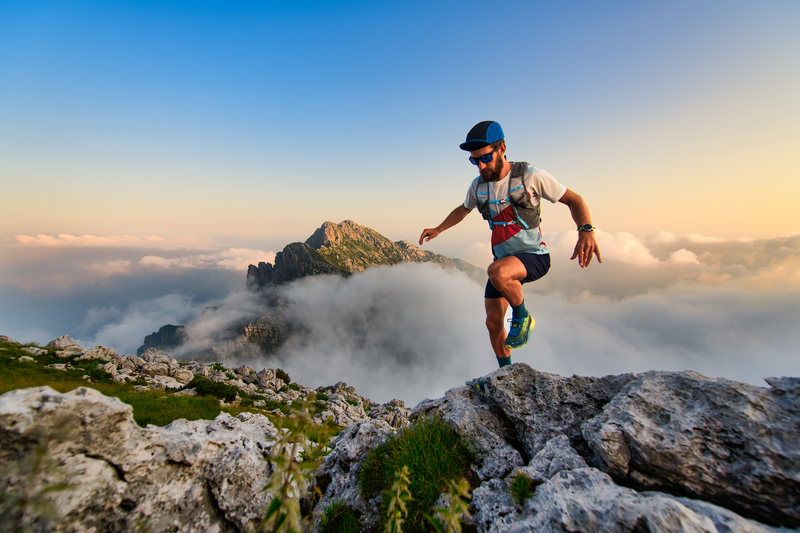
95% of researchers rate our articles as excellent or good
Learn more about the work of our research integrity team to safeguard the quality of each article we publish.
Find out more
CASE REPORT article
Front. Oncol. , 31 October 2024
Sec. Pediatric Oncology
Volume 14 - 2024 | https://doi.org/10.3389/fonc.2024.1422811
This article is part of the Research Topic Global Approaches to Molecular Diagnostics for Pediatric Cancer View all 8 articles
Next-generation sequencing technologies have not only defined a breakthrough in medical genetics, but also been able to enter routine clinical practice to determine individual genetic susceptibilities. Modern technological developments are routinely introduced to genetic analysis overtaking the established approaches, potentially raising a number of challenges. To what extent is the advantage of new methodologies in synthetic metrics, such as precision and recall, more important than stability and reproducibility? Could differences in the technical protocol for calling variants be crucial to the diagnosis and, by extension, the patient’s treatment strategy? A regulatory review process may delay the incorporation of potentially beneficial technologies, resulting in missed opportunities to make the right medical decisions. On the other hand, a blind adoption of new technologies based solely on synthetic metrics of precision and recall can lead to incorrect conclusions and adverse outcomes for the specific patient. Here, we use the example of a patient with a WHO-diagnosed desmoplastic/nodular SHH-medulloblastoma to explore how the choice of DNA variant search protocol affects the genetic diagnostics outcome.
Medulloblastoma (MB) is one of the most widespread malignant brain tumors in children (WHO Class IV). It accounts for approximately 62.4% of embryonal tumors, making it the most common type within this category (1). The annual incidence of MB in children is estimated to be approximately 5 cases per 1 million children (1).
Histomorphologically, MB is characterized as an embryonal tumor originating in the cerebellum and is believed to arise from distinct neural stem or progenitor cells during early developmental stages (2). MB encompasses four molecularly distinct subgroups: wingless-type (WNT), Sonic Hedgehog (SHH), Group 3, and Group 4 (3). SHH-type MB comprises approximately 30% of all MB cases and primarily affects patients below 3 years of age and those above 16 years, with a lower incidence observed in the 3- to 16-year age group (4). Survival outcomes for SHH-type MB are highly influenced by specific subtypes within the SHH subgroup (5).
The SHH signaling pathway plays a pivotal role in tissue and organ development, performing essential functions in morphogenesis, mitogenesis, and cellular differentiation processes (6). It is obvious that genetic variants in crucial genes of the SHH pathway, such as Protein patched homolog 1 (PTCH1), 2 (PTCH2), SUFU Negative Regulator Of Hedgehog Signaling (SUFU), and Smoothened, frizzled class receptor (SMO), as well as in related transcription factors such as Family Zinc Finger 1 (GLI1) and 2 (GLI2), directly influence MB pathogenesis. The vast majority of MB cases are explained by somatic mutations and epigenetic modifications. However, for 54% of cases, the molecular genetics profile of MB could not be established (7). The critical impact of germline and somatic mutations in TP53 on the prognosis of survival in SHH-MB has likewise long been known (8). Recently putative causal germline mutations have been reported in MB patients, for example, germline mutations in PTEN, ELP1, and PHOX2B genes (9–11). Similar effects of germline variants have been observed in pediatric MB cases, e.g., in MSH2, RAD50, and NBN genes (12).
Here, using the genetic data from a pediatric patient with WHO-diagnosed desmoplastic/nodular SHH-MB, we highlight how the choice of variant calling platform affects the results of molecular diagnosis. Resolution of the issues highlighted in this study could potentially reduce the number of undiagnosed MB.
The infant patient was born with multiple intellectual and developmental disabilities, including cleft of the hard and soft palate of the upper lip and alveolar process on the right, as well as anomalies of the eyeball development: microphthalmos, microcornea, and anterior colobomatous cyst of the orbit.
The health and treatment track included several surgeries and monitoring of the recovery dynamics by MRI (Figure 1). Prior to the onset of oncological symptoms, the patient underwent cleft lip and palate repair in the summer of 2020. The first MRI in the fall of 2020 showed dysgenesis of the corpus callosum, dilation of the subarachnoid liquor spaces, ventricular system, and right orbital abnormality—cystic eye, presumably congenital.
The second MRI dated in the summer of 2021 was diagnostic for MB (see the Diagnostics section). Microsurgical removal of the cerebellar vermis and IV ventricle tumor was performed later that summer using intraoperative navigation. Findings of the histological and immunohistochemical analysis were consistent with SHH-MB. Regarding family history, no inherited syndromes associated with MB were identified.
Based on the post-surgery third MRI data of the brain and spinal cord, the patient disease stage was classified as R0M0, according to the histological results following the HIT-SKK 2017 protocol [version 4.0, 2017; Therapieprotokoll für Säuglinge und Kleinkinder (SKK) mit Hirntumoren (Brain Tumor Radiotherapy for Infants and Toddlers with Medulloblastoma)] (13, 14). The patient was recommended for polychemotherapy for MB in the 0- to 5-year-old age group, with classification M0 under the SKK scheme (13). According to the protocol, the chemotherapy plan included three consequential cycles of regular SKK and two cycles of modified SKK. After the first SKK, the MRI analysis showed positive dynamics: reduction of signs of pathologic contrast agent accumulation in the periphery of the postoperative cavity. Taking into account the data of the MRI, continuation of chemotherapy (second block of SKK) was prescribed. No further signs of cancer progression were identified. At the time of the last contact with the patient in April 2024, there was no evidence of recurrence, suggesting remission for at least 32 months.
Baseline MRI showed a left temporal lobe arachnoid cyst and a mass in the vermis of the cerebellum on the right side (Figures 2A, B).
Figure 2. Clinical and histological characteristics. (A, B) MRI screens of the brain (green arrows indicate a cerebellar vermis tumor). (A) Axial plane; (B) sagittal plane. (C) Hematoxylin–eosin staining of the MB sample. (D–F) Immunohistochemical (IHC) staining of tumor sample: (D) beta-catenin; (E) filamin A; (F) GAB1. The scales were manually added to the figures.
Hematoxylin–eosin staining analysis was used for histomorphological classification of MB. The analysis revealed a large number of cells at the stage of mitotic division, indicating rapid tumor growth (Figure 2C). The tumor was assigned a Grade 4 status. Based on characteristic features such as nodular architecture, desmoplasia, areas of reduced cell density, and the presence of dense collagenous stroma surrounding the nodules, the tumor was classified as desmoplastic/nodular MB (Supplementary Figure S1).
A panel of three assays, beta-catenin, filamin A, and GAB1 staining, originally proposed by David Ellison et al., was used to immunohistochemically (IHC) confirm the diagnosis of MB and identify its genetically defined subtype (2, 15). Ki-67 was assessed as a marker of proliferation activity. An IHC study showed cytoplasmic expression of beta-catenin (Figure 2D), filamin A expression (Figure 2E), and GAB1 expression (Figure 2F). The level of Ki-67 in the tumor tissue registers at 25%–30% (Supplementary Figure S2).
Based on the findings from the histomorphological and histogenetic classification, the tumor was diagnosed as desmoplastic/nodular SHH-MB, Grade 4.
Parents of the patient provided informed consent for molecular genetic testing. Approval for the study was granted by the institutional ethics committee under Protocol #3502-22 dated 21 February 2020.
The genomic DNA from peripheral blood and tumor samples were prepared for sequencing utilizing Kapa Biosystems (Roche) kits. To target the coding regions of the genome, the TruSeq Exome Capture kit (Illumina) was employed. The Fragment Analyzer ensured the quality of the resultant libraries, while qPCR assessed the adequacy of DNA quantity. Following quality assurance and quantification of DNA, the library pool was sequenced across two lanes of the Illumina NovaSeq 6000.
The raw sequencing data, presented as FASTQ files, were acquired through the utilization of bcl2fastq v2.20 Conversion Software (Illumina). Subsequently, both germline and somatic variant calling were executed following the best practices recommended by GATK and Mutect2, respectively (16, 17). Germline variants were independently found using two different variant calling tools—HaplotypeCaller (16) and DeepVariant (18), each run with default settings.
DeepVariant and HaplotypeCaller are advanced tools used to identify germline genetic variants from sequencing data. DeepVariant, developed by Google, uses deep learning techniques to achieve high accuracy in variant detection by converting raw sequence data into a list of genetic variants. HaplotypeCaller, part of the Broad Institute’s GATK toolkit, was considered the gold standard in the field. It builds possible haplotypes in a region and assigns probabilities to them to determine the most probable genetic variants.
When using “default settings”, these tools apply preset parameters optimized for common use cases. It is expected that the majority of routine research will use the default settings.
Initially, we sought to analyze somatic and germline DNA sequencing since the patient was presenting multiple severe phenotypes.
Initial analysis of somatic mutations indicated the presence of nine variants that passed the technical quality filtering: (1) six with non-coding annotation and (2) two long InDels that lacked convincing support upon examining the IGV view of the sequencing reads. The remaining missense variant in SATL1 did not affect any conserved regions of the gene (MPC < 1). Given that SATL1 is not an oncogene, this variant was dismissed as a diagnostic candidate.
Then, two relevant germline variants were identified—NC_000018.9:g.22805738T>C (NM_015461:p.Asp715Gly, rs1390185292) in the ZNF521 and NC_000007.13:g.15197085856T>A (NM_170606:p.Thr316Ser, rs10454320) in the KMT2C. Both variants were assessed as “variants of uncertain significance” according to the joint recommendations of Clinical Genome Resource (ClinGen), Cancer Genomics Consortium (CGC), and Variant Interpretation for Cancer Consortium (VICC) (19).
For further variant interpretation, we investigated whether ZNF521 has any prior evidence of being relevant to MB or other phenotypes. We used both pre-assembled lists of genes for screening (20) and the pathway analysis tools, such as WikiPathways (21) or Genepanel.iobio (22). Although ZNF521 is a diagnostic gene for MB, it was absent from all lists used (23). ZNF521 was previously shown to modulate the SHH pathway through binding to GLI1 and GLI2 (24). The rs1390185292 missense variant affects a highly conserved region of ZNF521 (the Missense badness, PolyPhen-2, and Constraint summary metric score; MPC > 2.5) (25), potentially carrying serious implications for normal protein function. Further testing of rs1390185292 for de novo status in the patient revealed this SNP in one of the parents by Sanger sequencing data, preventing this variant from being considered as molecularly diagnostic, but leaving the possibility for incomplete penetrance effects.
According to the WGS study on relatively large MB cohorts, KMT2C is characterized by mutations of varying severity (26). The rs10454320 affects the highly conserved ePHD1_KMT2C domain “Extended PHD finger 1 found in histone-lysine N-methyltransferase 2C”, which is consistent with high levels of deleteriousness metrics (MPC>2.5) (25). This explains the high statistical difference in survival prognosis (27).
The variant rs10454320 was filtered out by GATK’s best practice protocol for germline variants using HaplotypeCaller but was successfully identified by DeepVariant with default parameters. rs10454320 was filtered out by GATK’s best practice protocol for germline variants based on HaplotypeCaller (16), but was successfully identified by DeepVariant with default parameters (18). The variant rs10454320 was a false positive according to Sanger sequencing (Figure 3). The observation implies that simply comparing variant calling tools using established benchmarks is inadequate to promptly revise the current standards of clinical practice.
Figure 3. Sanger sequencing chromatogram. The arrow indicates the reference allele for SNP rs10454320.
Clinical practice recommends tumor sequencing testing, but a fraction of patients affected by MB remain undiagnosed. Thus, the study of hereditary predisposition may provide additional insight into the contribution of rare/de novo variants to disease progression. However, germline analysis requires careful standardization of detection techniques specifically in the context of MB.
The false-positive rs10454320 in the KMT2C gene appears to be the result of a software problem rather than a technical error in the Illumina sequencer. Specifically, this problem was observed in DeepVariant but not in HaplotypeCaller, even though the data are the same.
The superiority of DeepVariant over other existing variant calling protocols based on synthetic parameters like precision and recall is known from the literature (28, 29). In some studies, it was shown that DeepVariant surpasses HaplotypeCaller in Mendelian error rate, Ti/Tv ratio, and clinical variant detection, although it faces challenges with quality score correlation and integration into downstream applications. The concordance rate between the two pipelines was 88.73%, indicating a high level of agreement in variant calling results. According to various articles, in summary, the difference between the protocols is attributed to the higher number of true-positive variants identified by DeepVariant (30). However, it has not yet been shown that choosing one or the other tool can dramatically determine the severity of the diagnosis and that sometimes this choice is not in favor of DeepVariant.
Adherence to standards of clinical practice is important not only for patient safety but also for maintaining the integrity and credibility of medical research. Without adherence to established standards, it becomes difficult to compare and replicate research findings, hindering the progress of medical science. Before integrating new tools into clinical practice, they must undergo a rigorous evaluation process to ensure their safety, efficacy, and practicality. This evaluation should consider established benchmarks and guidelines, as well as input from various constituents, including patients, clinicians, researchers, and regulatory authorities.
The datasets presented in this study can be found in online repositories. The names of the repository/repositories and accession number(s) can be found below: https://www.ncbi.nlm.nih.gov/snp/, rs1390185292, https://www.ncbi.nlm.nih.gov/snp/, rs10454320.
The studies involving humans were approved by the institutional ethics committee under Protocol #3502-22 dated 21.02.2020. The studies were conducted in accordance with the local legislation and institutional requirements. Written informed consent for participation in this study was provided by the participants’ legal guardians/next of kin. Written informed consent was obtained from the individual(s), and minor(s)’ legal guardian/next of kin, for the publication of any potentially identifiable images or data included in this article.
RS: Conceptualization, Data curation, Formal analysis, Investigation, Methodology, Resources, Software, Validation, Visualization, Writing – original draft, Writing – review & editing. SS: Data curation, Formal analysis, Investigation, Methodology, Validation, Writing – review & editing. MK: Data curation, Formal analysis, Methodology, Validation, Writing – review & editing. AS: Data curation, Formal analysis, Methodology, Validation, Writing – review & editing. MA: Conceptualization, Investigation, Methodology, Supervision, Validation, Writing – review & editing. AL: Funding acquisition, Project administration, Resources, Supervision, Writing – review & editing. YD: Conceptualization, Funding acquisition, Investigation, Project administration, Resources, Supervision, Validation, Writing – review & editing.
The author(s) declare financial support was received for the research, authorship, and/or publication of this article. RS, YD, SS, MK, AS, DM, and AL received support from the Ministry of Science and Higher Education of the Russian Federation under Agreement #075-15-2022-301. MA received support from the Nationwide Foundation Pediatric Innovation Fund.
The authors declare that the research was conducted in the absence of any commercial or financial relationships that could be construed as a potential conflict of interest.
All claims expressed in this article are solely those of the authors and do not necessarily represent those of their affiliated organizations, or those of the publisher, the editors and the reviewers. Any product that may be evaluated in this article, or claim that may be made by its manufacturer, is not guaranteed or endorsed by the publisher.
The Supplementary Material for this article can be found online at: https://www.frontiersin.org/articles/10.3389/fonc.2024.1422811/full#supplementary-material
1. Ostrom QT, Gittleman H, Truitt G, Boscia A, Kruchko C, Barnholtz-Sloan JS. CBTRUS statistical report: primary brain and other central nervous system tumors diagnosed in the United States in 2011-2015. Neuro Oncol. (2018) 20:iv1–iv86. doi: 10.1093/neuonc/noy131
2. Orr BA. Pathology, diagnostics, and classification of medulloblastoma. Brain Pathol. (2020) 30:664–78. doi: 10.1111/bpa.12837
3. Taylor MD, Northcott PA, Korshunov A, Remke M, Cho Y-J, Clifford SC, et al. Molecular subgroups of medulloblastoma: the current consensus. Acta Neuropathol. (2012) 123:465–72. doi: 10.1007/s00401-011-0922-z
4. Millard NE, De Braganca KC. Medulloblastoma. J Child Neurol. (2016) 31:1341–53. doi: 10.1177/0883073815600866
5. Dasgupta A, Gupta T, Sridhar E, Shirsat N, Krishnatry R, Goda JS, et al. Pediatric patients with SHH medulloblastoma fail differently as compared with adults: possible implications for treatment modifications. J Pediatr Hematol Oncol. (2019) 41:e499–505. doi: 10.1097/MPH.0000000000001484
6. Skoda AM, Simovic D, Karin V, Kardum V, Vranic S, Serman L. The role of the Hedgehog signaling pathway in cancer: A comprehensive review. Bosn J Basic Med Sci. (2018) 18:8–20. doi: 10.17305/bjbms.2018.2756
7. Hoffmann IL, Cardinalli IA, Yunes JA, Seidinger AL, Pereira RM. Clinical, demographic, anatomopathological, and molecular findings in patients with medulloblastoma treated in a single health facility. Rev Paul Pediatr. (2020) 39:e2019298. doi: 10.1590/1984-0462/2021/39/2019298
8. Carta R, Del Baldo G, Miele E, Po A, Besharat ZM, Nazio F, et al. Cancer predisposition syndromes and medulloblastoma in the molecular era. Front Oncol. (2020) 10:566822. doi: 10.3389/fonc.2020.566822
9. Tolonen J-P, Hekkala A, Kuismin O, Tuominen H, Suo-Palosaari M, Tynninen O, et al. Medulloblastoma, macrocephaly, and a pathogenic germline PTEN variant: Cause or coincidence? Mol Genet Genomic Med. (2020) 8:e1302. doi: 10.1002/mgg3.1302
10. Waszak SM, Robinson GW, Gudenas BL, Smith KS, Forget A, Kojic M, et al. Germline Elongator mutations in Sonic Hedgehog medulloblastoma. Nature. (2020) 580:396–401. doi: 10.1038/s41586-020-2164-5
11. Ke C, Shi X, Chen AM, Li C, Jiang B, Huang K, et al. Novel PHOX2B germline mutation in childhood medulloblastoma: a case report. Hered Cancer Clin Pract. (2021) 19:12. doi: 10.1186/s13053-021-00170-5
12. Trubicka J, Żemojtel T, Hecht J, Falana K, Piekutowska-Abramczuk D, Płoski R, et al. The germline variants in DNA repair genes in pediatric medulloblastoma: a challenge for current therapeutic strategies. BMC Cancer. (2017) 17:239. doi: 10.1186/s12885-017-3211-y
13. Merlin M-S, Schmitt E, Mezloy-Destracque M, Dufour C, Riffaud L, Puiseux C, et al. Neurocognitive and radiological follow-up of children under 5 years of age treated for medulloblastoma according to the HIT-SKK protocol. J Neurooncol. (2023) 163:195–205. doi: 10.1007/s11060-023-04328-0
14. Juhnke B, Mynarek M, von Hoff K. HIT-MED Guidance for patients with newly diagnosed Medulloblastoma, Ependymoma, CNS Embryonal Tumour, and Pineoblastoma. Hamburg-Eppendorf, Germany: Universitätsklinikum Hamburg-Eppendorf (2017).
15. Ellison DW, Dalton J, Kocak M, Nicholson SL, Fraga C, Neale G, et al. Medulloblastoma: clinicopathological correlates of SHH, WNT, and non-SHH/WNT molecular subgroups. Acta Neuropathol. (2011) 121:381–96. doi: 10.1007/s00401-011-0800-8
16. Van der Auwera GA, Carneiro MO, Hartl C, Poplin R, Del Angel G, Levy-Moonshine A, et al. From FastQ data to high confidence variant calls: the Genome Analysis Toolkit best practices pipeline. Curr Protoc Bioinf. (2013) 43(1110):11.10.1-11.10.3. doi: 10.1002/0471250953.bi1110s430
17. Benjamin DI, Sato T, Cibulskis K, Getz G, Stewart C, Lichtenstein L. Calling somatic SNVs and indels with mutect2. BioRxiv. (2019). doi: 10.1101/861054
18. Poplin R, Chang P-C, Alexander D, Schwartz S, Colthurst T, Ku A, et al. A universal SNP and small-indel variant caller using deep neural networks. Nat Biotechnol. (2018) 36:983–7. doi: 10.1038/nbt.4235
19. Horak P, Griffith M, Danos AM, Pitel BA, Madhavan S, Liu X, et al. Standards for the classification of pathogenicity of somatic variants in cancer (oncogenicity): Joint recommendations of Clinical Genome Resource (ClinGen), Cancer Genomics Consortium (CGC), and Variant Interpretation for Cancer Consortium (VICC). Genet Med. (2022) 24:986–98. doi: 10.1016/j.gim.2022.01.001
20. Skitchenko R, Dinikina Y, Smirnov S, Krapivin M, Smirnova A, Morgacheva D, et al. Case report: Somatic mutations in microtubule dynamics-associated genes in patients with WNT-medulloblastoma tumors. Front Oncol. (2022) 12:1085947. doi: 10.3389/fonc.2022.1085947
21. Martens M, Ammar A, Riutta A, Waagmeester A, Slenter DN, Hanspers K, et al. WikiPathways: connecting communities. Nucleic Acids Res. (2021) 49:D613–21. doi: 10.1093/nar/gkaa1024
22. Ekawade A, Velinder M, Ward A, DiSera T, Miller C, Qiao Y, et al. Genepanel.iobio - an easy to use web tool for generating disease- and phenotype-associated gene lists. BMC Med Genomics. (2019) 12:190. doi: 10.1186/s12920-019-0641-1
23. Kamiya D, Banno S, Sasai N, Ohgushi M, Inomata H, Watanabe K, et al. Intrinsic transition of embryonic stem-cell differentiation into neural progenitors. Nature. (2011) 470:503–9. doi: 10.1038/nature09726
24. Scicchitano S, Giordano M, Lucchino V, Montalcini Y, Chiarella E, Aloisio A, et al. The stem cell-associated transcription co-factor, ZNF521, interacts with GLI1 and GLI2 and enhances the activity of the Sonic hedgehog pathway. Cell Death Dis. (2019) 10:715. doi: 10.1038/s41419-019-1946-x
25. Samocha KE, Kosmicki JA, Karczewski KJ, O’Donnell-Luria AH, Pierce-Hoffman E, MacArthur DG, et al. Regional missense constraint improves variant deleteriousness prediction. BioRxiv. (2017). doi: 10.1101/148353
26. Northcott PA, Buchhalter I, Morrissy AS, Hovestadt V, Weischenfeldt J, Ehrenberger T, et al. The whole-genome landscape of medulloblastoma subtypes. Nature. (2017) 547:311–7. doi: 10.1038/nature22973
27. Wong GC-H, Li KK-W, Wang W-W, Liu AP-Y, Huang QJ, Chan AK-Y, et al. Clinical and mutational profiles of adult medulloblastoma groups. Acta Neuropathol Commun. (2020) 8:191. doi: 10.1186/s40478-020-01066-6
28. Zhao S, Agafonov O, Azab A, Stokowy T, Hovig E. Accuracy and efficiency of germline variant calling pipelines for human genome data. Sci Rep. (2020) 10:20222. doi: 10.1038/s41598-020-77218-4
29. Barbitoff YA, Abasov R, Tvorogova VE, Glotov AS, Predeus AV. Systematic benchmark of state-of-the-art variant calling pipelines identifies major factors affecting accuracy of coding sequence variant discovery. BMC Genomics. (2022) 23:155. doi: 10.1186/s12864-022-08365-3
Keywords: SHH medulloblastoma, pediatric oncology, germline, DeepVariant, variant calling, HaplotypeCaller, undiagnosed cases
Citation: Skitchenko R, Smirnov S, Krapivin M, Smirnova A, Artomov M, Loboda A and Dinikina Y (2024) Case report: A case study of variant calling pipeline selection effect on the molecular diagnostics outcome. Front. Oncol. 14:1422811. doi: 10.3389/fonc.2024.1422811
Received: 24 April 2024; Accepted: 27 September 2024;
Published: 31 October 2024.
Edited by:
Larissa V. Furtado, St. Jude Children’s Research Hospital, United StatesReviewed by:
Marija Kojic, The University of Queensland, AustraliaCopyright © 2024 Skitchenko, Smirnov, Krapivin, Smirnova, Artomov, Loboda and Dinikina. This is an open-access article distributed under the terms of the Creative Commons Attribution License (CC BY). The use, distribution or reproduction in other forums is permitted, provided the original author(s) and the copyright owner(s) are credited and that the original publication in this journal is cited, in accordance with accepted academic practice. No use, distribution or reproduction is permitted which does not comply with these terms.
*Correspondence: Rostislav Skitchenko, cm9zdDIwMTUxOTk1QGdtYWlsLmNvbQ==; Yulia Dinikina, ZGluaWtpbmFfeXV2QGFsbWF6b3ZjZW50cmUucnU=
Disclaimer: All claims expressed in this article are solely those of the authors and do not necessarily represent those of their affiliated organizations, or those of the publisher, the editors and the reviewers. Any product that may be evaluated in this article or claim that may be made by its manufacturer is not guaranteed or endorsed by the publisher.
Research integrity at Frontiers
Learn more about the work of our research integrity team to safeguard the quality of each article we publish.