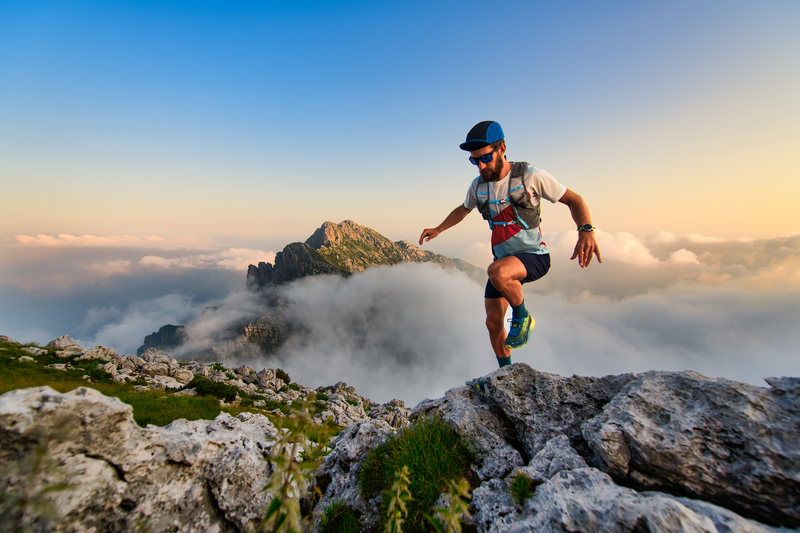
95% of researchers rate our articles as excellent or good
Learn more about the work of our research integrity team to safeguard the quality of each article we publish.
Find out more
EDITORIAL article
Front. Nutr.
Sec. Nutrition and Food Science Technology
Volume 12 - 2025 | doi: 10.3389/fnut.2025.1596699
This article is part of the Research Topic Defining the Role of Artificial Intelligence (AI) in the Food Sector and its Applications View all 13 articles
The final, formatted version of the article will be published soon.
You have multiple emails registered with Frontiers:
Please enter your email address:
If you already have an account, please login
You don't have a Frontiers account ? You can register here
One of the most promising applications of AI in the food sector lies in its ability to improve agricultural practices. In the study by Alhammad et al., deep learning and explainable AI were employed for the classification of potato leaf diseases. By employing advanced machine learning techniques, the authors showed how AI could identify and classify diseases in potato crops, offering a precise and scalable solution to disease detection. This method significantly enhances early intervention and crop protection, improving overall agricultural productivity. Similarly, Mayo et al. utilized deep learning models to detect maize diseases, such as maize streak virus and maize lethal necrosis, in Tanzania. Their findings demonstrate how AI-driven diagnostic tools can revolutionize crop disease management, offering faster and more accurate identification than traditional methods. These AI systems not only detect diseases early but can also predict their spread, contributing to more effective and sustainable crop management. The work by Taniguchi et al. further exemplifies AI's role in improving agricultural productivity. Their study focused on phenology analysis using UAVs (unmanned aerial vehicles) in a MAGIC rice population, allowing for trait prediction across different transplanting protocols. By combining drone technology with AI, the researchers were able to monitor rice growth and predict crop outcomes with greater accuracy, a critical factor in enhancing yield prediction and resource allocation in agriculture.Food waste remains a critical issue, with significant environmental and economic implications. Clark et al. explored the potential of AI-driven food waste management strategies in the hospitality industry, proposing that these techniques could be applied to household settings. Their study suggests that AI could streamline waste management processes, leading to reduced food wastage and improved sustainability in both commercial and household environments. By utilizing AI to predict consumption patterns, food waste could be minimized, and resources could be better allocated.AI's role extends beyond technological advancements; it also has the potential to drive inclusive and responsible innovation. Ozor et al. highlighted the importance of genderinclusive AI innovations in enhancing agriculture and food systems in Africa. Their study, which examines insights from the AI4AFS network, emphasizes how AI can empower marginalized communities, particularly women in agricultural sectors, by providing them with better access to data and resources that improve food security and agricultural sustainability.The recognition and analysis of food images have emerged as key areas for AI development, facilitating the digitalization of food systems. Dai explored a deep learning-based refrigerator food recognition system that can identify and categorize various food items, helping consumers manage their food more effectively and reduce waste. This system utilizes AI to automatically detect food items, analyze their condition, and offer suggestions for usage or disposal, making it a powerful tool for smart kitchens. Similarly, Wang et al. introduced a novel Swin Transformer approach to nutritional composition analysis in food images. Their work enables the accurate assessment of nutritional information by analyzing food images, thus providing consumers with valuable insights into their dietary choices without the need for manual input. This AI-driven method can help promote healthier eating habits and assist in the personalized nutrition field.AI's ability to integrate diverse data sources is another valuable asset in the food sector. Kierdorf et al. examined how image time series data could be used to predict cauliflower harvest readiness. By combining multiple data streams, including visual and temporal information, their AI model was able to accurately predict the optimal harvest time, ensuring better food quality and reduced waste. In another notable study, Kyalo et al. developed a decision support system using convolutional neural networks (CNN) that integrates image and numerical data to improve the farming of edible crickets as a sustainable food source. Their AI-driven system is designed to optimize farming conditions and predict cricket growth, demonstrating AI's potential to enhance the sustainability of alternative protein sources. Ali presents a Bayesian model designed to optimize wheat tilling practices in response to climatic challenges and sustainability concerns. The model integrates weather data, soil conditions, and crop growth patterns to provide actionable insights for farmers, helping them make informed decisions on when and how to till the soil for maximum yield. By addressing both environmental and sustainability aspects, the research offers a robust AI-driven approach to improve wheat farming, which is crucial for global food security. The study underscores the importance of leveraging AI to adapt traditional agricultural practices to the challenges posed by climate change.AI is not limited to food production and waste management; it also plays a critical role in understanding and improving the food environment. Etheredge et al. employed large language models (LLMs) to enhance food environment data by analyzing food store names and attributes. This application highlights AI's capability to mine vast amounts of unstructured data, leading to better insights into food availability, consumer behavior, and market trends. Furthermore, Ogrinc et al. applied AI for food named-entity recognition and linking, leveraging ChatGPT in zero-shot evaluations. Their study underscores how AI-powered tools can automatically recognize and link food-related entities, facilitating the creation of more efficient food databases and contributing to better decision-making in the food industry.The contributions to this special issue illustrate the transformative potential of AI in the food sector. From disease detection in crops to the optimization of food recognition systems, AI is paving the way for smarter, more sustainable food systems. As AI technologies continue to advance, they will undoubtedly play an even more prominent role in tackling global challenges such as food security, waste reduction, and sustainable agriculture. The studies presented here show the vast range of applications AI can have across various stages of the food supply chain, from production to consumption. With continued innovation and the responsible integration of AI, the future of the food sector holds the promise of more efficient, equitable, and sustainable food systems worldwide.
Keywords: food secuity, Data integration algorithm, Food waste management, Agriculture sustainability, nutrition, Crop management and monitoring, Decisioion making, Machin learning
Received: 20 Mar 2025; Accepted: 24 Mar 2025.
Copyright: © 2025 Belwal, Huang and Zhao. This is an open-access article distributed under the terms of the Creative Commons Attribution License (CC BY). The use, distribution or reproduction in other forums is permitted, provided the original author(s) or licensor are credited and that the original publication in this journal is cited, in accordance with accepted academic practice. No use, distribution or reproduction is permitted which does not comply with these terms.
* Correspondence:
Tarun Belwal, Texas A and M University, College Station, United States
Disclaimer: All claims expressed in this article are solely those of the authors and do not necessarily represent those of their affiliated organizations, or those of the publisher, the editors and the reviewers. Any product that may be evaluated in this article or claim that may be made by its manufacturer is not guaranteed or endorsed by the publisher.
Research integrity at Frontiers
Learn more about the work of our research integrity team to safeguard the quality of each article we publish.