing – review & editing. DB: Writing – original draft, Writing – review & editing. FB: Writing – original draft, Writing – review & editing. EB: Writing – original draft, Writing – review & editing. LB: Writing – original draft, Writing – review & editing. SC: Writing – original draft, Writing – review & editing. CC: Writing – original draft, Writing – review & editing. DC: Writing – original draft, Writing – review & editing. CG: Writing – original draft, Writing – review & editing. GL: Writing – original draft, Writing – review & editing. CM: Writing – original draft, Writing – review & editing. EM: Writing – original draft, Writing – review & editing. AO: Writing – original draft, Writing – review & editing. MS: Writing – original draft, Writing – review & editing. HT: Writing – original draft, Writing – review & editing. GV: Writing – original draft, Writing – review & editing. SG: Writing – original draft, Writing – review & editing. GS: Writing – original draft, Writing – review & editing.
Funding
The author(s) declare that no financial support was received for the research, authorship, and/or publication of this article.
Conflict of interest
The authors declare that the research was conducted in the absence of any commercial or financial relationships that could be construed as a potential conflict of interest.
Publisher’s note
All claims expressed in this article are solely those of the authors and do not necessarily represent those of their affiliated organizations, or those of the publisher, the editors and the reviewers. Any product that may be evaluated in this article, or claim that may be made by its manufacturer, is not guaranteed or endorsed by the publisher.
References
1. Ius T, Pignotti F, Della Pepa GM, La Rocca G, Somma T, Isola M, et al. A novel comprehensive clinical stratification model to refine prognosis of glioblastoma patients undergoing surgical resection. Cancers (Basel). (2020) 12. doi: 10.3390/cancers12020386
2. Lemee JM, Clavreul A, Menei P. Intratumoral heterogeneity in glioblastoma: don't forget the peritumoral brain zone. Neuro Oncol. (2015) 17:1322–32. doi: 10.1093/neuonc/nov119
3. Ohgaki H. Epidemiology of brain tumors. In: Verma M, editor. Cancer Epidemiology. Humana Press, Totowa, NJ (2009). p. 323–42.
4. Weller M, van den Bent M, Preusser M, Le Rhun E, Tonn JC, Minniti G, et al. EANO guidelines on the diagnosis and treatment of diffuse gliomas of adulthood. Nat Rev Clin Oncol. (2021) 18:170–86. doi: 10.1038/s41571-020-00447-z
5. Karschnia P, Vogelbaum MA, van den Bent M, Cahill DP, Bello L, Narita Y, et al. Evidence-based recommendations on categories for extent of resection in diffuse glioma. Eur J Cancer. (2021) 149:23–33. doi: 10.1016/j.ejca.2021.03.002
6. Wang L, Liang B, Li YI, Liu X, Huang J, Li YM. What is the advance of extent of resection in glioblastoma surgical treatment-a systematic review. Chin neurosurgical J. (2019) 5:2. doi: 10.1186/s41016-018-0150-7
7. Delgado-Lopez PD, Corrales-Garcia EM. Survival in glioblastoma: a review on the impact of treatment modalities. Clin Trans Oncol. (2016) 18:1062–71. doi: 10.1007/s12094-016-1497-x
8. Lacroix M, Abi-Said D, Fourney DR, Gokaslan ZL, Shi W, DeMonte F, et al. A multivariate analysis of 416 patients with glioblastoma multiforme: prognosis, extent of resection, and survival. J Neurosurg. (2001) 95:190–8. doi: 10.3171/jns.2001.95.2.0190
9. Nikova AS, Vlotinou P, Karelis L, Karanikas M, Birbilis TA. Gross total resection with fluorescence could lead to improved overall survival rates: a systematic review and meta-analysis. Br J Neurosurg. (2022) 36:316–22. doi: 10.1080/02688697.2021.1950637
10. Diez Valle R, Tejada Solis S, Idoate Gastearena MA, Garcia de Eulate R, Dominguez Echavarri P, Aristu Mendiroz J. Surgery guided by 5-aminolevulinic fluorescence in glioblastoma: volumetric analysis of extent of resection in single-center experience. J Neurooncol. (2011) 102:105–13. doi: 10.1007/s11060-010-0296-4
11. Eatz TA, Eichberg DG, Lu VM, Di L, Komotar RJ, Ivan ME. Intraoperative 5-ALA fluorescence-guided resection of high-grade glioma leads to greater extent of resection with better outcomes: a systematic review. J Neurooncol. (2022) 156:233–56. doi: 10.1007/s11060-021-03901-9
12. Eljamel S. 5-ALA fluorescence image guided resection of glioblastoma multiforme: A meta-analysis of the literature. Int J Mol Sci. (2015) 16:10443–56. doi: 10.3390/ijms160510443
13. Gandhi S, Tayebi Meybodi A, Belykh E, Cavallo C, Zhao X, Syed MP, et al. Survival outcomes among patients with high-grade glioma treated with 5-aminolevulinic acid-guided surgery: A systematic review and meta-analysis. Front Oncol. (2019) 9:620. doi: 10.3389/fonc.2019.00620
14. La Rocca G, Della Pepa GM, Menna G, Altieri R, Ius T, Rapisarda A, et al. State of the art of fluorescence guided techniques in neurosurgery. J neurosurgical Sci. (2019) 63:619–24. doi: 10.23736/S0390-5616.19.04854-9
15. Mazurek M, Kulesza B, Stoma F, Osuchowski J, Mandziuk S, Rola R. Characteristics of fluorescent intraoperative dyes helpful in gross total resection of high-grade gliomas-A systematic review. Diagnostics. (2020) 10. doi: 10.3390/diagnostics10121100
16. Pichlmeier U, Bink A, Schackert G, Stummer W, Group ALAGS. Resection and survival in glioblastoma multiforme: an RTOG recursive partitioning analysis of ALA study patients. Neuro Oncol. (2008) 10:1025–34. doi: 10.1215/15228517-2008-052
17. Stummer W, Reulen HJ, Meinel T, Pichlmeier U, Schumacher W, Tonn JC, et al. Extent of resection and survival in glioblastoma multiforme: identification of and adjustment for bias. Neurosurgery. (2008) 62:564–576; discussion 564-576. doi: 10.1227/01.neu.0000317304.31579.17
18. Della Pepa GM, Ius T, Menna G, La Rocca G, Battistella C, Rapisarda A, et al. Dark corridors" in 5-ALA resection of high-grade gliomas: combining fluorescence-guided surgery and contrast-enhanced ultrasonography to better explore the surgical field. J neurosurgical Sci. (2019) 63:688–96. doi: 10.23736/S0390-5616.19.04862-8
19. La Rocca G, Sabatino G, Menna G, Altieri R, Ius T, Marchese E, et al. 5-aminolevulinic acid false positives in cerebral neuro-oncology: not all that is fluorescent is tumor. A case-based update and literature review. World Neurosurg. (2020) 137:187–93. doi: 10.1016/j.wneu.2020.01.238
20. Poon MTC, Sudlow CLM, Figueroa JD, Brennan PM. Longer-term (>/= 2 years) survival in patients with glioblastoma in population-based studies pre- and post-2005: a systematic review and meta-analysis. Sci Rep. (2020) 10:11622. doi: 10.1038/s41598-020-68011-4
21. Delgado-Lopez PD, Rinones-Mena E, Corrales-Garcia EM. Treatment-related changes in glioblastoma: a review on the controversies in response assessment criteria and the concepts of true progression, pseudoprogression, pseudoresponse and radionecrosis. Clin Trans Oncol. (2018) 20:939–53. doi: 10.1007/s12094-017-1816-x
22. Zhou Q, Ke X, Xue C, Li S, Huang X, Zhang B, et al. A nomogram for predicting early recurrence in patients with high-grade gliomas. World Neurosurg. (2022) 164:e619–28. doi: 10.1016/j.wneu.2022.05.039
23. Ius T, Sabatino G, Panciani PP, Fontanella MM, Ruda R, Castellano A, et al. Surgical management of Glioma Grade 4: technical update from the neuro-oncology section of the Italian Society of Neurosurgery (SINch(R)): a systematic review. J Neurooncol. (2023) 162:267–93. doi: 10.1007/s11060-023-04274-x
24. Lambin P, Rios-Velazquez E, Leijenaar R, Carvalho S, van Stiphout RG, Granton P, et al. Radiomics: extracting more information from medical images using advanced feature analysis. Eur J Cancer. (2012) 48:441–6. doi: 10.1016/j.ejca.2011.11.036
25. Louis DN, Perry A, Wesseling P, Brat DJ, Cree IA, Figarella-Branger D, et al. The 2021 WHO classification of tumors of the central nervous system: a summary. Neuro Oncol. (2021) 23:1231–51. doi: 10.1093/neuonc/noab106
26. Z A. EP-1677: multicentre initiative for standardisation of image biomarkers. Radiotherapy Oncol. (2017) 123:S914–915. doi: 10.3252/pso.eu.ESTRO36.2017
27. Available online at: https://www.radiomics.io/pyradiomics.html. (accessed September 15, 2022).
28. B D. Cross-validation. In: Encyclopedia of Bioinformatics and Computational Biology. Amsterdam. Netherlands: Elsevier Science (2019). p. 542–5.
29. DiCiccio TJE B. Bootstrap confidence intervals. Statist Sci. (1996) 11:189–228. doi: 10.1214/ss/1032280214
30. Brown LDC T, Dasgupta A. Interval estimation for a binomial proportion. Stat Sci. (2001) 16:101–33. doi: 10.1214/ss/1009213286
31. Barboriak DP, Zhang Z, Desai P, Snyder BS, Safriel Y, McKinstry RC, et al. Interreader variability of dynamic contrast-enhanced MRI of recurrent glioblastoma: the multicenter ACRIN 6677/RTOG 0625 study. Radiology. (2019) 290:467–76. doi: 10.1148/radiol.2019181296
32. Lundemann M, Munck Af Rosenschold P, Muhic A, et al. Feasibility of multi-parametric PET and MRI for prediction of tumour recurrence in patients with glioblastoma. Eur J Nucl Med Mol Imaging. (2019) 46:603–13. doi: 10.1007/s00259-018-4180-3
33. Kim JY, Park JE, Jo Y, Shim WH, Nam SJ, Kim JH, et al. Incorporating diffusion- and perfusion-weighted MRI into a radiomics model improves diagnostic performance for pseudoprogression in glioblastoma patients. Neuro Oncol. (2019) 21:404–14. doi: 10.1093/neuonc/noy133
34. Tien AC, Li J, Bao X, Derogatis A, Kim S, Mehta S, et al. A phase 0 trial of ribociclib in recurrent glioblastoma patients incorporating a tumor pharmacodynamic- and pharmacokinetic-guided expansion cohort. Clin Cancer Res. (2019) 25:5777–86. doi: 10.1158/1078-0432.CCR-19-0133
35. Beig N, Bera K, Tiwari P. Introduction to radiomics and radiogenomics in neuro-oncology: implications and challenges. Neuro-oncology Adv. (2020) 2:iv3–iv14. doi: 10.1093/noajnl/vdaa148
36. Collins GS, Reitsma JB, Altman DG, Moons KG. Transparent reporting of a multivariable prediction model for individual prognosis or diagnosis (TRIPOD): the TRIPOD statement. Bmj. (2015) 350:g7594. doi: 10.1161/CIRCULATIONAHA.114.014508
37. Ius T, Somma T, Altieri R, Angileri FF, Barbagallo GM, Cappabianca P, et al. Is age an additional factor in the treatment of elderly patients with glioblastoma? A new stratification model: an Italian Multicenter Study. Neurosurgical Focus. (2020) 49:E13. doi: 10.3171/2020.7.FOCUS20420
38. Menna G, Manini I, Cesselli D, Dai G, Duan L, Luo Y, et al. Immunoregulatory effects of glioma-associated stem cells on the glioblastoma peritumoral microenvironment: a differential PD-L1 expression from core to periphery? Neurosurgical Focus. (2022) 52:E4. doi: 10.3171/2021.11.FOCUS21589
39. Li Z, Chen L, Song Y, et al. Predictive value of magnetic resonance imaging radiomics-based machine learning for disease progression in patients with high-grade glioma. Quantitative Imaging Med Surg. (2023) 13:224–36. doi: 10.21037/qims-22-459
40. Choi Y, Nam Y, Jang J, Shin NY, Lee YS, Ahn KJ, et al. Radiomics may increase the prognostic value for survival in glioblastoma patients when combined with conventional clinical and genetic prognostic models. Eur Radiol. (2021) 31:2084–93. doi: 10.1007/s00330-020-07335-1
41. Bathla G, Soni N, Ward C, Pillenahalli Maheshwarappa R, Agarwal A, Priya S. Clinical and magnetic resonance imaging radiomics-based survival prediction in glioblastoma using multiparametric magnetic resonance imaging. J Comput assisted tomography. (2023) 47:919–23. doi: 10.1097/RCT.0000000000001493
42. Da-Ano R, Masson I, Lucia F, Dore M, Robin P, Alfieri J, et al. Performance comparison of modified ComBat for harmonization of radiomic features for multicenter studies. Sci Rep. (2020) 10:10248. doi: 10.1038/s41598-020-66110-w
43. Fortin JP, Cullen N, Sheline YI, Taylor WD, Aselcioglu I, Cook PA, et al. Harmonization of cortical thickness measurements across scanners and sites. Neuroimage. (2018) 167:104–20. doi: 10.1016/j.neuroimage.2017.11.024
44. Fortin JP, Parker D, Tunc B, Watanabe T, Elliott MA, Ruparel K, et al. Harmonization of multi-site diffusion tensor imaging data. Neuroimage. (2017) 161:149–70. doi: 10.1016/j.neuroimage.2017.08.047
45. Mahon RN, Ghita M, Hugo GD, Weiss E. ComBat harmonization for radiomic features in independent phantom and lung cancer patient computed tomography datasets. Phys Med Biol. (2020) 65:015010. doi: 10.1088/1361-6560/ab6177
46. Masson I, Da-Ano R, Lucia F, Dore M, Castelli J, Goislard de Monsabert C, et al. Statistical harmonization can improve the development of a multicenter CT-based radiomic model predictive of nonresponse to induction chemotherapy in laryngeal cancers. Med Phys. (2021) 48:4099–109. doi: 10.1002/mp.14948
47. Fournier L, Costaridou L, Bidaut L, Michoux N, Lecouvet FE, de Geus-Oei LF, et al. Incorporating radiomics into clinical trials: expert consensus endorsed by the European Society of Radiology on considerations for data-driven compared to biologically driven quantitative biomarkers. Eur Radiol. (2021) 31:6001–12. doi: 10.1007/s00330-020-07598-8
48. Collins GS, Moons KGM, Dhiman P, Riley RD, Beam AL, Van Calster B, et al. TRIPOD+AI statement: updated guidance for reporting clinical prediction models that use regression or machine learning methods. BMJ. (2024) 16:385. doi: 10.1136/bmj-2023-078378
Keywords: machine learning, recurrence, high grade glioma, radiomics, prognosis
Citation: Pignotti F, Ius T, Russo R, Bagatto D, Beghella Bartoli F, Boccia E, Boldrini L, Chiesa S, Ciardi C, Cusumano D, Giordano C, La Rocca G, Mazzarella C, Mazzucchi E, Olivi A, Skrap M, Tran HE, Varcasia G, Gaudino S and Sabatino G (2024) Development and validation of a MRI-radiomics-based machine learning approach in High Grade Glioma to detect early recurrence. Front. Oncol. 14:1449235. doi: 10.3389/fonc.2024.1449235
Received: 14 June 2024; Accepted: 16 October 2024;
Published: 14 November 2024.
Edited by:
Domenico Aquino, IRCCS Carlo Besta Neurological Institute Foundation, ItalyReviewed by:
Fulvia Palesi, University of Pavia, ItalyRiccardo Pascuzzo, IRCCS Carlo Besta Neurological Institute Foundation, Italy
Copyright © 2024 Pignotti, Ius, Russo, Bagatto, Beghella Bartoli, Boccia, Boldrini, Chiesa, Ciardi, Cusumano, Giordano, La Rocca, Mazzarella, Mazzucchi, Olivi, Skrap, Tran, Varcasia, Gaudino and Sabatino. This is an open-access article distributed under the terms of the Creative Commons Attribution License (CC BY). The use, distribution or reproduction in other forums is permitted, provided the original author(s) and the copyright owner(s) are credited and that the original publication in this journal is cited, in accordance with accepted academic practice. No use, distribution or reproduction is permitted which does not comply with these terms.
*Correspondence: Rosellina Russo, cm9zZWxsaW5hLnJ1c3NvQHBvbGljbGluaWNvZ2VtZWxsaS5pdA==
†These authors have contributed equally to this work
‡These authors share senior authorship