- 1Cancer Center, Department of Ultrasound Medicine, Zhejiang Provincial People’s Hospital, Affiliated People’s Hospital, Hangzhou Medical College, Hangzhou, Zhejiang, China
- 2Zhejiang University School of Medicine, Hangzhou, China
Metabolomic analysis is a vital part of studying cancer progression. Metabonomic crosstalk, such as nutrient availability, physicochemical transformation, and intercellular interactions can affect tumor metabolism. Many original studies have demonstrated that metabolomics is important in some aspects of tumor metabolism. In this mini-review, we summarize the definition of metabolomics and how it can help change a tumor microenvironment, especially in pathways of three metabonomic tumors. Just as non-invasive biofluids have been identified as early biomarkers of tumor development, metabolomics can also predict differences in tumor drug response, drug resistance, and efficacy. Therefore, metabolomics is important for tumor metabolism and how it can affect oncology drugs in cancer therapy.
1 Introduction
Metabolism is dysregulated in tumor cells, thereby supporting the need for uncontrolled proliferation (1–3). Altered metabolism can result in different metabolic processes that can be targeted by drugs (4). Altered metabolism can also lead to unique metabolic phenotypes; thus, it can be used for diagnosing tumors earlier, selecting strategies for clinical trials, and as a biomarker of response after treatment. Many studies have demonstrated that metabolism is a vital link between environmental factors, host genes, diseases, and small metabolic molecules in tumor cells. Tumor cells reprogram metabolism and the microenvironment to support their proliferative ability (5, 6). Metabolic reprogramming of the tumor microenvironment is one of the characteristics of tumors and direction of tumor research (7). High-throughput bioinformatics technology and sequencing enable metabonomic analysis to determine the pathogenesis and etiology of tumors (8). Metabolomics is the last node of molecular pathways and last aim of omics (9).
Metabolomics includes systematic measurement of many metabolites, such as drugs, nutrients, signaling mediators, and some molecules in the urine and blood. It is also a useful tool for identifying biomarkers and drivers of tumorigenesis (10). Metabonomic analysis can measure the dynamic parameters of metabolic responses to many genetic changes and stimuli in large quantities (11). The techniques of metabolomics workflow include liquid chromatography-mass spectrometry, nuclear magnetic resonance, and gas chromatography-mass spectrometry (12, 13).
During tumor development, the famous part is the Warburg effect, which indicates that aerobic glycolysis pathway has a close relationship with cancer occurrence. Deregulated fatty acids and amino acids, such as serine, glutamine, and glycine can regulate metabolism to support tumor cells. Tumor cell development is closely related to three metabolic pathways (Figure 1) (9). Metabolomic analysis can supply tumor metabolism using the metabolic profiles of various tumor cells. This mini-review aims to explain how metabolomics can help change the tumor microenvironment, especially the three metabonomic pathways of tumors.
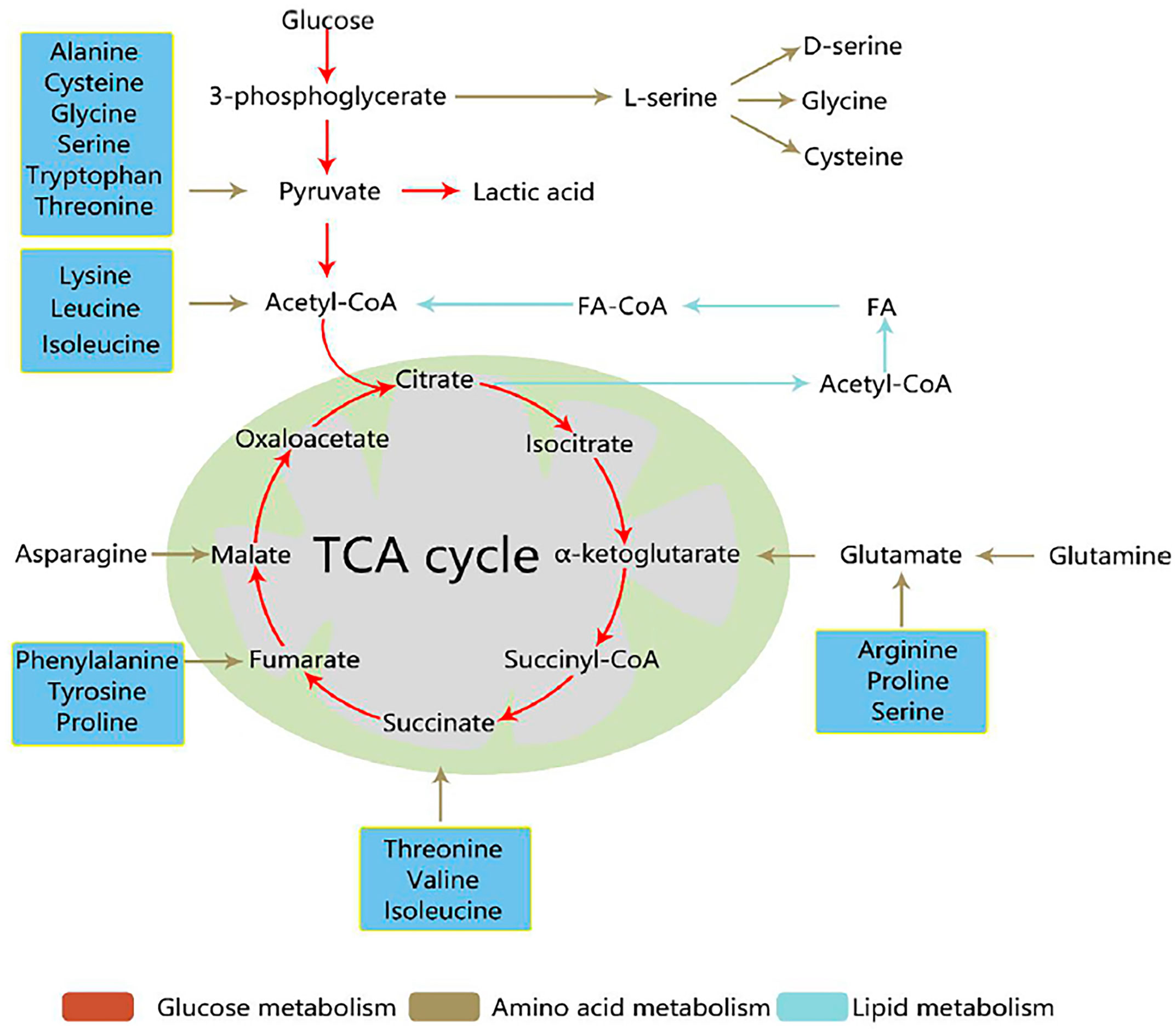
Figure 1 Three core metabolic pathways of cancer cells (9). Reprinted (adapted) with permission from Han, Li, Chen and Yang. (2021). Front Mol Biosci. 2021,8 (11),763902.
2 Definition of metabolomics
Biological systems have many aspects, such as genomics, proteomics, transcriptomics, and metabolomics. Metabolomics is different from other omics because it can provide a functional readout of metabolic processes, and is a target phenotype assessment (14, 15) (Figure 2). Metabolomics can provide a readout of alterations at RNA transcription, DNA replication, and protein levels. It is a sensitive method that identifies pathologic variants under small changes in protein and significant changes in metabolite levels (16). Metabolites can alter the activity of proteins and affect almost all biological processes.
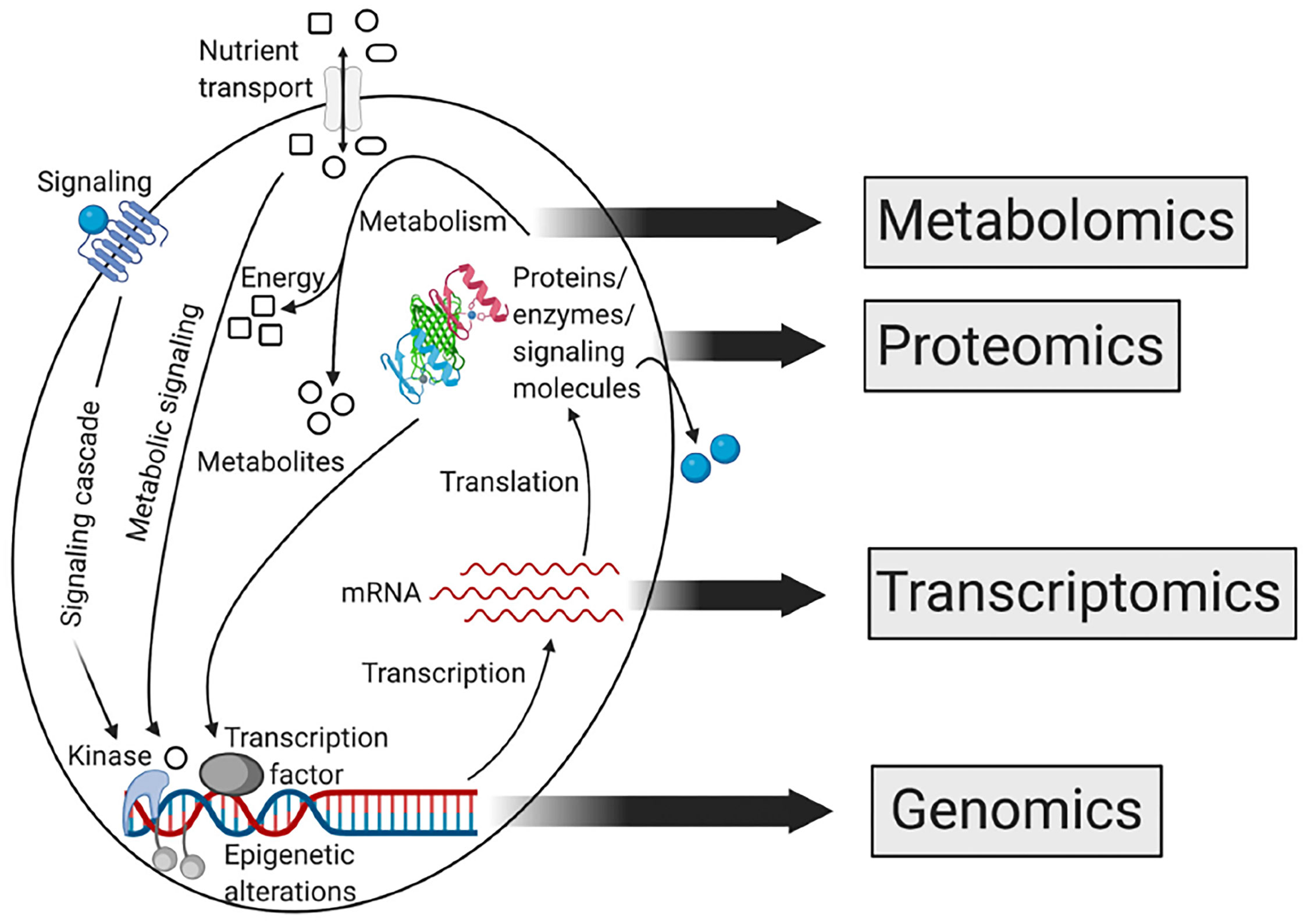
Figure 2 Relationship between different omics approaches of systems biology (15). Reprinted (adapted) with permission from the authors. CA: A Cancer Journal for Clinicians published by Wiley Periodicals LLC on behalf of American Cancer Society. (2021). CA Cancer J Clin. 2021,71(4),333-358.
Metabolomics seems to be the analysis of some small-molecule metabolites in a biological specimen (14). It is different from standard clinical metabolites, such as urea or glucose, which rely on enzymatic reactions and a separate test for every metabolite. Recent technological advances have significantly improved metabolomics. Metabolomics can be used to produce smaller footprints.
Metabolomics involves various methods with unique advantages and disadvantages. The selection point of the appropriate method is whether a(n) targeted/untargeted method is desired. Targeted metabolomics is always used to test hypotheses and implement pictures. Untargeted metabolomics is used for biomarker discovery and hypothesis generation (17). Metabolomics deals with a complex set of molecules and is chemically diverse, while genomics, proteomics, and transcriptomics aim to identify macromolecular structures that are the sequences of amino acids, nucleotides, and so on. These chemical constituents are always limited in diversity and are well defined. Metabonomic analysis is used for data acquisition and sample preparation because sugars, lipids, organic acids, and polar molecules have various physical features. Untargeted experiments require validation with chemical standards using targeted methods. Analyzing different groups using untargeted metabolomics requires significant effort. It is important for untargeted metabolomics to facilitate biological understanding.
3 Metabolomic analysis of tumor metabolic pathways
3.1 Glucose metabolism
Owing to the huge need for tumor cell proliferation, rapid glycolysis of tumor cells is called the Warburg effect. The Warburg effect always leads to hypoxia in the tumor microenvironment (18). Glycolysis is not as effective as aerobic respiration in terms of energy supply; however, it is faster and can provide essential amino acids and intermediate metabolites of pentose phosphate in proliferating tumor cells (19). Tumor cells can be weakened or cease to use the mitochondrial aerobic oxidation pathway (20). Tumor cells are more likely to use the glycolysis pathway to produce lactic acid. The key point of the Warburg phenotype is aerobic glycolysis, which supports tumor cell proliferation by active metabolism. Glycolysis and other pathways that require glucose are also involved in glucose metabolism (21). Perroud et al. found that in renal tumor cells, enzymes that include hexokinase-1 pyruvate kinase and lactate dehydrogenase A in glycolysis can be significantly increased (22). They also found that in renal tumor cells, metabolic reprogramming is important for metabolomics, transcriptomics, and proteomics (23). Dai et al. studied breast tumors and cultured MCF-7 and T47D cells with different glucose concentrations. They demonstrated that with lower glucose levels, the proliferation of breast tumor cells can be inhibited (24). Ding et al. found that in lung tumors, glucose metabolism disorders were associated with carcinogenesis of lung tumor, thereby indicating that glucose metabolism can be a therapeutic method for lung tumor cells (25). Increased expression of metabolic enzymes can cause the Warburg effect, which is related to glycolysis. However, regulation of rate-limiting enzymes has attracted much attention from researchers in recent years (26). Researchers confirmed that the KRAS gene’s effect on cancer cells metabolism can occur in the method of transcriptional regulation of glycolysis enzymes and glucose transporters (26). Upregulation of glycolysis can provide potential targets in cancer therapy in the tumor cell microenvironment and many tumor-promoting signaling pathways (27).
3.2 Amino acid metabolism
Amino acids are required by tumor cells to increase their proliferation. They can be used not only in protein synthesis but also as metabolites that regulate tumor cell growth (28). In terms of potential biomarkers and tumor pathogenesis, multiple amino acids have been confirmed to be useful (29). Among the amino acids, serine, glycine, and glutamine have attracted attention (30). Chen et al. found that when the amino acid metabolic spectrum changes, it is always associated with gastric tumor occurrence (31). Glutamine is the most abundant free amino acid and can be found in macromolecular synthesis, energy generation, and signal transmission in tumor cells when provided with carbon atoms and nitrogen (32, 33). Glutamine can synthesize amino acids and join the tricarboxylic acid cycle. In many tumor cells, mitochondrial-dependent bioenergy production and cell biosynthesis increases the need for glutamine (34). Wettersten et al. also found higher levels of glutamine in renal clear cell carcinoma than in normal renal cells (35, 36). Additionally, arginine and aspartic acid can also be involved in reprogramming amino acid metabolism in tumors. Aspartic acid concentration is significantly negatively correlated with breast cancer when compared to gas chromatography technology and combined liquid chromatography (37). Antimetabolites that interrupt amino acid synthesis can be developed and subjected to clinical trials (38).
3.3 Lipid metabolism
Significant features of lipid metabolism in cancer cells include upregulated mitochondrial fatty acid β-oxidation levels and increased adipogenesis rate (39). Many cancer cells exhibit similar trends, and some metabolites involved in lipid metabolism exhibit changes. Peng et al. indicated that in the tumor microenvironments, lipid synthesis in cancer cells increased (40). Lipids provide energy to maintain membrane synthesis in tumor cells. With increased saturated fat content, the risk of some cancers increases. Dietary saturated fat can contribute to cancer progression in primary prostate cancer (41). Poczobutt et al. found that lipid metabolomic techniques can provide important information on lipid changes in many tumor cells (42). Lipid metabolism resembles a network of pathways with feedback loops and crosstalk to meet the metabolic needs of tumor cells. It can affect not only the recombination of molecules in tumor cells but also regulate crosstalk and supply high metabolic needs for the tumor cells. For example, the lipid-activated transcription factor, liver X receptor, plays a vital role in modulating the tumor microenvironment. Traversari et al. found that apoptotic cancer cells, which contain oxysterols, activate the liver X receptor in macrophages, can recruit neutrophils, and suppress dendritic cell migration; thus, resulting in immunosuppression and tolerance (43). Brandi et al. studied metabolomic detection, lipid uptake of new fat to form lipid droplets, and changes in lipid desaturation and fatty oxidation, which are related to the regulation of cancer stem cells (44). Wang et al. indicated that lipid metabolism can meet not only the standard of energy needs and biomass production of cancer stem cells, but can also activate some carcinogenic signaling pathways, such as the Wnt/β-catenin and Hippo/YAP signaling pathways (45). In summary, the lipid metabolism of tumor cells and their important roles in cancer progression and metastasis have attracted the attention of researchers.
4 Metabolomics in oncology drugs for cancer therapy
There are many different metabolomic markers in cancer progression, for example plasma (blood biomarkers), urine (urine biomarkers), saliva (salivary biomarkers) and cerebrospinal fluid (cerebrospinal fluid biomarkers), these markers had great importance for early diagnosing malignant tumors. Metabolomics can be used to detect tumor cell metabolites in clinical practice. It can also act as a reflection of antitumor drugs. Metabolomics can be used to improve drug efficacy and reduce adverse reactions. Zhang et al. pointed out that lipid metabolism in imidazole ketone erastin has distinct features that can induce ferroptosis and slow tumor cell growth (46). Metabolic levels can reflect gene and protein changes in terminal metabolites, once drugs begin to act on the body. Early efficacy of drugs can be evaluated using metabolomics, which enables adjustment of medication regimens.
The metabolic patterns of cancer cells change after the development of drug resistance. The same drug can induce different metabolic changes in sensitive and drug-resistant cells (47). Thus, metabolomics can be used to detect metabolic changes in cells and their response to drugs to determine whether tumor cells are resistant to drugs, and to monitor drug resistance at an early stage. Metabolomics is an easy and effective method that uses dynamic and multivariable methods to evaluate metabolic results, and to predict and assess drug resistance and sensitivity to chemotherapy. Poschner et al. used liquid chromatography mass spectrometry to characterize the levels of active estrogen, steroids, and sulfated glucose in platinum-resistant ovarian tumor cells. They demonstrated that these metabolites are highly expressed in carboplatin-sensitive cells (48). Thus, metabolomics can be used to distinguish between metabolite levels and platinum resistance. Jiye et al. found that metabolic pattern analysis can monitor drug resistance in the early stages, and can help doctors carry out follow-up treatment by observing metabolic differences between drug-sensitive and drug-resistant patients (49). Metabolomics has made significant progress in the study of drug resistance genes in tumor cells.
5 Conclusion
Metabolomics is of great importance in various aspects of tumor metabolism, especially in helping to change the tumor microenvironment in the three metabonomic pathways of tumors: glucose, amino acid, and lipid metabolisms. Therefore, metabolomic analysis can be used in various aspects of tumor research, such as detecting tumor cell metabolites, evaluating the effects of anti-tumor drugs, and therapeutic response.
Author contributions
Conception of the study ZZ and C-CX, developing the search strategy ZZ and C-CX, conducting the literature search and summary ZZ, drafting the article ZZ, editing the article C-CX, and funding acquisition ZZ. All authors contributed to the article and approved the submitted version.
Funding
This study was supported by General Scientific Research Project of Zhejiang Provincial Education Department, China (Grant No.Y202249231).
Conflict of interest
The authors declare that the research was conducted in the absence of any commercial or financial relationships that could be construed as a potential conflict of interest.
Publisher’s note
All claims expressed in this article are solely those of the authors and do not necessarily represent those of their affiliated organizations, or those of the publisher, the editors and the reviewers. Any product that may be evaluated in this article, or claim that may be made by its manufacturer, is not guaranteed or endorsed by the publisher.
References
1. Pavlova NN, Thompson CB. The emerging hallmarks of cancer metabolism. Cell Metab (2016) 23(1):27–47. doi: 10.1016/j.cmet.2015.12.006
2. Teicher BA, Linehan WM, Helman LJ. Targeting cancer metabolism. Clin Cancer Res (2012) 18(20):5537–45. doi: 10.1158/1078-0432.CCR-12-2587
3. Vander Heiden MG, DeBerardinis RJ. Understanding the intersections between metabolism and cancer biology. Cell (2017) 168(4):657–69. doi: 10.1016/j.cell.2016.12.039
4. Vernieri C, Casola S, Foiani M, Pietrantonio F, de Braud F, Longo V. Targeting cancer metabolism: dietary and pharmacologic interventions. Cancer Discov (2016) 6(12):1315–33. doi: 10.1158/2159-8290.CD-16-0615
5. Wu T, Dai Y. Tumor microenvironment and therapeutic response. Cancer Lett (2017) 387:61–8. doi: 10.1016/j.canlet.2016.01.043
6. Ringel AE, Drijvers JM, Baker GJ, Catozzi A, Garcia-Canaveras JC, Gassaway BM, et al. Obesity shapes metabolism in the tumor microenvironment to suppress anti-tumor immunity. Cell (2020) 183(7):1848–1866 e26. doi: 10.1016/j.cell.2020.11.009
7. Huang Z, Lin L, Gao Y, Chen Y, Yan X, Xing J, et al. Bladder cancer determination via two urinary metabolites: a biomarker pattern approach. Mol Cell Proteomics (2011) 10(10):M111 007922. doi: 10.1074/mcp.M111.007922
8. Kaushik AK, DeBerardinis RJ. Applications of metabolomics to study cancer metabolism. Biochim Biophys Acta Rev Cancer (2018) 1870(1):2–14. doi: 10.1016/j.bbcan.2018.04.009
9. Han J, Li Q, Chen Y, Yang Y. Recent metabolomics analysis in tumor metabolism reprogramming. Front Mol Biosci (2021) 8:763902. doi: 10.3389/fmolb.2021.763902
10. Rinschen MM, Ivanisevic J, Giera M, Siuzdak G. Identification of bioactive metabolites using activity metabolomics. Nat Rev Mol Cell Biol (2019) 20(6):353–67. doi: 10.1038/s41580-019-0108-4
11. Johnson CH, Ivanisevic J, Siuzdak G. Metabolomics: beyond biomarkers and towards mechanisms. Nat Rev Mol Cell Biol (2016) 17(7):451–9. doi: 10.1038/nrm.2016.25
12. Fiehn O. Metabolomics by gas chromatography-mass spectrometry: combined targeted and untargeted profiling. Curr Protoc Mol Biol (2016) 114:30 4 1–30 4 32. doi: 10.1002/0471142727.mb3004s114
13. Lane AN, Higashi RM, Fan TW. NMR and MS-based stable isotope-resolved metabolomics and applications in cancer metabolism. Trends Analyt Chem (2019) 120:115322. doi: 10.1016/j.trac.2018.11.020
14. Wishart DS, Jewison T, Guo AC, Wilson M, Knox C, Liu Y, et al. HMDB 3.0–the human metabolome database in 2013. Nucleic Acids Res (2013) 41(Database issue):D801–7. doi: 10.1093/nar/gks1065
15. Schmidt DR, Patel R, Kirsch DG, Lewis CA, Vander Heiden MG, Locasale JW. Metabolomics in cancer research and emerging applications in clinical oncology. CA Cancer J Clin (2021) 71(4):333–58. doi: 10.3322/caac.21670
16. Griffin JL, Shockcor JP. Metabolic profiles of cancer cells. Nat Rev Cancer (2004) 4(7):551–61. doi: 10.1038/nrc1390
17. Schrimpe-Rutledge AC, Codreanu SG, Sherrod SD, McLean JA. Untargeted metabolomics strategies-challenges and emerging directions. J Am Soc Mass Spectrom (2016) 27(12):1897–905. doi: 10.1007/s13361-016-1469-y
18. Warburg O. On respiratory impairment in cancer cells. Science (1956) 124(3215):269–70. doi: 10.1126/science.124.3215.269
19. Cacciatore S, Loda M. Innovation in metabolomics to improve personalized healthcare. Ann N Y Acad Sci (2015) 1346(1):57–62. doi: 10.1111/nyas.12775
20. Chen WL, Wang JH, Zhao AH, Xu X, Wang YH, Chen TL, et al. A distinct glucose metabolism signature of acute myeloid leukemia with prognostic value. Blood (2014) 124(10):1645–54. doi: 10.1182/blood-2014-02-554204
21. Kishton RJ, Barnes CE, Nichols AG, Cohen S, Gerriets VA, Siska PJ, et al. AMPK is essential to balance glycolysis and mitochondrial metabolism to control T-ALL cell stress and survival. Cell Metab (2016) 23(4):649–62. doi: 10.1016/j.cmet.2016.03.008
22. Perroud B, Lee J, Valkova N, Dhirapong A, Lin PY, Fiehn O, et al. Pathway analysis of kidney cancer using proteomics and metabolic profiling. Mol Cancer (2006) 5:64. doi: 10.1186/1476-4598-5-64
23. Perroud B, Ishimaru T, Borowsky AD, Weiss RH. Grade-dependent proteomics characterization of kidney cancer. Mol Cell Proteomics (2009) 8(5):971–85. doi: 10.1074/mcp.M800252-MCP200
24. Dai X, Cheng H, Bai Z, Li J. Breast cancer cell line classification and its relevance with breast tumor subtyping. J Cancer (2017) 8(16):3131–41. doi: 10.7150/jca.18457
25. Ding M, Li F, Wang B, Chi G, Liu H. A comprehensive analysis of WGCNA and serum metabolomics manifests the lung cancer-associated disordered glucose metabolism. J Cell Biochem (2019) 120(6):10855–63. doi: 10.1002/jcb.28377
26. Kerr EM, Gaude E, Turrell FK, Frezza C, Martins CP. Mutant kras copy number defines metabolic reprogramming and therapeutic susceptibilities. Nature (2016) 531(7592):110–3. doi: 10.1038/nature16967
27. Zhang Y, Gao Y, Li Y, Zhang X, Xie H. Characterization of the relationship between the expression of aspartate beta-hydroxylase and the pathological characteristics of breast cancer. Med Sci Monit (2020) 26:e926752. doi: 10.12659/MSM.926752
28. Wen LY, Zhang YS, Zhou X, Li G, Hu CY, Li Y, et al. Effect of branched chain amino acids on perioperative temperature, glucose level and fat metabolism in patients with gastrointestinal tumors. J Biol Regul Homeost Agents (2018) 32(2):357–63.
29. Jung J, Jung Y, Bang EJ, Cho SI, Jang YJ, Kwak JM, et al. Noninvasive diagnosis and evaluation of curative surgery for gastric cancer by using NMR-based metabolomic profiling. Ann Surg Oncol (2014) 21 Suppl:4, S736–42. doi: 10.1245/s10434-014-3886-0
30. Yip-Schneider MT, Simpson R, Carr RA, Wu H, Fan H, Liu Z, et al. Circulating leptin and branched chain amino acids-correlation with intraductal papillary mucinous neoplasm dysplastic grade. J Gastrointest Surg (2019) 23(5):966–74. doi: 10.1007/s11605-018-3963-y
31. Chen JL, Tang HQ, Hu JD, Fan J, Hong J, Gu JZ. Metabolomics of gastric cancer metastasis detected by gas chromatography and mass spectrometry. World J Gastroenterol (2010) 16(46):5874–80. doi: 10.3748/wjg.v16.i46.5874
32. Coloff JL, Murphy JP, Braun CR, Harris IS, Shelton LM, Kami K, et al. Differential glutamate metabolism in proliferating and quiescent mammary epithelial cells. Cell Metab (2016) 23(5):867–80. doi: 10.1016/j.cmet.2016.03.016
33. Kappler M, Pabst U, Rot S, Taubert H, Wichmann H, Schubert J, et al. Normoxic accumulation of HIF1alpha is associated with glutaminolysis. Clin Oral Investig (2017) 21(1):211–24. doi: 10.1007/s00784-016-1780-9
34. Wang Y, Bai C, Ruan Y, Liu M, Chu Q, Qiu L, et al. Coordinative metabolism of glutamine carbon and nitrogen in proliferating cancer cells under hypoxia. Nat Commun (2019) 10(1):201. doi: 10.1038/s41467-018-08033-9
35. Wettersten HI, Hakimi AA, Morin D, Bianchi C, Johnstone ME, Donohoe DR, et al. Grade-dependent metabolic reprogramming in kidney cancer revealed by combined proteomics and metabolomics analysis. Cancer Res (2015) 75(12):2541–52. doi: 10.1158/0008-5472.CAN-14-1703
36. Hakimi AA, Reznik E, Lee CH, Creighton CJ, Brannon AR, Luna A, et al. An integrated metabolic atlas of clear cell renal cell carcinoma. Cancer Cell (2016) 29(1):104–16. doi: 10.1016/j.ccell.2015.12.004
37. Xie G, Zhou B, Zhao A, Qiu Y, Zhao X, Garmire L, et al. Lowered circulating aspartate is a metabolic feature of human breast cancer. Oncotarget (2015) 6(32):33369–81. doi: 10.18632/oncotarget.5409
38. Tabe Y, Lorenzi PL, Konopleva M. Amino acid metabolism in hematologic malignancies and the era of targeted therapy. Blood (2019) 134(13):1014–23. doi: 10.1182/blood.2019001034
39. Currie E, Schulze A, Zechner R, Walther TC, Farese RV Jr. Cellular fatty acid metabolism and cancer. Cell Metab (2013) 18(2):153–61. doi: 10.1016/j.cmet.2013.05.017
40. Peng X, Chen Z, Farshidfar F, Xu X, Lorenzi PL, Wang Y, et al. Molecular characterization and clinical relevance of metabolic expression subtypes in human cancers. Cell Rep (2018) 23(1):255–269 e4. doi: 10.1016/j.celrep.2018.03.077
41. Labbe DP, Zadra G, Yang M, Reyes JM, Lin CY, Cacciatore S, et al. High-fat diet fuels prostate cancer progression by rewiring the metabolome and amplifying the MYC program. Nat Commun (2019) 10(1):4358. doi: 10.1038/s41467-019-12298-z
42. Poczobutt JM, Nguyen TT, Hanson D, Li H, Sippel TR, Weiser-Evans MC, et al. Deletion of 5-lipoxygenase in the tumor microenvironment promotes lung cancer progression and metastasis through regulating T cell recruitment. J Immunol (2016) 196(2):891–901. doi: 10.4049/jimmunol.1501648
43. Traversari C, Sozzani S, Steffensen KR, Russo V. LXR-dependent and -independent effects of oxysterols on immunity and tumor growth. Eur J Immunol (2014) 44(7):1896–903. doi: 10.1002/eji.201344292
44. Brandi J, Dando I, Pozza ED, Biondani G, Jenkins R, Elliott V, et al. Proteomic analysis of pancreatic cancer stem cells: functional role of fatty acid synthesis and mevalonate pathways. J Proteomics (2017) 150:310–22. doi: 10.1016/j.jprot.2016.10.002
45. Wang H, Xi Q, Wu G. Fatty acid synthase regulates invasion and metastasis of colorectal cancer via wnt signaling pathway. Cancer Med (2016) 5(7):1599–606. doi: 10.1002/cam4.711
46. Zhang Y, Tan H, Daniels JD, Zandkarimi F, Liu H, Brown LM, et al. Imidazole ketone erastin induces ferroptosis and slows tumor growth in a mouse lymphoma model. Cell Chem Biol (2019) 26(5):623–33.e9. doi: 10.1016/j.chembiol.2019.01.008
47. Zhang X, Chen Y, Hao L, Hou A, Chen X, Li Y, et al. Macrophages induce resistance to 5-fluorouracil chemotherapy in colorectal cancer through the release of putrescine. Cancer Lett (2016) 381(2):305–13. doi: 10.1016/j.canlet.2016.08.004
48. Poschner S, Wackerlig J, Castillo-Tong DC, Wolf A, Decken IV, Rizner TL, et al. Metabolism of estrogens: turnover differs between platinum-sensitive and -resistant high-grade serous ovarian cancer cells. Cancers (Basel) (2020) 12(2):279. doi: 10.3390/cancers12020279
Keywords: metabonomic, metabolomic, metabolism, tumor, drug resistance
Citation: Zeng Z and Chen C-X (2023) Metabonomic analysis of tumor microenvironments: a mini-review. Front. Oncol. 13:1164266. doi: 10.3389/fonc.2023.1164266
Received: 12 February 2023; Accepted: 03 April 2023;
Published: 14 April 2023.
Edited by:
Yuanyuan Zheng, Tongji University School of Medicine, ChinaReviewed by:
Genyan Qin, Changde Hospital of Traditional Chinese Medicine, ChinaHang Qi, Philips, China
Copyright © 2023 Zeng and Chen. This is an open-access article distributed under the terms of the Creative Commons Attribution License (CC BY). The use, distribution or reproduction in other forums is permitted, provided the original author(s) and the copyright owner(s) are credited and that the original publication in this journal is cited, in accordance with accepted academic practice. No use, distribution or reproduction is permitted which does not comply with these terms.
*Correspondence: Cong-Xian Chen, a2VtYTMyMEBxcS5jb20=