- 1Almazov National Medical Research Centre, St. Petersburg, Russia
- 2Computer Technologies Laboratory, ITMO University, St. Petersburg, Russia
- 3The Institute for Genomic Medicine, Nationwide Children’s Hospital, Columbus, OH, United States
- 4Department of Pediatrics, Ohio State University, Columbus, OH, United States
Medulloblastoma (MB) is the most common pediatric brain tumor which accounts for about 20% of all pediatric brain tumors and 63% of intracranial embryonal tumors. MB is considered to arise from precursor cell populations present during an early brain development. Most cases (~70%) of MB occur at the age of 1–4 and 5–9, but are also infrequently found in adults. Total annual frequency of pediatric tumors is about 5 cases per 1 million children. WNT-subtype of MB is characterized by a high probability of remission, with a long-term survival rate of about 90%. However, in some rare cases there may be increased metastatic activity, which dramatically reduces the likelihood of a favorable outcome. Here we report two cases of MB with a histological pattern consistent with desmoplastic/nodular (DP) and classic MB, and genetically classified as WNT-MB. Both cases showed putative causal somatic protein truncating mutations identified in microtubule-associated genes: ARID2, TUBB4A, and ANK3.
Introduction
Medulloblastoma (MB) – is a solid neuroepithelial tumor arising from the cerebellum. MB accounts for about 20% of all childhood brain tumors and 63% of intracranial embryonal tumors (1). MB is considered to arise from precursor cell populations present during an early brain development (2). Most cases (~70%) of MB occur at the age of 1–4 and 5–9, but are also found in adults (3). Total annual frequency of pediatric tumors is about 5 cases per 1 million children (1).
WHO declares two classifications of MB according to the method of diagnosis: histologically determined and genetically determined (4). Both groups are divided into several subgroups according to the immunohistochemical and genetic features, respectively (4). For histologically determined MB there are the following subgroups: 1) classic MB; 2) Desmoplastic/nodular MB; 3) MB with extensive nodularity; 4) Large cell/Anaplastic MB; 5) MB not otherwise specified (4). In turn, the following subgroups are distinguished for genetically defined MB: 1) WNT‐activated MB; 2) SHH‐activated, TP53‐wild‐type MB; 3) SHH‐activated, TP53‐mutant MB; 4) Non‐WNT/non‐SHH MB which is commonly divided into Group 3 and Group 4 MB (4).
Of all cases of MB, about 10% are of the wingless-type (WNT) (5). WNT-MB are usually located along the brain midline with involvement of the brainstem or cerebellar bundle and cerebellopontine angle cistern (6). WNT-MB is thought to arise from progenitor cells in the inferior rhombic lip of the developing brainstem. The vast majority of WNT tumors (~90%) contain a mutation affecting CTNNB1, which encodes β-catenin. Mutations in the tumor suppressor gene APC explain the majority of WNT-cases which do not have CTNNB1 mutations (2).
Some studies suggested the existence of two subtypes of WNT: WNTα and WNTβ. The WNTα subtype occurs mainly in children and for 98% of cases is associated with chromosome 6 monosomy, whereas the WNTβ subtype occurs in older children and adults and infrequently (29%) has monosomy (7).
Here we present molecular diagnostics for two WNT-MB cases without chromosome 6 monosomy or mutations in CTNNB1 and APC.
Methods
Clinical and genetic data collection
Patients were observed at Almazov National Medical Research Center in 2020-2022. Informed consent for molecular genetic testing was provided by parents of patients. The study was approved by the institutional ethics committee (Protocol #3502-22 from 21.02.2020).
Hematoxylin-eosin staining analysis was used for the purpose of histological classification of medulloblastomas.
A panel of three staining assays: 1) beta-catenin staining, 2) filamin A, 3) GAB1 was used to obtain immunohistochemical (IHC) confirmation of the diagnosis of MB and determine its genetically defined subtype. Ki-67 was assessed as a marker of proliferation activity along with synaptophysin expression, which is used to distinguish MB from embryonal tumor with multilayered rosettes (ETMR) and most atypical teratoid rhabdoid tumors (ATRT), which can potentially mimic MB (4).
Genomic DNA samples were prepared for sequencing using Kapa Biosystems (Roche) kits. To enrich the coding part of the genome, the TruSeq Exome Capture kit (Illumina) was used. The quality of the obtained libraries was controlled using the Fragment Analyzer. Sufficiency of the DNA quantity was assessed with the qPCR. After quality control and DNA quantity estimation, the pool of libraries was sequenced on 2 lanes of the Illumina NovaSeq 6000.
Identification of putative causal variants
We assembled a list of 616 oncogenes, based on a broad list of 565 known oncogenes (8), and an overlapping set of 87 previously reported MB susceptibility genes (Sup. Materials – Susceptibility gene lists assembly; Sup. Table S1) (9–43).
Raw sequencing data in the form of FASTQ files were obtained using bcl2fastq v2.20 Conversion Software (Illumina). Germline and somatic variant calling were performed in accordance with GATK and Mutect2 best practices (44, 45).
Identified putative somatic variants were subjected to the quality filtration using the following thresholds based on GATK metrics: 1) DP>30, 2) GERMQ>90, 3) TLOD>3, 4) POPAF≥4, 5) ROQ>85.
We took extra caution in interpreting long indels. They often could be unreliably called and require a specialized approach for analysis (46, 47). Therefore, for indels greater than 10 nucleotides that could potentially be nominated as “causal” in both patients, we manually checked the alignment of the short reads with IGV. Such an approach was carried out consistently with common standards in the field (48).
All variant coordinates mentioned are based on the reference genome version of GRCh38 and are declared according to HGVS requirements (49). In assessing the functional effect of the variants found, we rely on the joint recommendations of Clinical Genome Resource (ClinGen), Cancer Genomics Consortium (CGC), and Variant Interpretation for Cancer Consortium (VICC) (Sup. Materials – Strategy for variant oncogenicity classification) (50).
To evaluate the functional importance of identified variants, we used databases of oncogenic variants. For this purpose, we used COSMIC (51) and PeCan (52) focused on pediatric oncology. Furthermore, we use PeCan’s built-in Pathogenicity Information Exchange (PIE) (53) tool, which estimates the pathogenicity of variants based on its sample cohort and additional estimates as Sorting Intolerant From Tolerant (SIFT) (54) score, likelihood ratio test (LRT) and Combined Annotation Dependent Depletion (CADD) (55) assessments.
Results
Report of cases
The patients were a female and a male of 10 years old (hereafter Patient #1 and Patient #2) presented with complaints of headache, vomiting and visual impairments. Both patients underwent MRI analysis, surgical removal of the tumors, histological and immunohistochemical analysis. An exome sequencing from the blood and tumor DNA was performed and followed by germline and somatic variant calling. The sequencing data analysis was then performed to identify the likely genetic causes for the disease.
Patient #1
A multi-spiral CT scan (MSCT) of the brain revealed a formation in the cerebellum and brainstem as well as triventricular hydrocephalus and periventricular oedema. A magnetic resonance imaging (MRI) of the brain confirmed the results of the MSCT and additionally revealed a mass in the IV ventricle of the brain (Figure 1A); MRI screen of the spinal cord showed no signs of metastasis (Figure 1B). An additional optometric exam revealed signs of optic disc stasis. The patient was prescribed dexamethasone, which had a positive effect on reducing the headaches.
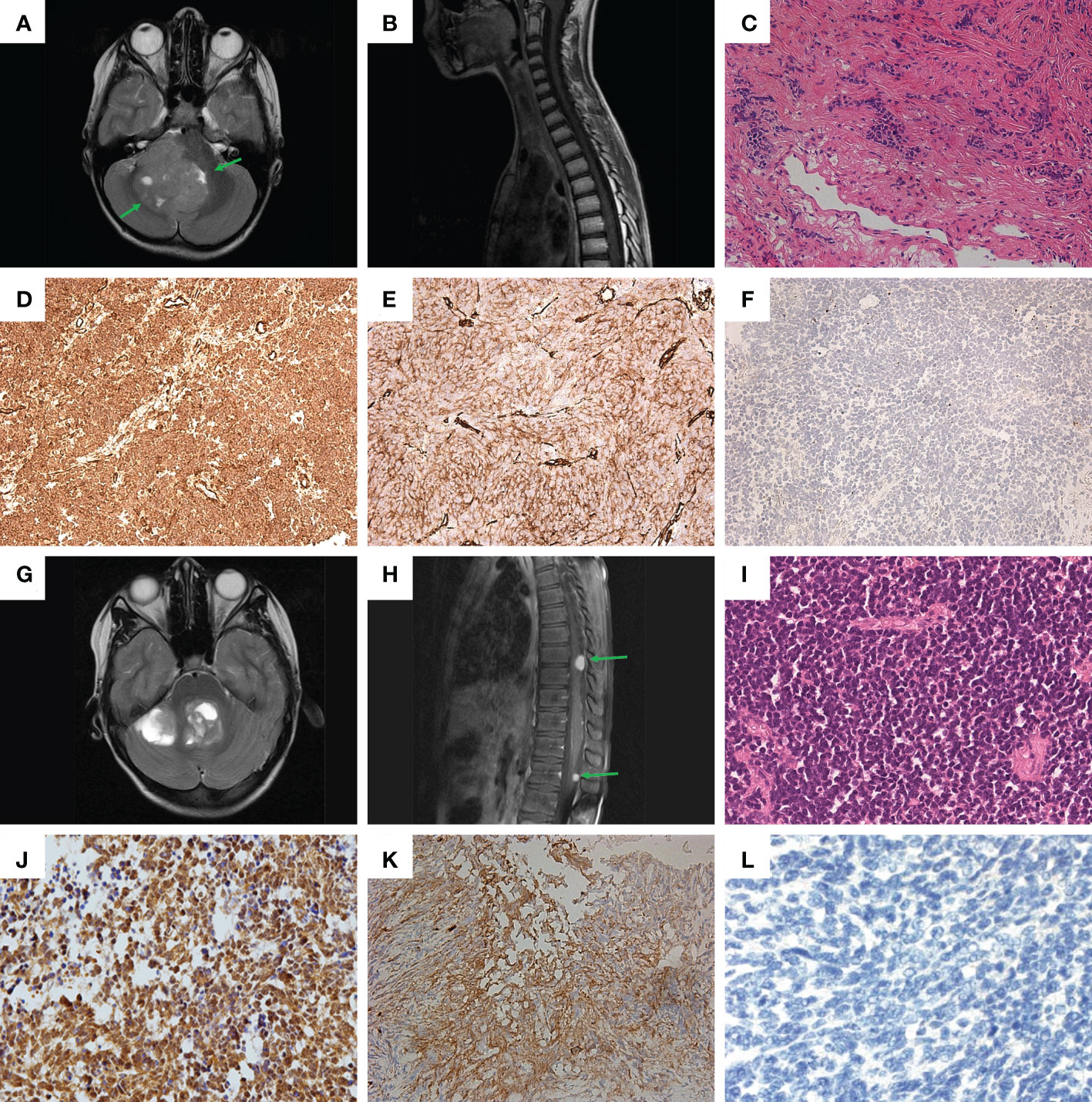
Figure 1 Clinical and histological characteristics. (A, B) – MRI screens in Patient #1: (A) brain; (B) spinal cord; (C) Hematoxylin-eosin staining of sample from Patient #1. (D-F) Immunohistochemical (IHC) staining of tumor sample from Patient #1: (D) beta-catenin; (E) filamin A; (F) GAB1. (G, H) MRI screens in Patient #2: (G) brain; (H) spinal cord. (I) Hematoxylin-eosin staining of sample from Patient #2. (J–L) IHC staining of tumor sample from Patient #2: (J) beta-catenin; (K) filamin A; (L) GAB1.
After 17 days of observation, a suboccipital bone-plastic craniotomy was performed under neurophysiological monitoring, with microsurgical removal of tumors of the cerebellum, IV ventricle and brainstem.
Further histological examination of the tumor fragments showed a highly cellular tissue sample of small cells with polymorphic hyperchromatic nuclei, with poor eosinophilic cytoplasm. Areas of nodular structure of light-colored cells were also present. The formation of Homer-Wright-type rosettes was noted. The sample was characterized by an increased number of mitoses, including atypical ones, and endothelial proliferation (Figure 1C). As a result, the tumor from Patient #1 was assigned to the desmoplastic/nodular type of MB according to the WHO classification (4).
Immunohistochemical (IHC) analysis for the sample obtained from Patient #1 revealed: 1) positive membrane-cytoplasmic and nuclear beta-catenin staining (Figure 1D); 2) positive cytoplasmic filamin A staining (Figure 1E); 3) negative GAB1 staining (Figure 1F). Therefore, the tumor was assigned to the WNT subtype, according to the genetically defined WHO classification (ICD-10-CM:C71.8; G97.9). Additionally, the proliferative activity of Ki-67 was assessed, which was about 25-30%, as well as synaptophysin expression (Figure S1A), which distinguished MB from ETMR and most ATRT, which can potentially mimic MB (4).
Patient #2
MRI of the brain showed formation in the IV ventricle and right hemisphere of the cerebellum and internal hydrocephalus (Figure 1G). In addition, MRI of the spinal cord showed signs of spinal metastasis (Figure 1H).
After 5 days, a partial surgical removal of a tumor of the right cerebellar hemisphere, IV ventricle, was performed.
Histological examination revealed a monotonous, dense-, small- and blue-cellular malignant tumor with rosettes and little stroma and numerous mitoses (Figure 1I). As a result, in the course of histological examination, the preparation from Patient #2 was assigned the classical type of MB according to the WHO classification (4).
Patient #2 had the same set of IHC confirmations as Patient #1: 1) positive membrane-cytoplasmic and nuclear beta-catenin staining (Figure 1J); 2) positive cytoplasmic filamin A staining (Figure 1K); 3) negative GAB1 staining (Figure 1L). Thus, the results of IHC analysis suggest that the tumor should be assigned to the WNT subtype, according to the genetically defined WHO classification (ICD-10-CM: C71.8; G91.1, G96.8, G83.2). Additional IHC analysis yielded the following: 1) positive expression of synaptophysin (Figure S1B); 2) Proliferative activity Ki-67 on level 20-30%.
Molecular diagnosis
Somatic variant calls were subjected to quality filtration to ensure only high-confidence somatic mutations entered the analysis (Methods). The chromosome 6 monosomy was ruled out for both patients using heterozygosity analysis that indicated presence of the two copies of the chromosome 6 (Figure S2). In total there were 50 and 37 good quality somatic variants for analysis in Patient #1 and Patient #2 respectively (Sup. Tables S2, 3). Out of these variants, 26 and 17 were eliminated from the analysis as non-coding, 3 and 1 as inframe indels, 2 and 1 were eliminated as synonymous for Patient #1 and Patient #2, respectively. Furthermore, 9 and 11 variants each with ambiguous or missing annotation were excluded from the analysis for Patient #1 and Patient #2, respectively.
Initially, we focused our analysis on missense variants and protein truncating variants (PTV). In the data, there were five and two missense variants and five PTV for each Patient #1 and Patient #2, respectively.
Patient #1 had only one variant in a gene from the list of MB susceptibility genes (87 genes list). For Patient #2, the genes from the MB susceptibility gene list did not contain any mutations.
None of the identified somatic missense variants was found in the two examined gene sets in both patients. Six of seven missense variants outside the lists of known oncogenes were eliminated as unlikely to affect any important conservative parts of the gene, as their missense deleteriousness (MPC) (56) score was ≤2 (Sup. Table S4).
Patient #1 had only one mutation in a known oncogenic gene from the analyzed list – a stop gain somatic mutation (NC_000012.12:g.45849701C>T, NM_152641:p.Gln613Ter) in ARID2 (Figure 2A), which disrupts cell cycle regulation and has previously been identified as a MB risk gene (87 genes list) (10, 11). Variant was found to be in a close proximity to RFX DNA-binding protein domain (the domain boundary is at amino acid 601). For Patient #1 it was the only PTV within the MB susceptibility gene list (87 genes list) and/or expanded gene list (616 genes list).
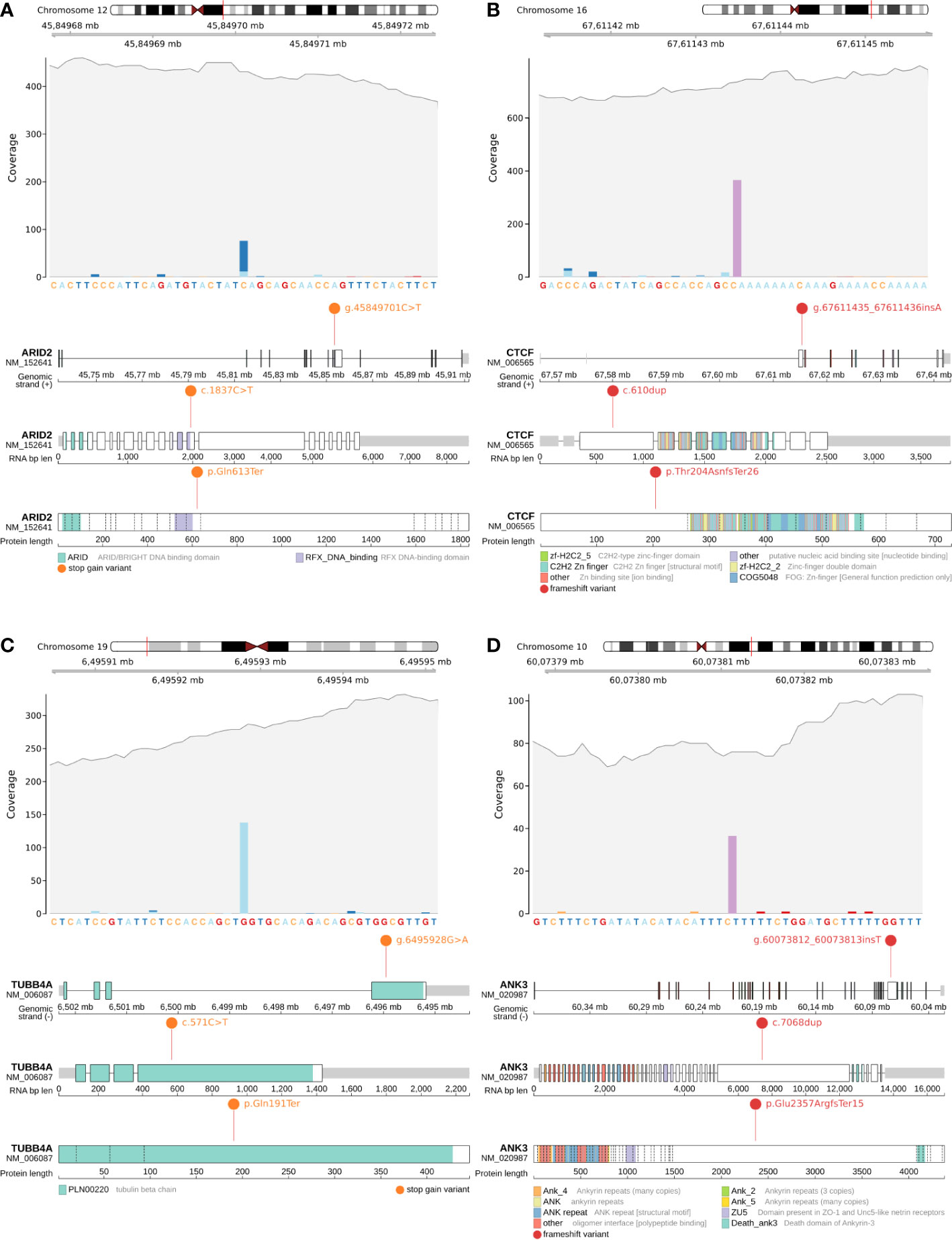
Figure 2 Candidate somatic protein truncating variants (GRCh38): (A, B) – coverage and functional effects of PTVs on DNA, RNA and protein level in Patient #1. (A)NC_000012.12:g.45849701C>T in ARID2 (NM_152641:p.Gln613Ter); (B) NC_000016.10:g.67611435_67611436insA in CTCF (NM_006565:p.Thr204AsnfsTer26); (C, D) – coverage and functional effects of PTVs on DNA, RNA and protein level in Patient #2. (C) NC_000019.10:g.6495928G>A in TUBB4A (NM_006087:p.Gln191Ter); (D) NC_000010.11:g.60073812_60073813insT in ANK3 (NM_020987:p.Glu2357ArgfsTer15).
Four other PTVs found in Patient #1 were located in NOBOX, SRRM2, CTCF, RAB11FIP4. Upon screening of these variants in IGV (57), frameshifts in SRRM2 and RAB11FIP4 were eliminated because of the poor mapping quality (Methods – Identification of putative causal variants). Frameshift variant in NOBOX was excluded from consideration because of its specific expression only in testis and ovarian tissues as was indicated by GTEX (58) (Sup. Table S5).
CTCF is an evolutionarily conserved gene responsible for the spatial properties of chromatin, including its accessibility to chromatin, so the frameshift indel (NC_000016.10:g.67611435_67611436insA, NM_006565:p.Thr204AsnfsTer26) (Figure 2B) in CTCF can potentially be considered as a secondary priority cause of MB in Patient #1.
For Patient #2, none of the variants were found in the 87 genes list. Next, we considered an extended list of 616 oncogenes in which the long frameshift in MAP2K4 was detected. We performed visual control of this PTV with IGV and eliminated this candidate variant due to poor mapping quality. In a further analysis, we considered variants in all genes and found 4 PTVs in AP003062.1, KLHL4, ANK3, TUBB4A. After visually screening all 4 variants in IGV (57), we discarded 2 long frameshifts in AP003062.1 and KLHL4 due to poor mapping quality (Methods – Identification of putative causal variants; Sup. Table S6).
The remaining pair of PTVs were stop gain somatic mutation (NC_000019.10:g.6495928G>A, NM_006087:p.Gln191Ter) in TUBB4A (rs1376427129, gnomAD_AF=6.57x10-6) (Figure 2C) and frameshift indel (NC_000010.11:g.60073812_60073813insT, NM_020987:p.Glu2357ArgfsTer15) in ANK3 (Figure 2D).
Conclusively, taking into account clinical symptoms, IHC and genetic analyses the diagnosis was defined as WNT-β medulloblastomas without chromosome 6 monosomy and no known mutations in CTNNB1 and APC. Novel identified risk variants align well with the previous knowledge of ANK3, TUBB4A, ARID2 and CTCF functionality in cancer but the specific variants that were identified in these patients have not been observed previously. In addition, the role of these variants in pediatric tumors of the central nervous system has not been previously reported.
Discussion
Patient #1
The ARID2 is a highly conservative gene (pLI=1) involved in various biological processes, including the cell cycle control, regulation of transcription and modification of chromatin structure and is a known tumor suppressor gene (8). The ARID2 gene product functions as a subunit of the PBAF (SWI/SNF-B) chromatin remodeling complex, which promotes ligand-dependent transcriptional activation by nuclear receptors. It was previously known that ARID2 co-immunoprecipitates with α-tubulin and that ARID2 localizes to the spindle pole during mitosis (59). Rare somatic mutations in ARID2 can lead to severe phenotypes, including MB. For one-third of WNT-MB cases, functional annotation of the recurrently altered genes revealed somatic dysregulation of chromatin modeling genes of the SWI/SNF family, which also includes ARID2 (10, 60). PeCan (52) did not show an exact match for the p.Gln613Ter in ARID2 in pediatric oncology reports. However, PeCan’s (52) built-in PIE classified p.Gln613Ter as “GOLD” [“truncation in gold gene (tumor suppressor)”], likewise based on LRT (“Deleterious”) and CADD (CADD=38, CADDraw=11.70) estimates. According to COSMIC, p.Gln613Ter in ARID2, has been reported several times in the database as a variant found in various cancer types, though not in the central nervous system (61–63). We categorize g.45849701C>T as “oncogenic” according to accumulated evidence, as suggested by Horak et al. (Sup. Materials – Strategy of variant oncogenicity classification) (50).
Considering CTCF as a secondary finding in Patient 1 it is worth noting its properties of regulating chromatin spatial regulation. It is known that CTCF-binding sites often define topological associating chromatin domains (TAD) boundaries and removal of these sites can lead to a moderate upregulation of a nearby gene. Therefore, alterations in CTCF genotype may potentially lead to significant gene expression alterations (64–66). Variant p.Thr204AsnfsTer26 was found to have an exact match with ClinVar and was assessed as “pathogenic” (Variation ID: 280869). PeCan (52) has shown that variant p.Thr204AsnfsTer26 has already been reported several times in pediatric oncology studies of lymphoblastic leukemia and solid tumors (67–69). PIE classified p.Thr204AsnfsTer26 as “GOLD”. Additionally, COSMIC shows multiple lines of evidence in studies involving various tumor types (65, 70, 71). The abundance of evidence in the database allows this variant to be identified as a cancer hotspot. CTCF is a very conservative gene, with almost no PTVs observed in germline DNA in large population-based cohorts (pLI=1), yet, there was no specific linkage to pediatric brain tumors reported to date. The accumulated evidence for g.67611435_67611436insA indicates that this is an “oncogenic” variant (Sup. Materials – Strategy of variant oncogenicity classification) (50).
Patient #2
In a previous survival analysis study, TUBB4A expression in tumors was found to be associated with MB patients survival, suggesting that TUBB4A may have oncogenic properties (72). Interestingly, observed PTV is found in the last exon of the gene. Previous studies indicated that in other genes, including cancer genes, such mutations result in gain-of-function effect (73–75). This is consistent with the observation of lower expression of TUBB4A benefiting the survival. TUBB4A is non-conservative gene (pLI=0.11), which could potentially reduce the effect of PTV on viability. Missense mutations in TUBB4A are known to affect various neurological phenotypes, including those associated with cerebellar atrophy, early infantile encephalopathy, which may be due to the selective effects of different mutations on cells and microtubule dynamics (76).
Microtubules are components of the cytoskeleton that contribute to the morphology of axons and dendrites in neurons and facilitate the transport of cell cargos. In dividing cells, microtubules of polymerized α-/β-tubulin dimers control the process of mitosis at different stages of its course, which has been previously well studied (77, 78). Microtubules are prone to constant phases of polymerization and depolymerization, and changes in microtubule dynamics can lead to errors in chromosome segregation and chromosome instability, a key feature of oncological cells (78–81).
In cancer cells, changes in microtubules dynamics, often associated with cancer-specific tubulin isotypes and tubulin post-translational modifications, are involved in metastatic cell migration, drug resistance, and tumor vascularization (81, 82). It is important to clarify that the hyperfunction of tubulin motility in mitosis is also a molecular target for numerous “antitubulin agents”, which have been shown to interact with multiple sites on α- or β-tubulin and have been successfully used as chemotherapeutic agents to induce mitotic arrest and cancer cell death (83, 84).
The ANK3 regulates the mitogen−activated protein kinase (MAPK) pathway related to extracellular matrix organization, cell motility through PTK2 signaling and somatodendritic inhibitory synapses, which determines its high conservativity (pLI=1) (85). Abnormalities in MAPK signaling are known to be associated with the process of metastasis and have long been proposed as targets for selective therapy for oncologies, since the presence or absence of metastasis often determines the prognosis of survival (85). But, even more importantly, that brain-specific Ank3 is linked to microtubule dynamics through a GSK3/CRMP2-dependent mechanism, which has been confirmed using mouse models (86). There is evidence that increased ANK3 expression in cancer tissues correlates with better survival in prostate cancer, suggesting that ANK3 is a tumor suppressor gene (87).
Early gene expression studies in the hippocampus of Ank3+/- and Ank3+/+ mice revealed altered expression of 282 genes that were enriched with microtubule-related functions (86). ANK3 binds microtubules directly or through the binding of microtubule-associated proteins at the plus-end stabilization cap, which prevents depolymerization and directly affects microtubule dynamics (88–90).
COSMIC and PeCan did not show an exact match with the p.Gln191Ter in TUBB4A and p.Glu2357ArgfsTer15 in ANK3, which makes it impossible to classify them as cancer hotspots. PIE has added evidence of p.Gln191Ter in TUBB4A oncogenicity through SIFT (“Damaging”), CADD (CADD=36, CADDraw=10.41) and LRT (“Deleterious”). PIE did not have sufficient information about p.Glu2357ArgfsTer15 in ANK3. Given involvement of these variants in oncological processes, the severity of the functional effect on the protein, and the available data from the survival analysis incline us to classify g.6495928G>A in TUBB4A as “variant of uncertain significance” and g.60073812_60073813insT in ANK3 as “oncogenic” (Sup. Materials – Strategy of variant oncogenicity classification) (50).
Conclusively, we identified four candidate somatic mutations potentially explaining the MB onset in two pediatric patients and providing new biological insights into the mechanisms of the pediatric tumor development.
Data availability statement
A full list of somatic mutations passing quality filtration is available in Sup. Tables S1 and S2. Patients’ consents for clinical DNA sequencing do not permit free data sharing, however, reasonable requests for specific details of genotypes, not conflicting with consent could be accommodated by contacting the corresponding author.
Ethics statement
The studies involving human participants were reviewed and approved by Almazov National Medical Research Center institutional ethics committee (Protocol # 3502-22 of 21.02.2020). Written informed consent to participate in this study was provided by the participants’ legal guardian/next of kin. Written informed consent was obtained from the minor(s)’ legal guardian/next of kin for the publication of any potentially identifiable images or data included in this article.
Author contributions
RK, YD, MA designed the study. YD, SS, MK, AS, DM directly supervised patients and obtained biospecimen. RK, MA, analyzed the data. RK, MA wrote the manuscript. All authors contributed to the article and approved the submitted version.
Funding
RS, YD, SS, MK, AS, DM were supported by funding from the Ministry of Science and Higher Education of the Russian Federation (Agreement #075-15-2022-301). MA was supported by the Nationwide Foundation Pediatric Innovation Fund.
Acknowledgments
RS and MA thank Dr. Alina Babenko (Almazov Centre) for her valuable support to the project.
Conflict of interest
The authors declare that the research was conducted in the absence of any commercial or financial relationships that could be construed as a potential conflict of interest.
Publisher’s note
All claims expressed in this article are solely those of the authors and do not necessarily represent those of their affiliated organizations, or those of the publisher, the editors and the reviewers. Any product that may be evaluated in this article, or claim that may be made by its manufacturer, is not guaranteed or endorsed by the publisher.
Supplementary material
The Supplementary Material for this article can be found online at: https://www.frontiersin.org/articles/10.3389/fonc.2022.1085947/full#supplementary-material
References
1. Ostrom QT, Gittleman H, Truitt G, Boscia A, Kruchko C, Barnholtz-Sloan JS. CBTRUS statistical report: Primary brain and other central nervous system tumors diagnosed in the united states in 2011-2015. Neuro Oncol (2018) 20:iv1–iv86. doi: 10.1093/neuonc/noy131
2. Juraschka K, Taylor MD. Medulloblastoma in the age of molecular subgroups: A review. J Neurosurg Pediatr (2019) 24:353–63. doi: 10.3171/2019.5.PEDS18381
3. Cervoni L, Maleci A, Salvati M, Delfini R, Cantore G. Medulloblastoma in late adults: Report of two cases and critical review of the literature. J Neurooncol (1994) 19:169–73. doi: 10.1007/BF01306459
4. Orr BA. Pathology, diagnostics, and classification of medulloblastoma. Brain Pathol (2020) 30:664–78. doi: 10.1111/bpa.12837
5. Taylor MD, Northcott PA, Korshunov A, Remke M, Cho Y-J, Clifford SC, et al. Molecular subgroups of medulloblastoma: The current consensus. Acta Neuropathol (2012) 123:465–72. doi: 10.1007/s00401-011-0922-z
6. Perreault S, Ramaswamy V, Achrol AS, Chao K, Liu TT, Shih D, et al. MRI Surrogates for molecular subgroups of medulloblastoma. AJNR Am J Neuroradiol (2014) 35:1263–9. doi: 10.3174/ajnr.A3990
7. Cavalli FMG, Remke M, Rampasek L, Peacock J, Shih DJH, Luu B, et al. Intertumoral heterogeneity within medulloblastoma subgroups. Cancer Cell (2017) 31:737–754.e6. doi: 10.1016/j.ccell.2017.05.005
8. Zhang J, Walsh MF, Wu G, Edmonson MN, Gruber TA, Easton J, et al. Germline mutations in predisposition genes in pediatric cancer. N Engl J Med (2015) 373:2336–46. doi: 10.1056/NEJMoa1508054
9. Williamson D, Schwalbe EC, Hicks D, Aldinger KA, Lindsey JC, Crosier S, et al. Medulloblastoma group 3 and 4 tumors comprise a clinically and biologically significant expression continuum reflecting human cerebellar development. Cell Rep (2022) 40:111162. doi: 10.1016/j.celrep.2022.111162
10. Northcott PA, Buchhalter I, Morrissy AS, Hovestadt V, Weischenfeldt J, Ehrenberger T, et al. The whole-genome landscape of medulloblastoma subtypes. Nature (2017) 547:311–7. doi: 10.1038/nature22973
11. Wong GC-H, Li KK-W, Wang W-W, Liu AP-Y, Huang QJ, Chan AK-Y, et al. Clinical and mutational profiles of adult medulloblastoma groups. Acta Neuropathol Commun (2020) 8191. doi: 10.1186/s40478-020-01066-6
12. Estiar MA, Mehdipour P. ATM In breast and brain tumors: A comprehensive review. Cancer Biol Med (2018) 15:210–27. doi: 10.20892/j.issn.2095-3941.2018.0022
13. Groves A, Clymer J, Filbin MG. Bromodomain and extra-terminal protein inhibitors: Biologic insights and therapeutic potential in pediatric brain tumors. Pharm (Basel) (2022) 15. doi: 10.3390/ph15060665
14. Raleigh DR, Choksi PK, Krup AL, Mayer W, Santos N, Reiter JF. Hedgehog signaling drives medulloblastoma growth via CDK6. J Clin Invest (2018) 128:120–4. doi: 10.1172/JCI92710
15. Packer RJ, Hoffman EP. Neuro-oncology: Understanding the molecular complexity of medulloblastoma. Nat Rev Neurol (2012) 8:539–40. doi: 10.1038/nrneurol.2012.197
16. Parsons DW, Li M, Zhang X, Jones S, Leary RJ, Lin JC-H, et al. The genetic landscape of the childhood cancer medulloblastoma. Science (2011) 331:435–9. doi: 10.1126/science.1198056
17. Fang FY, Rosenblum JS, Ho WS, Heiss JD. New developments in the pathogenesis, therapeutic targeting, and treatment of pediatric medulloblastoma. Cancers (Basel) (2022) 14. doi: 10.3390/cancers14092285
18. Lin CY, Erkek S, Tong Y, Yin L, Federation AJ, Zapatka M, et al. Active medulloblastoma enhancers reveal subgroup-specific cellular origins. Nature (2016) 530:57–62. doi: 10.1038/nature16546
19. Miele E, Mastronuzzi A, Po A, Carai A, Alfano V, Serra A, et al. Characterization of medulloblastoma in fanconi anemia: A novel mutation in the BRCA2 gene and SHH molecular subgroup. biomark Res (2015) 3:13. doi: 10.1186/s40364-015-0038-z
20. Zwergel C, Romanelli A, Stazi G, Besharat ZM, Catanzaro G, Tafani M, et al. Application of small epigenetic modulators in pediatric medulloblastoma. Front Pediatr (2018) 6:370. doi: 10.3389/fped.2018.00370
21. Sengupta S, Weeraratne SD, Sun H, Phallen J, Rallapalli SK, Teider N, et al. α5-GABAA receptors negatively regulate MYC-amplified medulloblastoma growth. Acta Neuropathol (2014) 127:593–603. doi: 10.1007/s00401-013-1205-7
22. Huang M, Tailor J, Zhen Q, Gillmor AH, Miller ML, Weishaupt H, et al. Engineering genetic predisposition in human neuroepithelial stem cells recapitulates medulloblastoma tumorigenesis. Cell Stem Cell (2019) 25:433–446.e7. doi: 10.1016/j.stem.2019.05.013
23. Ecker J, Oehme I, Mazitschek R, Korshunov A, Kool M, Hielscher T, et al. Targeting class I histone deacetylase 2 in MYC amplified group 3 medulloblastoma. Acta Neuropathol Commun (2015) 3:22. doi: 10.1186/s40478-015-0201-7
24. Gershanov S, Madiwale S, Feinberg-Gorenshtein G, Vainer I, Nehushtan T, Michowiz S, et al. Classifying medulloblastoma subgroups based on small, clinically achievable gene sets. Front Oncol (2021) 11:637482. doi: 10.3389/fonc.2021.637482
25. Pajtler KW, Weingarten C, Thor T, Künkele A, Heukamp LC, Büttner R, et al. The KDM1A histone demethylase is a promising new target for the epigenetic therapy of medulloblastoma. Acta Neuropathol Commun (2013) 1:19. doi: 10.1186/2051-5960-1-19
26. Azatyan A, Zaphiropoulos PG. Circular and fusion rnas in medulloblastoma development. Cancers (Basel) (2022) 14. doi: 10.3390/cancers14133134
27. Guo C, Chen LH, Huang Y, Chang C-C, Wang P, Pirozzi CJ, et al. KMT2D maintains neoplastic cell proliferation and global histone H3 lysine 4 monomethylation. Oncotarget (2013) 4:2144–53. doi: 10.18632/oncotarget.1555
28. Lee C, Rudneva VA, Erkek S, Zapatka M, Chau LQ, Tacheva-Grigorova SK, et al. Lsd1 as a therapeutic target in Gfi1-activated medulloblastoma. Nat Commun (2019) 10:332. doi: 10.1038/s41467-018-08269-5
29. Chang F-C, Wong T-T, Wu K-S, Lu C-F, Weng T-W, Liang M-L, et al. Magnetic resonance radiomics features and prognosticators in different molecular subtypes of pediatric medulloblastoma. PloS One (2021) 16:e0255500. doi: 10.1371/journal.pone.0255500
30. Dahlin AM, Wibom C, Andersson U, Bybjerg-Grauholm J, Deltour I, Hougaard DM, et al. A genome-wide association study on medulloblastoma. J Neurooncol (2020) 147:309–15. doi: 10.1007/s11060-020-03424-9
31. Northcott PA, Korshunov A, Witt H, Hielscher T, Eberhart CG, Mack S, et al. Medulloblastoma comprises four distinct molecular variants. J Clin Oncol (2011) 29:1408–14. doi: 10.1200/JCO.2009.27.4324
32. Garancher A, Lin CY, Morabito M, Richer W, Rocques N, Larcher M, et al. NRL and CRX define photoreceptor identity and reveal subgroup-specific dependencies in medulloblastoma. Cancer Cell (2018) 33:435–449.e6. doi: 10.1016/j.ccell.2018.02.006
33. Menyhárt O, Giangaspero F, Győrffy B. Molecular markers and potential therapeutic targets in non-WNT/non-SHH (group 3 and group 4) medulloblastomas. J Hematol Oncol (2019) 12:29. doi: 10.1186/s13045-019-0712-y
34. Niesen J, Ohli J, Sedlacik J, Dührsen L, Hellwig M, Spohn M, et al. Pik3ca mutations significantly enhance the growth of SHH medulloblastoma and lead to metastatic tumour growth in a novel mouse model. Cancer Lett (2020) 477:10–8. doi: 10.1016/j.canlet.2020.02.028
35. Skowron P, Farooq H, Cavalli FMG, Morrissy AS, Ly M, Hendrikse LD, et al. The transcriptional landscape of shh medulloblastoma. Nat Commun (2021) 12:1749. doi: 10.1038/s41467-021-21883-0
36. Shi D-L. RBM24 in the post-transcriptional regulation of cancer progression: Anti-tumor or pro-tumor activity? Cancers (Basel) (2022) 14. doi: 10.3390/cancers14071843
37. Ma J-X, Li H, Chen X-M, Yang X-H, Wang Q, Wu M-L, et al. Expression patterns and potential roles of SIRT1 in human medulloblastoma cells in vivo and in vitro. Neuropathology (2013) 33:7–16. doi: 10.1111/j.1440-1789.2012.01318.x
38. Remke M, Ramaswamy V, Peacock J, Shih DJH, Koelsche C, Northcott PA, et al. TERT promoter mutations are highly recurrent in SHH subgroup medulloblastoma. Acta Neuropathol (2013) 126:917–29. doi: 10.1007/s00401-013-1198-2
39. Kaur K, Kakkar A, Kumar A, Mallick S, Julka PK, Gupta D, et al. Integrating molecular subclassification of medulloblastomas into routine clinical practice: A simplified approach. Brain Pathol (2016) 26:334–43. doi: 10.1111/bpa.12293
40. Yi J, Shi X, Xuan Z, Wu J. Histone demethylase UTX/KDM6A enhances tumor immune cell recruitment, promotes differentiation and suppresses medulloblastoma. Cancer Lett (2021) 499:188–200. doi: 10.1016/j.canlet.2020.11.031
41. Zou H, Poore B, Broniscer A, Pollack IF, Hu B. Molecular heterogeneity and cellular diversity: implications for precision treatment in medulloblastoma. Cancers (Basel) (2020) 12. doi: 10.3390/cancers12030643
42. Geron L, Salomão KB, Borges KS, Andrade AF, Corrêa CAP, Scrideli CA, et al. Molecular characterization of wnt pathway and function of β-catenin overexpression in medulloblastoma cell lines. Cytotechnology (2018) 70:1713–22. doi: 10.1007/s10616-018-0260-2
43. Aruga J, Nozaki Y, Hatayama M, Odaka YS, Yokota N. Expression of ZIC family genes in meningiomas and other brain tumors. BMC Cancer (2010) 10:79. doi: 10.1186/1471-2407-10-79
44. Van der Auwera GA, Carneiro MO, Hartl C, Poplin R, Del Angel G, Levy-Moonshine A, et al. From FastQ data to high confidence variant calls: The genome analysis toolkit best practices pipeline. Curr Protoc Bioinf (2013) 11:11.10.1–11.10.33. doi: 10.1002/0471250953.bi1110s43
45. Benjamin DI, Sato T, Cibulskis K, Getz G, Stewart C, Lichtenstein L. Calling somatic SNVs and indels with Mutect2. BioRxiv (2019). doi: 10.1101/861054
46. Fang H, Wu Y, Narzisi G, O’Rawe JA, Barrón LTJ, Rosenbaum J, et al. Reducing INDEL calling errors in whole genome and exome sequencing data. Genome Med (2014) 6:89. doi: 10.1186/s13073-014-0089-z
47. Bhuyan MSI, Pe’er I, Rahman MS. SICaRiO: Short indel call filtering with boosting. Brief Bioinf (2021) 22. doi: 10.1093/bib/bbaa238
48. Koboldt DC. Best practices for variant calling in clinical sequencing. Genome Med (2020) 12:91. doi: 10.1186/s13073-020-00791-w
49. den Dunnen JT, Dalgleish R, Maglott DR, Hart RK, Greenblatt MS, McGowan-Jordan J, et al. HGVS recommendations for the description of sequence variants: 2016 update. Hum Mutat (2016) 37:564–9. doi: 10.1002/humu.22981
50. Horak P, Griffith M, Danos AM, Pitel BA, Madhavan S, Liu X, et al. Standards for the classification of pathogenicity of somatic variants in cancer (oncogenicity): Joint recommendations of clinical genome resource (ClinGen), cancer genomics consortium (CGC), and variant interpretation for cancer consortium (VICC). Genet Med (2022) 24:986–98. doi: 10.1016/j.gim.2022.01.001
51. Tate JG, Bamford S, Jubb HC, Sondka Z, Beare DM, Bindal N, et al. COSMIC: the catalogue of somatic mutations in cancer. Nucleic Acids Res (2019) 47:D941–7. doi: 10.1093/nar/gky1015
52. McLeo C, Gout AM, Zhou X, Thrasher A, Rahbarinia D, Brady AW, et al. St. Jude Cloud: A pediatric cancer genomic data-sharing ecosystem. Cancer Discov (2021) 11:1082–1099. doi: 10.1158/2159-8290.CD-20-1230
53. Edmonson MN, Patel AN, Hedges DJ, Wang Z, Rampersaud E, Kesserwan CA, et al. Pediatric cancer variant pathogenicity information exchange (PeCanPIE): A cloud-based platform for curating and classifying germline variants. Genome Res (2019) 29:1555–1565. doi: 10.1101/gr.250357.119
54. Ng PC, Henikoff S. SIFT: Predicting amino acid changes that affect protein function. Nucleic Acids Res (2003) 31:3812–4. doi: 10.1093/nar/gkg509
55. Rentzsch P, Witten D, Cooper GM, Shendure J, Kircher M. CADD: predicting the deleteriousness of variants throughout the human genome. Nucleic Acids Res (2019) 47:D886–94. doi: 10.1093/nar/gky1016
56. Samocha KE, Kosmicki JA, Karczewski KJ, O’Donnell-Luria AH, Pierce-Hoffman E, MacArthur DG, et al. Regional missense constraint improves variant deleteriousness prediction. BioRxiv (2017). doi: 10.1101/148353
57. Robinson JT, Thorvaldsdóttir H, Winckler W, Guttman M, Lander ES, Getz G, et al. Integrative genomics viewer. Nat Biotechnol (2011) 29:24–6. doi: 10.1038/nbt.1754
58. GTEx Consortium. The genotype-tissue expression (GTEx) project. Nat Genet (2013) 45:580–5. doi: 10.1038/ng.2653
59. Karki M, Jangid RK, Anish R, Seervai RNH, Bertocchio J-P, Hotta T, et al. A cytoskeletal function for PBRM1 reading methylated microtubules. Sci Adv (2021) 7. doi: 10.1126/sciadv.abf2866
60. Hovestadt V, Ayrault O, Swartling FJ, Robinson GW, Pfister SM, Northcott PA. Medulloblastomics revisited: biological and clinical insights from thousands of patients. Nat Rev Cancer (2020) 20:42–56. doi: 10.1038/s41568-019-0223-8
61. Wardell CP, Fujita M, Yamada T, Simbolo M, Fassan M, Karlic R, et al. Genomic characterization of biliary tract cancers identifies driver genes and predisposing mutations. J Hepatol (2018) 68:959–69. doi: 10.1016/j.jhep.2018.01.009
62. Kan Z, Zheng H, Liu X, Li S, Barber TD, Gong Z, et al. Whole-genome sequencing identifies recurrent mutations in hepatocellular carcinoma. Genome Res (2013) 23:1422–33. doi: 10.1101/gr.154492.113
63. Zehir A, Benayed R, Shah RH, Syed A, Middha S, Kim HR, et al. Mutational landscape of metastatic cancer revealed from prospective clinical sequencing of 10,000 patients. Nat Med (2017) 23:703–13. doi: 10.1038/nm.4333
64. Aulmann S, Bläker H, Penzel R, Rieker RJ, Otto HF, Sinn HP. CTCF gene mutations in invasive ductal breast cancer. Breast Cancer Res Treat (2003) 80:347–52. doi: 10.1023/A:1024930404629
65. Zighelboim I, Mutch DG, Knapp A, Ding L, Xie M, Cohn DE, et al. High frequency strand slippage mutations in CTCF in MSI-positive endometrial cancers. Hum Mutat (2014) 35:63–5. doi: 10.1002/humu.22463
66. Debaugny RE, Skok JA. CTCF and CTCFL in cancer. Curr Opin Genet Dev (2020) 61:44–52. doi: 10.1016/j.gde.2020.02.021
67. Ma X, Liu Y, Liu Y, Alexandrov LB, Edmonson MN, Gawad C, et al. Pan-cancer genome and transcriptome analyses of 1,699 paediatric leukaemias and solid tumours. Nature (2018) 555:371–6. doi: 10.1038/nature25795
68. Liu Y, Easton J, Shao Y, Maciaszek J, Wang Z, Wilkinson MR, et al. The genomic landscape of pediatric and young adult T-lineage acute lymphoblastic leukemia. Nat Genet (2017) 49:1211–8. doi: 10.1038/ng.3909
69. Zhang J, Ding L, Holmfeldt L, Wu G, Heatley SL, Payne-Turner D, et al. The genetic basis of early T-cell precursor acute lymphoblastic leukaemia. Nature (2012) 481:157–63. doi: 10.1038/nature10725
70. Walker CJ, Miranda MA, O’Hern MJ, McElroy JP, Coombes KR, Bundschuh R, et al. Patterns of CTCF and ZFHX3 mutation and associated outcomes in endometrial cancer. J Natl Cancer Inst (2015) 107. doi: 10.1093/jnci/djv249
71. Yang J, Lin Y, Huang Y, Jin J, Zou S, Zhang X, et al. Genome landscapes of rectal cancer before and after preoperative chemoradiotherapy. Theranostics (2019) 9:6856–66. doi: 10.7150/thno.37794
72. Qin C, Pan Y, Li Y, Li Y, Long W, Liu Q. Novel molecular hallmarks of group 3 medulloblastoma by single-cell transcriptomics. Front Oncol (2021) 11:622430. doi: 10.3389/fonc.2021.622430
73. Hamanaka K, Imagawa E, Koshimizu E, Miyatake S, Tohyama J, Yamagata T, et al. De novo truncating variants in the last exon of SEMA6B cause progressive myoclonic epilepsy. Am J Hum Genet (2020) 106:549–58. doi: 10.1016/j.ajhg.2020.02.011
74. Dinan AM, Atkins JF, Firth AE. ASXL gain-of-function truncation mutants: Defective and dysregulated forms of a natural ribosomal frameshifting product? Biol Direct (2017) 12:24. doi: 10.1186/s13062-017-0195-0
75. Ruark E, Snape K, Humburg P, Loveday C, Bajrami I, Brough R, et al. Mosaic PPM1D mutations are associated with predisposition to breast and ovarian cancer. Nature (2013) 493:406–10. doi: 10.1038/nature11725
76. Curiel J, Rodríguez Bey G, Takanohashi A, Bugiani M, Fu X, Wolf NI, et al. TUBB4A mutations result in specific neuronal and oligodendrocytic defects that closely match clinically distinct phenotypes. hum. Mol Genet (2017) 26:4506–18. doi: 10.1093/hmg/ddx338
77. Mitchison T, Kirschner M. Dynamic instability of microtubule growth. Nature (1984) 312:237–42. doi: 10.1038/312237a0
78. Trisciuoglio D, Degrassi F. The tubulin code and tubulin-modifying enzymes in autophagy and cancer. Cancers (Basel) (2021) 14. doi: 10.3390/cancers14010006
79. Bakhoum SF, Compton DA. Chromosomal instability and cancer: a complex relationship with therapeutic potential. J Clin Invest (2012) 122:1138–43. doi: 10.1172/JCI59954
80. Cirillo L, Gotta M, Meraldi P. The elephant in the room: the role of microtubules in cancer. Adv Exp Med Biol (2017) 1002:93–124. doi: 10.1007/978-3-319-57127-0_5
81. Lopes D, Maiato H. The tubulin code in mitosis and cancer. Cells (2020) 9. doi: 10.20944/preprints202010.0433.v1
82. Nekooki-Machida Y, Hagiwara H. Role of tubulin acetylation in cellular functions and diseases. Med Mol Morphol (2020) 53:191–7. doi: 10.1007/s00795-020-00260-8
83. Matthew S, Chen Q-Y, Ratnayake R, Fermaintt CS, Lucena-Agell D, Bonato F, et al. Gatorbulin-1, a distinct cyclodepsipeptide chemotype, targets a seventh tubulin pharmacological site. Proc Natl Acad Sci USA (2021) 118. doi: 10.1073/pnas.2021847118
84. Zhang D, Kanakkanthara A. Beyond the paclitaxel and vinca alkaloids: Next generation of plant-derived microtubule-targeting agents with potential anticancer activity. Cancers (Basel) (2020) 12:1721. doi: 10.3390/cancers12071721
85. MacDonald TJ, Brown KM, LaFleur B, Peterson K, Lawlor C, Chen Y, et al. Expression profiling of medulloblastoma: PDGFRA and the RAS/MAPK pathway as therapeutic targets for metastatic disease. Nat Genet (2001) 29:143–52. doi: 10.1038/ng731
86. Garza JC, Qi X, Gjeluci K, Leussis MP, Basu H, Reis SA, et al. Disruption of the psychiatric risk gene ankyrin 3 enhances microtubule dynamics through GSK3/CRMP2 signaling. Transl Psychiatry (2018) 8:135. doi: 10.1038/s41398-018-0182-y
87. Wang T, Abou-Ouf H, Hegazy SA, Alshalalfa M, Stoletov K, Lewis J, et al. Ankyrin G expression is associated with androgen receptor stability, invasiveness, and lethal outcome in prostate cancer patients. J Mol Med (2016) 94:1411–22. doi: 10.1007/s00109-016-1458-4
88. Bennett V, Davis J. Erythrocyte ankyrin: immunoreactive analogues are associated with mitotic structures in cultured cells and with microtubules in brain. Proc Natl Acad Sci USA (1981) 78:7550–4. doi: 10.1073/pnas.78.12.7550
89. Davis JQ, Bennett V. Brain ankyrin. a membrane-associated protein with binding sites for spectrin, tubulin, and the cytoplasmic domain of the erythrocyte anion channel. J Biol Chem (1984) 259:13550–9. doi: 10.1016/S0021-9258(18)90728-3
Keywords: medulloblastoma, exome sequence data, somatic mutation analysis, Wnt, microtubule - associated proteins
Citation: Skitchenko R, Dinikina Y, Smirnov S, Krapivin M, Smirnova A, Morgacheva D and Artomov M (2023) Case report: Somatic mutations in microtubule dynamics-associated genes in patients with WNT-medulloblastoma tumors. Front. Oncol. 12:1085947. doi: 10.3389/fonc.2022.1085947
Received: 31 October 2022; Accepted: 07 December 2022;
Published: 12 January 2023.
Edited by:
Lucia Quaglietta, AORN Santobono-Pausilipon, ItalyReviewed by:
Pasqualino De Antonellis, University of Toronto, CanadaDomenico Roberti, University of Campania Luigi Vanvitelli, Italy
Copyright © 2023 Skitchenko, Dinikina, Smirnov, Krapivin, Smirnova, Morgacheva and Artomov. This is an open-access article distributed under the terms of the Creative Commons Attribution License (CC BY). The use, distribution or reproduction in other forums is permitted, provided the original author(s) and the copyright owner(s) are credited and that the original publication in this journal is cited, in accordance with accepted academic practice. No use, distribution or reproduction is permitted which does not comply with these terms.
*Correspondence: Mykyta Artomov, bXlreXRhLmFydG9tb3ZAbmF0aW9ud2lkZWNoaWxkcmVucy5vcmc=