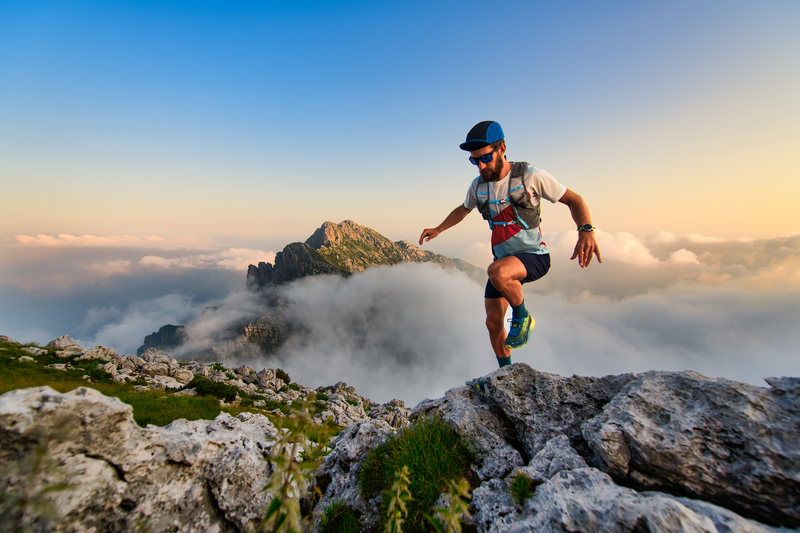
94% of researchers rate our articles as excellent or good
Learn more about the work of our research integrity team to safeguard the quality of each article we publish.
Find out more
ORIGINAL RESEARCH article
Front. Oncol. , 07 October 2024
Sec. Cancer Imaging and Image-directed Interventions
Volume 14 - 2024 | https://doi.org/10.3389/fonc.2024.1444115
This article is part of the Research Topic Towards Precision Oncology: Assessing the Role of Radiomics and Artificial Intelligence View all 12 articles
Background: The liver is a frequent location of metastatic disease in various malignant tumor entities. Computed tomography (CT) is the most frequently employed modality for initial diagnosis. However, liver metastases may only be delineated vaguely on CT. Calculating radiomics features in feature maps can unravel textures not visible to the human eye on a standard CT reconstruction (SCTR). This study aimed to investigate the comparative diagnostic accuracy of radiomics feature maps and SCTR for liver metastases.
Materials and methods: Forty-seven patients with hepatic metastatic colorectal cancer were retrospectively enrolled. Whole-liver maps of original radiomics features were generated. A representative feature was selected for each feature class based on the visualization of example lesions from five patients. These maps and the conventional CT image data were viewed and evaluated by four readers in terms of liver parenchyma, number of lesions, visual contrast of lesions and diagnostic confidence. T-tests and chi²-tests were performed with a significance cut off of p<0.05 to compare the feature maps with SCRT, and the data were visualized as boxplots.
Results: Regarding the number of lesions detected, SCTR showed superior performance compared to radiomics maps. However, the feature map for firstorder RootMeanSquared was ranked superior in terms of very high visual contrast in 57.4% of cases, compared to 41.0% in standard reconstructions (p < 0.001). All other radiomics maps ranked significantly lower in visual contrast when compared to SCTR. For diagnostic confidence, firstorder RootMeanSquared reached very high ratings in 47.9% of cases, compared to 62.8% for SCTR (p < 0.001). The conventional CT images showed superior results in all categories for the other features investigated.
Conclusion: The application of firstorder RootMeanSquared feature maps may help visualize faintly demarcated liver lesions by increasing visual contrast. However, reading of SCTR remains necessary for diagnostic confidence.
● Selected radiomics feature maps may increase the visual contrast of liver metastases compared with standard CT reconstructions, potentially improving detectability.
With more than 19 million new cases per year and up to 10 million deaths, cancer is among the most pressing global health issues worldwide (1–3). In the course of cancer progression, metastases are an essential driver of cancer-related mortality, especially in the case of liver metastases (4). Therefore, early and valid detection of malignant liver lesions is favorable for sufficient therapy of patients.
Due to the metastatic spread through the portal venous system, gastrointestinal (GI) cancers, particularly colon cancer, are prone to developing liver metastases. Although the superior diagnostic accuracy of MRI with hepatocyte-specific contrast agents is known (5), computed tomography is still employed in the majority of patients, partly due to cost considerations (6). CT imaging can be problematic for detecting metastatic liver lesions due to suboptimal soft tissue contrast (7). Especially in the early development of metastases or after a response to therapy, liver lesions may appear faint despite the administration of a contrast agent, resulting in a lower diagnostic performance when compared to MRI and PET/CT (8). Yet, CT remains the commonly performed modality for the primary staging of metastases. Therefore, additional techniques and image analytics would be desirable to improve the sensitivity and the visual delineation of liver metastases.
Radiomics has emerged as a promising tool in medical imaging, offering the potential to extract quantitative features from standard imaging modalities that are not visible to the human eye. These features can provide insights into the underlying tissue characteristics, potentially leading to improved diagnostic accuracy. Several studies have demonstrated the advantages of radiomics in various clinical applications. Radiomics offers a promising approach in oncologic imaging by providing quantitative data that can reveal tumor characteristics not visible on conventional imaging techniques like standard CT reconstructions (SCTR). For example, radiomics has been shown to be effective in predicting treatment response and patient outcomes across various cancer types. In gastrointestinal cancers, radiomics has demonstrated its utility in diagnosing and staging tumors, predicting prognosis, and assessing response to therapy, particularly in cases where traditional imaging methods may fall short. Studies have shown that radiomics can reflect tumor heterogeneity, which is crucial for tailoring treatment strategies and improving patient management (9). Furthermore, radiomics has been used to assess the aggressiveness of liver tumors and predict patient survival, offering a non-invasive complement to traditional biopsy methods, which often have limitations such as sampling bias and procedural risks (10). These applications illustrate the potential of radiomics to enhance diagnostic precision and provide more personalized care, thereby overcoming some of the limitations associated with SCTR.
In this regard, the increasing importance of radiomics analysis of CT image datasets with the ability to generate visualized parameter maps of previously segmented lesions may provide new opportunities to facilitate the diagnosis of difficult-to-define lesions in the liver and potentially improve detectability (11–13). Therefore, the study aimed to investigate the diagnostic confidence and visual distinguishability for metastatic colorectal cancer (mCRC) liver metastases on radiomics feature maps compared to SCTR images.
Forty-seven patients were retrospectively included in our data analysis based on the following inclusion criteria: the selected patients had known colorectal liver metastases confirmed in histopathology. Corresponding CT images were acquired as part of the clinical routine using iodine contrast agent (Imeron® 350, Bracco IMAGING Deutschland GmbH, Konstanz, Germany) on commercially available clinical CT scanners (SOMATOM Emotion, SOMATOM Go Up ®, SOMATOM Flash, Siemens Healthcare GmbH, Erlangen, Germany). Patient data are presented in Table 1. The Institutional Review Board (2020-861R) of the Ethik Kommission II at the University Medical Center Mannheim, Germany, approved the proposed retrospective study protocol and the study was conducted in accordance with the Declaration of Helsinki. Image data were acquired according to local standard operating procedures with the following settings: Slice thickness of 1.5 mm, axial reconstruction, 130 -kV tube voltage, tube current modulation enabled, B30s kernel, pitch of 1.0, FOV of 400 mm, matrix 512×512, and pixel size of 0.78 mm × 0.78 mm.
Manual whole-liver segmentation was performed using MITK workbench (MITK Workbench v2021.02, Deutsches Krebsforschungszentrum, DKFZ, Heidelberg, Germany). The results were saved as a compressed nifti (nii.gz) data. Using a custom Docker container (Docker Desktop; Version 4.3.1, Docker, Inc., Palo Alto, CA, USA) based on pyradiomics (14) (version 3.0.1) scripts, the radiomics feature maps for the set of original radiomics features were calculated. The feature extraction process was conducted using the default settings in pyradiomics and adheres to the guidelines set by the Image Biomarker Standardization Initiative (IBSI) (15). The extraction involved gray-level discretization with a fixed bin width of 32 bins, resampling the images to a voxel size of 1 mm³, and applying a mask dilation with a radius of 1 voxel. The radiomic features extracted encompass various feature families, including first-order (FO) statistics, shape-based (SH) features, gray level co-occurrence matrix (GLCM), gray level run length matrix (GLRLM), gray level size zone matrix (GLSZM), neighboring gray tone difference matrix (NGTDM), and gray level dependence matrix (GLDM). KernelRadius was set to its default value of 1, and the voxelBatch parameter was set to “None”. MaskedKernel setting was set to “True”.
Feature maps for all original radiomics features were reviewed in five example patients by two radiology residents with 4 years and 2 years of experience in oncologic imaging and quantitative image analysis as preparation for the next step of feature selection: one representative parameter from each of the different classes (FO, GLCM, GLDM, GLRLM, GLSZM, and NGTDM) was selected based on different factors. The selection of radiomics features for this study was guided by their ability to enhance diagnostic accuracy in detecting and characterizing liver metastases. Features were prioritized based on their capacity to improve visual contrast between liver parenchyma, metastatic lesions, and anatomical landmarks, which is crucial for accurate lesion identification. Additionally, features demonstrating low variability in healthy liver tissue were selected to reduce the risk of false positives. One representative feature from each radiomics feature class was selected to ensure a comprehensive evaluation of the diverse types of textural information provided by different feature families. This approach ensured that the selected features were both practically effective and clinically relevant. A structured multi-reader analysis was performed for these six parameter maps and SCTR. To avoid bias, the readers examined the different radiomics maps in a predefined order that was staggered for each reader and rotated per patient. The study protocol and workflow are summarized in Figure 1. The reading criteria are shown in Supplementary Table 1.
Figure 1. Study protocol. Whole liver segmentations were performed in five example CT datasets. Standard radiomics features were extracted and visualized parameter maps. Based on visual assessment by two readers, one feature of each feature class was selected. Subsequently, all parameter maps as well as the standard CT reconstructions of all patients were evaluated by four readers in a structured manner and evaluated according to the number of lesion, visual contrast, diagnostic confidence, and liver parenchyma homogeneity.
A master table was created for the evaluation of the selected parameter maps. Homogeneity of liver parenchyma, number of metastases, contrast with surrounding parenchyma, and diagnostic confidence was evaluated based on a dedicated scheme (see the Supplementary Material). In addition, the contrast of the parameter maps was compared with that of conventional CT images and evaluated. To minimize the influence of window settings on lesion detectability, standardized window settings were used for each radiomics map, and radiologists did not alter these settings during the evaluation. All relevant image data (six radiomics feature maps plus SCTR per patient) of the 47 selected patients were assessed by four radiology residents with more than 2 years of experience in oncologic CT imaging.
All data analyses were performed with R statistics in R Studio (version 4.1.0) (16). Plots were created with the package ggplot2 (17). Statistical analysis was performed on the pooled results from all radiologists. Each radiologist independently assessed the images, and their evaluations were then combined for the statistical analysis to provide an overall assessment of the performance of the radiomics feature maps versus the standard CT reconstructions. The differences in the reading results of the various radiomics maps and SCRT were tested for significance using Student’s t-test for paired distributions and the chi-squared test as well as Kruskal–Wallis H-test. A cutoff of p below 0.05 was applied for statistical significance. Distributions were visualized as boxplots.
The patients' collective characteristics have been published previously (18). Patient characteristics relevant to this analysis are summarized in Table 1.
Based on a structured rating of visual contrast for five patients by two radiology residents, the original radiomics features firstorder RootMeanSquared, GLCM sum entropy, GLDM non-uniformity, GLRLM non-uniformity, GLSZM variance, and NGTDM contrast were selected for further analysis (Figure 1).
Overall, the liver parenchyma was more heterogeneous in all selected radiomics maps than conventional CT imaging except for firstorder RootMeanSquared (85.6% more homogenous, overall p < 0.001). Significantly fewer lesions were detected in the radiomics maps GLCM sum entropy, GLDM non-uniformity, GLRLM non-uniformity, GLSZM variance, and NGTDM contrast (e.g., 0.39 lesions on average in NGTDM contrast) compared to the conventional CT images (p < 0.001; Figure 2). Also, a lower detection rate was found in the firstorder RootMeanSquared maps, with an average number of 7.55 lesions detected per patient compared to 8.84 lesions detected in the conventional CT images (p < 0.001) (see Table 2). The number of lesions detected individually by the four readers is displayed in Figures 3, 4. The p-value for the number of detected lesions between the individual features for each reader was <0.0001.
Figure 2. Example reading of one patient. The image section of an exemplary lesion is shown in the standard CT reconstruction as well as in all evaluated radiomics maps. In addition, the Average number of lesions is shown as well as the number of readers who rated the liver parenchyma as more heterogeneous than in the SCTR, the visual contrast as high or very high, and the diagnostic confidence as high or very high. Original firstorder RootMeanSquared shows a superior contrast for two liver lesions.
Figure 3. Number of detected lesions. Number of detected lesions by the four readers plotted by SCTR and radiomics features visualized as box plots.
Figure 4. Number of lesions summarized for all readers. Number of lesions summarized for all readers plotted by SCTR and radiomics features visualized as box plots.
For visual contrast, firstorder RootMeanSquared achieved a very high ranking in 108 reads (57.4%) compared to 77 reads (41.0%) for SCTR (p < 0.001). However, for diagnostic confidence, firstorder RootMeanSquared achieved a very high ranking in 90 reads (47.9%) compared to 118 reads (62.8%) for SCTR (p < 0.001). All other radiomics features showed a significantly lower visual contrast and diagnostic confidence (Table 2).
This study compared radiomics feature maps to conventional CT reconstructions in terms of liver parenchyma visualization, liver lesion detection rate, visual contrast and diagnostic confidence. The conventional CT images performed significantly better than the selected radiomics maps concerning liver parenchyma homogeneity, lesions detected, and diagnostic confidence. However, firstorder RootMeanSquared showed a superior rating for visual contrast.
As the detectability of liver lesions strongly depends on the visual contrast of lesions in computed tomography, the increased contrast may help in lesions delineated faintly, especially in the case of inexperienced readers, by enhancing their sensitivity. In contrast, the comparative advantage of SCTR, in terms of diagnostic confidence, may be expected, as the standard CT reconstruction contains more visual information on the lesions’ properties for the reader. Since the radiomics maps differ significantly from the SCTR in terms of visual impression, this may be a possible explanation for the lower diagnostic certainty compared to the SCTR, with which radiologists have been familiar in routine practice for years. While some radiomics feature maps exhibited reduced resolution compared to SCTR images, which may have limited the detection of smaller liver lesions, others demonstrated an improved contrast between lesions and liver parenchyma, highlighting a trade-off between image resolution and contrast enhancement. Therefore, the application of firstorder RootMeanSquared feature maps would be recommended as an additional read, comparable to computer-aided diagnosis (CAD) systems, than as a replacement for SCTRs. The feature itself is defined as the square root of the mean of all squared intensity values. It is an indicator of the magnitude of the image values (19).
Radiomics feature maps have been applied for several use cases in oncologic imaging, especially in response assessment to medical treatment: A study by Correa et al. (20) investigated the association of glioblastoma lesion habitat with response to treatment. Several radiomics features could be identified that can be used to differentiate a low or high risk of treatment failure.
A similar approach has been successfully applied by Beig et al. (21) to the distinction between granuloma and adenocarcinoma: Using deep learning mechanisms of selected intra- and peri nodular radiomics features, differentiation between adenocarcinomas and granulomas is possible with an area under the curve (AUC) value of 0.8.
Penzias et al. (22) have shown that radiomics analysis of prostate MRI (T2w), especially by Gabor texture features, is feasible for predicting Gleason score in patients with prostate cancer with an AUC of 0.69.
In the work of Algohary et al. (23), T2w and apparent diffusion coefficient (ADC) sequences of prostate MRIs were radiomically evaluated, and selected radiomics features were presented as visualized maps. In the group of negative MRIs with positive biopsy (the MRIs had been assessed in accordance with the PI-RADS standard), carcinoma areas could be retrospectively identified in the radiomics maps, which could not be delineated in the mere T2w or ADC images.
However, the application of radiomics maps to improve the visual contrast of lesions has not been studied comprehensively. While this study demonstrates the potential of radiomics feature maps, particularly the firstorder RootMeanSquared map, to enhance the visual contrast of liver metastases on CT images, it also opens several avenues for future research. These findings could drive the development of more advanced radiomics-based diagnostic tools, which may complement SCTR. The application of such selected parameter maps could support radiologists in the routine interpretation of oncological imaging datasets, similar to how CAD systems assist in the detection of pulmonary nodules. Further research should focus on refining and validating these parameters across diverse clinical settings to ensure their robustness and reproducibility. Additionally, integrating these tools into clinical practice could lead to more personalized and accurate diagnostics, ultimately improving patient outcomes. Addressing these aspects will be crucial in realizing the full potential of radiomics in routine clinical use.
The implementation of radiomics feature maps in routine clinical practice presents significant challenges, both technical and organizational. The technical complexity of radiomics analysis requires specialized expertise in image processing and data analysis, necessitating extensive training for radiologists and technicians. Furthermore, integrating radiomics into existing clinical workflows poses an additional hurdle, as current systems and processes must be adapted to effectively incorporate these new technologies. Addressing these challenges requires interdisciplinary collaboration and continuous development of technology and training programs to facilitate the adoption of radiomics in clinical practice. Despite the promising potential of radiomics feature maps, their immediate applicability in everyday diagnostic settings is hindered by several factors. The lack of widespread validation and standardization of radiomics features poses a significant challenge, as it limits the reproducibility and generalizability of results across different clinical settings. Moreover, potential regulatory and cost barriers further complicate the integration of radiomics into routine practice.
Furthermore, while this study demonstrates the potential of radiomics maps, it also reveals the poor performance of certain radiomics features compared to standard CT reconstructions. This discrepancy may be attributed to several factors, including the inherent variability in feature extraction, the influence of image acquisition parameters, and the complexity of interpreting radiomics features in heterogeneous tissues such as liver parenchyma. Identifying and addressing these factors are crucial for enhancing the performance and clinical utility of radiomics. Future research should focus on optimizing feature selection and standardization processes, as well as improving the robustness of radiomics features to better match or exceed the diagnostic confidence provided by conventional imaging techniques.
Regarding the analysis of different radiomics maps, further limitations arise particularly in cases where the metastases are immediately subcapsular to the margin of the liver. These cannot be delineated partly for technical shortcomings in the segmentations and the basic calculation of the radiomics features due to the density differences between liver parenchyma and surrounding soft tissue. This problem could be addressed by methodologically optimized segmentation and radiomics map generation techniques. Since this study is a methodologically novel approach, optimized segmentation and visualization techniques must be further explored in future research. Additionally, the selection of representative features based on visual contrast in a subset of five patients may introduce bias, potentially limiting the generalizability of the findings.
In summary, conventional CT images are superior to the selected radiomics feature maps GLCM sum entropy, GLDM non-uniformity, GLRLM non-uniformity, GLSZM variance, and NGTDM contrast in terms of homogeneity of liver parenchyma, detectability of liver lesions, visual contrast as well as diagnostic confidence. Only firstorder RootMeanSquared maps showed a comparable lesion detection rate. Furthermore, visual contrast was significantly more often rated as very high in the latter than in the SCTR. In the case of liver lesions that are only faintly delineated on conventional CT imaging, evaluating firstorder RootMeanSquared maps may offer an additive advantage in terms of detectability.
The raw data supporting the conclusions of this article will be made available by the authors, without undue reservation.
The studies involving humans were approved by Ethik Kommission II at the University Medical Center Mannheim, Germany (2020-861R). The studies were conducted in accordance with the local legislation and institutional requirements. The ethics committee/institutional review board waived the requirement of written informed consent for participation from the participants or the participants' legal guardians/next of kin due to retrospective analysis of anonymized image data
AH: Conceptualization, Data curation, Formal analysis, Investigation, Methodology, Project administration, Supervision, Validation, Visualization, Writing – original draft, Writing – review & editing. MK: Data curation, Formal analysis, Methodology, Writing – review & editing. FT: Data curation, Formal analysis, Methodology, Writing – review & editing. HT: Writing – review & editing. DN: Writing – review & editing. NR: Writing – review & editing. SS: Methodology, Supervision, Writing – review & editing. MF: Conceptualization, Data curation, Formal analysis, Investigation, Methodology, Resources, Supervision, Validation, Visualization, Writing – original draft, Writing – review & editing.
The author(s) declare that no financial support was received for the research, authorship, and/or publication of this article.
All contributions are mentioned above in detail.
The authors declare that the research was conducted in the absence of any commercial or financial relationships that could be construed as a potential conflict of interest.
All claims expressed in this article are solely those of the authors and do not necessarily represent those of their affiliated organizations, or those of the publisher, the editors and the reviewers. Any product that may be evaluated in this article, or claim that may be made by its manufacturer, is not guaranteed or endorsed by the publisher.
The Supplementary Material for this article can be found online at: https://www.frontiersin.org/articles/10.3389/fonc.2024.1444115/full#supplementary-material
Supplementary Figure 1 | Exemplary high-resolution representation of several feature maps.
Supplementary Figure 2 | High-resolution images of the liver maps of a liver lesion that can be faintly delineated in the SCTR.
CAD, computer-aided diagnosis; CT, computed tomography; FO, first-order; GI, gastrointestinal; GLCM, gray level co-occurrence matrix; GLDM, gray level dependence matrix; GLRLM, gray level run length matrix; GLSZM, gray level size zone matrix; mCRC, metastatic colorectal cancer; MRI, magnetic resonance imaging; NGTDM, neighboring gray tone difference matrix; SCTR, standard CT reconstructions; SH, shape-based.
1. Sung H, Ferlay J, Siegel RL, Laversanne M, Soerjomataram I, Jemal A, et al. Global cancer statistics 2020: GLOBOCAN estimates of incidence and mortality worldwide for 36 cancers in 185 countries. CA A Cancer J Clin. (2021) 71:209–49. doi: 10.3322/caac.21660
2. Labianca R, Beretta GD, Kildani B, Milesi L, Merlin F, Mosconi S. Colon cancer. Crit Rev Oncology/Hematology. (2010) 74:106–33. doi: 10.1016/j.critrevonc.2010.01.010
3. Ghadirian P, Maisonneuve P, Perret C, Lacroix A, Boyle P. Epidemiology of sociodemographic characteristics, lifestyle, medical history, and colon cancer: A case-control study among French Canadians in Montreal. Cancer Detect Prev. (1998) 22:396–404. doi: 10.1046/j.1525-1500.1998.00058.x
4. Engstrand J, Nilsson H, Strömberg C, Jonas E, Freedman J. Colorectal cancer liver metastases – a population-based study on incidence, management and survival. BMC Cancer. (2018) 18:78. doi: 10.1186/s12885-017-3925-x
5. Lee DH, Lee JM. Whole-body PET/MRI for colorectal cancer staging: Is it the way forward?: Whole-Body PET/MRI for Colorectal Cancer. J Magn Reson Imaging. (2017) 45:21–35. doi: 10.1002/jmri.25337
6. Sica GT, Ji H, Ros PR. Computed tomography and magnetic resonance imaging of hepatic metastases. Clinics Liver Dis. (2002) 6:165–79. doi: 10.1016/S1089-3261(03)00071-0
7. Zocholl G, Kuhn F-P, Augustin N, Thelen M. Diagnostische Aussagekraft von Sonographie und Computertomographie bei Lebermetastasen. Fortschr Röntgenstr. (1988) 148:8–14. doi: 10.1055/s-2008-1048137
8. Choi SH, Kim SY, Park SH, Kim KW, Lee JY, Lee SS, et al. Diagnostic performance of CT, gadoxetate disodium-enhanced MRI, and PET/CT for the diagnosis of colorectal liver metastasis: Systematic review and meta-analysis. Magnetic Resonance Imaging. (2018) 47:1237–50. doi: 10.1002/jmri.25852
9. Caruso D, Polici M, Zerunian M, Pucciarelli F, Guido G, Polidori T, et al. Radiomics in oncology, part 1: technical principles and gastrointestinal application in CT and MRI. Cancers. (2021) 13:2522. doi: 10.3390/cancers13112522
10. Brunese MC, Fantozzi MR, Fusco R, De Muzio F, Gabelloni M, Danti G, et al. Update on the applications of radiomics in diagnosis, staging, and recurrence of intrahepatic cholangiocarcinoma. Diagnostics. (2023) 13:1488. doi: 10.3390/diagnostics13081488
11. Gillies RJ, Kinahan PE, Hricak H. Radiomics: images are more than pictures, they are data. Radiology. (2016) 278:563–77. doi: 10.1148/radiol.2015151169
12. Yip SSF, Aerts HJWL. Applications and limitations of radiomics. Phys Med Biol. (2016) 61:R150–66. doi: 10.1088/0031-9155/61/13/R150
13. Fiz F, Viganò L, Gennaro N, Costa G, La Bella L, Boichuk A. Radiomics of liver metastases: A systematic review. Cancers. (2020) 12:2881. doi: 10.3390/cancers12102881
14. Van Griethuysen JJM, Fedorov A, Parmar C, Hosny A, Aucoin N, Narayan V, et al. Computational radiomics system to decode the radiographic phenotype. Cancer Res. (2017) 77:e104–7. doi: 10.1158/0008-5472.CAN-17-0339
15. Zwanenburg A, Vallières M, Abdalah MA, Aerts HJWL, Andrearczyk V, Apte A, et al. The image biomarker standardization initiative: standardized quantitative radiomics for high-throughput image-based phenotyping. Radiology. (2020) 295:328–38. doi: 10.1148/radiol.2020191145
16. R Studio Team. R Studio, Version 1.3.1093. Boston, MA, USA: R Studio Team (2020). Available at: http://www.rstudio.com.
17. Wickham H. ggplot2: Elegant Graphics for Data Analysis. Springer-Verlag New York (2016). Available at: https://ggplot2.tidyverse.org.
18. Tharmaseelan H, Hertel A, Tollens F, Rink J, Woźnicki P, Haselmann V, et al. Identification of CT imaging phenotypes of colorectal liver metastases from radiomics signatures-towards assessment of interlesional tumor heterogeneity. Cancers (Basel). (2022) 14(7):1646. doi: 10.3390/cancers14071646
19. Available online at: https://pyradiomics.readthedocs.io/en/latest/features.html (Accessed 23.08.2022).
20. Verma R, Correa R, Hill V, Beig N, Mahammedi A, Madabhushi A, et al. Radiomics of the lesion habitat on pre-treatment MRI predicts response to chemo-radiation therapy in Glioblastoma. In: Hahn HK, Mori K, editors. Medical Imaging 2019: Computer-Aided Diagnosis. SPIE, San Diego, United States (2019). p. 10.
21. Beig N, Khorrami M, Alilou M, Prasanna P, Braman N, Orooji M, et al. Perinodular and intranodular radiomic features on lung CT images distinguish adenocarcinomas from granulomas. Radiology. (2019) 290:783–92. doi: 10.1148/radiol.2018180910
22. Penzias G, Singanamalli A, Elliott R, Gollamudi J, Shih N, Feldman M, et al. Identifying the morphologic basis for radiomic features in distinguishing different Gleason grades of prostate cancer on MRI: Preliminary findings. PLoS One. (2018) 13:e0200730. doi: 10.1371/journal.pone.0200730
Keywords: radiomics, radiomics maps, lesion detectability, liver metastases, colorectal cancer
Citation: Hertel A, Kuru M, Tollens F, Tharmaseelan H, Nörenberg D, Rathmann N, Schoenberg SO and Froelich MF (2024) Comparison of diagnostic accuracy of radiomics parameter maps and standard reconstruction for the detection of liver lesions in computed tomography. Front. Oncol. 14:1444115. doi: 10.3389/fonc.2024.1444115
Received: 05 June 2024; Accepted: 29 August 2024;
Published: 07 October 2024.
Edited by:
Salvatore Claudio Fanni, University of Pisa, ItalyReviewed by:
Emina Talakic, Medical University of Graz, AustriaCopyright © 2024 Hertel, Kuru, Tollens, Tharmaseelan, Nörenberg, Rathmann, Schoenberg and Froelich. This is an open-access article distributed under the terms of the Creative Commons Attribution License (CC BY). The use, distribution or reproduction in other forums is permitted, provided the original author(s) and the copyright owner(s) are credited and that the original publication in this journal is cited, in accordance with accepted academic practice. No use, distribution or reproduction is permitted which does not comply with these terms.
*Correspondence: Alexander Hertel, YWxleGFuZGVyLmhlcnRlbEB1bW0uZGU=
Disclaimer: All claims expressed in this article are solely those of the authors and do not necessarily represent those of their affiliated organizations, or those of the publisher, the editors and the reviewers. Any product that may be evaluated in this article or claim that may be made by its manufacturer is not guaranteed or endorsed by the publisher.
Research integrity at Frontiers
Learn more about the work of our research integrity team to safeguard the quality of each article we publish.