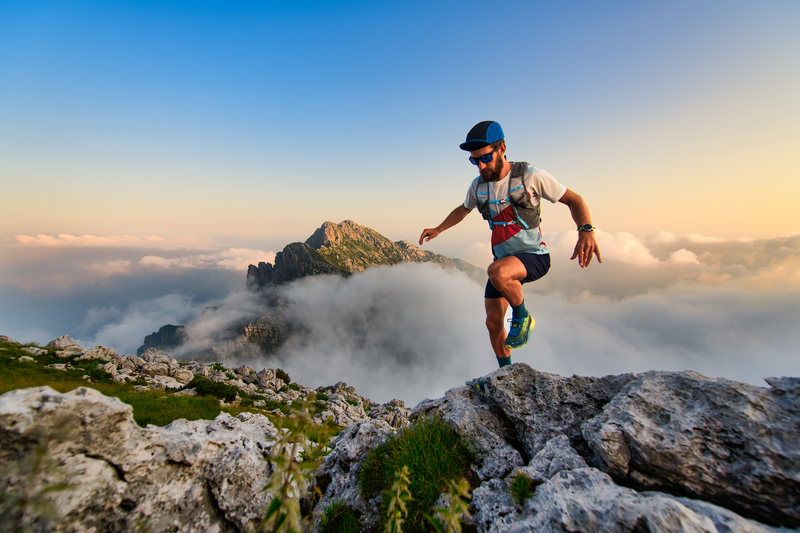
95% of researchers rate our articles as excellent or good
Learn more about the work of our research integrity team to safeguard the quality of each article we publish.
Find out more
ORIGINAL RESEARCH article
Front. Nutr.
Sec. Food Chemistry
Volume 12 - 2025 | doi: 10.3389/fnut.2025.1582576
This article is part of the Research Topic Applications of metabolomics in the formation of food flavor View all 3 articles
The final, formatted version of the article will be published soon.
You have multiple emails registered with Frontiers:
Please enter your email address:
If you already have an account, please login
You don't have a Frontiers account ? You can register here
Colored onions are preferred by consumers for their unique aroma, abundant phytochemicals and various biological activities. The total phenols, flavonoids, anthocyanins, carotenoids, and antioxidant activities of three colored onion bulbs were evaluated in this study, and their volatile metabolites were further detected via headspace solid-phase microextraction combined with gas chromatography-mass spectrometry (HS-SPME/GC-MS). The results showed that the major phytochemicals and antioxidant activities varied among colored onion bulbs. A total of 243 volatile metabolites were detected, comprising sulfur compounds (51%-64%), organic acids and their derivatives (4%-19%), and others. Multivariate statistical analysis revealed significant variations in the volatile metabolites of three pigmented onions, and 19 characteristic metabolites were identified as biomarkers. In addition, 33 and 38 feature metabolites were also extracted via the SelectKBest and LASSO methods, respectively, and 38 features selected via Lasso can effectively distinguish the three colored onions via PCA, uniform manifold approximation and projection (UMAP), and the k-means algorithm. Four machine learning models were further used for the classification of colored onions, and the random forest model was the optimum model, with a classification accuracy of 1.00. Furthermore, SHAP analysis confirmed that 20 features could be potential markers for representing the overall metabolites. These results imply that HS-SPME/GC-MS combined with random forest could be a novel and powerful method for extracting important features of volatile metabolites in colored onion bulbs.
Keywords: Pigmented onion, Volatile flavor, Metabolomics, machine learning models, HS-SPME-GC-MS
Received: 24 Feb 2025; Accepted: 02 Apr 2025.
Copyright: © 2025 Cheng, Xiao, He, Yang, Pei, Jin and Abd El-Aty. This is an open-access article distributed under the terms of the Creative Commons Attribution License (CC BY). The use, distribution or reproduction in other forums is permitted, provided the original author(s) or licensor are credited and that the original publication in this journal is cited, in accordance with accepted academic practice. No use, distribution or reproduction is permitted which does not comply with these terms.
* Correspondence:
Wengang Jin, Shaanxi University of Technology, Hanzhong, Shaanxi, China
A. M. Abd El-Aty, Cairo University, Giza, 12613, Giza, Egypt
Disclaimer: All claims expressed in this article are solely those of the authors and do not necessarily represent those of their affiliated organizations, or those of the publisher, the editors and the reviewers. Any product that may be evaluated in this article or claim that may be made by its manufacturer is not guaranteed or endorsed by the publisher.
Research integrity at Frontiers
Learn more about the work of our research integrity team to safeguard the quality of each article we publish.