- 1The First Hospital of Huzhou, Huzhou, China
- 2Zhejiang University of Science and Technology, Hangzhou, China
Immune-related genes are important factors in tumor progression. The main aim of this study was to identify the immune-related genes in kidney papillary cell carcinoma (pRCC) patients. We downloaded RNAseq data and clinical information of pRCC patients from the TCGA database and retrieved the immune-related genes list from Immport. From the data, we mined out 2,468 differential expression genes (DEGs) and 183 immune-related DEGs. Four hub DEGs (NTS, BIRC5, ELN, and CHGA) were identified after conducting Cox analysis and LASSO analysis. Moreover, the prognostic value of the signature based on four hub DEGs was verified using Kaplan–Meier analysis (P = 0.0041 in the training set and p = 0.021 in the test set) and ROC analysis (AUC: 0.957 in 1 year, 0.965 in 2 years, and 0.901 in 3 years in the training set, and 0.963 in 1 year, 0.898 in 2 years, and 0.742 in 3 years in the test set). Furthermore, we found that the high-risk score group had a higher percentage of B cells in the immune component, a higher expression of immune-related genes (CTLA4, LAG3, PDCD1LG2, and TIGIT), and a better immunotherapy response.
Introduction
Kidney papillary cell carcinoma (pRCC) is the second most common type of renal cancer after renal clear cell carcinoma (1). It is worth noting that the first choice treatment method for pRCC patients is maximum resection of the tumor. However patients, who had done the resection of the tumor, face the challenge of disease progression (2). Therefore, immunotherapy has become the latest choice for advanced metastatic pRCC patients (3). Nivolumab, a programmed death 1 (PD-1) immune checkpoint inhibitor monoclonal antibody, was approved as monotherapy in 2015 for metastatic RCC patients after treatment with a VEGF-targeting agent. In April 2018, the combination of nivolumab and ipilimumab, a CTLA-4 inhibitor, was approved for intermediate- and poor-risk, previously untreated patients with metastatic RCC. Then, in 2019, combination therapies consisting of pembrolizumab (anti-PD-1) or avelumab [anti-PD-ligand (L) 1] with axitinib (a VEGF receptor tyrosine kinase inhibitor) were also approved to treat metastatic RCC and were likely to produce dramatic shifts in the therapeutic landscape (4, 5). According to EAU guidelines (6), immunotherapy was a second-line therapy option for advanced metastatic pRCC patients by the end of 2020.
The prognostic value of immune-related genes has become a subject of persistent focus in cancer research. Some special immune-related genes or the signature has a significant survival prognostic value for tumor patients (7, 8). However, the studies which focused on the relationship with immune related genes and pRCC were few. The main aim of this study was to identify the immune-related genes in pRCC patients.
Methods and Results
Identification of Immune-Related Genes Using Differential Expression Data
We downloaded the gene expression RNAseq data and clinical phenotype of TCGA kidney papillary cell carcinoma (KIRP) from the UCSC Xena database (https://xenabrowser.net/datapages/). The data was then pre-processed with the cancer tissue using the following steps: 1. Exclusion of the samples without clinical data; 2. Exclusion of genes with FPKM <1 from all samples. We used the R package “limma” (condition: adjusted P Value <0.01, and |logFC|>2) to find the differentially expressed genes. Furthermore, we downloaded the list of the immune-related genes from the ImmPort Portal database (https://www.immport.org/home/) which contained 2,483 immune-related genes (9).
In total, we obtained 321 sample data which contained 289 cases of kidney papillary cell carcinoma (pRCC) and 32 normal samples from the TCGA KIPR data. After the data was pre-processed, all the 289 pRCC and 32 normal samples were enrolled. Among them, 2,468 differentially expressed genes (DEGs): 638 up-regulated genes and 1,830 downregulated genes (Figures 1A, B) were identified using R package “limma” (10). Intersection of the immune-related genes and 2,483 DEGs resulted in the identification of 183 immune-related DEGs.
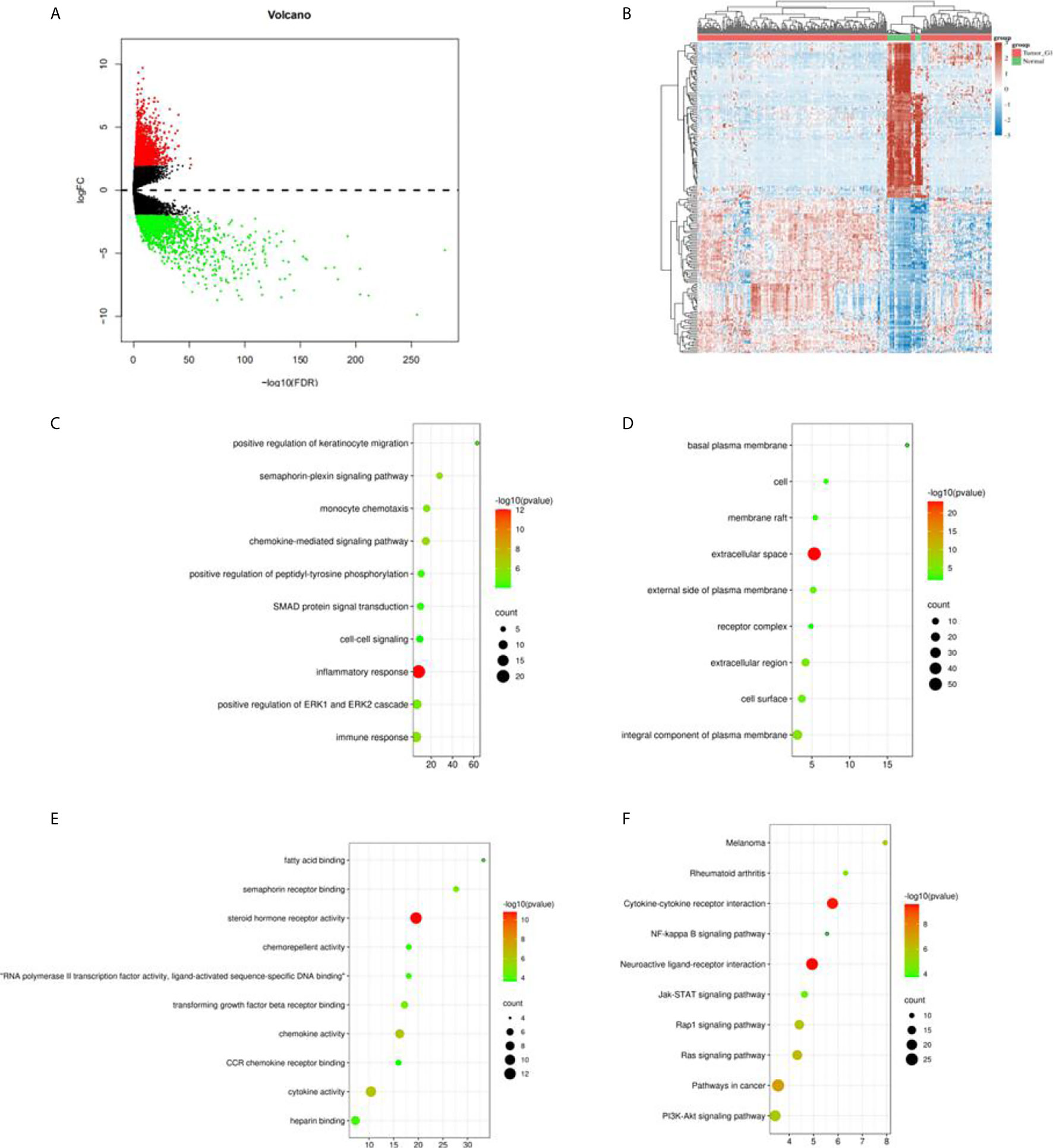
Figure 1 Differential expression genes in TCGA KIRC data and enrichment analysis. (A) Volcano plot in the differential expression genes (DEGs) in TCGA KIRC data; (B) the heatmap of top 50 DEGs; (C) the biological process in GO term; (D) the cellular component in GO term; (E) the molecular function in GO term; (F) the pathway analysis in KEGG function.
Functional and Pathway Enrichment Analysis of Immune-Related DEGs
We used an online analysis tool created by David (https://david.ncifcrf.gov/) to perform gene ontology (GO) analysis and Kyoto Encyclopedia of Genes and Genomes (KEGG) analysis for all the 183 immune-related DEGs (11).
GO analysis classified the DEGs into three groups: molecular function group, biological process group, and cellular component group. The biological results revealed that DEGs were primarily enriched in inflammatory response, positive regulation of ERP1 and ERK2 cascade, and immune response (Figure 1C). The cellular component results indicated that DEGs were mainly enriched in extracellular space, an integral component of the plasma membrane (Figure 1D). The molecular function results showed that DEGs were mainly enriched in semaphorin receptor binding, chemokine activity, and cytokine activity (Figure 1E). Moreover, KEGG analysis indicated that the DEGs’ pathways were mainly enriched in neuroactive ligand–receptor interaction and cytokine–cytokine receptor interaction (Figure 1F).
Training and Test Sets
We divided 289 samples into a training set (n = 203) and a test set (n = 86). The two sets satisfied the following criteria: 1. Samples were randomly divided into two sets; 2. Age, clinical stage, and follow-up time between the two sets were similar. The clinical information obtained from the two sets is shown in Table 1.
Identification of Survival Genes From the Immune-Related DEGs in the Training Set
Firstly, we used univariate Cox proportional hazard regression to analyze the RNAseq expression and survival date of all the 183 DEGs in the training set. Secondly, LASSO Cox regression analysis was used to analyze the valuable DEGs. Finally, multivariate Cox regression analysis was performed to identify survival DEGs. R package “survival” and “glmnt’ were used for the above calculation, and p <0.05 was considered to be statistically significant.
Univariate Cox regression analysis results identified 39 DEGs (Table 2), of which five were left after conducting LASSO Cox regression analysis for a thousand times (Figures 2A, B). Finally, four DEGs (NTS, BIRC5, ELN, and CHGA) were selected as the survival genes after conducting multivariate Cox regression analysis (Table 3). The survival state, risk score, and heatmap of four hub genes in the training set were shown (Figures 2C–E).
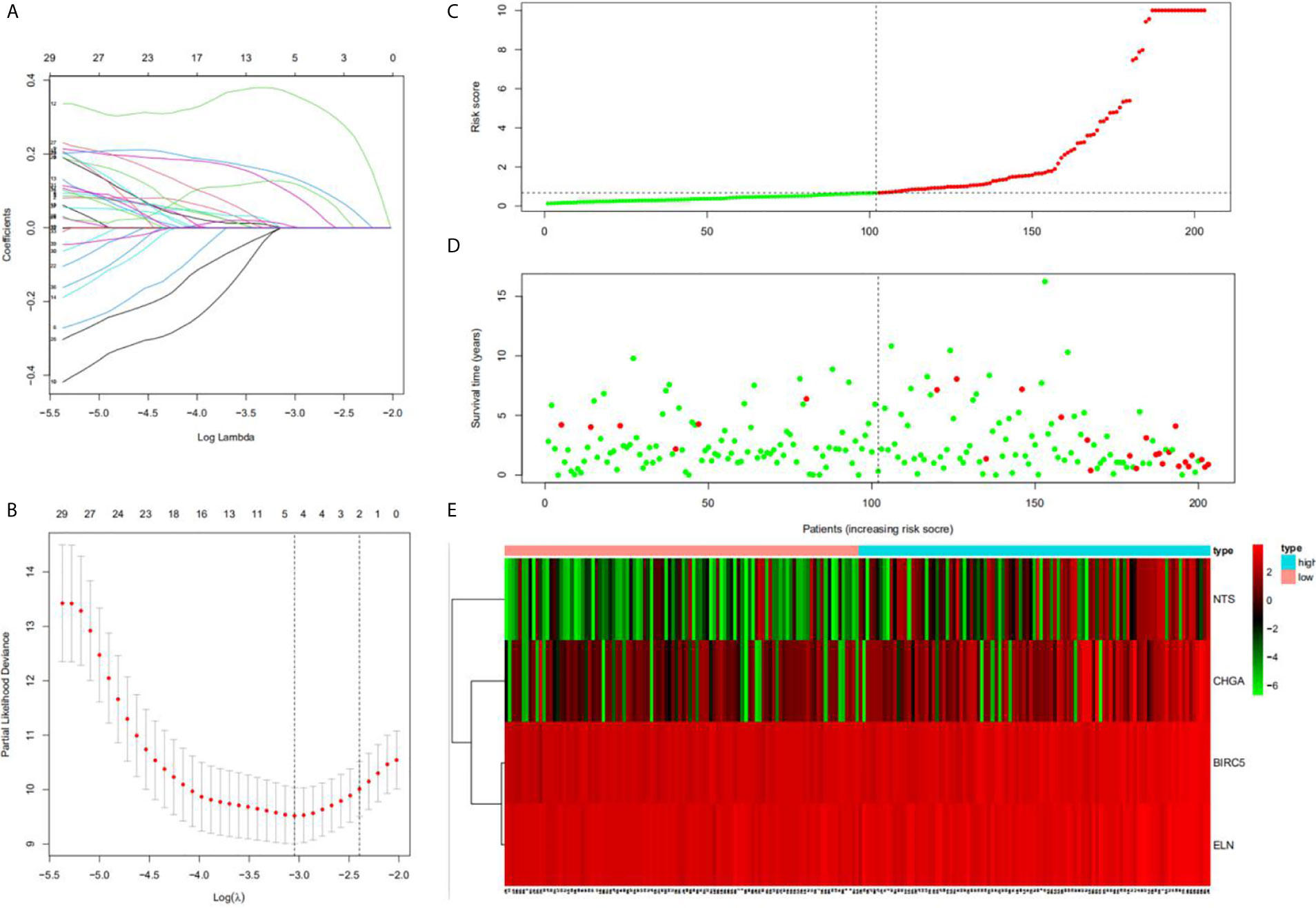
Figure 2 Construction of the hub DEGs and risk score prognostic value. (A, B) The determination of the number of factors by the LASSO analysis; (C) the distribution of risk score patients; (D) the survival state of patients; (E) a heatmap of hub DEGs in the training set.
Analysis of Hub DEGs
Three hub genes (NTS, ELN, and CHGA) had lower mRNA expression, and one hub gene (BIRC5) had higher mRNA expression in primary cancer tissue compared to the normal kidney tissue (Figure 3A). In addition, Kaplan–Meier analysis results indicated that three genes (BIRC5, ELN, and CHGA) were survival-related (Figures 3B–E). We used the STRING online tool (https://string-db.org/) (12) to determine the protein–protein interactive relationship of the four hub genes (Figure 3F). The DNA methylation analysis function of UALCAN (http://ualcan.path.uab.edu/) (13) revealed that DNA hyper-methylation occurred as a result of high-level mRNA expression of BIRC5 (p = 0.0013, Figure 4C).
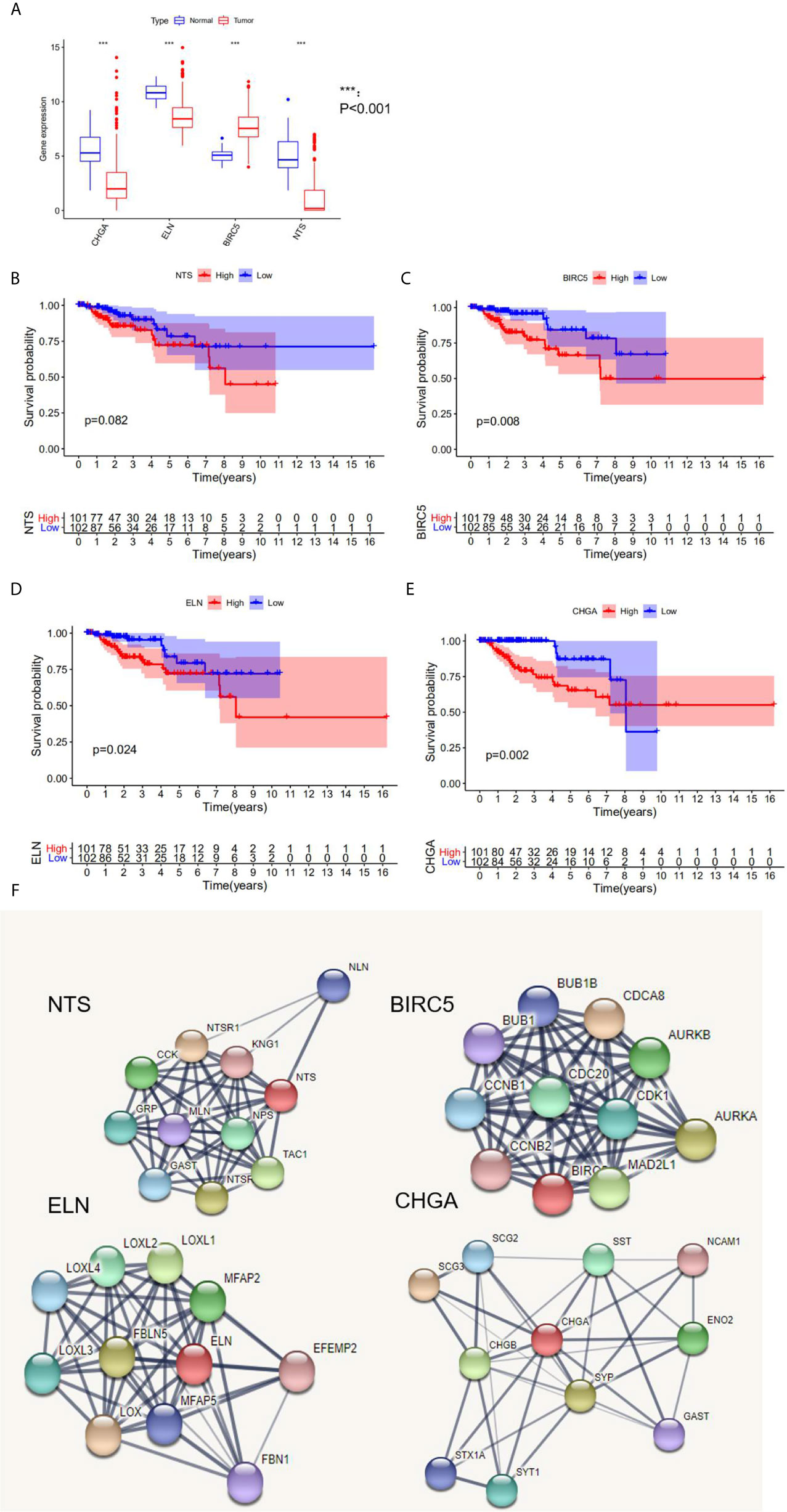
Figure 3 The analysis of hub DEGs’ expression, survival results, and protein–protein interaction. (A) The mRNA expression of four hub DEGs in normal vs tumor tissue; (B–E) the survival results in Kaplan-Meier analysis; (F) the protein-protein interaction of four hub DEGs.
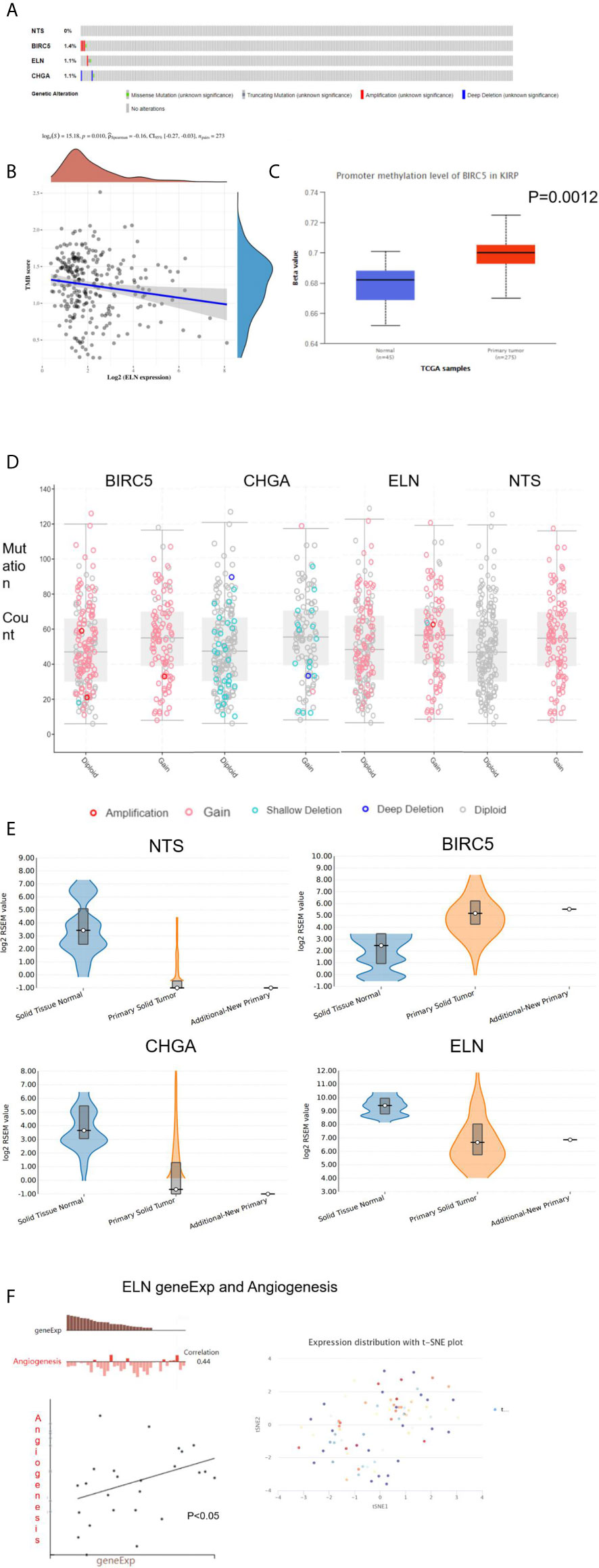
Figure 4 The analysis of hub DEGs’ DNA methylation, TMB, CBV, and variable shear. (A) The genetic alterations of four hub DEGs; (B) The correlation between ELN geneEpx and TMB; (C) the correlation between BIRC5 geneEpx and DNA methylation level; (D) the correlation between hub DEGs geneEpx and CNV; (E) the correlation between hub DEGs geneEpx and variable shear; (F) the correlation between ELN geneEpx and angiogenesis.
The cbioportal website (https://www.cbioportal.org/) (14) was then used to explore the genetic alterations (Figure 4A) and copy numbers (CNV, Figure 4D) of the four hub genes. On the other hand, the ACB website (https://www.aclbi.com/static/index.html) (15) indicated that ELN gene expression was correlated with tumor mutation load (TMB, p = 0.01, Figure 4B). We also investigated the variable shear situation of the four hub genes in the TSVdb website (http://www.tsvdb.com/) (16). Results obtained from the online single-cell library of CancerSea (http://biocc.hrbmu.edu.cn/CancerSEA/, Figure 4E) (17) indicated that the mRNA expression of ELN was associated with angiogenesis (correlation = 0.44, p < 0.05, Figure 4F).
Prognostic Value Verification of the Four-mRNA Signature in the Training Set and Test Set
The risk score of each patient was calculated based on the coefficients: Risk score = (0.250656 * Exp NTS) + (0.465259 * Exp BIRC5) + (0.251223 * Exp ELN) + (0.241936 * Exp CHGA). We found that the four-mRNA signature risk score was an independent factor in multivariate Cox analysis (Table 4).
A total of 203 samples in the training set were divided into high-risk score group (n = 101) and low-risk score group (n = 102) according to the risk score. A comparison of the two groups indicated that the high-risk score group had a higher mortality rate, while the low-risk score group had a large number of surviving patients (Figures 3C–E). Moreover, similar results were observed when 86 samples in the test set were divided into high-risk score group (n = 42) and low-risk score group (n = 44).
We also performed Kaplan–Meier analysis and ROC analysis for risk score on the training set and test set. Kaplan–Meier analysis in the training set found that the high-risk score group had a significantly shorter overall survival time (p = 0.0041, Figure 5A), while the ROC curves showed that the four-mRNA signature had good accuracy with 0.957 in 1 year, 0.965 in 2 years, and 0.901 in 3 years (Figure 5A). On the other hand, Kaplan–Meier analysis in the test set indicated that the high score group also had a significantly shorter overall survival time (p = 0.021, Figure 5B), and the time-dependent ROC curves had good accuracy (0.963 in 1 year, 0.898 in 2 years, and 0.742 in 3 years, Figure 5B). Furthermore, nomogram models for the training set were drawn (Figure 5B).
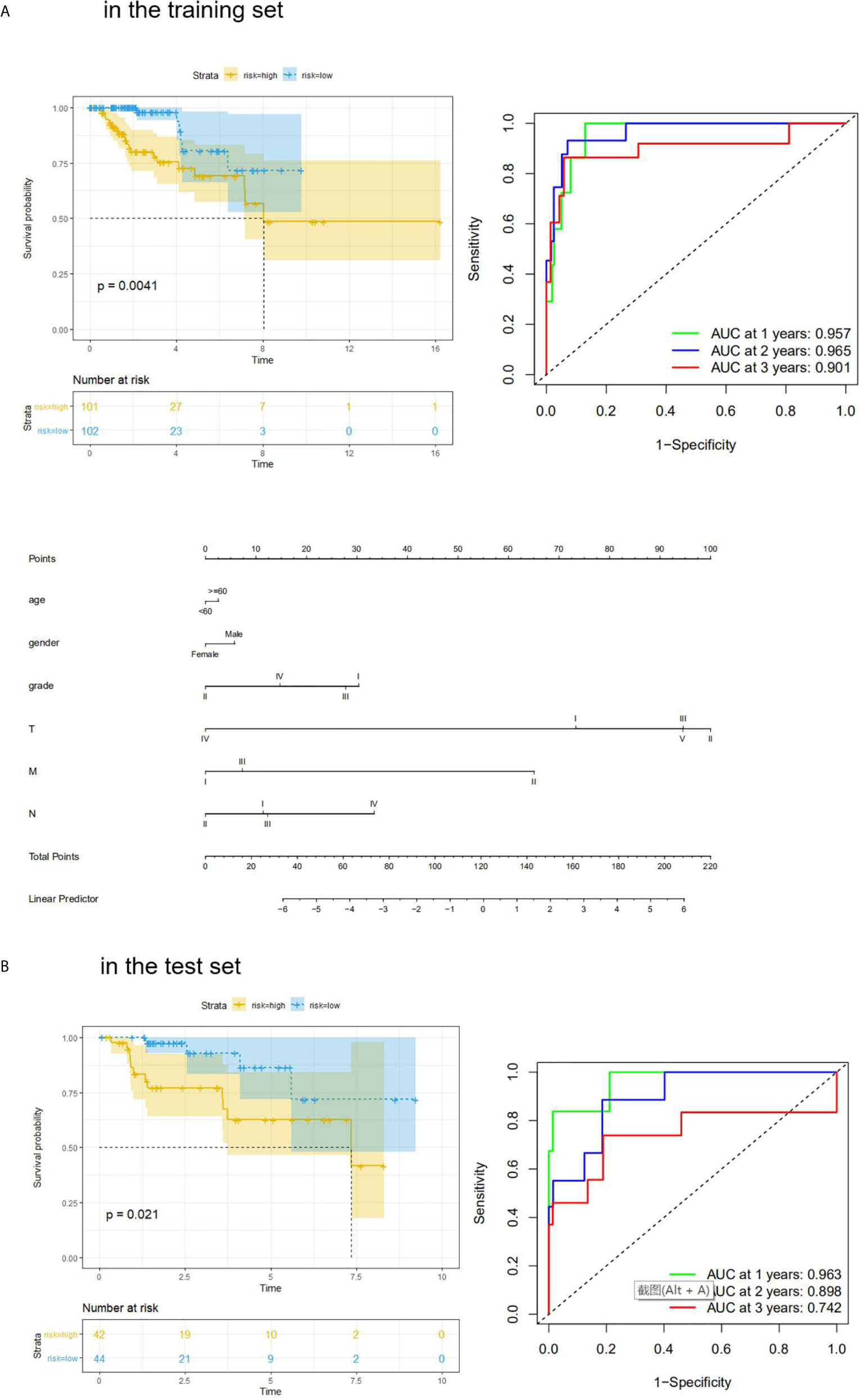
Figure 5 KM analysis and ROC analysis of risk score value in both sets. (A) KM analysis, ROC analysis, and clinical nomogram model of risk score value in the training set; (B) KM analysis, and ROC analysis of risk score value in the test set.
Correlation Between Risk Score and Clinical Feature
We analyzed the correlation between risk score and different clinical information (tumor, lymph node, metastasis degrees, and grades). The results showed that the risk score had significant differences in tumor, lymph node, metastasis degrees, and grades (Figure 6A). Moreover, time-dependent ROC analysis found that the four-mRNA signature had better accuracy compared to other clinical features (Figure 6B).
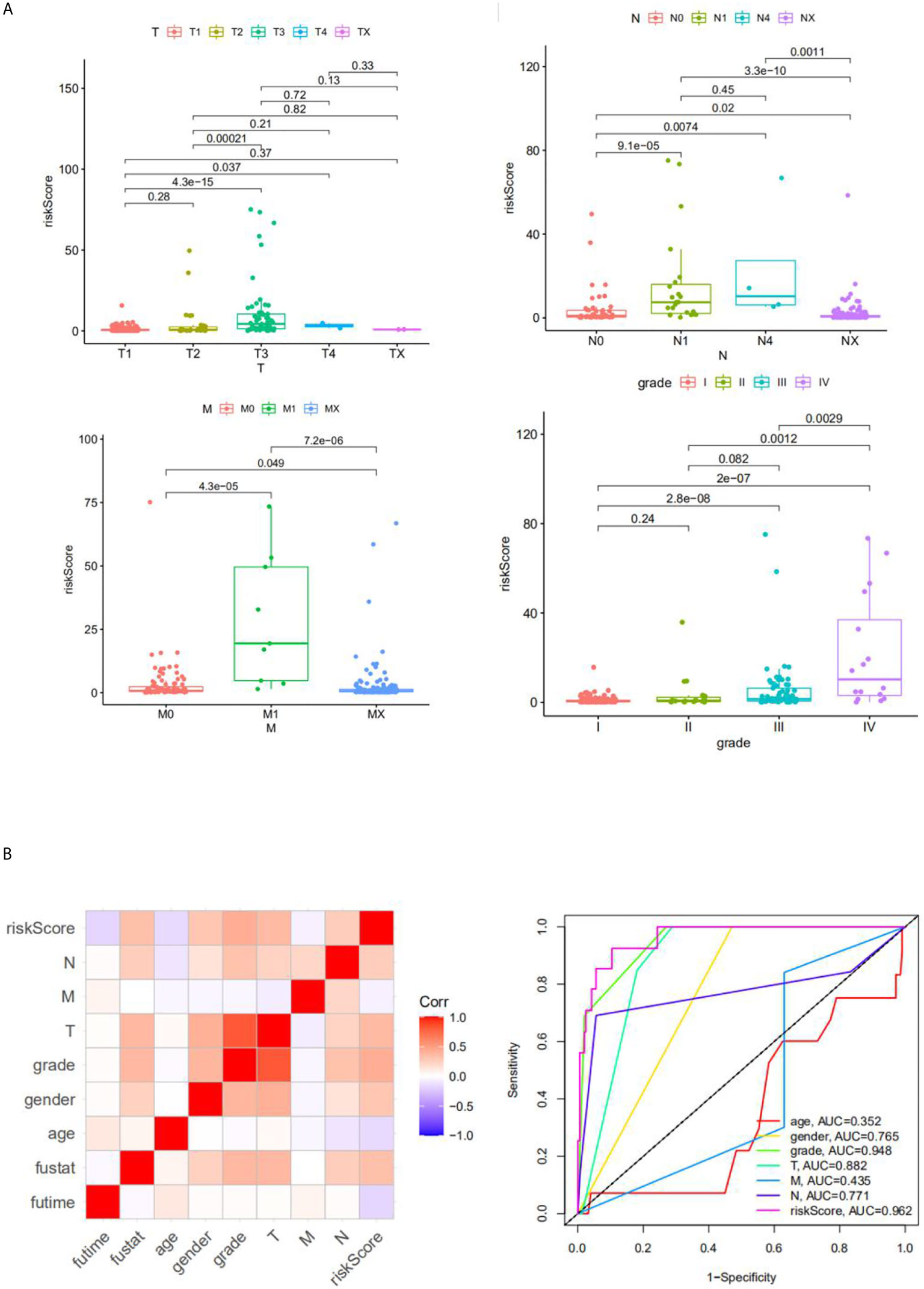
Figure 6 The correlation between the risk score and clinical classifier. (A) The correlation between the risk score and TNM grade information; (B) the ROC.
The Relationship Between the Risk Score and Immune Cell Component, Expression of Immune-Related Genes, and Immunotherapy Response
We explored the immune cell component using two online analysis tools (TIMER: https://cistrome.shinyapps.io/timer/ (18) and ImmuCellAI: http://bioinfo.life.hust.edu.cn/ImmuCellAI/ (19)). TIMER analysis results showed that a significantly higher percentage of B cells (P = 0.0029), T cell + CD4 (P = 0.0002), T cell + CD8 (P = 0.0029), Neutrophil cells (P = 0.0004), and DC cells (P = 0.0047) would appear in the high-risk score group in the training set (Figure 7A). On the other hand, ImmuCellAI analysis results indicated that the high-risk score group had a higher percentage of Exhausted cells (P = 0.00003) and B cells (P = 0.0003) (Figure 7B). We also analyzed the expression of seven immune-related genes (CTLA4, CD274, LAG3, SIGLEC15, PDCD1LG2, HAVCR2, and TIGIT) and correlated the expression with the risk score. The results showed that CTLA4, LAG3, PDCD1LG2, and TIGIT had a higher expression in the high-risk score group (P < 0.05, Figure 7C). In addition, the expression of PDCD1LG2 and TIGIT genes correlated with the risk score value (P < 0.05, Figures 7D, E). According to the immunotherapy response results obtained after ImmuCellAI analysis, the high-risk score group had a better immunotherapy response (P = 0.0013, Figure 7F).
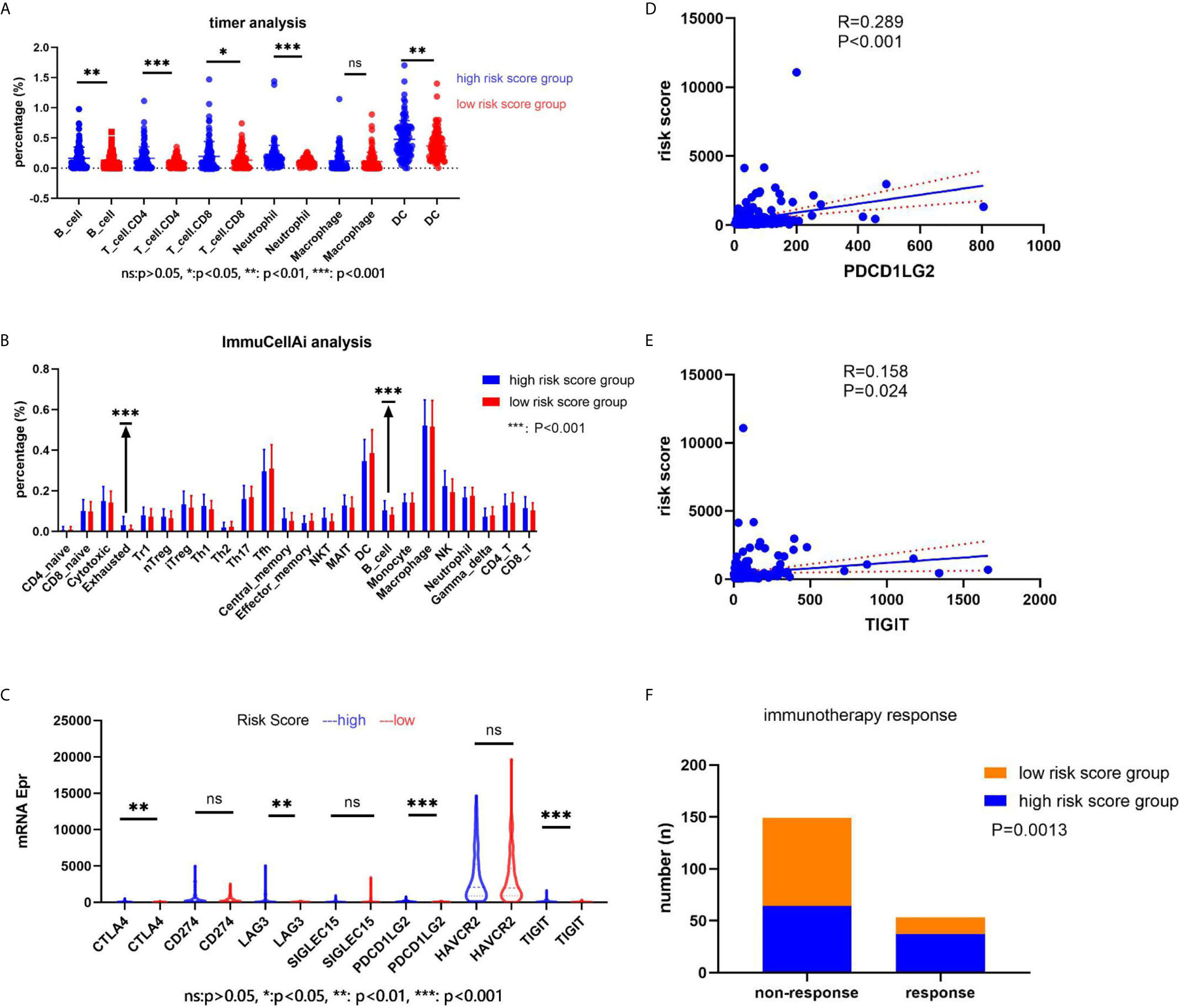
Figure 7 The relationship between the risk score and immune cell component, the immune-related genes’ expression, and the immunotherapy response. (A) The immune cell component in the web of timer; (B) the immune cell component in the web of ImmuCellAI; (C) the immune-related genes’ expression in two groups; (D, E) the correlation between the risk score and the immune-related genes; (F) the immunotherapy response.
Discussion
In this study, we identified four hub mRNA genes (NTS, BIRC5, ELN, and CHGA) using univariate and multivariate Cox analysis and LASSO analysis. Pro Qiu team have reported that NTS was a neurotensin receptor that participates in the colorectal cancer tissue (20). The pro AKter team found that NTS had the function of cell migration and invasion in gastro-intestinal and cardiovascular functions (21). The Ye team also found that the NTS gene activates the Wnt/β-catenin signaling pathway, thereby promoting tumor metastasis (22). BIRC5 is a member of the apoptosis inhibitor gene family, which encodes regulatory proteins that prevent apoptotic cell death (23). It regulates several types of cancer cells by activating a multiple-step cell apoptosis process (24, 25). ELN encodes the elastin protein, which is a key protein in the tumor microenvironment (26). Moreover, the protein encoded by the CHGA gene is a member of the chromogranin/secretogranin family of neuroendocrine secretory proteins (27). Its gene product is a precursor of the peptides which act as autocrine or paracrine negative modulators of the neuroendocrine system (28).
We then verified the prognostic value of the signature in both training and test sets. Kaplan–Meier analysis showed that the high-risk score group had a bad survival time, while ROC analysis found that the AUC of the signature was excellent (0.957 in 1 year, 0.965 in 2 years, and 0.901 in 3 years in the training set). A previous study had developed a five-mRNA gene signature for pRCC patients and proved that the AUC of the signature was 0.82 (29). Furthermore, we conducted a correlation between the risk score and clinical classification and found that the risk score was correlated with the TNM stage. The above results convinced us that the signature had an accurate prognostic value.
Finally, we conducted an analysis of the immune component and found different immune components in the two risk score groups. TIMER and ImmuCellAI analyses results indicated that the high-risk score group had a higher percentage of B cells in the immune component. Moreover, we conducted a correlation between the risk score and expression of immune-related genes. Our results indicated that the high-risk score group had a higher expression level of CTLA4, LAG3, PDCD1LG2, and TIGIT. In addition, the high-risk score group had a better immunotherapy response.
In summary, this study has identified four hub immune-related genes (NTS, BIRC5, ELN, and CHGA) in pRCC patients. We also developed a signature of four hub genes which can act as an independent prognostic factor for overall survival. Our results suggest that pRCC patients with a high-risk score have a shorter survival time and a better immunotherapy response.
Data Availability Statement
The original contributions presented in the study are included in the article/supplementary material. Further inquiries can be directed to the corresponding author.
Ethics Statement
Ethical review and approval was not required for the study on human participants in accordance with the local legislation and institutional requirements. Written informed consent for participation was not required for this study in accordance with the national legislation and the institutional requirements.
Author Contributions
JS wrote the paper. RW and LZ edited the paper. YC, ZF, JY, XZ, and YL analyzed the data. JT made the images out. All authors contributed to the article and approved the submitted version.
Funding
The Zhejiang province medical and health project (2020KY937).
Conflict of Interest
The authors declare that the research was conducted in the absence of any commercial or financial relationships that could be construed as a potential conflict of interest.
References
1. Courthod G, Tucci M, Di Maio M, Scagliotti GV. Papillary Renal Cell Carcinoma: A Review of the Current Therapeutic Landscape. Crit Rev Oncol (2015) 96:100–12. doi: 10.1016/j.critrevonc.2015.05.008
2. Kuroda N, Ohe C, Kawakami F, Mikami S, Furuya M, Matsuura K, et al. Clear Cell Papillary Renal Cell Carcinoma: A Review. Int J Clin Exp Pathol (2014) 7:7312–8.
3. Chen Q, Cheng L, Li Q. The Molecular Characterization and Therapeutic Strategies of Papillary Renal Cell Carcinoma. Expert Rev Anticancer Ther (2019) 19:169–75. doi: 10.1080/14737140.2019.1548939
4. McDermott DF, Huseni MA, Atkins MB, Motzer RJ, Rini BI, Escudier B, et al. Clinical Activity and Molecular Correlates of Response to Atezolizumab Alone or in Combination With Bevacizumab Versus Sunitinib in Renal Cell Carcinoma. Nat Med (2018) 24:749–57. doi: 10.1038/s41591-018-0053-3
5. Rini BI, Battle D, Figlin RA, George DJ, Hammers H, Hutson T, et al. The Society for Immunotherapy of Cancer Consensus Statement on Immunotherapy for the Treatment of Advanced Renal Cell Carcinoma (RCC). J Immunother Cancer (2019) 7:354. doi: 10.1186/s40425-019-0813-8
6. Rouprêt M, Babjuk M, Burger M, Capoun O, Cohen D, Compérat EM, et al. European Association of Urology Guidelines on Upper Urinary Tract Urothelial Carcinoma: 2020 Update. Eur Urol (2021) 79:62–79. doi: 10.1016/j.eururo.2020.05.042
7. Huang R, Mao M, Lu Y, Yu Q, Liao L. A Novel Immune-Related Genes Prognosis Biomarker for Melanoma: Associated With Tumor Microenvironment. Aging (2020) 12:6966–80. doi: 10.18632/aging.103054
8. Zhang C, Zheng JH, Lin ZH, Lv HY, Ye ZM, Chen YP, et al. Profiles of Immune Cell Infiltration and Immune-Related Genes in the Tumor Microenvironment of Osteosarcoma. Aging (2020) 12:3486–501. doi: 10.18632/aging.102824
9. Bhattacharya S, Andorf S, Gomes L, Dunn P, Schaefer H, Pontius J, et al. Immport: Disseminating Data to the Public for the Future of Immunology. Immunol Res (2014) 58:234–9. doi: 10.1007/s12026-014-8516-1
10. Ritchie ME, Phipson B, Wu D, Hu Y, Law CW, Shi W, et al. Limma Powers Differential Expression Analyses for RNA-Sequencing and Microarray Studies. Nucleic Acids Res (2015) 43:e47. doi: 10.1093/nar/gkv007
11. Huang DW, Sherman BT, Tan Q, Kir J, Liu D, Bryant D, et al. DAVID Bioinformatics Resources: Expanded Annotation Database and Novel Algorithms to Better Extract Biology From Large Gene Lists. Nucleic Acids Res (2007) 35:W169–75. doi: 10.1093/nar/gkm415
12. Zhao C, Sahni S. String Correction Using the Damerau-Levenshtein Distance. BMC Bioinf (2019) 20:277. doi: 10.1186/s12859-019-2819-0
13. Chandrashekar DS, Bashel B, Balasubramanya SAH, Creighton CJ, Ponce-Rodriguez I, Chakravarthi B, et al. UALCAN: A Portal for Facilitating Tumor Subgroup Gene Expression and Survival Analyses. Neoplasia (N Y NY) (2017) 19:649–58. doi: 10.1016/j.neo.2017.05.002
14. Unberath P, Knell C, Prokosch HU, Christoph J. Developing New Analysis Functions for a Translational Research Platform: Extending the Cbioportal for Cancer Genomics. Stud Health Technol Inf (2019) 258:46–50.
15. Bonneville R, Krook MA, Kautto EA, Miya J, Wing MR, Chen HZ, et al. Landscape of Microsatellite Instability Across 39 Cancer Types. JCO Precis Oncol (2017) 2017:PO.17.00073. doi: 10.1200/po.17.00073
16. Li J, Cao F, Yin HL, Huang ZJ, Lin ZT, Mao N, et al. Ferroptosis: Past, Present and Future. Cell Death Dis (2020) 11:88. doi: 10.1038/s41419-020-2298-2
17. Yuan H, Yan M, Zhang G, Liu W, Deng C, Liao G, et al. Cancersea: A Cancer Single-Cell State Atlas. Nucleic Acids Res (2019) 47:D900–8. doi: 10.1093/nar/gky939
18. Li T, Fan J, Wang B, Traugh N, Chen Q, Liu JS, et al. TIMER: A Web Server for Comprehensive Analysis of Tumor-Infiltrating Immune Cells. Cancer Res (2017) 77:e108–10. doi: 10.1158/0008-5472.Can-17-0307
19. Miao YR, Zhang Q, Lei Q, Luo M, Xie GY, Wang H, et al. Immucellai: A Unique Method for Comprehensive T-Cell Subsets Abundance Prediction and Its Application in Cancer Immunotherapy. Advanced Sci (Weinheim Baden-Wurttemberg Germany) (2020) 7:1902880. doi: 10.1002/advs.201902880
20. Qiu S, Nikolaou S, Fiorentino F, Rasheed S, Darzi A, Cunningham D, et al. Exploratory Analysis of Plasma Neurotensin as a Novel Biomarker for Early Detection of Colorectal Polyp and Cancer. Hormones Cancer (2019) 10:128–35. doi: 10.1007/s12672-019-00364-3
21. Akter H, Park M, Kwon OS, Song EJ, Park WS, Kang MJ. Activation of Matrix Metalloproteinase-9 (MMP-9) by Neurotensin Promotes Cell Invasion and Migration Through ERK Pathway in Gastric Cancer. Tumour Biol J Int Soc Oncodevelopmental Biol Med (2015) 36:6053–62. doi: 10.1007/s13277-015-3282-9
22. Ye Y, Long X, Zhang L, Chen J, Liu P, Li H, et al. NTS/NTR1 Co-Expression Enhances Epithelial-to-Mesenchymal Transition and Promotes Tumor Metastasis by Activating the Wnt/β-Catenin Signaling Pathway in Hepatocellular Carcinoma. Oncotarget (2016) 7:70303–22. doi: 10.18632/oncotarget.11854
23. Gil-Kulik P, Świstowska M, Kondracka A, Chomik P, Krzyżanowski A, Kwaśniewska A, et al. Increased Expression of BIRC2, BIRC3, and BIRC5 From the IAP Family in Mesenchymal Stem Cells of the Umbilical Cord Wharton’s Jelly (WJSC) in Younger Women Giving Birth Naturally. Oxid Med Cell Longevity (2020) 2020:9084730. doi: 10.1155/2020/9084730
24. Sasso E, Froechlich G, Cotugno G, D’Alise AM, Gentile C, Bignone V, et al. Replicative Conditioning of Herpes Simplex Type 1 Virus by Survivin Promoter, Combined to ERBB2 Retargeting, Improves Tumour Cell-Restricted Oncolysis. Sci Rep (2020) 10:4307. doi: 10.1038/s41598-020-61275-w
25. Eslami F, Mahdavi M, Babaei E, Hussen BM, Mostafavi H, Shahbazi A, et al. Down-Regulation of Survivin and Bcl-2 Concomitant With the Activation of Caspase-3 as a Mechanism of Apoptotic Death in KG1a and K562 Cells Upon Exposure to a Derivative From Ciprofloxacin Family. Toxicol Appl Pharmacol (2020) 409:115331. doi: 10.1016/j.taap.2020.115331
26. Wang Y, Song EC, Resnick MB. Elastin in the Tumor Microenvironment. Adv Exp Med Biol (2020) 1272:1–16. doi: 10.1007/978-3-030-48457-6_1
27. Tutar N, Yetkin NA, Yazıcı C, Önal Ö., Kontaş O, Keleştemur F. Clinical Significance of Progastrin-Releasing Peptide, Neuron-Specific Enolase, Chromogranin a, and Squamous Cell Cancer Antigen in Pulmonary Neuroendocrine Tumors. Turkish J Med Sci (2019) 49:774–81. doi: 10.3906/sag-1810-147
28. Eissa N, Hussein H, Kermarrec L, Ali AY, Marshall A, Metz-Boutigue MH, et al. Chromogranin-a Regulates Macrophage Function and the Apoptotic Pathway in Murine DSS Colitis. J Mol Med (Berlin Germany) (2018) 96:183–98. doi: 10.1007/s00109-017-1613-6
Keywords: kidney papillary cell carcinoma, immune-related, genes, prognostic, signature
Citation: Shen J, Wang R, Chen Y, Fang Z, Tang J, Yao J, Ling Y, Zhang L and Zhang X (2021) An Immune-Related Signature Predicted Survival in Patients With Kidney Papillary Cell Carcinoma. Front. Oncol. 11:670047. doi: 10.3389/fonc.2021.670047
Received: 20 February 2021; Accepted: 08 April 2021;
Published: 07 June 2021.
Edited by:
Matteo Ferro, European Institute of Oncology (IEO), ItalyReviewed by:
Daniela Terracciano, University of Naples Federico II, ItalyBiagio Barone, University of Naples Federico II, Italy
Copyright © 2021 Shen, Wang, Chen, Fang, Tang, Yao, Ling, Zhang and Zhang. This is an open-access article distributed under the terms of the Creative Commons Attribution License (CC BY). The use, distribution or reproduction in other forums is permitted, provided the original author(s) and the copyright owner(s) are credited and that the original publication in this journal is cited, in accordance with accepted academic practice. No use, distribution or reproduction is permitted which does not comply with these terms.
*Correspondence: Lisha Zhang, zls688757@163.com