- 1Department of Radiology, Affiliated Hospital of Nanjing University of Chinese Medicine, Nanjing, China
- 2The First Clinical Medical College, Henan University of Chinese Medicine, Zhengzhou, China
- 3Science and Technology Department, Jiangsu Collaborative Innovation Center of Traditional Chinese Medicine Prevention and Treatment of Tumor, Nanjing University of Chinese Medicine, Nanjing, China
- 4College of Pharmacy, Nanjing University of Chinese Medicine, Nanjing, China
- 5Department of Oncology, Affiliated Hospital of Nanjing University of Chinese Medicine, Nanjing, China
Introduction: Xiaoai Jiedu recipe (XJR), a classical prescription of traditional Chinese medicine (TCM), has been clinically proven to be effective in ameliorating colorectal cancer (CRC). However, its exact mechanism of action is still elusive, limiting its clinical application and promotion to a certain extent. This study aims to evaluate the effect of XJR on CRC and further illustrate mechanism underlying its action.
Methods: We investigated the anti-tumor efficacy of XJR in vitro and vivo experiments. An integrated 16S rRNA gene sequencing and UPLC-MS based metabolomics approach were performed to explore possible mechanism of XJR anti-CRC on the gut microbiota and serum metabolic profiles. The correlation between altered gut microbiota and disturbed serum metabolites was investigated using Pearson’s correlation analysis.
Results: XJR effectively displayed anti-CRC effect both in vitro and in vivo. The abundance of aggressive bacteria such as Bacteroidetes, Bacteroides, and Prevotellaceae decreased, while the levels of beneficial bacteria increased (Firmicutes, Roseburia, and Actinobacteria). Metabolomics analysis identified 12 potential metabolic pathways and 50 serum metabolites with different abundances possibly affected by XJR. Correlation analysis showed that the relative abundance of aggressive bacteria was positively correlated with the levels of Arachidonic acid, Adrenic acid, 15(S)−HpETE, DL−Arginine, and Lysopc 18:2, which was different from the beneficial bacteria.
Discussion: The regulation of gut microbiota and related metabolites may be potential breakthrough point to elucidate the mechanism of XJR in the treatment of the CRC. The strategy employed would provide theoretical basis for clinical application of TCM.
Introduction
Colorectal cancer (CRC) is currently the second most commonly diagnosed malignancy in China, the incidence of CRC has increased by an average of 550,000 per year due to the complex interactions among genetic, environmental, economic development and lifestyle factors(1). Current the main treatments for CRC are surgery, radiotherapy and chemotherapy, but the therapeutic effect is not optimistic with high rate of recurrence and accompanied obvious side effects(2). Therefore, the prevention and treatment of CRC have attracted worldwide attention in the field of drug research and development, and the more effective, safer, and cheaper anti-tumor drugs need to be developed.
It is well known that cancer is a complex disease, which is generally caused by a combined action of multi-factors in nature (3). Therefore, monotherapy does not necessarily produce ideal efficacy for malignant tumors. With the characteristics of “multi-components” and “multi-targets”, Traditional Chinese Medicine (TCM) has been applied as the principle or auxiliary drugs in the treatment of various complex diseases for more than 2,500 years(4). TCM contributes to promoting homeostasis mainly in two ways: (1) it contains plentiful active ingredients, which usually provide beneficial synergy by acting on diverse biological targets; (2) most of them are natural herbs, sometimes even edible, so they with fewer side effects and low toxicities. Xiaoai Jiedu recipe (XJR) is a classical anti-tumor prescription proposed by Zhou Zhongying, a master of TCM, based on the cancer toxin theory and after his years of clinical practice experience(5). The formula is composed of 7 herbs, Hedyotis diffusa (20 g), Radix pseudostellariae (15 g), Akebia trifoliata Koidz (12 g), Radix ophiopogonis (12 g), Bombyx batryticatus (10 g), Cremastra appendiculata (10 g), and Centipede (3 g) in a specific proportion. Although its efficacy in CRC treatment has been confirmed by a number of clinical applications, its exact mechanism of action is still elusive, limiting its clinical application and promotion to a certain extent (6).
The microbiota is an integral part of the body composition, which is equivalent to a virtual organ and plays an important role in maintaining intestinal physiological functions and regulating immunity. As the location with the highest distribution density of microbiota, the imbalance of microbiota can lead to colorectal disorder (7). Recently, accumulating evidences have verified that intestinal flora plays an essential role in the occurrence and development of CRC (8, 9). Chen et al. found that C. butyricum, as a kind of butyric-producing bacteria commonly used in clinical treatment of gastrointestinal diseases, could play an anti-intestinal tumor role by modulating the gut microbiota composition, as demonstrated by increases in some beneficial bacteria and decreases in some pathogenic bacteria (10). Flemer et al. found that the microbiota profiles of CRC patients was differ from those of healthy subjects, the abundance of Bacteroidetes Cluster 1 and Firmicutes Cluster 1 in the intestinal mucosa of CRC patients decreased, while the abundance of Bacteroides cluster 2, Firmicutes cluster 2, Pathogen cluster and Prevotella cluster increased (11). TCM can play an anti-tumor role by regulating the intestinal flora structure (12, 13). In the previous study, we found that Wenzi Jiedu Recipe (WJR) could effectively inhibit the development of CRC by regulating the structure of gut microbiota via enriching Oscillibacter and Bacteroides_acidifacien (14).
Intestinal flora is also the main participant and regulator of host metabolism, so the metabolic process is not only regulated by gene, but also by symbiotic bacteria (15). Therefore, it is vital to evaluate the alterations of intestinal flora-related metabolic phenotype. The combination of microbiological technique and metabolomics provides an effective means for further study of the relationship between host gut microbiota and metabolism, and contributes to in-depth analysis of the molecular mechanism of drug action (16).
In the present study, we confirmed the anti-CRC effect of XJR in vitro and vivo. 16s rRNA sequencing analysis was conducted to evaluate the effect of XJR on intestinal flora regulation, and UPLC-Q-TOF/MS-based serum metabolomics analysis was performed to find specific metabolic biomarkers and metabolic pathways related to XJR anti-CRC. Moreover, correlation analysis of the specific gut microbiota profiles and serum metabolites was established to understand the potential anti-CRC mechanisms of XJR. We hope the obtained results would provide a reliable scientific basis for promoting the TCM in the clinical treatment of complex diseases.
Materials and methods
Preparation of Xiaoai Jiedu recipe
The herbal formula XJR is a combination of seven medicinal herbs: Hedyotis diffusa (20 g), Radix pseudostellariae (15 g), Akebia trifoliata Koidz (12 g), Radix ophiopogonis (12 g), Bombyx batryticatus (10 g), Cremastra appendiculata (10g), and Centipede (3 g). The seven different herbal ingredients that constitute XJR were purchased from Anhui Bozhou Traditional Chinese and Western Medicine Co., Ltd. (Anhui, China) and authenticated by two experienced pharmacists. XJR was decocted according to the standard method and concentrated to 2.0 g/ml, diluted with normal saline (v/v, 1:1), and stored at 4 °C.
Preparation of medicated serum
18 adult Sprague-Dawley (SD) rats weighing 220~250 g were randomly divided into three groups: control group (NC, normal saline), low-dose group (LD, XJR 5.0 g/kg), and high-dose group (HD, XJR 20.0 g/kg), and each group contained six animals. Rats were gavaged with the different doses of XJR or normal saline twice a day for 3 consecutive days. Within one hour after the last administration, we collected blood samples from rats through retinal vein plexus and obtained the medicated serum through centrifugation and filtration. The XJR-containing serum was stored at –80°C for standby.
Cell proliferation assays
MTT assay was used to determine cell viability in order to verify the anti-tumor effect of XJR in vitro. Briefly, colorectal adenocarcinoma DLD-1 cells were seeded in 96-well plates at 1×104/pore. After incubation for overnight at 37°C in 5% CO2 to allow cell attachment, different concentrations of drug serum were added to the wells and incubated for 48 h. After treatments, the cells were further incubated MTT (Sigma St. Louis, MO, USA) for 4 h. Thereafter, 150 µL/well of DMSO (Sigma St. Louis, MO, USA) was added to the plates. The optical density (OD) at a wavelength of 492 nm was measured using a multifunctional microplate reader (Thermo Scientific., Waltham, MA, USA).
Animal study
Male BALB/c mice (4-6 weeks of age, 18–20 g) were obtained from the Qinglongshan Experimental Animal Center (SCXK-2017-0007) and kept under SPF conditions. The mice were raised in accordance with the guideline for laboratory animals in the Declaration of Helsinki. This animal experiment was approved by the Ethics Committee of Nanjing University of Chinese Medicine (202204A058).
HCT116 cells were subcutaneously administered into the axillary region of the mice to establish the CRC tumor bearing mice model. Based on the conventional dosage of clinical human body and using the dosage conversion formula, we obtained the high (XJR 20.0 g/kg, HD) and low dosage (XJR 5.0 g/kg, LD) for mice(5). Therefore, when the tumors volume reached to 50 mm3, the mice were randomly divided into three groups, with six in each group: ① NC group, gavaged with equal volume of normal saline; ② LD group of XJR, gavaged with 5 g/kg XJR; ③ HD group of XJR, gavaged with 20 g/kg XJR. All mice were administrated once a day for 14 days with different concentrations of drug. The tumor volume was calculated as follows: length × width2 × 0.5, and tumor growth inhibition rate (TGI) was estimated as follows: (tumor volume in the NC group – tumor volume in the treated group)/tumor volume in the NC group ×100%.
Histopathology
Xenograft tumors of mice were harvested from each group, preserved in 4% paraformaldehyde, embedded in paraffin, and then cut into 4 μm thick tissue slices as described. After the sample slices were dehydrated with gradient ethanol, they were stained with hematoxylin and eosin (H&E). The sections were assessed by a pathologist using an optical microscope.
Gut microbiota analysis
After administrating with different agents, we collected the mice feces for gut microbiota analyses(14). The stool DNA was extracted using the E.Z.N.A. soil kit (Omega Bio-tek, Norcross, GA, U.S.) as per manufacturer instructions. The V3–V4 region of bacteria 16S rDNA gene was amplified by PCR (pre-denaturation at 95°C for 3min, followed by 27 cycles at 95°C for 30s, 55°C for 30s, and 72°C for 30s and a last extension at 72°C for 5min) using the primers 338 F (5’-barcode-ACTCCTACGGGAGGCAGCA-3’) and 806R (5’-GGACTACHVGGGTWTCTAAT-3’) to analyze the classification composition of bacterial community. The PCR product was purified with the AxyPrep DNA Gel Extraction Kit (Axygen Biosciences, Union City, CA, USA) and quantified with the Quanti FluorTM-ST (Promega, USA) according to the standard protocols. Sequencing was then performed on the Illumina MiSeq HiSeq platform (Illumina Inc., San Diego, CA). Operational taxonomic units (OTUs) were picked with a threshold of 97% sequence, and the identified taxonomy was aligned with the Silva database (Release 128). At a given taxonomic rank, 16S rRNA sequences was assigned using the RDP classifier (version 2.2).
Metabolomics analysis on serum
After therapy, whole blood was collected from the orbital vein plexus, centrifuged for 15 min at 15,000 rpm for 20 min, and the serum sample was obtained for UPLC-Q-TOF/MS analysis(17). The separation was performed on a Hypesil Gold column (100×2.1 mm, 1.9µm) at a flow rate of 0.2mL/min. The mobile phase consisted of 0.1% formic acid in water as solvent A and acetonitrile as solvent B, and the solvent gradient was set as follows: 0–3 min, 5%–45% B; 3–13 min, 40%–95% B; 13–14 min, 95% B; 14–15 min, 95%–5% B.
The scanning of mass spectrometry was performed for the positive and negative ionization modes, respectively. The optimal conditions were set as follows: Positive ion mode: ion source temperature, 100 °C; capillary voltage, 2.5 kV; cone voltage, 24 V; desolvation gas, 800 L/h, cone gas flow, 50 L/h. Negative ion mode: ion source temperature, 100 °C; capillary voltage, 2.5 kV; cone voltage, 25 V; desolvation gas, 600 L/h, cone gas flow, 10 L/h. The production MS scan range was from 50 to 1500 Da, and TOF-MS scanning range was from 100 to 2000 Da.
The UPLC-Q/TOF-MS original data were processed using the MarkViewTM software. Principal components analysis (PCA), orthogonal partial least squares-discriminant analysis (OPLS-DA), and Partial least squares discriminant analysis (PLS-DA) were performed at metaX to cluster the sample plots across different groups. The metabolites with variable importance (VIP) > 1 and p-value< 0.05 were selected as the differential metabolites. The HMDB Database (http://www.hmdb.ca/), METLIN Database (http://metlin.scripps.edu/), MassBank Database (http://www.massbank.jp/) and KEGG Database (http://www.genome.jp/kegg/) were utilized to identify the potential biomarkers and metabolic pathways.
Statistical analysis
Statistical significance was analyzed with SPSS 22.0 (SPSS Inc., Chicago, IL, USA). Quantitative data were presented as mean ± standard deviation (SD), and statistical significance was defined by P<0.05. The comparisons between two groups made using two-tailed unpaired Student’s t-tests, and the comparisons between multiple groups made using one-way analysis of variance (ANOVA). Pearson’s correlation coefficient was established to show the association between the altered gut microbiota and disturbed serum metabolites.
Results
Anti-tumor efficacy of XJR in vitro and vivo
MTT assay was utilized to assess the effect of XJR on proliferation ability of CRC cells. We found that XJR significantly suppressed DLD-1 cells proliferation compared with the NC group, and the inhibitory effect of low-dose of XJR was stronger than that of high-dose of XJR (Figure 1A).
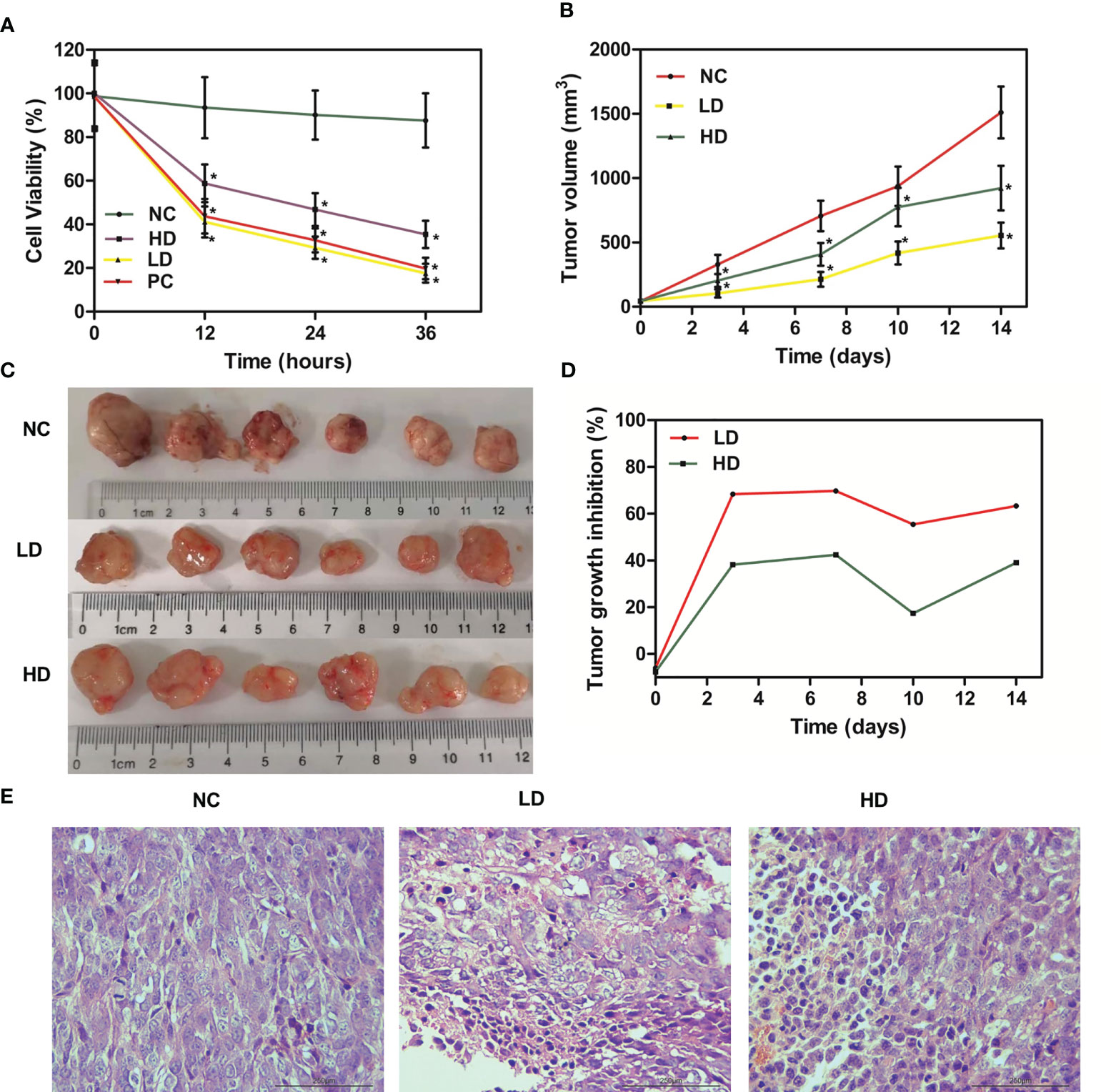
Figure 1 Anti-tumor effect of XJR in vitro and in vivo. (A) Cell viability was determined by MTT assay. (B) Tumor volume changes of tumor-bearing mice in different treatment groups. (C) Tumor photographs of different treatment groups after 14 days. (D) Tumor growth inhibition rate (TGI) of mice in different treatment groups. (E) H&E staining of tumor samples. NC, control group; LD, low-dose group of XJR; HD, high-dose group of XJR; PC, positive group; XJR, Xiaoai Jiedu Recipe. H&E, hematoxylin and eosin; TGI, tumor growth inhibition rate. *vs. NC, p < 0.05.
In this study, we also investigated the in vivo effects of XJR therapy on the growth of CRC with HCT116 tumor-bearing mice. Compared with the NC group, tumor progression was dramatically inhibited by LD therapy, and the HD group suppressed tumor growth to a certain degree. The relative tumor volume of LD and HD groups were smaller than that of the NC group, and the tumor volume of LD group was also smaller than that of HD group (Figures 1B, C). During the treatment phase, we also conducted the TGI score to evaluate the efficacy. The result showed that the TGI of the LD and HD groups were significant higher than that of the NC group, and the TGI of the LD group was more significant, consistent with the results of MTT and tumor volume (Figure 1D).
Meanwhile, we performed H&E staining on tumor samples to better demonstrate the anti-tumor effect of XJR in vivo. According to the histopathology changes, we found that there was moderate damage to the tumor tissue in the HD group, and the structural damage of the tumor tissue in the LD group was more apparent and extensive, such as irregular widening of the intercellular space and severe necrosis (Figure 1E). In conclusion, these results showed that XJR can inhibit tumor growth of CRC in vitro and vivo, and the LD therapy had the stronger inhibitory effect on tumor progression. Therefore, we chose the low dose of XJR for the following experiments.
Gut microbiota analysis
In order to explore whether the anti-tumor efficacy of XJR was associated with fecal microbiota, we analyzed the altered fecal microbiota composition profiles after XJR treatment by the 16S rDNA sequencing method. We assessed the phylogenetic composition of the intestinal flora in the NC group and XJR treatment group, and found the relative diversity of communities at the genus level in the different groups (Figure 2A). Principal coordinate analysis (PCoA) was preformed to compare the microbial community structure. PCoA analysis revealed the community composition of the NC group was far from that of the XJR treatment group, indicating that the flora composition had significant changes after XJR treatment (Figure 2B). Based on Chao, Observed, Shannon, and Simpson indices, there was no significant difference observed in the alpha diversity of the intestinal flora between the NC group and the XJR group (Figure 2C). Firmicutes and Bacteroidetes accounted for more than 90% at the phylum level (Figure 2D). Compared with the NC group, the proportion of Bacteroidetes in the XJR group decreased to 56.6%, and that of Firmicutes increased to 38.8%.
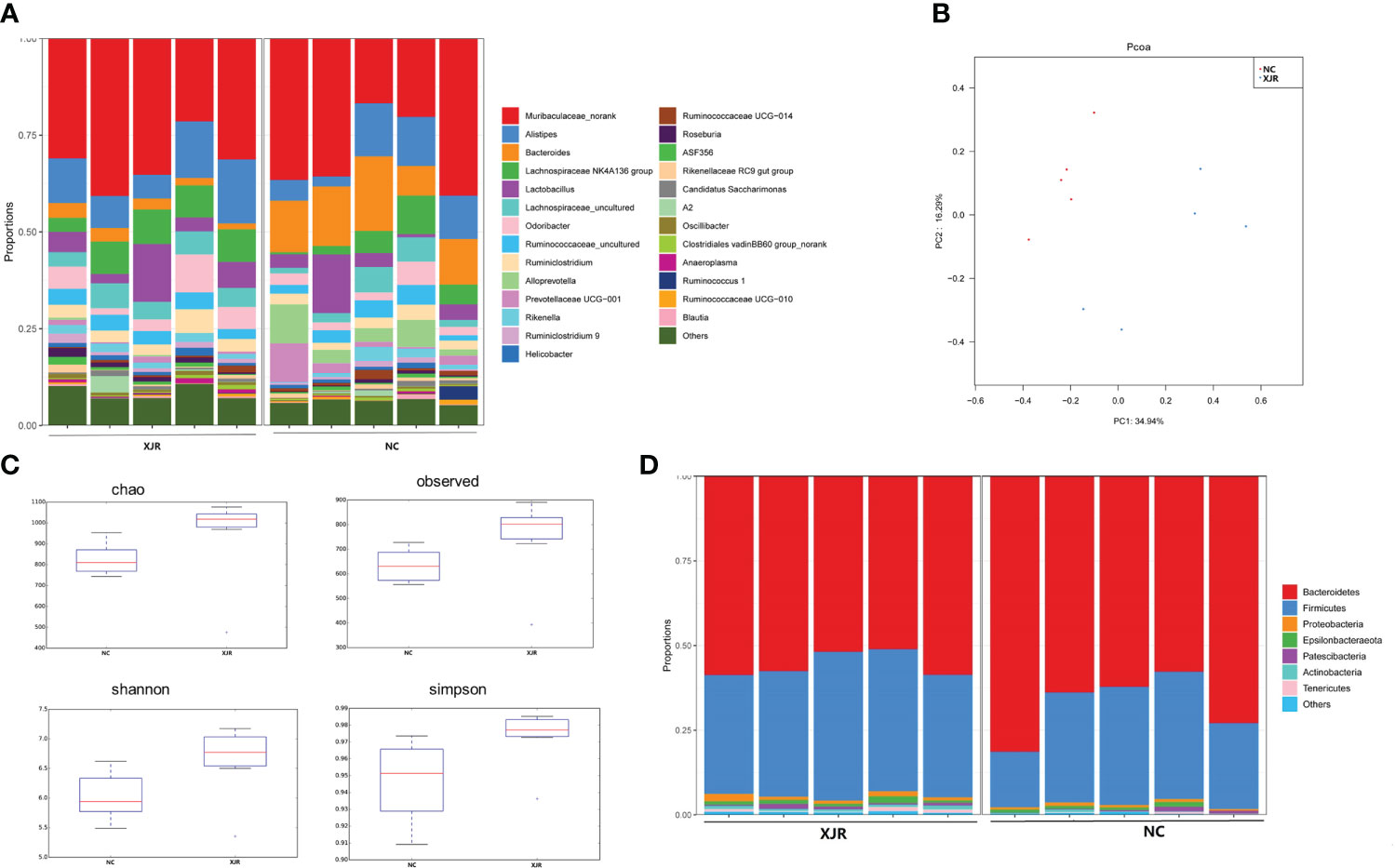
Figure 2 XJR modulated the gut microbiome composition. (A) Bar plot of the relative composition of gut microbiome at the genus level in the NC and XJR groups. (B) PCoA score plots in different groups. (C)Alpha diversity of the gut microbiome between the NC and XJR groups based on Chao, Observed, Shannon, and Simpson indices. (D) Relative gut microbiota abundance at the phylum level in the NC and XJR groups. NC, control group; XJR, Xiaoai Jiedu Recipe.
To further identify the dominant altered fecal microbiota composition profiles after XJR treatment, we performed the high-dimensional class comparison through linear discriminant analysis effect size (LEfSe) analysis. The results again demonstrated there were differences in the abundance of bacteria between the different groups, with Bacteroidales, Bacteroidia, Bacteroidetes, Bacteroides, and Prevotellaceae enriched in the NC group and Firmicutes, Roseburia, Actinobacteria, Enterobacter, and Deferribacteres enriched in the XJR group (Figures 3A, B).
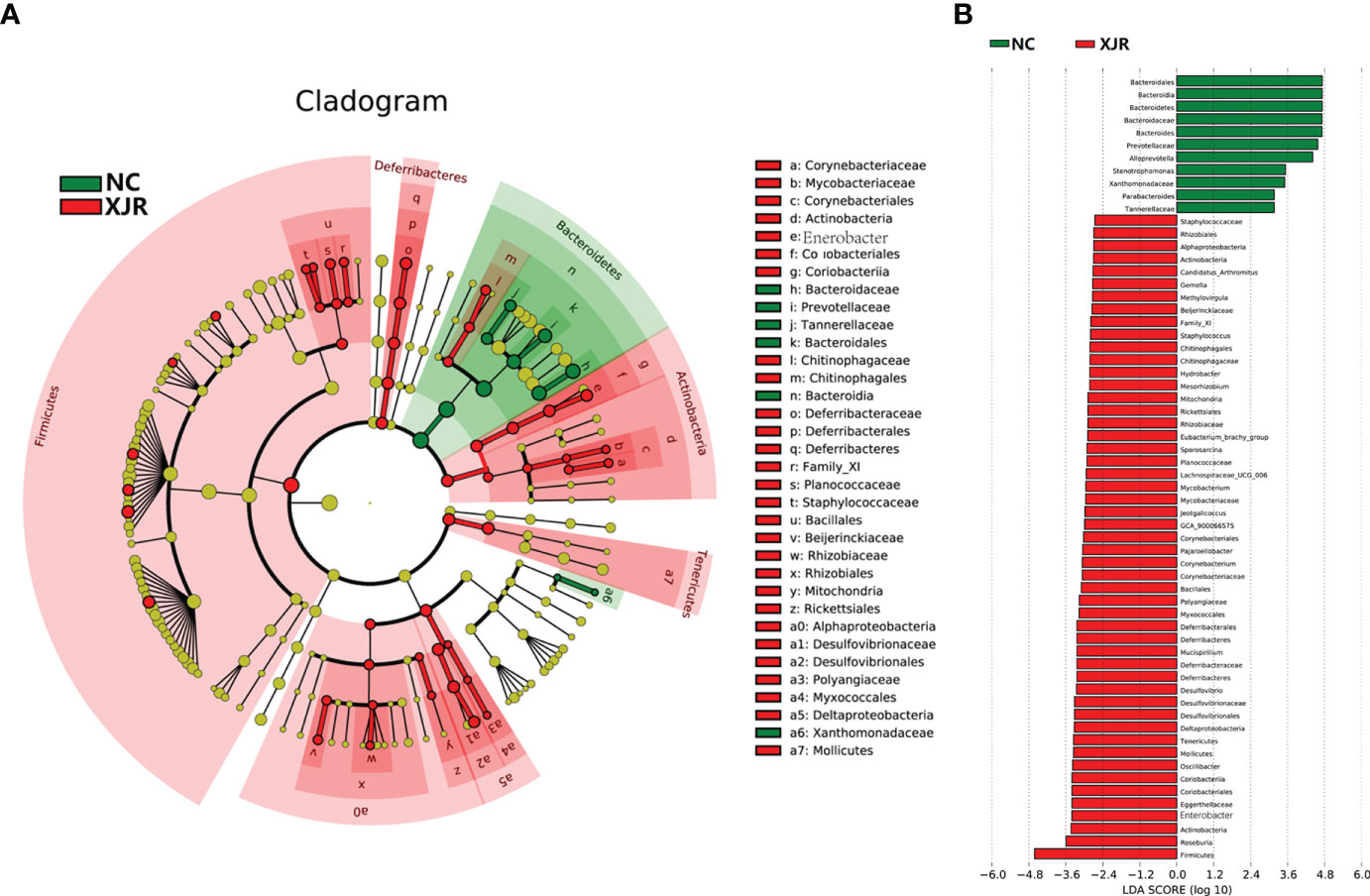
Figure 3 LEfSe analysis. (A) LDA scores of different abundant taxa in the faecal microbiomes from the NC and XJR groups. (B) Taxonomic cladogram from LEfSe. NC, control group; XJR, Xiaoai Jiedu Recipe.
Serum metabolomics
The altered serum metabolic profiles after XJR treatment was acquired by UPLC-MS. PCA results showed that the QC samples were closely clustered in the score plots, indicating that the analytical methods had good data quality and reproducibility (Figure 4A). Moreover, OPLS-DA model showed significant differences in the distribution of serum metabolic profile between the NC group and the XJR-treatment group (Figure 4B), suggesting that XJR led to significant biochemical changes. Identify metabolites involved in the clustering according to variable importance (VIP) values >1.0 and p-values<0.05, and these metabolites were selected for further identification. The HMDB Database (http://www.hmdb.ca/), METLIN Database (http://metlin.scripps.edu/), MassBank Database (http://www.massbank.jp/) and KEGG Database (http://www.genome.jp/kegg/) were utilized to identify the potential biomarkers.
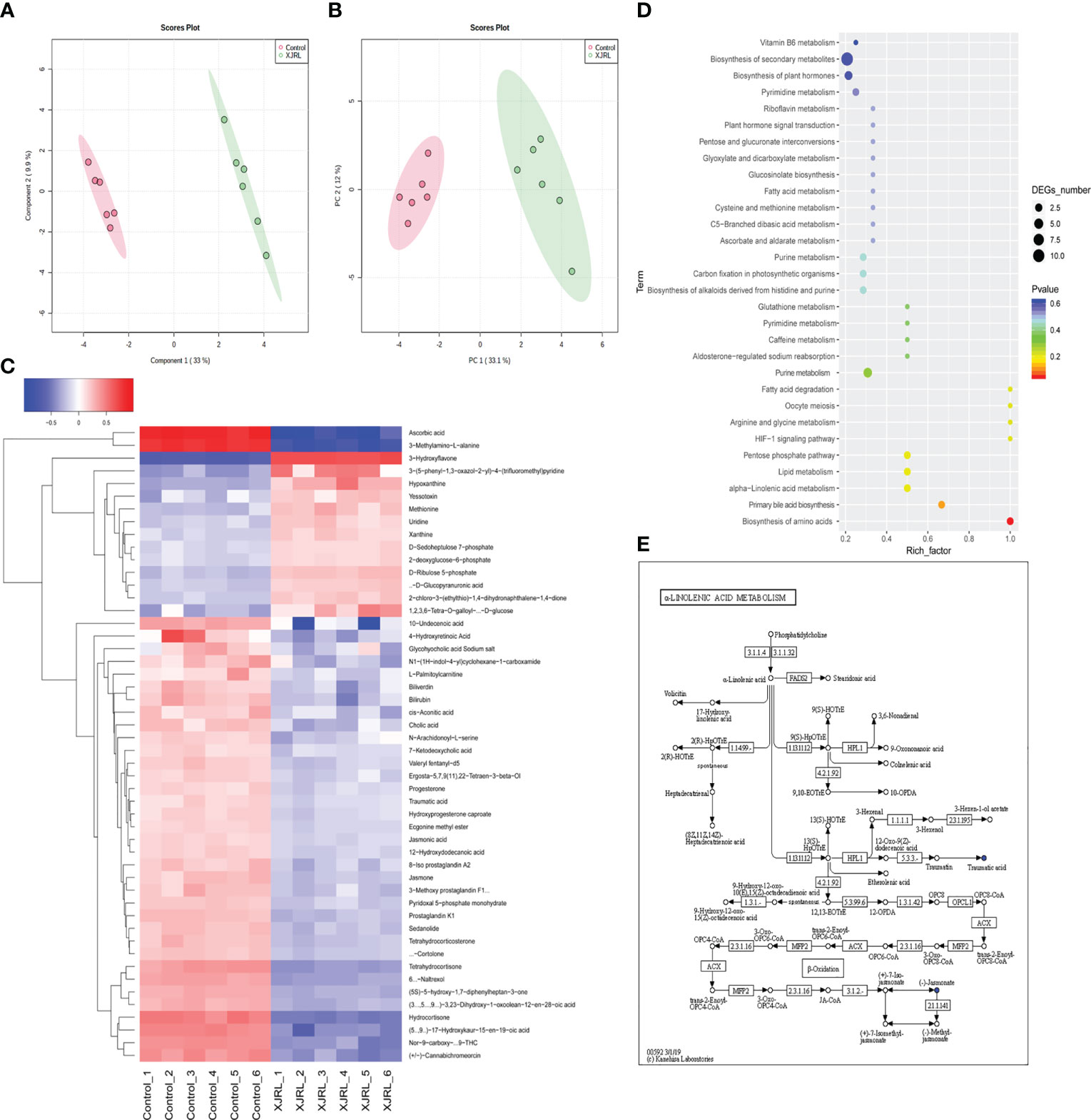
Figure 4 XJR altered serum metabolic profiles. (A) PCA plots and (B) OPLS-DA plots of the serum metabolic from NC and XJR groups. (C) Heat map showed normalised relative abundances of metabolites in the XJR group were significantly changed from those in the NC group. (D) Pathway enrichment of differential metabolites in the XJR group compared with the NC group. (E) Overview of alpha−linolenic acid metabolism with MetPA. NC, control group; XJR, Xiaoai Jiedu Recipe.
Finally, 50 serum metabolites with different abundances were identified. The heat map showed that the serum metabolites in the XJR-treatment group were well separated from those in the NC group, and the variation tendencies of these metabolites were also described in the heat map (Figure 4C). Thirteen serum metabolites (Table 1) were significantly increased and thirty-seven (Table 2) were significantly decreased after treatment of XJR compared with control.
Further pathway enrichment and network possibly affected by XJR were searched via Metabo Analyst. It mainly involved 12 metabolic pathways, including the biosynthesis of amino acids, primary bile acid biosynthesis, alpha−linolenic acid metabolism, ascorbic acid pathway, pentose phosphate pathway, HIF−1 signaling pathway, arginine and glycine metabolism, fatty acid degradation, purine metabolism, lipid metabolism, pyrimidine metabolism, and glutathione metabolism (Figure 4D). An overview of correlation analysis of alpha−linolenic acid metabolism according to Metabo was shown in Figure 4E.
Associations between the gut microbiota and serum metabolic phenotype
To investigate the associations between the altered gut microbiota and disturbed serum metabolites, we calculated the correlation matrixe based on Pearson’s correlation coefficients. We found a strong correlation between some bacteria and metabolites (r > 0.5 or r < –0.5, p<0.05). At the phylum level, there was a significant negative correlation between the protective bacteria such as Actinobacteria, Firmicutes, and Deferribacteres and Arachidonic acid, Adrenic acid, 15(S)−HpETE, DL−Arginine and Lysopc 18:2. However, there was a significant positive correlation between the aggressive bacteria such as Bacteroidetes and Arachidonic acid, Adrenic acid, 15(S)−HpETE, DL−Arginine and Lysopc 18:2 (Figure 5A). At the genus level, Roseburia and Enterorhabdus were negatively correlated with Arachidonic acid, Adrenic acid, 15(S)−HpETE, DL−Arginine and Lysopc 18:2. Bacteroides Prevotellaceae and UCG−001 were positively correlated with Arachidonic acid, Adrenic acid, 15(S)−HpETE, DL−Arginine and Lysopc 18:2 (Figure 5B). These results indicated that the altered intestinal flora was closely related to the arachidonic acid metabolism, and these two factors interacted to modulate inflammation. XJR may play a role in inhibiting CRC by fostering the growth of beneficial gut microbiota, inhibiting the growth of harmful gut microbiota, regulating the intestinal microecology balance and restoring inflammation.
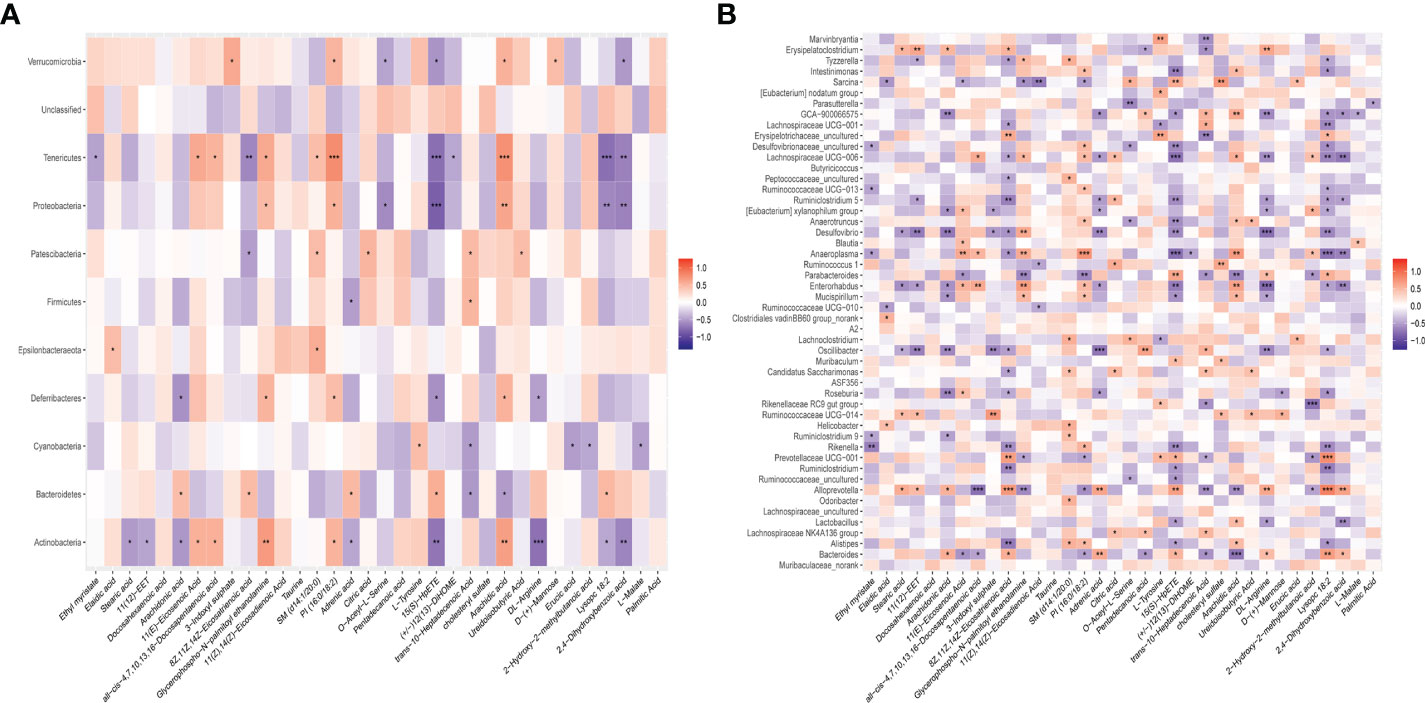
Figure 5 The close correlations between the relative abundance of gut microbiota and potential biomarkers. (A) The close correlations between the relative abundance of the main phylum and (B) genus in the gut microbiota and the potential serum biomarkers differently expressed between the NC and XJR group. NC, control group; XJR, Xiaoai Jiedu Recipe. *, p < 0.05; **, p< 0.01; ***, p < 0.001.
Discussion
CRC is one of the most common cancers of the digestive system with a high mortality rate, and causing serious health issues worldwide. Despite advances in treatment strategies, the prognosis for CRC remains unsatisfactory (18). As a classical anti-tumor prescription, XJR is composed of Hedyotis diffusa, Radix pseudostellariae, Akebia trifoliata Koidz, Radix ophiopogonis, Bombyx batryticatus, Cremastra appendiculata, and Centipede in a specific proportion. Although XJR has been clinically proven to prolong the survival and improve the quality of life of patients with various malignant tumors, its mechanism of action has not been clarified. In the study, we investigated the anti-CRC activity of XJR in vivo and vitro. The results indicated that XJR could inhibit tumor growth of CRC in vitro and vivo, and the low-dose XJR had the stronger inhibitory effect on tumor progression rather than the high-dose XJR. The complex mechanisms involved need to be further explored.
As a multifactorial disease, increasing researches had shown that intestinal flora played a decisive role in the occurrence and development of CRC, which may provide new treatment strategy of CRC (19). Therefore, we explored the regulatory effect of XJR on intestinal microflora in tumor-bearing mice with 16S rRNA gene sequencing. Compared with pre-treatment of XJR, the abundance of Bacteroidetes, Bacteroides, and Prevotellaceae decreased, while the abundance of Firmicutes, Roseburia, and Actinobacteria increased post-treatment. As opportunistic pathogens, the contents of Bacteroidetes, Bacteroides,and Prevotellaceae in CRC patients were higher than in healthy volunteers, which could be used as non-invasive markers for detection of CRC (20). Yuan et al. also found that at the phylum level, the abundance of Firmicutes and Actinobacteria was higher in healthy samples than in CRC patients, suggesting that they were probably “favourable”(20). Wang et al. found that the abundance of Roseburia was lower in CRC patients compared to normal people (21). In our study, Roseburia was significantly enriched after the treatment of XJR. Therefore, consistent with previous studies, we considered Roseburia to be the “beneficial bacteria”. As expected, the results demonstrated that XJR might effectively intervene in the progression of CRC by altering the composition profiles of fecal microbiota.
Metabolomics focuses on investigating the composition and change of endogenous metabolites in the biological specimen, which could reflect the impact of internal and external factors on their overall metabolism (22). In this study, there were 12 metabolic pathways possibly affected by XJR, among which the primary bile acid biosynthesis, alpha−linolenic acid metabolism, and ascorbic acid pathway had particularly attracted our attention. Liu et al. found the imbalance of bile acid metabolism can promote the development of CRC (23). Blood level of alpha−linolenic was inversely associated with the risk of CRC, which suggested that alpha−linolenic could be biomarkers for monitoring efficacy in patients with CRC (24). It has been reported that ascorbic acid could promote tumor regression, improved tolerability and side effects in patients with advanced CRC(25). Hence, we speculated that XJR play a role in inhibiting CRC by regulating the pathways of primary bile acid, alpha−linolenic acid, and ascorbic acid metabolism. We found that thirteen serum metabolites were more abundant while another thirty-seven serum metabolites were less abundant in the XJR group compared with the control group. In this study, the level of prostaglandin K1 was significantly reduced after the administration of XJR. Prostaglandins are inflammatory mediators generated from arachidonic acids under the action of cyclooxygenase-2 (COX-2), which are related to pathological processes, such as inflammation, the occurrence and progression of cancer, and cardiovascular disease(26). Previous studies have report that prostaglandin could promote CRC occurrence and progression, and inhibition of prostaglandin with COX-2 selective inhibitors or non-steroidal anti-inflammatory drugs (NSAIDs) could prevention of CRC (27, 28). Therefore, based on the above metabolomics results, we speculated that XJR could interfere in the development of CRC by regulating these metabolites.
Accumulated data indicate that the intestinal microbiota disturbances related to changes in metabolic phenotypes have been used to explore the possible mechanisms of disease progression and drug therapy (29). Herein, we investigated the correlation between altered gut microbiota and disturbed serum metabolites using Pearson’s correlation analysis. Of particular interest, there was a significant negative correlation between the protective bacteria such as Firmicutes, Roseburia, and Actinobacteria and Arachidonic acid, Adrenic acid, 15(S)−HpETE, DL−Arginine and Lysopc 18:2. The aggressive bacteria such as Bacteroidetes, Bacteroides, and Prevotellaceae were positively correlated with Arachidonic acid, Adrenic acid, 15(S)−HpETE, DL−Arginine and Lysopc 18:2. These results indicated that the altered intestinal flora was closely related to the arachidonic acid metabolism, and these two factors interacted to modulate inflammation(30). XJR may play a role in inhibiting CRC by fostering the growth of beneficial gut microbiota, inhibiting the growth of harmful gut microbiota, regulating the intestinal microecology balance and restoring inflammation. However, further research is needed to confirm this hypothesis.
In this study, XJR displayed significant anti-tumor effects without obvious side effects, suggesting it is a promising treatment for CRC. We also found that XJR significantly altered not only the fecal microbiota composition but also serum metabolic profiles. Furthermore, correlation analysis revealed that the altered gut microbiota had significant correlations with disturbed serum metabolites. However, we temporarily have no evidence to show that there is a direct causal connection between the ability of XJR to regulate intestinal flora, alter serum metabolic profile, and its ability to ameliorate CRC, which will be the focus of our next study. Overall, the regulation of gut microbiota and related metabolites may be potential breakthrough point to elucidate the mechanism of XJR in the treatment of the CRC. The strategy employed would provide theoretical basis for clinical application of TCM.
Data availability statement
The datasets presented in this study can be found in online repositories. The names of the repository/repositories and accession number(s) can be found in the article/supplementary material.
Ethics statement
The animal study was reviewed and approved by Nanjing University of Chinese Medicine.
Author contributions
Design of experiment: HGZ; paper drafting: WQ; in vitro experiment: XH; establishment of mouse model and in vivo experiment: HC and XH; data analysis: WQ and XH; paper correction: WQ, HLZ and ZW. All authors contributed to the article and approved the submitted version.
Funding
This work was supported by National Natural Science Foundation of China (No. 81973737 and 82001883).
Conflict of interest
The authors declare that the research was conducted in the absence of any commercial or financial relationships that could be construed as a potential conflict of interest.
Publisher’s note
All claims expressed in this article are solely those of the authors and do not necessarily represent those of their affiliated organizations, or those of the publisher, the editors and the reviewers. Any product that may be evaluated in this article, or claim that may be made by its manufacturer, is not guaranteed or endorsed by the publisher.
References
1. Siegel RL, Miller KD, Fuchs HE, Jemal A. Cancer statistic. CA Cancer J Clin (2022) 72:7–33. doi: 10.3322/caac.21708
2. Gerger A, Eisterer W, Fuxius S, Bastian S, Koeberle D, Welslau M, et al. Retrospective analysis of treatment pathways in patients with BRAF(V600E)-mutant metastatic colorectal carcinoma - MORSE(CRC). Anticancer Res (2022) 42:4773–85. doi: 10.21873/anticanres.15982
3. Menche J, Sharma A, Kitsak M, Ghiassian SD, Vidal M, Loscalzo J, et al. Disease networks. uncovering disease-disease relationships through the incomplete interactome. Science (2015) 347:1257601. doi: 10.1126/science.1257601
4. Guo J, Zhang L, Shang Y, Yang X, Li J, He J, et al. A strategy for intelligent chemical profiling-guided precise quantitation of multi-components in traditional Chinese medicine formulae-QiangHuoShengShi decoction. J Chromatogr A (2021) 1649:462178. doi: 10.1016/j.chroma.2021.462178
5. Qiu W, Wang Z, Chen R, Shi H, Ma Y, Zhou H, et al. Xiaoai jiedu recipe suppresses hepatocellular carcinogenesis through the miR-200b-3p /Notch1 axis. Cancer Manag Res (2020) 12:11121–31. doi: 10.2147/CMAR.S269991
6. Sang T, Qiu W, Li W, Zhou H, Chen H. The relationship between prevention and treatment of colorectal cancer and cancerous toxin pathogenesis theory basing on gut microbiota. Evid Based Complement Alternat Med (2020) 2020:7162545. doi: 10.1155/2020/7162545
7. Yang Y, Misra BB, Liang L, Bi D, Weng W, Wu W, et al. Integrated microbiome and metabolome analysis reveals a novel interplay between commensal bacteria and metabolites in colorectal cancer. Theranostics (2019) 9:4101–14. doi: 10.7150/thno.35186
8. Song M, Chan AT, Sun J. Influence of the gut microbiome, diet, and environment on risk of colorectal cancer. Gastroenterology. (2020) 158:322–40. doi: 10.1053/j.gastro.2019.06.048
9. Dalal N, Jalandra R, Bayal N, Yadav AK, Harshulika, Sharma M, et al. Gut microbiota-derived metabolites in CRC progression and causation. J Cancer Res Clin Oncol (2021) 147:3141–55. doi: 10.1007/s00432-021-03729-w
10. Chen D, Jin D, Huang S, Wu J, Xu M, Liu T, et al. Clostridium butyricum, a butyrate-producing probiotic, inhibits intestinal tumor development through modulating wnt signaling and gut microbiota. Cancer Lett (2020) 469:456–67. doi: 10.1016/j.canlet.2019.11.019
11. Flemer B, Lynch DB, Brown JM, Jeffery IB, Ryan FJ, Claesson MJ, et al. Tumour-associated and non-tumour-associated microbiota in colorectal cancer. Gut. (2017) 66:633–43. doi: 10.1136/gutjnl-2015-309595
12. Sun Q, Yang H, Liu M, Ren S, Zhao H, Ming T, et al. Berberine suppresses colorectal cancer by regulation of hedgehog signaling pathway activity and gut microbiota. Phytomedicine. (2022) 103:154227. doi: 10.1016/j.phymed.2022.154227
13. Zhao H, Ren S, Yang H, Tang S, Guo C, Liu M, et al. Peppermint essential oil: its phytochemistry, biological activity, pharmacological effect and application. BioMed Pharmacother (2022) 154:113559. doi: 10.1016/j.biopha.2022.113559
14. Qiu W, Sang T, Chen H, Zhou H, Wang Z. Wenzi jiedu recipe ameliorates colorectal cancer by remodeling the gut microbiota and tumor microenvironment. Front Oncol (2022) 12:915498. doi: 10.3389/fonc.2022.915498
15. Chen F, Dai X, Zhou CC, Li KX, Zhang YJ, Lou XY, et al. Integrated analysis of the faecal metagenome and serum metabolome reveals the role of gut microbiome-associated metabolites in the detection of colorectal cancer and adenoma. Gut. (2022) 71:1315–25. doi: 10.1136/gutjnl-2020-323476
16. Li T, Zhang T, Gao H, Liu R, Gu M, Yang Y, et al. Tempol ameliorates polycystic ovary syndrome through attenuating intestinal oxidative stress and modulating of gut microbiota composition-serum metabolites interaction. Redox Biol (2021) 41:101886. doi: 10.1016/j.redox.2021.101886
17. Xie H, Hua Z, Guo M, Lin S, Zhou Y, Weng Z, et al. Gut microbiota and metabonomics used to explore the mechanism of qing'e pills in alleviating osteoporosis. Pharm Biol (2022) 60:785–800. doi: 10.1080/13880209.2022.2056208
18. Canellas-Socias A, Cortina C, Hernando-Momblona X, Palomo-Ponce S, Mulholland EJ, Turon G, et al. Metastatic recurrence in colorectal cancer arises from residual EMP1(+) cells. Nature (2022) 611:603–13. doi: 10.1038/s41586-022-05402-9
19. Ren C, Yang M, Yang Z. The role of intestinal flora and ethnic differences in colorectal cancer risk. Gastroenterology (2022) 163:782–3. doi: 10.1053/j.gastro.2022.05.022
20. Yuan B, Ma B, Yu J, Meng Q, Du T, Li H, et al. Fecal bacteria as non-invasive biomarkers for colorectal adenocarcinoma. Front Oncol (2021) 11:664321. doi: 10.3389/fonc.2021.664321
21. Wang T, Cai G, Qiu Y, Fei N, Zhang M, Pang X, et al. Structural segregation of gut microbiota between colorectal cancer patients and healthy volunteers. ISME J (2012) 6:320–9. doi: 10.1038/ismej.2011.109
22. Clos-Garcia M, Andres-Marin N, Fernandez-Eulate G, Abecia L, Lavin JL, van Liempd S, et al. Gut microbiome and serum metabolome analyses identify molecular biomarkers and altered glutamate metabolism in fibromyalgia. EBioMedicine. (2019) 46:499–511. doi: 10.1016/j.ebiom.2019.07.031
23. Liu L, Yang M, Dong W, Liu T, Song X, Gu Y, et al. Gut dysbiosis and abnormal bile acid metabolism in colitis-associated cancer. Gastroenterol Res Pract (2021) 2021:6645970. doi: 10.1155/2021/6645970
24. Dai ZB, Ren XL, Xue YL, Tian Y, He BB, Xu CL, et al. Association of dietary intake and biomarker of alpha-linolenic acid with incident colorectal cancer: a dose-response meta-analysis of prospective cohort studies. Front Nutr (2022) 9:948604. doi: 10.3389/fnut.2022.948604
25. El Halabi I, Bejjany R, Nasr R, Mukherji D, Temraz S, Nassar FJ, et al. Ascorbic acid in colon cancer: from the basic to the clinical applications. Int J Mol Sci (2018) 19(9):2752. doi: 10.3390/ijms19092752
26. Mizuno R, Kawada K, Sakai Y. Prostaglandin E2/EP signaling in the tumor microenvironment of colorectal cancer. Int J Mol Sci (2019) 20(24):6254. doi: 10.3390/ijms20246254
27. Tsioulias GJ, Go MF, Rigas B. NSAIDs and colorectal cancer control: promise and challenges. Curr Pharmacol Rep (2015) 1:295–301. doi: 10.1007/s40495-015-0042-x
28. Zhang Z, Ghosh A, Connolly PJ, King P, Wilde T, Wang J, et al. Gut-restricted selective cyclooxygenase-2 (COX-2) inhibitors for chemoprevention of colorectal cancer. J Med Chem (2021) 64:11570–96. doi: 10.1021/acs.jmedchem.1c00890
29. Yu M, Jia H, Zhou C, Yang Y, Zhao Y, Yang M, et al. Variations in gut microbiota and fecal metabolic phenotype associated with depression by 16S rRNA gene sequencing and LC/MS-based metabolomics. J Pharm BioMed Anal (2017) 138:231–9. doi: 10.1016/j.jpba.2017.02.008
Keywords: traditional Chinese medicine, Xiaoai Jiedu recipe, colorectal cancer, gut microbiota, metabolic profiles
Citation: Qiu W, Xie H, Chen H, Zhou H, Wang Z and Zhou H (2023) Integrated gut microbiota and metabolome analysis reveals the mechanism of Xiaoai Jiedu recipe in ameliorating colorectal cancer. Front. Oncol. 13:1184786. doi: 10.3389/fonc.2023.1184786
Received: 19 April 2023; Accepted: 30 May 2023;
Published: 22 June 2023.
Edited by:
Hor Yue Tan, Hong Kong Baptist University, Hong Kong SAR, ChinaReviewed by:
Haibo Xu, Chengdu University of Traditional Chinese Medicine, ChinaShaohui Wang, Chengdu University of Traditional Chinese Medicine, China
Copyright © 2023 Qiu, Xie, Chen, Zhou, Wang and Zhou. This is an open-access article distributed under the terms of the Creative Commons Attribution License (CC BY). The use, distribution or reproduction in other forums is permitted, provided the original author(s) and the copyright owner(s) are credited and that the original publication in this journal is cited, in accordance with accepted academic practice. No use, distribution or reproduction is permitted which does not comply with these terms.
*Correspondence: Hongguang Zhou, zhouhongguang2002@163.com
†These authors have contributed equally to this work and share first authorship