- 1Department of Health Information Management, School of Allied Medical Sciences, Tehran University of Medical Sciences, Tehran, Iran
- 2Department of Health Information Technology and Management, School of Allied Medical Sciences, Shahid Beheshti University of Medical Sciences, Tehran, Iran
- 3Department of Aerospace Engineering, Khaje Nasir Toosi University of Technology, Tehran, Iran
- 4Department of Electrical and Software Engineering, University of Calgary, Calgary, AB, Canada
- 5Department of Computer Science, University of Arizona, Tucson, AZ, United States
- 6Department of Electrical Engineering, University of Guilan, Rasht, Iran
- 7Cancer Research Center, Shahid Beheshti University of Medical Sciences, Tehran, Iran
Background: Breast cancer (BC), as a leading cause of cancer mortality in women, demands robust prediction models for early diagnosis and personalized treatment. Artificial Intelligence (AI) and Machine Learning (ML) algorithms offer promising solutions for automated survival prediction, driving this study’s systematic review and meta-analysis.
Methods: Three online databases (Web of Science, PubMed, and Scopus) were comprehensively searched (January 2016-August 2023) using key terms (“Breast Cancer”, “Survival Prediction”, and “Machine Learning”) and their synonyms. Original articles applying ML algorithms for BC survival prediction using clinical data were included. The quality of studies was assessed via the Qiao Quality Assessment tool.
Results: Amongst 140 identified articles, 32 met the eligibility criteria. Analyzed ML methods achieved a mean validation accuracy of 89.73%. Hybrid models, combining traditional and modern ML techniques, were mostly considered to predict survival rates (40.62%). Supervised learning was the dominant ML paradigm (75%). Common ML methodologies included pre-processing, feature extraction, dimensionality reduction, and classification. Deep Learning (DL), particularly Convolutional Neural Networks (CNNs), emerged as the preferred modern algorithm within these methodologies. Notably, 81.25% of studies relied on internal validation, primarily using K-fold cross-validation and train/test split strategies.
Conclusion: The findings underscore the significant potential of AI-based algorithms in enhancing the accuracy of BC survival predictions. However, to ensure the robustness and generalizability of these predictive models, future research should emphasize the importance of rigorous external validation. Such endeavors will not only validate the efficacy of these models across diverse populations but also pave the way for their integration into clinical practice, ultimately contributing to personalized patient care and improved survival outcomes.
Systematic Review Registration: https://www.crd.york.ac.uk/prospero/, identifier CRD42024513350.
1 Introduction
Breast cancer (BC) remains the most prevalent cancer and the leading cause of cancer-related mortality in women globally (1). This significant heterogeneity translates to substantial variations in individual patient survival following a BC diagnosis (2). Several factors influence BC prognosis, including patient demographics (e.g., age) (3, 4), tumor characteristics (e.g., size and lymph node involvement) (5), and tumor-derived biomarkers (e.g., hormone receptor status) (6, 7). Accurate survival prediction is crucial for understanding patient outcomes, guiding clinical decision-making, evaluating treatment efficacy, identifying prognostic factors, and developing personalized therapeutic strategies.
Artificial intelligence (AI), particularly machine learning (ML), has a pivotal role in data analysis, including medical data (8–11). These techniques offer promising avenues for enhancing BC survival prediction accuracy (12, 13). Survival analysis aims to model the time-to-event relationship, linking patient outcomes with associated variables (14, 15).
Researchers have developed various ML-based survival models to predict BC outcomes. Studies like (16) employed diverse ML classifiers, including multilayer perceptron (MLP), random forest (RF), decision tree (DT), and Support vector machine (SVM) to predict BC survival in a dataset of 4,902 patients. Additionally (17), explored deep learning (DL) approaches for predicting BC patient survival post-operatively.
Several literature reviews have emerged, discussing, and comparing outcomes of ML-derived prediction models in BC (18–22, 96). Some specifically focus on BC survival prediction, with (22) reviewing ML applications in predicting 5-year BC survival rates. Others, like (15), surveyed various ML and DL algorithms used in BC patient survival prediction. However, the rapid evolution of AI necessitates frequent review updates. Moreover, we are motivated to carry out this systematic review to synthesize and classify all AI algorithms used in BC survival prediction and present them in a structured form to help researchers select appropriate methodologies in upcoming research.
This systematic review aims to comprehensively explore the application of AI techniques in predicting BC survivability and examine the challenges associated with developing and refining these models. We will analyze all prediction models published from 2017 to 2023, evaluating their performance across diverse contexts. Through a broad analysis, we intend to investigate the advancements, challenges, and potential of AI-based BC survival prediction. Our review will encompass the spectrum of AI methods, data sources, and testing methodologies employed in prior research. Furthermore, we will critically evaluate the strengths and limitations of existing studies, with a particular focus on external validation and real-world performance assessment. By synthesizing these findings, we seek to illuminate the effectiveness, reliability, and clinical utility of ML-derived BC survival prediction models.
The remaining sections are structured as follows: Section 2 details the employed methodology, outlining eligibility criteria, reviewed databases, search strategy, study selection, and data extraction processes. Section 3 presents the review findings, encompassing the characteristics of reviewed studies aligned with conventional ML workflow steps (database selection, pre-processing, data augmentation, segmentation, feature extraction, dimensionality reduction, classification, and performance evaluation). Section 4 delves into a discussion of our findings, highlighting existing challenges and future research directions. Finally, the concluding section summarizes the key findings and their implications for clinical practice and further research.
2 Materials and methods
This systematic review and meta-analysis were conducted following the Preferred Reporting Items for Systematic Reviews and Meta-Analyses (PRISMA) guidelines (23), and registered in the International Prospective Register of Systematic Reviews (PROSPERO) (24) under registration number CRD42024513350.
2.1 Eligibility criteria
The selection of studies for this systematic review and meta-analysis was based on specific inclusion and exclusion criteria outlined in Table 1.
2.2 Information sources and search strategy
A systematic search for relevant studies was conducted across three electronic databases: Web of Science, PubMed, and Scopus, encompassing the period from January 2016 to August 2023. The search strategy, developed collaboratively by two authors (M.A. and ME.A.), incorporated three core concepts: “Breast Cancer,” “Survival Prediction,” and “Machine Learning,” along with their synonyms derived from MeSH terms and standardized vocabulary. English-language journal articles were exclusively included. Details of the search strategy are provided in Appendix A.
2.3 Study selection
We employed EndNote software to manage and deduplicate the retrieved articles. Titles and abstracts were then screened independently by four reviewers (Z.J., S.Z., A.O., and S.R.) to assess eligibility based on predefined criteria. Disagreements were resolved by the other two reviewers (K.S. and M.R.). Full texts of potentially eligible studies were subsequently assessed for final inclusion.
2.3.1 Quality assessment
The methodological quality of eligible studies was evaluated using the Qiao Quality Assessment tool (25). This tool encompasses five key domains: unmet need, reproducibility, robustness, generalizability, and clinical significance, further delineated by nine specific items. Studies were assessed independently by reviewers using a binary response format (‘Yes’ or ‘No’) for each item in a pre-defined table. Scores were assigned (‘1’ for ‘Yes’, ‘0’ for ‘No’), and the sum for each study determined its overall methodological quality. A pre-established threshold of 5 or more points (detailed in Appendix B) categorized studies as high-quality for further inclusion in the meta-analysis.
2.4 Data extraction
Following quality assessment, data were independently extracted from all high-quality studies using a pre-defined data extraction form created in Google Sheets. This form captured key characteristics of the included studies, including publication year, data availability, modality, number of datasets/samples, training data details (attributes, features, pre-processing, augmentation), feature selection and classification methods, model presentation, ML generation, paradigm and algorithms, time window, validation strategy, method and metrics, and best-achieved validation accuracy. Disagreements arising during extraction were resolved through discussion among all researchers.
2.5 Meta-analysis
In this systematic review, we extracted the best accuracy of ML models presented in studies. Then, we averaged the reported accuracies of each particular methodology to gain a holistic insight. This synchronization approach was carried out to identify the overall performance of methodologies and ease the comparison of different categories in various conditions.
3 Results
3.1 Search results
A systematic search of three databases yielded 140 articles. After deduplication (n=82), 58 studies underwent initial screening. Ultimately, 32 articles met the pre-defined inclusion criteria (see PRISMA diagram, Figure 1).
3.2 Data extraction
Data from the 32 included studies were systematically extracted using a pre-defined form. Extracted characteristics are presented in tabular format within Appendix C.
3.3 Characteristics of the included studies
As shown in Table 2, a marked increase in studies utilizing ML algorithms for BC survival prediction and classification is evident. The majority of included studies (n=26, 81.3%) were published between 2019 and 2023.
3.4 Machine learning methods
3.4.1 ML generation
Established machine learning methods categorized as “Traditional”, prioritize well-understood algorithms with strong theoretical underpinnings and interpretable results (e.g., K-nearest neighbors). “Modern” approaches leverage deep neural networks inspired by the brain’s structure and function (e.g., convolutional neural networks), alongside nature-inspired metaheuristic algorithms. “Hybrid” techniques strategically combine these approaches to capitalize on their complementary strengths. The integration of ML and DL applications in medical decision-making has witnessed significant growth in recent years, often complementing traditional methods (26–30). Modern ML leverages automated, integrated algorithms, particularly multi-layer neural networks, offering a unified learning framework that addresses the limitations of traditional approaches by eliminating the need for separate feature extraction and classification stages (31). Common modern techniques include Convolutional Neural Networks (CNNs), Deep Neural Networks (DNNs), Long Short-Term Memory (LSTMs), Recurrent Neural Networks (RNNs), ensemble methods like Random Forests, and XGBoost.
Traditional ML algorithms typically rely on a sequential approach with distinct stages for feature extraction and classification (32). Support Vector Machines (SVMs), K-Means Clustering, Artificial Neural Networks (ANNs), Decision Trees (DTs), AdaBoost, and Naive Bayes (NB) are commonly employed traditional algorithms.
This review analyzed the distribution of ML algorithm generations used for BC survival prediction. Modern techniques were employed in 21.87% (7 studies) of the included studies with an average accuracy of 88.72%. Traditional techniques were used in 37.5% (12 studies) with an average accuracy of 87.23%, while hybrid methods (combining modern and traditional approaches) were used in 40.62% (13 studies) with an average accuracy of 91.73%. Figure 2 depicts the chronological trend of ML generation usage in BC survival prediction studies across the eight years examined.
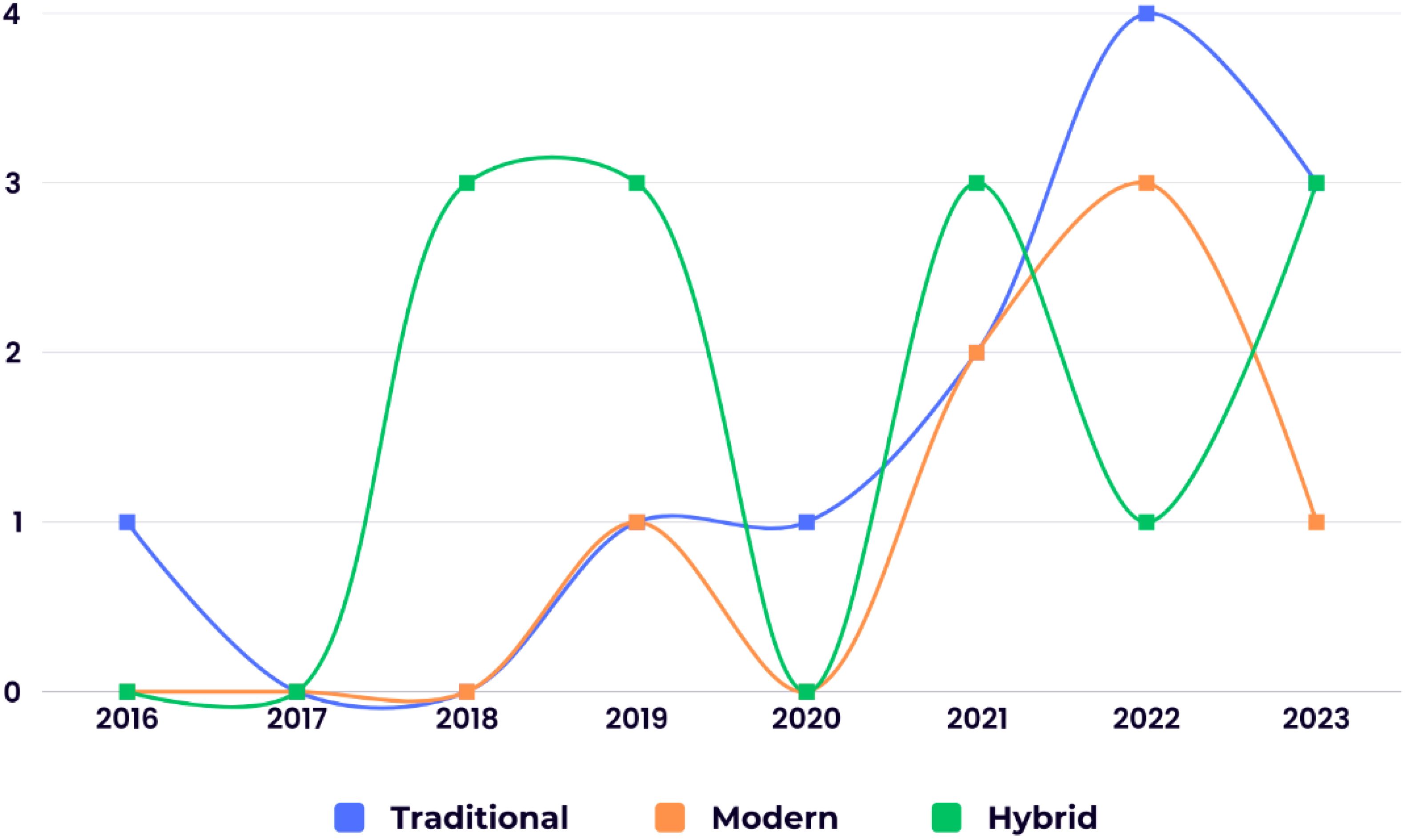
Figure 2. The chronological distribution of the studies utilizing different ML generations in BC survival prediction.
3.4.2 ML paradigm
ML encompasses four primary learning paradigms: supervised, unsupervised, semi-supervised, and reinforcement learning (33). Supervised learning utilizes labeled data for training, encompassing popular algorithms like DT and SVM. Conversely, unsupervised learning trains models using unlabeled data (34, 35). Semi-supervised learning leverages both labeled and unlabeled data, while reinforcement learning focuses on adapting to an environment through trial and error (33, 36).
This review examined the distribution of ML paradigms for BC survival prediction. Supervised learning emerged as the dominant paradigm, employed in 75% (n=24) of studies with an average accuracy of 88.82%. The combination of supervised and unsupervised learning was the next most frequent (25%, n=8), achieving an average accuracy of 92.07%. Notably, none of the studies utilized semi-supervised, reinforcement, or unsupervised learning alone (Figure 3).
3.5 Datasets
The reviewed studies employed a variety of BC datasets.
3.5.1 Data availability and analysis
Private datasets were the predominant choice, utilized in over half of the studies (56.25%, n=18, acc=89.42%). Notably, the use of private datasets has increased since 2022. Publicly available datasets, including established resources like The Cancer Genome Atlas (TCGA) (37), Molecular Taxonomy of Breast Cancer International Consortium (METABRIC) (38), and Surveillance, Epidemiology, and End Results (SEER) (39), were used in a smaller proportion of studies (34.37%, n=11, acc=89.80%). The characteristics of publicly available datasets are detailed in Table 3. A small subset of studies (9.37%, n=3, acc=93.1%) employed a combination of both public and private data.
The number of datasets per study ranged from 1 to 4. The majority of studies (75%, n=24, acc=88.52%) relied on a single dataset. Among these, METABRIC (n=4, 12.5%, acc=89.8%), SEER (n=4, 12.5%, acc=87.58%), and TCGA (n=3, 9.38%, acc=93.1%) were the most frequently used. Other, less common datasets included WBC (40) (n=2, 6.25%, acc=98.62%), WDBC (41) (n=1, 3.13%, acc=99.04%), WPBC (42) (n=1, 3.13%, acc=99.04%), MM-Dataset (43) (n=1, 3.13%, acc=99.04%), and TCIA (44) (n=1, 3.13%, acc=81%). Furthermore, 32.11% of studies (n=7, acc=96.57%) incorporated two datasets, while one study utilized four datasets.
3.5.2 Training data partitioning
The reviewed studies employed various training data partitioning strategies. The majority (93.75%, n=30, acc=89.34%) utilized a single dataset for training, potentially encompassing it for both training and testing phases. A smaller subset (6.25%, n=2, acc=99.04%) employed multiple datasets specifically for training.
3.5.3 Sample size and data modality
The datasets used in the studies exhibited significant variation in sample size. Values ranged from 38 to 163,413 cases per dataset, with an average of 12,041 cases. However, two studies lacked information regarding sample size. Additionally, the studies incorporated diverse data modalities, as illustrated in Figure 4.
3.5.4 Time window analysis
The reviewed studies employed various time windows to evaluate prediction accuracy. The 5-year window emerged as the most frequently explored timeframe, with 12 studies (37.5%) reporting an average accuracy of 86.81%. Notably, accuracy rates appeared to peak at the 10-year window, with 5 studies (15.625%) achieving an impressive 97.95% average accuracy. Conversely, shorter windows (1 and 3 years) yielded lower accuracy rates, with only 1 study (3.125%) in each achieving accuracy above 90%. Time windows exceeding 10 years also resulted in an average accuracy of 89.23% across 5 studies (15.625%). These findings suggest a potential trend of increasing accuracy with longer prediction horizons, although further investigation is warranted.
3.6 Features
The studies incorporated a variety of BC features for model development. The number of features employed ranged from 5 to 625. However, four studies (12.5%, acc=94.02%) did not report the specific features utilized in their ML models.
Several studies incorporated clinicopathological features directly related to BC, including age, cancer stage, grade, tumor size, estrogen receptor (ER) status, progesterone receptor (PR) status, and human epidermal growth factor receptor 2 (HER2) status. A good description of the predictors may be accessed in (45).
3.7 Methodology for breast cancer survival prediction
3.7.1 Pre-processing
Pre-processing is a critical step that transforms raw data into a format suitable for ML algorithms, ultimately enhancing model performance and accuracy (46, 47). Common techniques include data normalization (e.g., standardization, rescaling), augmentation, segmentation, and feature selection. Pre-processing also improves data quality by handling missing values, reducing noise (48, 49), correcting anomalies, and ensuring compatibility with modeling algorithms (50, 51).
This review found that 78.125% (n=25, acc=89.54%) of studies acknowledged employing at least one pre-processing technique. Various standardization methods were utilized, including mean removal and unit variance scaling (52), Transformation and resampling techniques (14, 16, 53, 54), data integration (16), SMOTE for imbalanced data (55), outlier detection with boxplots (56), one-hot encoding for categorical data (57), segmentation (58), and min-max scaling (59, 60) were also reported.
For missing value imputation, some studies employed methods like DL and K-Nearest Neighbors (KNN) (61), R mice package Predictive mean matching (PMM) (62), multiple imputation (MI) (56), Missing value estimation (63), or manual imputation (16, 63–67). Notably, 7 studies (21.875%, acc=90.05%) did not explicitly mention their pre-processing techniques.
3.7.1.1 Data augmentation
Data augmentation is a critical pre-processing technique that mitigates the risk of overfitting in ML models by artificially expanding the training data (68–70). This is achieved through methods like generating new data samples, modifying existing data (e.g., cropping, flipping, rotating), or both (71, 72). None of the reviewed studies utilized data augmentation techniques, so the effect of incorporating this method needs further investigation.
3.7.1.2 Feature selection and dimensionality reduction
Dimensionality reduction is an essential step that improves ML model performance by selecting relevant features and eliminating redundant ones (73, 74). Common feature selection techniques include Principal Component Analysis (PCA), Probabilistic PCA (PPCA), and Linear Discriminant Analysis (LDA). This review found that most studies (n=22, 68.75%, acc=89.08%) employed a combination of these methods, while a smaller portion (n=10, 31.25%, acc=91.11%) did not utilize any feature selection technique (Figure 5).
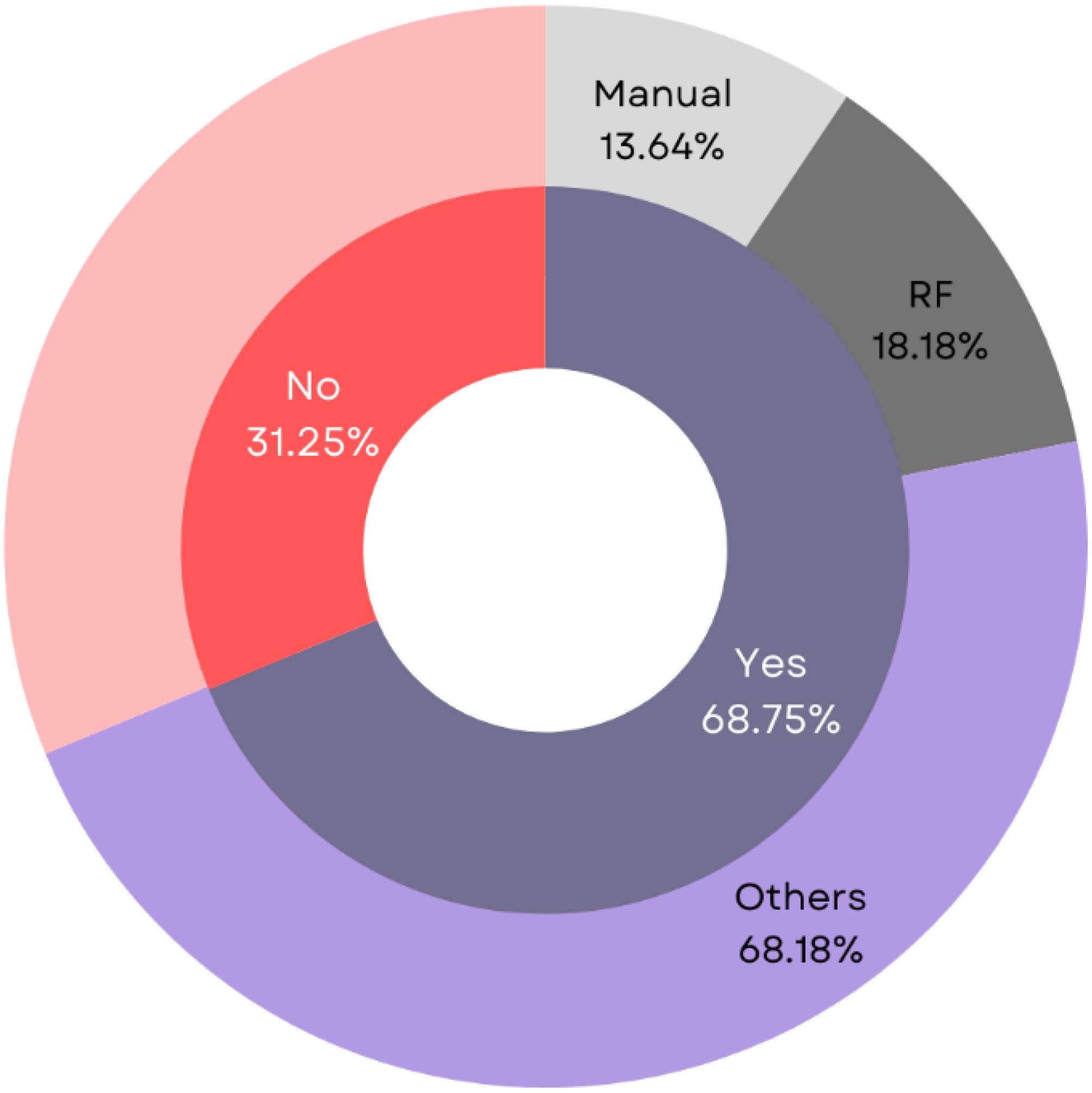
Figure 5. Analysis of different dimensionality reduction techniques employed for BC survival prediction.
Within the studies that employed feature selection and dimensionality reduction, supervised methods were the dominant choice (46.875%, n=15, acc=87.88%). Unsupervised techniques were implemented in a smaller subset of studies (21.875%, n=7, acc=91.28%). Notably, only one study (31.25%, acc=99.04%) utilized a combination of both approaches (75).
The following sections categorize and detail the various feature selection techniques reported in the reviewed studies, differentiating between traditional and modern methods.
3.7.1.2.1 Traditional feature selection techniques
This review identified traditional methods as the feature selection approach of choice in 31.25% (n=10, acc=89.24%) of the studies. Commonly employed techniques included manual selection (n=3, 9.38%, acc=89.70%), K-means clustering (n=2, 6.25%, acc=82.55%), PCA (n=2, 6.25%, acc=96.68%), minimum Redundancy Maximum Relevance (mRMR) (n=2, 6.25%, acc=94.60%), and Recursive Feature Elimination (RFE) (n=2, 6.25%, acc=99.04%).
For instance, studies like (66) employed manual selection, where researchers identified and compared features to determine the most significant ones. Similarly (17), incorporated medical expertise for feature selection.
• Supervised Traditional Feature Selection Methods
Approximately 15.63% (n=5, acc=90.13%) of the studies utilized supervised traditional techniques, including K-means clustering (76), and RFE (75, 77). Other reported methods were Variable Sensitivity Ratio (VSR) (65) and Backward Elimination (BE) (54).
• Unsupervised Traditional Feature Selection Methods
Unsupervised traditional methods were implemented in 15.63% (n=5, acc=88.58%) of the reviewed studies. These techniques included PCA (78, 79), mRMR (59, 63), and LightGBM (80).
3.7.1.2.2 Modern feature selection techniques
Modern feature selection techniques, minimizing human intervention, were prevalent in the reviewed studies (n=12, 37.5%, acc=88.97%).
• Supervised Modern Feature Selection Methods
Supervised modern techniques were the dominant choice for feature selection within this category (n=10, acc=87.04%). The most frequently used methods were RF (n=4, 12.5%, acc=86.27%), CNNs (n=2, 6.25%, acc=91.92%), and SHAP (n=2, 6.25%, acc=90.00%).
• Unsupervised Modern Feature Selection Methods
Modern unsupervised techniques were employed by a smaller proportion of studies (n=2, acc=96.68%) for feature selection. PCA was the method of choice in both studies for identifying important features (53, 75).
3.7.2 Classification
Classification is a fundamental objective in many healthcare-oriented ML projects (81–83). In BC survival prediction, classification algorithms aim to distinguish between patients who succumb to the disease and those who survive. Various ML techniques are employed to achieve this goal.
This review found that supervised learning was the dominant approach for classification tasks in all reviewed studies (n=32, 100%). These studies achieved an average accuracy of 89.73% in classifying BC patient survival. Notably, two studies (6.25%, avg. accuracy=94.95%) explored the use of unsupervised classification algorithms alongside supervised techniques.
The following sections categorize and detail the various classification techniques identified in the reviewed studies, differentiating between traditional and modern methods.
3.7.2.1 Traditional classification techniques
Traditional classification algorithms were employed in 62.5% (n=20, acc=89.38%) of the reviewed studies. Notably, some studies implemented multiple classifiers. For example (56), used C5.0 and RIPPER to classify BC patient survival, and (66) employed both ANN and LR for BC survivability estimation.
• Supervised Traditional Classifiers
Supervised traditional methods were the dominant choice within the traditional category (n=20, 62.5%, acc=89.38%). The most frequent method was SVM (n=12, 37.5%, acc=89.24%). Other commonly used classifiers included DT (n=11, 34.37%, acc=88.74%), Neural Networks (n=10, 31.25%, acc=87.52%), NB (n=6, 18.75%, acc=96.20%), KNN (n=5, 15.625%, acc=94.51%), and AdaBoost (n=4, 12.5%, acc=93.0%).
• Unsupervised Traditional Classifiers
Only one study (3.125%, accuracy=93.1%) utilized unsupervised traditional classification. The authors employed K-means clustering for predicting survival curves in BC patients (14).
3.7.2.2 Modern classification techniques
Modern classification algorithms were adopted in over 87.5% of the studies (n=28, acc=90.45%), demonstrating a notable increase since late 2018. For instance (54), developed a WTTE-RNN model to determine BC recurrence probability, and (67) used CNNs to predict BC survival time windows.
• Supervised Modern Classifiers
Supervised methods were the prevalent choice for modern classification (n=28, acc=90.45%). Deep Neural Networks (DNNs) were frequently employed (n=12, 37.5%, acc=91.14%). Among DNNs, CNNs (n=6, 50%, acc=90.57%) were most common, followed by Dense Neural Networks (n=5, 41.67%, acc=91.89%), LSTM (n=2, 16.67%, acc=98.0%), and RNN (n=1, 8.33%, acc=91.0%). Bagging algorithms (n=16, 50%, acc=90.46%) and XGBoost (n=10, 31.25%, acc=90.77%) were also widely used.
• Unsupervised Modern Classifiers
The use of modern unsupervised classification algorithms was infrequent, with only one study (3.125%, accuracy=96.8%) employing Restricted Boltzmann Machines (RBM) for BC survivability prediction (17).
3.8 Performance evaluation analysis
This section analyzes the performance metrics and validation methods employed in the reviewed studies.
3.8.1 Evaluation metrics
Accuracy emerged as the dominant evaluation metric, utilized in 78.13% (n=25) of the studies. Consequently, it serves as the primary basis for performance comparison across the reviewed studies (details in the Validation Accuracy section).
3.8.2 Validation methods
Two primary validation approaches were identified: internal and external validation. Internal validation assesses model performance using the training data, while external validation employs a separate dataset, improving generalizability (25).
Analysis revealed that internal validation was the predominant approach (81.25%, n=26, acc=89.20%). A smaller portion of studies utilized external validation (6.25%, n=2, acc=89.55%), and four studies (12.5%, acc=93.4%) combined both methods (Figure 6).
Table 4 details the studies that employed external validation for performance evaluation.
3.8.3 Validation strategy
The reviewed studies employed various validation strategies. K-fold cross-validation emerged as the dominant approach (71.88%, n=23, acc=89.69%). The train/test split method followed in prevalence (21.88%, n=7, acc=90.73%). Notably, bootstrapping was the least frequently used strategy (3.13%, n=1, accuracy=87.1%). One study (3.13%, accuracy=84.42%) did not report the validation strategy (Figure 7).
3.8.4 Validation accuracy
To comprehensively assess the validation performance of the reviewed ML models, a statistical analysis of their accuracies was conducted. This analysis included minimum, maximum, average, median, variance, and standard deviation (Table 5).
Across the 25 studies reporting validation accuracy (78.13% of the total), model accuracy ranged from 72% to 99.04% (Table 5). The average accuracy was 89.73%, and the median accuracy was 91% (n=32). Figure 8 visually depicts the distribution of accuracy scores within the reviewed studies.
3.9 Model presentation
The reviewed studies employed various methods to present their models (Figure 9). Graphs or schematics were the most prevalent approach (n=11, 34.38%), followed by a combined presentation using graphs and formulas (n=7, 21.88%). Formula-only presentations were the least common (n=3, 9.38%). Notably, over a third of the studies (n=11, 34.38%) did not visually represent their models or utilize equations.
4 Discussion
This systematic review and meta-analysis investigated the application of artificial intelligence in breast cancer survival prediction research published between 2016 and 2023. The analysis of 32 eligible studies revealed several noteworthy findings.
A diverse range of ML methods were employed for BC survival prediction, with an overall mean validation accuracy of 89.91%. Traditional methods remained popular, used in 25 studies (78.1%) either solely or combined with modern techniques. Studies like (20) also reported the prevalence of traditional ML models in BC recurrence prediction. However, a recent surge in modern techniques, particularly deep learning, is evident. Studies like (87, 88) highlighted the growing use of deep learning algorithms in BC detection.
Private datasets (56.25%) were more commonly used than public datasets (34.38%) for BC survival prediction. This aligns with the findings of (20). However, some studies like (22, 87) emphasized the wide use of public datasets like METABRIC, SEER, and TCGA for BC diagnosis. Notably, reviewed studies utilizing multiple public datasets achieved the highest validation accuracies (e.g., 99.04% and 98.2% in (55, 75)). This suggests the potential benefit of standard and valuable data from public sources for enhancing ML model performance.
The number of training datasets significantly impacted the results. Studies using multiple datasets (n=8) achieved a superior mean validation accuracy (95.76%). This finding emphasizes the importance of employing diverse training data to mitigate challenges associated with limited or inappropriate datasets. Furthermore, because of the emergence of different BC data modalities in recent decades and their impact on the detection and prediction of BC (89, 90), we extracted this data from datasets used in studies as well. Review of included studies showed that no study, except (85), employed all BC data modalities to predict BC survival using AI-based methodologies. This issue strengthens the necessity of utilizing diverse data to gain more precise and trustful results.
The 5-year window was the most prevalent timeframe for BC survival prediction, aligning with the established threshold for assessing survival rates as reported in (22). However, studies with a 10-year window achieved the highest mean validation accuracy (97.95%) (e.g (31, 65)). This suggests a potential benefit from considering longer prediction horizons.
The number of features used in the reviewed studies ranged from 5 to 625. Similar reviews like (19, 20, 22) reported findings on features like age, tumor size, grade, and stage as crucial predictors in BC prognostic models.
Pre-processing was a common step, with most studies employing at least one technique. Data augmentation, a valuable method for managing unbalanced or limited data, was absent in all reviewed studies. Future research should consider data augmentation to strengthen prediction models by addressing restricted data issues, as suggested in (20).
Over 68% of the studies employed feature selection techniques, primarily modern supervised methods. However, 10 studies did not utilize any selection methods. Feature selection is recommended to improve model performance by eliminating irrelevant features, as suggested in (18, 88). However, studies like (22) suggest that powerful classification methods might handle high-dimensional datasets without requiring selection.
Both traditional and modern classification techniques were widely used, either individually or in combination. SVM emerged as the most popular traditional supervised method, aligning with findings in (20) for BC recurrence prediction. Additionally, studies like (22, 91) listed SVM among common BC prediction algorithms. Its popularity can be attributed to its generalization capabilities, excellent classification performance, and ability to handle high-dimensional data as discussed in (92).
Bagging algorithms, DNNs, and XGBoost were frequently used among modern methods. While (88) identified CNNs as the leading model for both binary and multiclass classification, similar to (87), the second most common algorithm reported in (22) was ANN. While the potential utility of advanced optimization techniques, including metaheuristic algorithms (93) game theory (94), and quantum computing (95), warrants investigation, none of the reviewed studies employed these approaches.
Traditional ML techniques such as DT, SVM, and LR are often more interpretable than DL models. This interpretability is vital in clinical environments where comprehending the decision-making process is essential for trust and validation. Traditional ML methods are also well-suited for smaller datasets, which are common in medical research due to the limited availability of patient data. Techniques like RF and SVM possess well-established theoretical foundations and are widely utilized, making them reliable choices for numerous applications.
However, traditional ML methods often necessitate extensive manual feature engineering, which can be labor-intensive and may not capture all pertinent patterns within the data. These methods may encounter difficulties with very large datasets or high-dimensional data, limiting their applicability in certain contemporary medical applications. While effective, traditional ML approaches may not achieve the same level of accuracy as DL methods, particularly in complex predictive tasks.
Deep learning methods, particularly CNNs and DNNs, have demonstrated superior performance in terms of accuracy for breast cancer survival prediction. DL models can autonomously extract relevant features from raw data, thereby reducing the necessity for manual feature engineering. This capability is particularly advantageous when dealing with complex data such as medical images. DL methods excel in handling large datasets and intricate data structures, rendering them suitable for applications involving high-dimensional data.
Nevertheless, DL models are often characterized as “black boxes” due to their lack of interpretability, which can pose a significant drawback in clinical settings where understanding the rationale behind predictions is crucial. DL methods typically require substantial amounts of labeled data for effective training, which can be a limitation in medical research where annotated data is scarce. Furthermore, training DL models is computationally intensive and demands considerable resources, which may not be accessible in all research contexts.
Hybrid models that integrate traditional ML and DL techniques leverage the strengths of both approaches. For example, they can employ DL for feature extraction and traditional ML for classification, resulting in enhanced performance and interpretability. These models can adapt to various types of data and often exhibit greater robustness in handling diverse clinical scenarios. However, hybrid models can be complex to design and implement, requiring expertise in both traditional ML and DL techniques. The integration of different model types can introduce challenges, particularly in ensuring that the strengths of each component are effectively harnessed.
The analysis highlights that although traditional ML and DL methodologies each have unique advantages and limitations, hybrid models offer a promising solution by integrating the strengths of both approaches. However, these models’ complexity and substantial resource demands are critical factors that must be considered. Future research should prioritize enhancing the interpretability and efficiency of DL models, alongside developing robust hybrid models that can be more effectively incorporated into clinical settings. By overcoming these challenges, the potential of artificial intelligence to enhance breast cancer survival prediction can be fully harnessed, leading to improved patient outcomes and more personalized treatment strategies.
Accuracy, due to its frequent use, was the primary metric for meta-analysis and performance comparison. Internal validation techniques were dominant, and employed in over 80% of the studies, with K-fold cross-validation being the most common approach. These findings align with other studies like (19, 20, 22). Notably, studies employing both internal and external validation achieved the highest mean validation accuracy.
Graphs or schematics were the most prevalent methods for visualizing BC survival prediction models (34.38%). Formulas, and their combination with graphs, were used in about 31% of the reviewed studies. This aligns with the findings of (22) who reported a combined formula and graph approach as a widely used presentation model.
The recent proliferation of AI techniques for classification tasks has undeniably led to an upsurge in publications exploring their implementation outcomes. Notably, while advanced analysis techniques are readily available, access to large, curated datasets reflecting real-world clinical data appears limited among researchers employing approaches discussed in this review. Among the momentous issues documented in our study, the appropriateness of dated datasets for the training and testing of traditional, modern, and hybrid ML algorithms was questionable. So that, replacing this data with new ones or mixing them with up-to-date data may alter outcomes. This issue should be considered and examined in forthcoming studies. Our meta-analysis suggests that recent hybrid approaches utilizing unsupervised modern algorithms, particularly when applied to image data, show promising potential. However, the heterogeneity observed in study designs across the included research limited the ability to definitively identify a single superior method. Future research with more homogenous datasets is warranted to validate these findings and further explore the capabilities of these emerging techniques in breast cancer survival prediction.
There is a discernible shift towards hybrid models that synergistically integrate traditional ML and DL techniques. These hybrid frameworks capitalize on the respective advantages of each approach, such as employing DL for sophisticated feature extraction and utilizing conventional ML for classification tasks, thereby enhancing both performance and interpretability. In particular, DL architectures, notably CNNs, are increasingly utilized for the automated extraction of features from intricate data types, such as medical imaging, which significantly diminishes the necessity for manual feature engineering. Combining diverse data modalities, including clinical, genomic, and imaging data, is becoming prevalent, offering a holistic understanding of breast cancer and augmenting predictive accuracy. There is a pronounced emphasis on the development of explainable AI models to mitigate the “black box” nature inherent in DL. Techniques such as SHAP and LIME are being investigated to enhance model interpretability. Furthermore, the adoption of transfer learning, wherein pre-trained models are fine-tuned on specific datasets, is gaining momentum. This strategy can substantially reduce the requisite data and computational resources for training.
Subsequent research endeavors should prioritize rigorous external validation to ascertain the robustness and generalizability of predictive models across heterogeneous populations. Conducting extensive prospective studies to validate the clinical efficacy of AI algorithms is imperative, as these investigations will facilitate the assessment of the real-world applicability of such models. Formulating strategies for the seamless integration of AI models into clinical workflows is crucial, encompassing the development of user-friendly interfaces and ensuring models function effectively in real-time clinical environments. Establishing ethical guidelines and regulatory frameworks is vital to guarantee the responsible deployment of AI in healthcare, addressing concerns related to data privacy, algorithmic bias, and transparency. Future research should also concentrate on devising cost-effective AI solutions that can be widely adopted, particularly in resource-constrained settings.
Implementing data augmentation techniques and fostering data sharing among research institutions can ameliorate the challenge of limited data availability. The development and incorporation of explainable AI techniques can enhance the interpretability of DL models, rendering them more acceptable in clinical practice. Leveraging cloud computing and federated learning can alleviate computational resource constraints by distributing the training process across multiple nodes. Emphasizing external validation using diverse and independent datasets can bolster the generalizability and robustness of AI models.
This study has some limitations. First, the search strategy was restricted to English articles, potentially excluding relevant non-English publications. Second, limited access to some full texts prevented their review.
This systematic review and meta-analysis provided valuable insights into the application of AI in BC survival prediction research. The findings highlighted the prevalence of traditional and modern ML methods, the importance of data quality and quantity, and the potential benefits of longer prediction horizons and feature selection techniques. Additionally, the review identified areas for improvement, such as the underutilization of data augmentation and the need for more robust validation strategies. Future research should address these limitations and strive to develop more generalizable and accurate ML models for BC survival prediction.
5 Conclusion
This systematic review and meta-analysis comprehensively analyzed the application of ML in BC survival prediction research. Our findings revealed the extensive use of diverse ML algorithms, achieving promising results. However, a critical limitation identified across studies was the predominant reliance on internal validation for performance evaluation. This restricts generalizability – a crucial factor for real-world clinical implementation. Future research should prioritize external validation on independent datasets and rigorous model benchmarking to enhance the applicability of ML models in BC survival prediction.
Furthermore, the analysis identified shortcomings in data utilization. Inappropriate datasets and a lack of essential pre-processing techniques, such as feature selection and data augmentation, were frequently observed. Addressing these issues through the appropriate selection and application of datasets and pre-processing methods in future studies is crucial for improving model performance and generalizability.
In conclusion, AI has emerged as a significant tool for BC survival prediction. However, further exploration and research are essential to fully understand the true impact and effectiveness of these methods. This study provides valuable insights into current research trends and methodological considerations. Researchers can leverage these findings to guide the design of future BC survival prediction projects and address the identified shortcomings of previous studies, ultimately propelling advancements in this domain.
Data availability statement
The original contributions presented in the study are included in the article/Supplementary Material. Further inquiries can be directed to the corresponding authors.
Author contributions
ZJ: Visualization, Writing – original draft, Writing – review & editing. SZS: Writing – original draft, Data curation. KS: Formal analysis, Resources, Validation, Writing – review & editing. AO: Writing – review & editing, Investigation, Software, Validation. SR: Software, Validation, Writing – review & editing, Formal analysis, Methodology. MR: Writing – review & editing, Data curation, Investigation, Visualization. MEA: Conceptualization, Project administration, Supervision, Writing – review & editing. MA: Data curation, Investigation, Software, Visualization, Writing – review & editing, Conceptualization, Formal analysis, Methodology, Project administration, Resources, Supervision, Validation, Writing – original draft.
Funding
The author(s) declare that no financial support was received for the research, authorship, and/or publication of this article.
Acknowledgments
During the preparation of this work the authors used Perplexity in order to check the grammar and improve readability. After using this tool, the authors reviewed and edited the content as needed and take full responsibility for the content of the publication.
Conflict of interest
The authors declare that the research was conducted in the absence of any commercial or financial relationships that could be construed as a potential conflict of interest.
Publisher’s note
All claims expressed in this article are solely those of the authors and do not necessarily represent those of their affiliated organizations, or those of the publisher, the editors and the reviewers. Any product that may be evaluated in this article, or claim that may be made by its manufacturer, is not guaranteed or endorsed by the publisher.
Supplementary material
The Supplementary Material for this article can be found online at: https://www.frontiersin.org/articles/10.3389/fonc.2024.1420328/full#supplementary-material
Abbreviations
AI, Artificial Intelligence; ANN, Artificial Neural Network; BC, Breast Cancer; BE, Backward Elimination; CNN, Convolutional Neural Network; DL, Deep Learning; DNN, Deep Neural Network; DT, Decision Tree; ER, Estrogen Receptor; HER2, Human Epidermal growth factor Receptor 2; KNN, K-Nearest Neighbors; LDA, Linear Discriminant Analysis; LSTM, Long Short-Term Memory; ML, Machine Learning; MLP, Multilayer Perceptron; mRMR, Minimum Redundancy Maximum Relevance; NB, Naive Bayes; PCA, Principal Component Analysis; PMM, Predictive Mean Matching; PR, Progesterone Receptor; RBM, Restricted Boltzmann Machine; RF, Random Forest; RFE, Recursive Feature Elimination; RNN, Recurrent Neural Network; SVM, Support Vector Machine; VSR, Variable Sensitivity Ratio.
References
1. Sung H, Ferlay J, Siegel RL, Laversanne M, Soerjomataram I, Jemal A, et al. Global Cancer Statistics 2020: GLOBOCAN Estimates of Incidence and Mortality Worldwide for 36 Cancers in 185 Countries. CA: A Cancer J Clin. (2021) 71(3):209–49. doi: 10.3322/caac.21660
2. Jubair S, Alkhateeb A, Tabl AA, Rueda L, Ngom A. A novel approach to identify subtype-specific network biomarkers of breast cancer survivability. Network Modeling Anal Health Inf Bioinf. (2020) 9(1):43. doi: 10.1007/s13721-020-00249-4
3. Łukasiewicz S, Czeczelewski M, Forma A, Baj J, Sitarz R, Stanisławek A. Breast Cancer—Epidemiology, Risk Factors, Classification, Prognostic Markers, and Current Treatment Strategies—An Updated Review. Cancers. (2021) 13(17):4287.
4. Anbari F, Yazdani Kachooei Z, Salemi M, Anbari F. Anxiety and temporomandibular joint disorders among law students in Iran. J Dentomaxillofacial Radiology Pathol Surg. (2020) 9(4):33–9.
5. Liu Y, He M, Zuo W-J, Hao S, Wang Z-H, Shao Z-M. Tumor Size Still Impacts Prognosis in Breast Cancer With Extensive Nodal Involvement. Front Oncol. (2021) 11. doi: 10.3389/fonc.2021.585613
6. Cobleigh M, Yardley DA, Brufsky AM, Rugo HS, Swain SM, Kaufman PA, et al. Baseline Characteristics, Treatment Patterns, and Outcomes in Patients with HER2-Positive Metastatic Breast Cancer by Hormone Receptor Status from SystHERs. Clin Cancer Res. (2020) 26(5):1105–13. doi: 10.1158/1078-0432.CCR-19-2350
7. Kiarashi M, Bayat H, Shahrtash SA, Etajuri EA, Khah MM, Al-Shaheri NA, et al. Mesenchymal Stem Cell-based Scaffolds in Regenerative Medicine of Dental Diseases. Stem Cell Rev Rep. (2024) p:1–34. doi: 10.1007/s12015-024-10687-6
8. Dastani M, Ehtesham H, Javanmard Z, Sabahi A, Bahador F. Identifying the Trends of Global Publications in Health Information Technology Using Text-mining Techniques. Shiraz E-Med J. (2022) 23(11):e123803. doi: 10.5812/semj-123803
9. Arefinia F, Aria M, Rabiei R, Hosseini A, Ghaemian A, Roshanpoor A. Non-invasive fractional flow reserve estimation using deep learning on intermediate left anterior descending coronary artery lesion angiography images. Sci Rep. (2024) 14(1):1818. doi: 10.1038/s41598-024-52360-5
10. Ghaderzadeh M, Aria M, Hosseini A, Asadi F, Bashash D, Abolghasemi H. A fast and efficient CNN model for B-ALL diagnosis and its subtypes classification using peripheral blood smear images. Int J Intelligent Syst. (2022) 37(8):5113–33. doi: 10.1002/int.22753
11. Bayani A, Asadi F, Hosseini A, Hatami B, Kavousi K, Aria M, et al. Performance of machine learning techniques on prediction of esophageal varices grades among patients with cirrhosis. Clin Chem Lab Med (CCLM). (2022) 60(12):1955–62. doi: 10.1515/cclm-2022-0623
12. Kourou K, Exarchos TP, Exarchos KP, Karamouzis MV, Fotiadis DI. Machine learning applications in cancer prognosis and prediction. Comput Struct Biotechnol J. (2015) 13:8–17. doi: 10.1016/j.csbj.2014.11.005
13. Gorgzadeh A, Hheidari A, Ghanbarikondori P, Arastonejad M, Goki TG, Aria M, et al. Investigating the Properties and Cytotoxicity of Cisplatin-Loaded Nano-Polybutylcyanoacrylate on Breast Cancer Cells. Asian Pacific J Cancer Biol. (2023) 8(4):345–50. doi: 10.31557/apjcb.2023.8.4.345-350
14. Maabreh RSA, Alazzam MB, AlGhamdi AS. Machine Learning Algorithms for Prediction of Survival Curves in Breast Cancer Patients. Appl Bionics Biomech. (2021) 2021:9338091. doi: 10.1155/2021/9338091
15. Deepa P, Gunavathi C. A systematic review on machine learning and deep learning techniques in cancer survival prediction. Prog Biophysics Mol Biol. (2022) 174:62–71. doi: 10.1016/j.pbiomolbio.2022.07.004
16. Kalafi EY, Nor NAM, Taib NA, Ganggayah MD, Town C, Dhillon SK. Machine Learning and Deep Learning Approaches in Breast Cancer Survival Prediction Using Clinical Data. Folia Biol (Praha). (2019) 65(5-6):212–20. doi: 10.14712/fb2019065050212
17. Gupta S, Gupta MK. A Comparative Analysis of Deep Learning Approaches for Predicting Breast Cancer Survivability. Arch Comput Methods Eng. (2022) 29(5):2959–75. doi: 10.1007/s11831-021-09679-3
18. Salod Z, Singh Y. A five-year (2015 to 2019) analysis of studies focused on breast cancer prediction using machine learning: A systematic review and bibliometric analysis. J Public Health Res. (2020) 9(1):1792. doi: 10.4081/jphr.2020.1772
19. Phung MT, Tin Tin S, Elwood JM. Prognostic models for breast cancer: a systematic review. BMC Cancer. (2019) 19(1):230. doi: 10.1186/s12885-019-5442-6
20. Mazo C, Aura C, Rahman A, Gallagher WM, Mooney C. Application of Artificial Intelligence Techniques to Predict Risk of Recurrence of Breast Cancer: A Systematic Review. J Personalized Med. (2022) 12(9):1496. doi: 10.3390/jpm12091496
21. Kajala A, Jain VK. (2020). Diagnosis of Breast Cancer using Machine Learning Algorithms-A Review, in: 2020 International Conference on Emerging Trends in Communication, Control and Computing (ICONC3), Lakshmangarh, India, 2020, pp. 1–5. doi: 10.1109/ICONC345789.2020.9117320
22. Li J, Zhou Z, Dong J, Fu Y, Li Y, Luan Z, et al. Predicting breast cancer 5-year survival using machine learning: A systematic review. PloS One. (2021) 16(4):e0250370. doi: 10.1371/journal.pone.0250370
23. Page MJ, McKenzie JE, Bossuyt PM, Boutron I, Hoffmann TC, Mulrow CD, et al. The PRISMA 2020 statement: An updated guideline for reporting systematic reviews. Int J Surg. (2021) 88:105906. doi: 10.1016/j.ijsu.2021.105906
24. Booth A, Clarke M, Ghersi D, Moher D, Petticrew M, Stewart L, et al. An international registry of systematic-review protocols. Lancet. (2011) 377(9760):108–9. doi: 10.1016/S0140-6736(10)60903-8
25. Qiao N. A systematic review on machine learning in sellar region diseases: quality and reporting items. Endocrine Connections. (2019) 8(7):952–60. doi: 10.1530/EC-19-0156
26. Habehh H, Gohel S. Machine Learning in Healthcare. Curr Genomics. (2021) 22(4):291–300. doi: 10.2174/1389202922666210705124359
27. Farhad A, Reza R, Azamossadat H, Ali G, Arash R, Mehrad A, et al. Artificial intelligence in estimating fractional flow reserve: a systematic literature review of techniques. BMC Cardiovasc Disord. (2023) 23(1):407. doi: 10.1186/s12872-023-03447-w
28. Aria M, Nourani E, Golzari Oskouei A. ADA-COVID: Adversarial Deep Domain Adaptation-Based Diagnosis of COVID-19 from Lung CT Scans Using Triplet Embeddings. Comput Intell Neurosci. (2022) 2022:2564022. doi: 10.1155/2022/2564022
29. Bayani A, Hosseini A, Asadi F, Hatami B, Kavousi K, Aria M, et al. Identifying predictors of varices grading in patients with cirrhosis using ensemble learning. Clin Chem Lab Med (CCLM). (2022) 60(12):1938–45. doi: 10.1515/cclm-2022-0508
30. Ghaderzadeh M, Asadi F, Jafari R, Bashash D, Abolghasemi H, Aria M. Deep Convolutional Neural Network–Based Computer-Aided Detection System for COVID-19 Using Multiple Lung Scans: Design and Implementation Study. J Med Internet Res. (2021) 23(4):e27468. doi: 10.2196/27468
31. Othman NA, Abdel-Fattah MA, Ali AT. A Hybrid Deep Learning Framework with Decision-Level Fusion for Breast Cancer Survival Prediction. Big Data Cogn Computing. (2023) 7(1):50. doi: 10.3390/bdcc7010050
32. Atban F, Ekinci E, Garip Z. Traditional machine learning algorithms for breast cancer image classification with optimized deep features. Biomed Signal Process Control. (2023) 81:104534. doi: 10.1016/j.bspc.2022.104534
33. Al-Azzam N, Shatnawi I. Comparing supervised and semi-supervised Machine Learning Models on Diagnosing Breast Cancer. Ann Med Surg. (2021) 62:53–64. doi: 10.1016/j.amsu.2020.12.043
34. Sindhu Meena K, Suriya S. A Survey on Supervised and Unsupervised Learning Techniques. In: Kumar L, Jayashree L, Manimegalai R. (eds) Proceedings of International Conference on Artificial Intelligence, Smart Grid and Smart City Applications. AISGSC 2019. Springer, Cham. (2020). doi: 10.1007/978-3-030-24051-6_58
35. Omidi A, Mohammadshahi A, Gianchandani N, King R, Leijser L, Souza R. editors. (2024). Unsupervised domain adaptation of MRI skull-stripping trained on adult data to newborns. Proceedings of the IEEE/CVF Winter Conference on Applications of Computer Vision.
36. Eckardt J-N, Wendt K, Bornhäuser M, Middeke JM. Reinforcement Learning for Precision Oncology. Cancers. (2021) 13(18):4624. doi: 10.3390/cancers13184624
37. The Cancer Genome Atlas Program (TCGA) . Available online at: https://www.cancer.gov/ccg/research/genome-sequencing/tcga (Accessed August 15, 2023).
38. Curtis C, Shah SP, Chin SF, Turashvili G, Rueda OM. The genomic and transcriptomic architecture of 2,000 breast tumours reveals novel subgroups. Nature. (2012) 486(7403):346–52. doi: 10.1038/nature10983
39. The Surveillance, Epidemiology, and End Results (SEER) Program . Available online at: https://seer.cancer.gov/ (Accessed August 15, 2023).
40. Wolberg WM, Olvi N. Street and W. Street, Breast Cancer Wisconsin (Original). UCI Machine Learning Repository (1992).
41. Wolberg W, Mangasarian O, Street N, Street W. Breast Cancer Wisconsin (Diagnostic). UCI Machine Learning Repository (1995).
42. Wolberg W, Mangasarian O, Street N, Street W. Breast Cancer Wisconsin (Prognostic). UCI Machine Learning Repository (1995).
44. Clark K, Vendt B, Smith K, Freymann J, Kirby J, Koppel P, et al. The Cancer Imaging Archive (TCIA): maintaining and operating a public information repository. J Digit Imaging. (2013) 26(6):1045–57. doi: 10.1007/s10278-013-9622-7
45. Tarighati E, Keivan H, Mahani H. A review of prognostic and predictive biomarkers in breast cancer. Clin Exp Med. (2023) 23(1):1–16.
46. Kolivand P, Saberian P, Tanhapour M, Karimi F, Kalhori SRN, Javanmard Z, et al. A systematic review of Earthquake Early Warning (EEW) systems based on Artificial Intelligence. Earth Sci Inf. (2024), 1–28. doi: 10.1007/s12145-024-01253-2
47. Aria M, Hashemzadeh M, Farajzadeh N. QDL-CMFD: a Quality-independent and Deep Learning-based Copy-Move image Forgery Detection method. Neurocomputing. (2022) 511:213–36. doi: 10.1016/j.neucom.2022.09.017
48. Rajabi M, Golshan H, Hasanzadeh RP. Non-local adaptive hysteresis despeckling approach for medical ultrasound images. Biomed Signal Process Control. (2023) 85:105042. doi: 10.1016/j.bspc.2023.105042
49. Rajabi M, Hasanzadeh RP. A Modified adaptive hysteresis smoothing approach for image denoising based on spatial domain redundancy. Sens Imaging. (2021) 22(1):42. doi: 10.1007/s11220-021-00364-0
50. Aria M, Ghaderzadeh M, Asadi F, Jafari R. COVID-19 Lung CT Scans: A large dataset of lung CT scans for COVID-19 (SARS-CoV-2) detection. Kaggle (2021).
51. Arefanjazi H, Ataei M, Ekramian M, Montazeri A. A robust distributed observer design for Lipschitz nonlinear systems with time-varying switching topology. J Franklin Institute. (2023) 360(14):10728–44. doi: 10.1016/j.jfranklin.2023.07.036
52. Mihaylov I, Nisheva M, Vassilev D. Application of machine learning models for survival prognosis in breast cancer studies. Inf (Switzerland). (2019) 10(3). doi: 10.3390/info10030093
53. Chang CC, Chen SH. Developing a Novel Machine Learning-Based Classification Scheme for Predicting SPCs in Breast Cancer Survivors. Front Genet. (2019) 10. doi: 10.3389/fgene.2019.00848
54. Kim JY, Lee YS, Yu J, Park Y, Lee SK, Lee M, et al. Deep Learning-Based Prediction Model for Breast Cancer Recurrence Using Adjuvant Breast Cancer Cohort in Tertiary Cancer Center Registry. Front Oncol. (2021) 11:596364. doi: 10.3389/fonc.2021.596364
55. Mahesh TR, Kumar VV, Muthukumaran V, Shashikala HK, Swapna B, Guluwadi S. Performance Analysis of XGBoost Ensemble Methods for Survivability with the Classification of Breast Cancer. J Sensors. (2022) 2022. doi: 10.1155/2022/4649510
56. Lotfnezhad Afshar H, Jabbari N, Khalkhali HR, Esnaashari O. Prediction of Breast Cancer Survival by Machine Learning Methods: An Application of Multiple Imputation. Iranian J Public Health. (2021) 50(6):598–605. doi: 10.18502/ijph.v50i3.5606
57. Shahraki SZ, Looha MA, Kazaj PM, Aria M, Akbari A, Emami H, et al. Time-related survival prediction in molecular subtypes of breast cancer using time-to-event deep-learning-based models. Front Oncol. (2023) 13.
58. Dammu H, Ren TM, Duong TQ. Deep learning prediction of pathological complete response, residual cancer burden, and progression-free survival in breast cancer patients. PloS One. (2023) 18(1). doi: 10.1371/journal.pone.0280148
59. Mustafa E, Jadoon EK, Khaliq-uz-Zaman S, Humayun MA, Maray M. An Ensembled Framework for Human Breast Cancer Survivability Prediction Using Deep Learning. Diagnostics. (2023) 13(10). doi: 10.3390/diagnostics13101688
60. Jang W, Jeong C, Kwon K, Yoon TI, Yi O, Kim KW, et al. Artificial intelligence for predicting five-year survival in stage IV metastatic breast cancer patients: A focus on sarcopenia and other host factors. Front Physiol. (2022) 13. doi: 10.3389/fphys.2022.977189
61. Moncada-Torres A, van Maaren MC, Hendriks MP, Siesling S, Geleijnse G. Explainable machine learning can outperform Cox regression predictions and provide insights in breast cancer survival. Sci Rep. (2021) 11(1). doi: 10.1038/s41598-021-86327-7
62. Li H, Liu RB, Long CM, Teng Y, Cheng L, Liu Y. Development and Validation of a New Multiparametric Random Survival Forest Predictive Model for Breast Cancer Recurrence with a Potential Benefit to Individual Outcomes. Cancer Manage Res. (2022) 14:909–23. doi: 10.2147/CMAR.S346871
63. Arya N, Saha S. Multi-modal advanced deep learning architectures for breast cancer survival prediction. Knowledge-Based Syst. (2021) 221. doi: 10.1016/j.knosys.2021.106965
64. Liu P, Fu B, Yang SX, Deng L, Zhong XR, Zheng H. Optimizing Survival Analysis of XGBoost for Ties to Predict Disease Progression of Breast Cancer. IEEE Trans Biomed Eng. (2021) 68(1):148–60. doi: 10.1109/TBME.10
65. Lou SJ, Hou MF, Chang HT, Lee HH, Chiu CC, Yeh SCJ, et al. Breast Cancer Surgery 10-Year Survival Prediction by Machine Learning: A Large Prospective Cohort Study. Biology-Basel. (2022) 11(1).
66. Mudunuru VR, Skrzypek LA. A Comparison of Artificial Neural Network and Decision Trees with Logistic Regression as Classification Models for Breast Cancer Survival. Int J Math Eng Manage Sci. (2020) 5(6):1170–90. doi: 10.33889/24557749
67. Salehi M, Razmara J, Lotfi S, Mahan F. A One-Dimensional Probabilistic Convolutional Neural Network for Prediction of Breast Cancer Survivability. Comput J. (2022) 65(10):2641–53.
68. Maharana K, Mondal S, Nemade B. A review: Data pre-processing and data augmentation techniques. Global Transitions Proc. (2022) 3(1):91–9. doi: 10.1016/j.gltp.2022.04.020
69. Zandi S, Luhan GA. (2023). Exploring User Interactions in AR/VR Interfaces: A Simulation-Based Study, in: 2023 International Conference on Electrical, Computer and Energy Technologies (ICECET), IEEE.
70. Aria M, Ghaderzadeh M, Bashash D, Abolghasemi H, Asadi F, Hosseini A. Acute lymphoblastic leukemia (ALL) image dataset. Kaggle (2021).
71. Kazerouni A, Heydarian A, Soltany M, Mohammadshahi A, Omidi A, Ebadollahi S, et al. An intelligent modular real-time vision-based system for environment perception. arXiv (2023).
72. Zandi S, Luhan G. Exploring Gaze Dynamics in Virtual Reality through Multiscale Entropy Analysis. Sensors. (2024) 24(6):1781. doi: 10.3390/s24061781
73. Xie H, Li J, Zhang Q, Wang Y. Comparison among dimensionality reduction techniques based on Random Projection for cancer classification. Comput Biol Chem. (2016) 65:165–72. doi: 10.1016/j.compbiolchem.2016.09.010
74. Mousavi A, Arefanjazi H, Sadeghi M, Ghahfarokhi AM, Beheshtinejad F, Masouleh MM. Comparison of feature extraction with PCA and LTP methods and investigating the effect of dimensionality reduction in the bat algorithm for face recognition. Int J Robotics Control Syst. (2023) 3(3):501–9. doi: 10.31763/ijrcs.v3i3.1057
75. El Rahman SA. Predicting breast cancer survivability based on machine learning and features selection algorithms: a comparative study. J Ambient Intell Humanized Computing. (2021) 12(8):8585–623. doi: 10.1007/s12652-020-02590-y
76. Zhao M, Tang YS, Kim H, Hasegawa K. Machine Learning With K-Means Dimensional Reduction for Predicting Survival Outcomes in Patients With Breast Cancer. Cancer Inf. (2018) 17. doi: 10.1177/1176935118810215
77. Tahmassebi A, Wengert GJ, Helbich TH, Bago-Horvath Z, Alaei S, Bartsch R, et al. Impact of Machine Learning With Multiparametric Magnetic Resonance Imaging of the Breast for Early Prediction of Response to Neoadjuvant Chemotherapy and Survival Outcomes in Breast Cancer Patients. Invest Radiol. (2019) 54(2):110–7. doi: 10.1097/RLI.0000000000000518
78. Dinčić M, Popović TB, Kojadinović M, Trbovich AM, Ilić AŽ. Morphological, fractal, and textural features for the blood cell classification: the case of acute myeloid leukemia. Eur Biophysics J. (2021) 50(8):1111–27. doi: 10.1007/s00249-021-01574-w
79. Das BK, Dutta HS. GFNB: Gini index–based Fuzzy Naive Bayes and blast cell segmentation for leukemia detection using multi-cell blood smear images. Med Biol Eng Computing. (2020) 58(11):2789–803. doi: 10.1007/s11517-020-02249-y
80. Huang KY, Zhang J, Yu YS, Lin YX, Song CG. The impact of chemotherapy and survival prediction by machine learning in early Elderly Triple Negative Breast Cancer (eTNBC): a population based study from the SEER database. BMC Geriatrics. (2022) 22(1). doi: 10.1186/s12877-022-02936-5
81. Al-Hashem MA, Alqudah AM, Qananwah Q. Performance Evaluation of Different Machine Learning Classification Algorithms for Disease Diagnosis. Int J E-Health Med Commun. (2021) 12(6):1–28. doi: 10.4018/IJEHMC.20211101.oa5
82. Borji A, Seifi A, Hejazi T. An efficient method for detection of Alzheimer’s disease using high-dimensional PET scan images. Intelligent Decision Technol. (2023). doi: 10.3233/IDT-220315
83. McFarlin BL, Villegas-Downs M, Mohammadi M, Han A, Simpson DG, O'Brien WD Jr. Enhanced identification of women at risk for preterm birth via quantitative ultrasound: a prospective cohort study. Am J Obstetrics Gynecology MFM. (2023), 101250.
84. Nguyen QTN, Nguyen PA, Wang CJ, Phuc PT, Lin RK, Hung CS, et al. Machine learning approaches for predicting 5-year breast cancer survival: A multicenter study. Cancer Sci. (2023). doi: 10.1111/cas.v114.10
85. Albusayli R, Graham JD, Pathmanathan N, Shaban M, Raza SEA, Minhas F, et al. Artificial intelligence-based digital scores of stromal tumour-infiltrating lymphocytes and tumour-associated stroma predict disease-specific survival in triple-negative breast cancer. J Pathol. (2023) 260(1):32–42. doi: 10.1002/path.v260.1
86. Ji LC, Zhang W, Zhong XG, Zhao TX, Sun XX, Zhu SB, et al. Osteoporosis, fracture and survival: Application of machine learning in breast cancer prediction models. Front Oncol. (2022) 12.
87. Nasser M, Yusof UK. Deep Learning Based Methods for Breast Cancer Diagnosis: A Systematic Review and Future Direction. Diagnostics. (2023) 13(1):161. doi: 10.3390/diagnostics13010161
88. Nassif AB, Talib MA, Nasir Q, Afadar Y, Elgendy O. Breast cancer detection using artificial intelligence techniques: A systematic literature review. Artif Intell Med. (2022) 127:102276. doi: 10.1016/j.artmed.2022.102276
89. Iranmakani S, Mortezazadeh T, Sajadian F, Ghaziani MF, Ghafari A, Khezerloo D, et al. A review of various modalities in breast imaging: technical aspects and clinical outcomes. Egyptian J Radiol Nucl Med. (2020) 51(1):57. doi: 10.1186/s43055-020-00175-5
90. Pang T, Wong JHD, Ng WL, Chan CS. Deep learning radiomics in breast cancer with different modalities: Overview and future. Expert Syst Appl. (2020) 158:113501. doi: 10.1016/j.eswa.2020.113501
91. Fatima N, Liu L, Hong S, Ahmed H. Prediction of Breast Cancer, Comparative Review of Machine Learning Techniques, and Their Analysis. IEEE Access. (2020) 8:150360–76. doi: 10.1109/Access.6287639
92. Abreu PH, Santos MS, Abreu MH, Andrade B, Silva DC. Predicting Breast Cancer Recurrence Using Machine Learning Techniques: A Systematic Review. ACM Comput Surv. (2016) 49(3):1–40.
93. Havaeji H, Dao T-M, Wong T. Optimizing a Transportation System Using Metaheuristics Approaches (EGD/GA/ACO): A Forest Vehicle Routing Case Study. World J Eng Technol. (2023) 12(1):141–57. doi: 10.4236/wjet.2024.121009
94. Zarreh M, Yaghoubi S, Bahrami H. Pricing of Drinking Water under Dynamic Supply and Demand based on Government Role: A Game-Theoretic Approach. Water Resour Manage. (2024), 1–33. doi: 10.1007/s11269-024-03745-8
95. Shavandi M, Taghavi A. Maps preserving n-tuple A* B– B* A derivations on factor von Neumann algebras. Publications l’Institut Mathematique. (2023) 113(127):131–40. doi: 10.2298/PIM2327131S
Keywords: breast cancer, survival prediction, machine learning, deep learning, clinical data, systematic review, meta-analysis
Citation: Javanmard Z, Zarean Shahraki S, Safari K, Omidi A, Raoufi S, Rajabi M, Akbari ME and Aria M (2025) Artificial intelligence in breast cancer survival prediction: a comprehensive systematic review and meta-analysis. Front. Oncol. 14:1420328. doi: 10.3389/fonc.2024.1420328
Received: 19 April 2024; Accepted: 10 December 2024;
Published: 07 January 2025.
Edited by:
Isabella Castellano, University of Turin, ItalyReviewed by:
Rashid Ibrahim Mehmood, Islamic University of Madinah, Saudi ArabiaDayakar Naik Lavadiya, University of Mary, United States
Copyright © 2025 Javanmard, Zarean Shahraki, Safari, Omidi, Raoufi, Rajabi, Akbari and Aria. This is an open-access article distributed under the terms of the Creative Commons Attribution License (CC BY). The use, distribution or reproduction in other forums is permitted, provided the original author(s) and the copyright owner(s) are credited and that the original publication in this journal is cited, in accordance with accepted academic practice. No use, distribution or reproduction is permitted which does not comply with these terms.
*Correspondence: Mehrad Aria, bWVocmFkLmFyaWFAb3V0bG9vay5jb20=; Mohammad Esmaeil Akbari, cHJvZm1lYWtiYXJpQGdtYWlsLmNvbQ==
†ORCID: Mohammad Esmaeil Akbari, https://orcid.org/0000-0002-9938-3587
Mehrad Aria, https://orcid.org/0000-0002-6027-6952