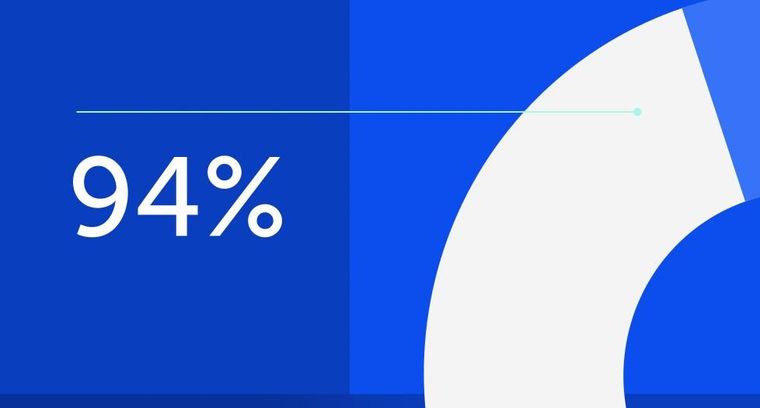
94% of researchers rate our articles as excellent or good
Learn more about the work of our research integrity team to safeguard the quality of each article we publish.
Find out more
ORIGINAL RESEARCH article
Front. Nutr., 10 April 2025
Sec. Nutrition and Microbes
Volume 12 - 2025 | https://doi.org/10.3389/fnut.2025.1573249
This article is part of the Research TopicDietary Modulation of Gut Microbiota-X axisView all 10 articles
Objective: To explore the correlation of dietary index for gut microbiota (DI-GM) with non-alcoholic fatty liver disease (NAFLD).
Methods: Data of 6,711 participants were extracted from the National Health and Nutrition Examination Survey (NHANES) during 2007–2018. A weighted logistic regression analysis was employed for assessment of the correlation of DI-GM with NAFLD, and a restricted cubic spline (RCS) analysis was implemented to examine potential non-linear associations. Subgroup analyses were conducted to identify particularly susceptible groups. Additionally, the synergistic effects of different DI-GM components on NAFLD risk was assessed by weighted quantile sum (WQS) regression.
Results: The DI-GM exhibited statistically significant correlation with NAFLD [OR (95%CI):0.91 (0.85, 0.98), p = 0.015]. The results of the RCS analysis indicated a linear correlation of DI-GM and NAFLD (p = 0.810 for non-linearity). Further stratified analyses indicated that the negative correlation of DI-GM with NAFLD were significant and consistent for all subgroups. The results of WQS regression revealed that soybean (27%), refined grains (17%), coffee (16%), and red meat (9%) had the highest contribution weights to NAFLD.
Conclusion: As an important tool for assessment of the influences of diet on gut microbiota, DI-GM is negatively correlated with NAFLD risk factors. Soybean, refined grains, coffee, and red meat are key factors influencing NAFLD. The direct correlation of DI-GM with NAFLD shall be explored and the effectiveness of prevention and treatment of NAFLD shall be evaluated by improving DI-GM scores via dietary interventions.
As a liver disease correlated to metabolic disorders, non-alcoholic fatty liver disease (NAFLD) is characterized by abnormal fat accumulation in liver induced by factors other than alcohol consumption (1–3). NAFLD comprises non-alcoholic fatty liver (NAFL) and non-alcoholic steatohepatitis (NASH), and it may further progress to hepatocellular carcinoma (1, 4–6). The incidence of NAFLD is approximately 38% globally, with regional variations ranging from 25.10% in Western Europe to 44.37% in Latin America (7). The prevalence of NAFLD and NASH in key regions such as China and USA is expected to increase by up to 30–56% from 2016 to 2030 (8). NAFLD has been demonstrated to be correlated with various factors, including insulin resistance (9, 10), lipotoxicity (11), inflammatory response (12), genetic polymorphisms (13), epigenetics (14), adipokines (15), myokines (15), hepatokines (15, 16), bile acids (17, 18), and gut microbiota (19–22).
Gut microbiota (GM), also known as the gut flora, refers to the microbial community in human intestine, and it plays a significant role in digestion and metabolism (23–25). Gut microbiota coexists symbiotically with the human body as the “second genome” (22). Previous studies have shown that GM is a dominant factor influencing incidence and progression of NAFLD (19–22). Diet determines the composition and diversity of GM (26–28). Furthermore, dietary interventions that can alter gut microbiota have attracted great attention (28). Kase et al. (26) reported a review of 14 dietary components with different influences on gut microbiota. The Dietary Index for the Gut Microbiota (DI-GM) was proposed for assessment of dietary quality associated with gut microbiota (26). Contrary to other dietary indices such as healthy eating index-2015 (HEI-2015) and alternative healthy eating index-2010 (AHEI-2010), DI-GM was established on the basis of gut microbiota but not food groups. Moreover, DI-GM had a positive correlation with urinary levels of intestinal diols and lactones, both of which are markers of gut microbiota diversity, indicating that DI-GM is associated with the diversity of GM (26). Hence, DI-GM can be used to effectively evaluate the influences of dietary patterns on GM (26) and serve as a standardized tool for diet assessment. Recent studies have shown that high DI-GM indicates low risk of accelerated aging (29), and DI-GM is inversely related to the prevalence of depression (30). Furthermore, compelling evidences have revealed that gut microbiota generates a variety of bioactive substances that interact with the host liver cells through the portal vein, which may lead to inflammation and further liver damage (31). However, the specific correlation of DI-GM with NAFLD remains unclear.
In this study, the correlation of DI-GM and NAFLD was investigated on the basis of the data extracted from the National Health and Nutrition Examination Survey (NHANES), and the potential of DI-GM which has been widely used for diet assessment for GM in prevention and dietary treatment of NAFLD was explored.
Information acquired from public files for NHANES data cycles in 2007–2018 was analyzed. Across these cycles, 40,959 participants were enrolled, while the final cohort comprised 6,711 subjects only (Figure 1) as participants who provided incomplete data of fatty liver index (FLI) (n = 23,670), incomplete data of DI-GM (n = 845), with viral hepatitis (n = 315), excessive alcohol consumption (n = 4,527), or incomplete data for any covariate (n = 4,891) were excluded.
The FLI was employed for non-invasive diagnosis of NAFLD (32), and its accuracy and clinical significance in screening and diagnosis of NAFLD have been demonstrated in various studies (33). The FLI can be determined by: FLI = (e0.953 × loge [Triglycerides (TG)] + 0.139 × Body mass index (BMI) + 0.718 × loge [γ-glutamyltransferase (GGT)] + 0.053 × Waist circumference (WC) – 15.745)/(1 + e0.953 × loge [TG] + 0.139 × BMI + 0.718 × loge [GGT] + 0.053 × WC – 15.745) × 100, and the FLI of 60 or higher indicates a high risk of NAFLD (32).
Fourteen food or nutrients constituting key elements of DI-GM were identified (26). Beneficial elements include chickpeas, avocados, coffee, broccoli, fermented dairy products, cranberries, soybean, green tea, fiber, and whole grains. Meanwhile, detrimental elements such as refined grains, processed meat, red meat, and a high-fat diet were identified (26). The DI-GM was determined on the basis of the dietary recall data of NHANES 2007–2018, as shown in Supplementary Table S2. For food items with positive impacts on gut microbiota, 1 point was assigned when the gender-specific median was reached for intake, otherwise 0; for food items with negative impacts on gut microbiota, 0 was assigned when the gender-specific median was reached for intake or it accounts for 40% of total caloric intake (for high-fat diets), otherwise 1 point (26). A DI-GM score ranging from 0 to 13 (ranging from 0 to 9 for promoting the gut microbiota and 0 to 4 for negatively affecting the gut microbiota) was determined based on these scores, and then the participants were classified into Groups A (0–3), B (4), C (5) and D (≥6) (30), as presented in Supplementary Table S1.
In this survey, several potential confounding factors were considered: (1) Demographic factors: age, gender (male/female), race, marital status, and education. (2) Lifestyle factors: Smoking history (never, now, former). (3) Financial status: family poverty income ratio (PIR) (low, middle, high). (4) Health comorbidities: hypertension, cardiovascular disease (CVD), and diabetes mellitus (DM). (5) BMI (kg/m2). (6) Liver function indicators: Alanine aminotransferase (ALT) and aspartate aminotransferase (AST) (both in U/L). Detailed information could be achieved in Supplementary Table S2.
Recommended sample weights were employed to enhance accuracy and undesired influences were mitigated by intricate multistage sampling. The results of categorical variables were denoted as weighted percentages, while the results of continuous variables were presented as weighted means ± standard deviations. To clarify the correlation of DI-GM and NAFLD, weighted multivariate logistic regression analyses were conducted. Herein, various confounding factors were considered and adjusted for. Three models were employed in this study. Model 1 was non-adjusted; Model 2 incorporated adjustments for key demographic variables; Model 3 additionally adjusted for BMI, PIR, smoking history, hypertension, DM, CVD, ALT and AST. Weighted restricted cubic spline (RCS) curves were introduced to Model 3 to investigate the correlation of DI-GM and NAFLD. Additionally, subgroup analyses were executed based on age, race, gender, education, marital status, smoking history, and common chronic diseases (hypertension and CVD). Considered the potential influences of physical activity, alcohol intake, medication use on the results. Accordingly, we further adjusted for these variables as outlined in Model 3 to assess the robustness of our findings in sensitivity analysis. Weighted quantile sum (WQS) regression models were employed for assessment of the synergistic effects of different DI-GM components on the risk of NAFLD, and the WQS index was determined on the basis of 60% training dataset, 40% validation dataset and 1,000 bootstrapping (34). To address the multicollinearity, weights were assigned to components according to their contributions to the results. The integrity of the statistical computations was validated using R software, with p < 0.05 denoting statistical significance.
Table 1 shows the characteristics of the 6,711 subjects enrolled grouped by DI-GM. The average age was 51.06 ± 0.30 years. 51.28% were male and 48.72% were female. Significant differences were detected (p < 0.05) across DI-GM for demographic characteristics, financial status (PIR), lifestyle (smoking history), physical well-being (NAFLD, hypertension, CVD, DM), and anthropometric measures (BMI).
Logistic modeling was executed to explore the association of DI-GM and NAFLD. According to Table 2, a significant negative correlation was identified [OR (95%CI): 0.88 (0.85, 0.92), p < 0.001], and the negative correlation remained robust after variable adjustment [OR (95%CI):0.91 (0.85, 0.98), p = 0.015] in Model 3, suggesting a 9% relative risk reduction per DI-GM unit which is modest at the individual level and meaningful at the population level. Furthermore, slight increase in DI-GM could also offer significant benefits for NAFLD, especially for the implementation of large scale and high-risk populations strategies. Meanwhile, the percentage of participants with DI-GM ≥ 6 had a significantly negative correlation with NAFLD [OR (95%CI):0.59 (0.48, 0.71), p < 0.001], and the negative correlation remained robust after variable adjustment [OR (95%CI):0.65 (0.47, 0.90), p = 0.010] in Model 3.
To explore the non-linearity of the associations of DI-GM and the NAFLD, a weighted multivariable–adjusted RCS analysis was executed in this study. As shown in Figure 2, no non-linear correlation of DI-GM and NAFLD was detected (p = 0.810 for non-linearity), and a negative dose–response correlation of DI-GM and NAFLD was identified (p = 0.003).
Figure 2. Association between DI-GM with NAFLD. Adjustment factors included age, sex, race, education attainment, marital status, BMI, PIR, smoking status, hypertension, DM, CVD, ALT and AST.
In this study, subgroup analysis was used to explore the specific correlations of DI-GM and NAFLD in different subgroups, indicating that the negative correlation of DI-GM and NAFLD was consistent (Figure 3). The results of the sensitivity analyses indicate that, even after further adjusting for physical activity, alcohol intake, medication use, the positive correlation between DI-GM and NAFLD persists (Supplementary Table S3).
Figure 3. Subgroup analyses between DI-GM with NAFLD. Each subgroup analysis adjusted for age, sex, race, education attainment, marital status, BMI, PIR, smoking status, hypertension, DM, CVD, ALT and AST.
A weighted index was developed based on the WQS regression to assess the impacts and weight contributions of different DI-GM components on NAFLD (Figure 4). Herein, consistent variable adjustments were applied. The results demonstrated that soybean (27%), refined grains (17%), coffee (16%) and red meat (9%) had high weight contributions, while fiber (0%) and whole grains (0%) had low weight contributions.
Figure 4. Weighted quantile sum (WQS) model regression index weights for the NAFLD, adjusted for age, sex, race, education attainment, marital status, BMI, PIR, smoking status, hypertension, DM, CVD, ALT and AST.
As demonstrated, DI-GM remained an independent risk factor for NAFLD after variable control. According to the RCS curves, DI-GM had negligible non-linear, dose-dependent association with NAFLD. Additionally, DI-GM was negatively related to the probability of NAFLD, suggesting that DI-GM can serve as an effective indicator for NAFLD, and adjustment of the diet structure could aid in preventing NAFLD.
In previous studies, DI-GM has been found to be associated with biological age through the mediation role of body mass index, while biological age is linked with various biomarkers possibly in relation with NAFLD (29). After consulting relevant literature, gut microbiota can interact with the pathogenesis of NAFLD by various mechanisms: (1) gut microbiota translocation: dysbiosis of gut microbiota induces translocation of bacteria or their metabolites from gut to liver, causing liver inflammation and damage (35–38); (2) production of endogenous ethanol: gut microbiota can produce endogenous ethanol, which may affect the metabolism and inflammatory response of liver (36, 39–44); (3) abnormal regulation of bile acid and choline metabolism: gut microbiota participates in the metabolism of bile acids and choline, and changes in these metabolites may affect liver health (22, 36, 41, 45–48); (4) endotoxemia: dysbiosis of gut microbiota could result in increased level of endotoxins (e.g., lipopolysaccharides), resulting in inflammation and insulin resistance (36, 49–53). Overall, GM is closely related to NAFLD, with a two-way influence through the gut-liver axis regulatory mechanism (22, 35, 36, 46, 49, 54–57). Therefore, a balanced gut microbiota helps maintain hepatic homeostasis, while disruption of GM may promote the incidence and progression of NAFLD.
The associations of various dietary indicators and the NAFLD have been thoroughly investigated. It has been demonstrated that specific dietary patterns could significantly reduce the risk of MASLD and relevant liver fibrosis (MASLD-LF) (58). Meanwhile, high scores of the HEI or the AHEI were significantly correlated with low incidence of MAFLD (59), and high-quality diets led to low mortality rates of MAFLD (60). To date, several dietary assessment tools, including HEI and AHEI, have been employed to evaluate dietary quality. Nevertheless, they barely considered the specific correlations of dietary components with gut microbiota, which are essentially important for liver health (61).
The DI-GM is distinctive in that it specifically examines the impact of the gut microbiota, addressing a gap in existing dietary indices. Based on the integrated effects of 14 key dietary components on gut microbiota, the DI-GM’s structured approach not only offers insights into dietary impacts on the microbiota but also reflects overall dietary quality (26). The DI-GM provides an assessment framework, making it exceedingly valuable for exploration of the relationship between microbiota and metabolic health. Its correlation with the HEI exemplifies the dual applicability and scientific relevance of the DI-GM in assessing nutritional status and gut microbiota (26). Therefore, future dietary interventions could leverage the DI-GM to optimize dietary structures, thereby improving the conditions of GM and relieving NAFLD.
The results of the WQS regression model indicate that soy, refined grains, coffee, and red meat are key food components influencing NAFLD. Among them, soybean and coffee, as beneficial components of the DI-GM, may play critical roles in the prevention and management of NAFLD. Indeed, soybean can regulate lipid metabolism and oxidative stress, thus protecting the liver of NAFLD patients (62). Consequently, soybean may serve as an effective dietary intervention for NAFLD (62). Some studies claimed that coffee consumption could reduce the risk of NAFLD (63), while conflicting results regarding its impact on prevention of NAFLD have also been reported. For NAFLD patients, coffee intake can relieve liver fibrosis (64). On the contrary, refined grains and red meat, as detrimental components of the DI-GM, may elevate the risk of NAFLD. Indeed, the consumption of refined grains led to increased incidence of NAFLD, while the intake of whole grains may positively affect the clinical conditions of NAFLD patients and relieve disease progression (65). Furthermore, the consumption of red meat has been confirmed to have a positive correlation with NAFLD risk (66). Eating one serving of legumes per week instead of red or processed meats or poultry led to reduced incidence of NAFLD (67). However, the mechanism by which these foods influence the pathogenesis of NAFLD by modulating gut microbiota remains unclear. Moreover, the content of GM in dietary fiber and whole grains were relatively low, which may lead to the 0% of dietary fiber and whole grains of the DI-GM in the contributions of NAFLD.
This study provides epidemiological evidence supporting the inverse association between DI-GM scores and NAFLD prevalence. These findings underscore the potential utility of DI-GM optimization as a preventive strategy in metabolic health management. To advance clinical translation, future longitudinal investigations should employ standardized DI-GM assessments across diverse cohorts to establish causal relationships, validate risk prediction models, and facilitate early risk stratification in susceptible populations.
This study made contributions in several aspects. First, a broad cross-sectional approach leveraging NHANES data was used for the first time, revealing a linear negative correlation of the DI-GM related to GM diversity and NAFLD. Second, sampling weights, variable adjustment, and statistical tools were involved, resulting in significantly improved precision and robustness. Third, a WQS regression model was employed to evaluate the overall impacts and their weights of different DI-GM components on NAFLD.
Nevertheless, this study has several limitations: (1) the causality of DI-GM and NAFLD cannot be determined due to the cross-sectional approach used; (2) the findings may not be applicable for other populations; (3) recall bias arises from self-reported 24-h dietary records, which weakens the reliability of our results; (4) the confounding effects induced by measurement errors in unknown confounding factors or unmeasured variables cannot be completely ruled out.
DI-GM can effectively assess the influences of diet on GM, and it is negatively correlated with NAFLD risk. Soybean, refined grains, coffee, and red meat are key food components influencing NAFLD. Future studies may focus on the direct correlation of DI-GM with NAFLD, assessment of the effectiveness of prevention and treatment of NAFLD by improving DI-GM scores through dietary interventions.
Publicly available datasets were analyzed in this study. This data can be found: the official website of the National Center for Health Statistics (URL: https://www.cdc.gov/nchs/nhanes).
This study utilized anonymized data from the publicly accessible NHANES database, fundamentally ensuring the privacy and safety of the research subjects. The study has undergone strict ethical approval by the National Center for Health Statistics Ethics Review Committee, which conducted a comprehensive assessment and review of the research plan in accordance with internationally accepted ethical guidelines and regulatory requirements. Moreover, this study strictly adhered to the norms established by the Declaration of Helsinki, ensuring ethical compliance throughout the research process. Importantly, all participants in the NHANES project signed legally binding and ethically binding written consent forms before participating in the study. This consent procedure was completed properly and in strict accordance with standard processes before the initiation of data collection, ensuring that the research subjects were fully informed about the purpose, methods, risks, and benefits of the study through a comprehensive information disclosure and communication mechanism, and that they explicitly consented to participate in the survey on a fully voluntary and autonomous basis. The studies were conducted in accordance with the local legislation and institutional requirements. Written informed consent for participation was not required from the participants or the participants' legal guardians/next of kin in accordance with the national legislation and institutional requirements.
YW: Writing – original draft, Writing – review & editing, Data curation, Formal analysis, Methodology. BZ: Writing – review & editing, Supervision, Validation, Funding acquisition. LF: Writing – review & editing, Supervision, Project administration, Validation. CC: Writing – review & editing, Funding acquisition, Project administration, Supervision, Validation. XF: Data curation, Formal analysis, Investigation, Writing – original draft, Methodology, Resources, Software, Visualization.
The author(s) declare that financial support was received for the research and/or publication of this article. This study received financial support from the Jiaxing City Provincial and Municipal Co-construction Cultivation Discipline (Oncology, Project Number: 2023-SGJ-001) and the Medical Health Science and Technology Project of Zhejiang Provincial Health Commission (Project Number: 2022KY1246) during its implementation.
The smooth progress of this study would not have been possible without the valuable support of the National Health and Nutrition Examination Survey (NHANES) program of the United States. The comprehensive database and diverse resources provided by this project laid a solid foundation for our research and offered critical support, becoming the core elements that enabled the implementation of this study. At the same time, we would like to express our heartfelt thanks to all participants who contributed their datasets and to every volunteer who selflessly provided their personal information and participated in this study. Without the collaborative efforts and strong support of all parties involved, this research would have been difficult to advance due to the lack of necessary conditions.
The authors declare that the research was conducted in the absence of any commercial or financial relationships that could be construed as a potential conflict of interest.
The author(s) declare that no Gen AI was used in the creation of this manuscript.
All claims expressed in this article are solely those of the authors and do not necessarily represent those of their affiliated organizations, or those of the publisher, the editors and the reviewers. Any product that may be evaluated in this article, or claim that may be made by its manufacturer, is not guaranteed or endorsed by the publisher.
The Supplementary material for this article can be found online at: https://www.frontiersin.org/articles/10.3389/fnut.2025.1573249/full#supplementary-material
1. Powell, EE, Wong, VW, and Rinella, M. Non-alcoholic fatty liver disease. Lancet. (2021) 397:2212–24. doi: 10.1016/S0140-6736(20)32511-3
2. Chalasani, N, Younossi, Z, Lavine, JE, Charlton, M, Cusi, K, Rinella, M, et al. The diagnosis and management of nonalcoholic fatty liver disease: Practice guidance from the American Association for the Study of Liver Diseases. Hepatology. (2018) 67:328–57. doi: 10.1002/hep.29367
3. Estes, C, Razavi, H, Loomba, R, Younossi, Z, and Sanyal, AJ. Modeling the epidemic of nonalcoholic fatty liver disease demonstrates an exponential increase in burden of disease. Hepatology. (2018) 67:123–33. doi: 10.1002/hep.29466
4. Younossi, Z, Anstee, QM, Marietti, M, Hardy, T, Henry, L, Eslam, M, et al. Global burden of NAFLD and NASH: trends, predictions, risk factors and prevention. Nat Rev Gastroenterol Hepatol. (2018) 15:11–20. doi: 10.1038/nrgastro.2017.109
5. Huang, DQ, El-Serag, HB, and Loomba, R. Global epidemiology of NAFLD-related HCC: trends, predictions, risk factors and prevention. Nat Rev Gastroenterol Hepatol. (2021) 18:223–38. doi: 10.1038/s41575-020-00381-6
6. Parola, M, and Pinzani, M. Liver fibrosis in NAFLD/NASH: from pathophysiology towards diagnostic and therapeutic strategies. Mol Asp Med. (2024) 95:101231. doi: 10.1016/j.mam.2023.101231
7. Younossi, ZM, Golabi, P, Paik, JM, Henry, A, Van Dongen, C, and Henry, L. The global epidemiology of nonalcoholic fatty liver disease (NAFLD) and nonalcoholic steatohepatitis (NASH): a systematic review. Hepatology. (2023) 77:1335–47. doi: 10.1097/HEP.0000000000000004
8. Estes, C, Anstee, QM, Arias-Loste, MT, Bantel, H, Bellentani, S, Caballeria, J, et al. Modeling NAFLD disease burden in China, France, Germany, Italy, Japan, Spain, United Kingdom, and United States for the period 2016-2030. J Hepatol. (2018) 69:896–904. doi: 10.1016/j.jhep.2018.05.036
9. Marušić, M, Paić, M, Knobloch, M, and Liberati Pršo, AM. NAFLD, Insulin Resistance, and Diabetes Mellitus Type 2. Can J Gastroenterol Hepatol. (2021) 2021:6613827–9. doi: 10.1155/2021/6613827
10. Tanase, DM, Gosav, EM, Costea, CF, Ciocoiu, M, Lacatusu, CM, Maranduca, MA, et al. The Intricate Relationship between Type 2 Diabetes Mellitus (T2DM), Insulin Resistance (IR), and Nonalcoholic Fatty Liver Disease (NAFLD). J Diabetes Res. (2020) 2020:3920196–16. doi: 10.1155/2020/3920196
11. Rada, P, González-Rodríguez, Á, García-Monzón, C, and Valverde, ÁM. Understanding lipotoxicity in NAFLD pathogenesis: is CD36 a key driver? Cell Death Dis. (2020) 11:802. doi: 10.1038/s41419-020-03003-w
12. Hammerich, L, and Tacke, F. Hepatic inflammatory responses in liver fibrosis. Nat Rev Gastroenterol Hepatol. (2023) 20:633–46. doi: 10.1038/s41575-023-00807-x
13. Sulaiman, SA, Dorairaj, V, and Adrus, MNH. Genetic Polymorphisms and Diversity in Nonalcoholic Fatty Liver Disease (NAFLD): A Mini Review. Biomedicines. (2022) 11:106. doi: 10.3390/biomedicines11010106
14. Sodum, N, Kumar, G, Bojja, SL, Kumar, N, and Rao, CM. Epigenetics in NAFLD/NASH: Targets and therapy. Pharmacol Res. (2021) 167:105484. doi: 10.1016/j.phrs.2021.105484
15. Lu, Y. Editorial: The roles and mechanisms of hepatokines, adipokines and myokines in the development of non-alcoholic fatty liver disease (NAFLD). Front Endocrinol. (2022) 13:1074842. doi: 10.3389/fendo.2022.1074842
16. Stefan, N, Schick, F, Birkenfeld, AL, Häring, HU, and White, MF. The role of hepatokines in NAFLD. Cell Metab. (2023) 35:236–52. doi: 10.1016/j.cmet.2023.01.006
17. Rivera-Andrade, A, and Álvarez, CS. The importance of bile Acids in NAFLD: current evidence and future directions. Ann Hepatol. (2022) 27:100773. doi: 10.1016/j.aohep.2022.100773
18. Wang, S, Sheng, F, Zou, L, Xiao, J, and Li, P. Hyperoside attenuates non-alcoholic fatty liver disease in rats via cholesterol metabolism and bile acid metabolism. J Adv Res. (2021) 34:109–22. doi: 10.1016/j.jare.2021.06.001
19. Chen, J, and Vitetta, L. Gut Microbiota Metabolites in NAFLD Pathogenesis and Therapeutic Implications. Int J Mol Sci. (2020) 21:5214. doi: 10.3390/ijms21155214
20. Aron-Wisnewsky, J, Vigliotti, C, Witjes, J, Le, P, Holleboom, AG, Verheij, J, et al. Gut microbiota and human NAFLD: disentangling microbial signatures from metabolic disorders. Nat Rev Gastroenterol Hepatol. (2020) 17:279–97. doi: 10.1038/s41575-020-0269-9
21. Gómez-Pérez, AM, Ruiz-Limón, P, Salas-Salvadó, J, Vioque, J, Corella, D, Fitó, M, et al. Gut microbiota in nonalcoholic fatty liver disease: a PREDIMED-Plus trial sub analysis. Gut Microbes. (2023) 15:2223339. doi: 10.1080/19490976.2023.2223339
22. Fang, J, Yu, CH, Li, XJ, Yao, JM, Fang, ZY, Yoon, SH, et al. Gut dysbiosis in nonalcoholic fatty liver disease: pathogenesis, diagnosis, and therapeutic implications. Front Cell Infect Microbiol. (2022) 12:997018. doi: 10.3389/fcimb.2022.997018
23. Sommer, F, and Bäckhed, F. The gut microbiota--masters of host development and physiology. Nat Rev Microbiol. (2013) 11:227–38. doi: 10.1038/nrmicro2974
24. Nicholson, JK, Holmes, E, Kinross, J, Burcelin, R, Gibson, G, Jia, W, et al. Host-gut microbiota metabolic interactions. Science (New York, NY). (2012) 336:1262–7. doi: 10.1126/science.1223813
25. Dixit, K, Chaudhari, D, Dhotre, D, Shouche, Y, and Saroj, S. Restoration of dysbiotic human gut microbiome for homeostasis. Life Sci. (2021) 278:119622. doi: 10.1016/j.lfs.2021.119622
26. Kase, BE, Liese, AD, Zhang, J, Murphy, EA, Zhao, L, and Steck, SE. The Development and Evaluation of a Literature-Based Dietary Index for Gut Microbiota. Nutrients. (2024) 16:1045. doi: 10.3390/nu16071045
27. Beam, A, Clinger, E, and Hao, L. Effect of Diet and Dietary Components on the Composition of the Gut Microbiota. Nutrients. (2021) 13:2795. doi: 10.3390/nu13082795
28. Losno, EA, Sieferle, K, Perez-Cueto, FJA, and Ritz, C. Vegan Diet and the Gut Microbiota Composition in Healthy Adults. Nutrients. (2021) 13:2402. doi: 10.3390/nu13072402
29. An, S, Qin, J, Gong, X, Li, S, Ding, H, Zhao, X, et al. The mediating role of body mass index in the association between dietary index for gut microbiota and biological age: a study based on NHANES 2007-2018. Nutrients. (2024) 16:4164. doi: 10.3390/nu16234164
30. Zhang, X, Yang, Q, Huang, J, Lin, H, Luo, N, and Tang, H. Association of the newly proposed dietary index for gut microbiota and depression: the mediation effect of phenotypic age and body mass index. Eur Arch Psychiatry Clin Neurosci. (2024). doi: 10.1007/s00406-024-01912-x
31. Ji, Y, Yin, Y, Li, Z, and Zhang, W. Gut Microbiota-Derived Components and Metabolites in the Progression of Non-Alcoholic Fatty Liver Disease (NAFLD). Nutrients. (2019) 11:1712. doi: 10.3390/nu11081712
32. Bedogni, G, Bellentani, S, Miglioli, L, Masutti, F, Passalacqua, M, Castiglione, A, et al. The Fatty Liver Index: a simple and accurate predictor of hepatic steatosis in the general population. BMC Gastroenterol. (2006) 6:33. doi: 10.1186/1471-230X-6-33
33. Contreras, D, González-Rocha, A, Clark, P, Barquera, S, and Denova-Gutiérrez, E. Diagnostic accuracy of blood biomarkers and non-invasive scores for the diagnosis of NAFLD and NASH: Systematic review and meta-analysis. Ann Hepatol. (2023) 28:100873. doi: 10.1016/j.aohep.2022.100873
34. Yorita Christensen, KL, Carrico, CK, Sanyal, AJ, and Gennings, C. Multiple classes of environmental chemicals are associated with liver disease: NHANES 2003-2004. Int J Hyg Environ Health. (2013) 216:703–9. doi: 10.1016/j.ijheh.2013.01.005
35. Albillos, A, de Gottardi, A, and Rescigno, M. The gut-liver axis in liver disease: Pathophysiological basis for therapy. J Hepatol. (2020) 72:558–77. doi: 10.1016/j.jhep.2019.10.003
36. Lang, S, and Schnabl, B. Microbiota and fatty liver disease-the known, the unknown, and the future. Cell Host Microbe. (2020) 28:233–44. doi: 10.1016/j.chom.2020.07.007
37. Wang, H, Guo, Y, Han, W, Liang, M, Xiao, X, Jiang, X, et al. Tauroursodeoxycholic acid improves nonalcoholic fatty liver disease by regulating gut microbiota and bile acid metabolism. J Agric Food Chem. (2024) 72:20194–210. doi: 10.1021/acs.jafc.4c04630
38. Wu, MY, and Fan, JG. Gut microbiome and nonalcoholic fatty liver disease. Hepatobiliary Pancreat Dis Int. (2023) 22:444–51. doi: 10.1016/j.hbpd.2023.06.006
39. Meijnikman, AS, Davids, M, Herrema, H, Aydin, O, Tremaroli, V, Rios-Morales, M, et al. Microbiome-derived ethanol in nonalcoholic fatty liver disease. Nat Med. (2022) 28:2100–6. doi: 10.1038/s41591-022-02016-6
40. Meijnikman, AS, Nieuwdorp, M, and Schnabl, B. Endogenous ethanol production in health and disease. Nat Rev Gastroenterol Hepatol. (2024) 21:556–71. doi: 10.1038/s41575-024-00937-w
41. Giraud, J, and Saleh, M. Host-Microbiota Interactions in Liver Inflammation and Cancer. Cancers. (2021) 13:4342. doi: 10.3390/cancers13174342
42. Xue, G, Feng, J, Zhang, R, Du, B, Sun, Y, Liu, S, et al. Three Klebsiella species as potential pathobionts generating endogenous ethanol in a clinical cohort of patients with auto-brewery syndrome: a case control study. EBioMedicine. (2023) 91:104560. doi: 10.1016/j.ebiom.2023.104560
43. Mbaye, B, Magdy Wasfy, R, Borentain, P, Tidjani Alou, M, Mottola, G, Bossi, V, et al. Increased fecal ethanol and enriched ethanol-producing gut bacteria Limosilactobacillus fermentum, Enterocloster bolteae, Mediterraneibacter gnavus and Streptococcus mutans in nonalcoholic steatohepatitis. Front Cell Infect Microbiol. (2023) 13:1279354. doi: 10.3389/fcimb.2023.1279354
44. Mbaye, B, Wasfy, RM, Alou, MT, Borentain, P, Andrieu, C, Caputo, A, et al. Limosilactobacillus fermentum, Lactococcus lactis and Thomasclavelia ramosa are enriched and Methanobrevibacter smithii is depleted in patients with non-alcoholic steatohepatitis. Microb Pathog. (2023) 180:106160. doi: 10.1016/j.micpath.2023.106160
45. Liu, J, Sun, J, Yu, J, Chen, H, Zhang, D, Zhang, T, et al. Gut microbiome determines therapeutic effects of OCA on NAFLD by modulating bile acid metabolism. NPJ Biofilms Microb. (2023) 9:29. doi: 10.1038/s41522-023-00399-z
46. Kuang, J, Wang, J, Li, Y, Li, M, Zhao, M, Ge, K, et al. Hyodeoxycholic acid alleviates non-alcoholic fatty liver disease through modulating the gut-liver axis. Cell Metab. (2023) 35:1752–1766.e8. doi: 10.1016/j.cmet.2023.07.011
47. Yan, M, Man, S, Liang, Y, Ma, L, Guo, L, Huang, L, et al. Diosgenin alleviates nonalcoholic steatohepatitis through affecting liver-gut circulation. Pharmacol Res. (2023) 187:106621. doi: 10.1016/j.phrs.2022.106621
48. Chen, X, Qiu, W, Ma, X, Ren, L, Feng, M, Hu, S, et al. Roles and Mechanisms of Choline Metabolism in Nonalcoholic Fatty Liver Disease and Cancers. Front Biosci. (2024) 29:182. doi: 10.31083/j.fbl2905182
49. Jayachandran, M, and Qu, S. Non-alcoholic fatty liver disease and gut microbial dysbiosis- underlying mechanisms and gut microbiota mediated treatment strategies. Rev Endocr Metab Disord. (2023) 24:1189–204. doi: 10.1007/s11154-023-09843-z
50. Tang, R, Liu, R, Zha, H, Cheng, Y, Ling, Z, and Li, L. Gut microbiota induced epigenetic modifications in the non-alcoholic fatty liver disease pathogenesis. Eng Life Sci. (2024) 24:2300016. doi: 10.1002/elsc.202300016
51. Violi, F, Pastori, D, Pignatelli, P, and Cammisotto, V. Endotoxemia and Platelets: 2 Players of Intrahepatic Microthrombosis in NAFLD. JACC Basic Transl Sci. (2024) 9:404–13. doi: 10.1016/j.jacbts.2023.07.003
52. Zuo, G, Chen, M, Zuo, Y, Liu, F, Yang, Y, Li, J, et al. Tea polyphenol epigallocatechin gallate protects against nonalcoholic fatty liver disease and associated endotoxemia in rats via modulating gut microbiota dysbiosis and alleviating intestinal barrier dysfunction and related inflammation. J Agric Food Chem. (2024) 72:9067–9086. doi: 10.1021/acs.jafc.3c04832
53. Violi, F, Nocella, C, Bartimoccia, S, Castellani, V, Carnevale, R, Pignatelli, P, et al. Gut dysbiosis-derived low-grade endotoxemia: A common basis for liver and cardiovascular disease. Kardiol Pol. (2023) 81:563–71. doi: 10.33963/KP.a2023.0115
54. Gudan, A, Kozłowska-Petriczko, K, Wunsch, E, Bodnarczuk, T, and Stachowska, E. Small intestinal bacterial overgrowth and non-alcoholic fatty liver disease: what do we know in 2023? Nutrients. (2023) 15:1323. doi: 10.3390/nu15061323
55. Vallianou, NG, Kounatidis, D, Psallida, S, Vythoulkas-Biotis, N, Adamou, A, Zachariadou, T, et al. NAFLD/MASLD and the Gut-Liver Axis: From Pathogenesis to Treatment Options. Meta. (2024) 14:366. doi: 10.3390/metabo14070366
56. Li, HY, Huang, SY, Zhou, DD, Xiong, RG, Luo, M, Saimaiti, A, et al. Theabrownin inhibits obesity and non-alcoholic fatty liver disease in mice via serotonin-related signaling pathways and gut-liver axis. J Adv Res. (2023) 52:59–72. doi: 10.1016/j.jare.2023.01.008
57. De Cól, JP, de Lima, EP, Pompeu, FM, Cressoni Araújo, A, de Alvares, GR, Bechara, MD, et al. Underlying mechanisms behind the brain-gut-liver axis and metabolic-associated fatty liver disease (MAFLD): an update. Int J Mol Sci. (2024) 25:3694. doi: 10.3390/ijms25073694
58. Xu, M, Zhan, Y, Gao, G, Zhu, L, Wu, T, and Xin, G. Associations of five dietary indices with metabolic dysfunction-associated steatotic liver disease and liver fibrosis among the United States population. Front Nutr. (2024) 11:1446694. doi: 10.3389/fnut.2024.1446694
59. Taheri, E, Yilmaz, Y, Ghorat, F, Moslem, A, and Zali, MR. Association of diet quality scores with risk of metabolic-associated fatty liver disease in Iranian population: a nested case-control study. J Diabetes Metab Disord. (2025) 24:46. doi: 10.1007/s40200-024-01544-x
60. Huang, J, Wu, Y, Zheng, J, Wang, M, Goh, GB, and Lin, S. The prognostic role of diet quality in patients with MAFLD and physical activity: data from NHANES. Nutr Diabetes. (2024) 14:4. doi: 10.1038/s41387-024-00261-x
61. Hsu, CL, and Schnabl, B. The gut-liver axis and gut microbiota in health and liver disease. Nat Rev Microbiol. (2023) 21:719–33. doi: 10.1038/s41579-023-00904-3
62. Li, Y, Deng, X, Guo, X, Zhang, F, Wu, H, Qin, X, et al. Preclinical and clinical evidence for the treatment of non-alcoholic fatty liver disease with soybean: A systematic review and meta-analysis. Front Pharmacol. (2023) 14:1088614. doi: 10.3389/fphar.2023.1088614
63. Ungvari, Z, and Kunutsor, SK. Coffee consumption and cardiometabolic health: a comprehensive review of the evidence. GeroScience. (2024) 46:6473–510. doi: 10.1007/s11357-024-01262-5
64. Kositamongkol, C, Kanchanasurakit, S, Auttamalang, C, Inchai, N, Kabkaew, T, Kitpark, S, et al. Coffee Consumption and Non-alcoholic Fatty Liver Disease: An Umbrella Review and a Systematic Review and Meta-analysis. Front Pharmacol. (2021) 12:786596. doi: 10.3389/fphar.2021.786596
65. Georgoulis, M, Kontogianni, MD, Tileli, N, Margariti, A, Fragopoulou, E, Tiniakos, D, et al. The impact of cereal grain consumption on the development and severity of non-alcoholic fatty liver disease. Eur J Nutr. (2014) 53:1727–35. doi: 10.1007/s00394-014-0679-y
66. Zhou, Q, Hu, H, Hu, L, Liu, S, Chen, J, and Tong, S. Association between processed and unprocessed red meat consumption and risk of nonalcoholic fatty liver disease: a systematic review and dose-response meta-analysis. J Glob Health. (2024) 14:04060. doi: 10.7189/jogh.14.04060
Keywords: dietary index for gut microbiota, non-alcoholic fatty liver disease, cross-sectional study, National Health and Nutrition Examination Survey, gut microbiota, dietary
Citation: Wang Y, Zhang B, Feng L, Cao C and Fei X (2025) A study of correlation of the dietary index for gut microbiota with non-alcoholic fatty liver disease based on 2007–2018 National Health and Nutrition Examination Survey. Front. Nutr. 12:1573249. doi: 10.3389/fnut.2025.1573249
Received: 08 February 2025; Accepted: 27 March 2025;
Published: 10 April 2025.
Edited by:
Bowen Li, Southwest University, ChinaReviewed by:
Fahrul Nurkolis, Medical Research Center of Indonesia, IndonesiaCopyright © 2025 Wang, Zhang, Feng, Cao and Fei. This is an open-access article distributed under the terms of the Creative Commons Attribution License (CC BY). The use, distribution or reproduction in other forums is permitted, provided the original author(s) and the copyright owner(s) are credited and that the original publication in this journal is cited, in accordance with accepted academic practice. No use, distribution or reproduction is permitted which does not comply with these terms.
*Correspondence: Xiaoliang Fei, ZmVpeGlhb2xpYW5nNDE5NEAxNjMuY29t
Disclaimer: All claims expressed in this article are solely those of the authors and do not necessarily represent those of their affiliated organizations, or those of the publisher, the editors and the reviewers. Any product that may be evaluated in this article or claim that may be made by its manufacturer is not guaranteed or endorsed by the publisher.
Research integrity at Frontiers
Learn more about the work of our research integrity team to safeguard the quality of each article we publish.