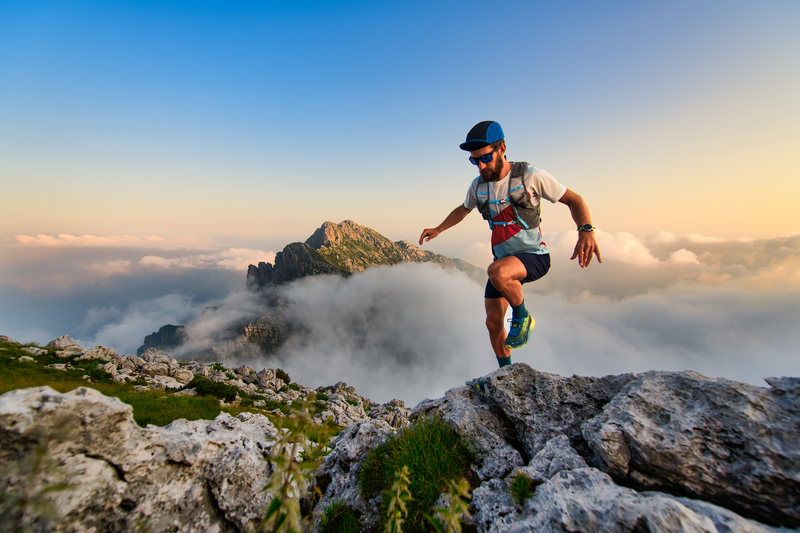
94% of researchers rate our articles as excellent or good
Learn more about the work of our research integrity team to safeguard the quality of each article we publish.
Find out more
ORIGINAL RESEARCH article
Front. Nutr.
Sec. Clinical Nutrition
Volume 12 - 2025 | doi: 10.3389/fnut.2025.1568527
The final, formatted version of the article will be published soon.
You have multiple emails registered with Frontiers:
Please enter your email address:
If you already have an account, please login
You don't have a Frontiers account ? You can register here
Background: Acute exacerbation of chronic obstructive pulmonary disease (AECOPD) significantly impacts patient quality of life and prognosis. This study aimed to quantitatively assess skeletal muscle mass in AECOPD patients using computed tomography and explore the association between muscle mass-related indices and all-cause mortality risk.Methods: A total of 319 patients were enrolled in this single-center retrospective cohort study. Muscle mass-related indices, including skeletal muscle area, mean muscle density, intermuscular fat density, intermuscular fat area, and skeletal muscle index (SMI), were considered as independent variables. All-cause mortality was considered as the dependent variable. Cox regression, subgroup and sensitivity analyses, receiver operating characteristic curve (ROC), restricted cubic spline plot (RCS), and Kaplan-Meier survival curves were used to examine the association between these indices and all-cause mortality.Results: During a median follow-up of 14.63 (6.33, 21.13) months, the all-cause mortality was 113 (35.4%).Multivariate Cox regression revealed that, regardless of whether SMI was grouped based on the median of 26.08 or the cut-off point of 24.01, the low SMI group had a higher risk of all-cause mortality (P < 0.001). Moreover, as a continuous variable, lower levels of SMI were independently associated with a higher risk of all-cause mortality (SMI, HR = 0.964, 95% CI: 0.934-0.996, P = 0.027; Standardized SMI, HR = 0.748, 95% CI: 0.578-0.967, P = 0.027). Subgroup and sensitivity analyses confirmed the significant association between SMI and all-cause mortality (P < 0.05). ROC analysis showed good predictive value for SMI (area under the curve = 0.663, 95% CI: 0.559-0.728, P < 0.001), and RCS analysis revealed a non-linear relationship between SMI and mortality (P nonlinear = 0.019). The Kaplan-Meier survival curves analysis indicated that regardless of whether SMI was grouped by median or by cut-off point, there were significant differences in the survival probability of all-cause mortality among different SMI groups, with the low SMI group having a poorer prognosis (P < 0.001).Conclusion: Among patients with AECOPD, higher levels of SMI are significantly associated with a lower risk of all-cause mortality, suggesting that SMI may have important prognostic value in the assessment of mortality risk in AECOPD patients.
Keywords: chronic obstructive pulmonary disease, Acute exacerbation, Skeletal muscle mass, Skeletal muscle index, All-cause mortality
Received: 30 Jan 2025; Accepted: 19 Mar 2025.
Copyright: © 2025 Li, Xu, Chen, Xu, Zheng, Zhao, Chen and Zaisheng. This is an open-access article distributed under the terms of the Creative Commons Attribution License (CC BY). The use, distribution or reproduction in other forums is permitted, provided the original author(s) or licensor are credited and that the original publication in this journal is cited, in accordance with accepted academic practice. No use, distribution or reproduction is permitted which does not comply with these terms.
* Correspondence:
Hongjun Zhao, Department of Pulmonary and Critical Care Medicine, Quzhou City People's Hospital, Quzhou, 324000, China
Chengshui Chen, Department of Pulmonary and Critical Care Medicine, Quzhou City People's Hospital, Quzhou, 324000, China
Zhu Zaisheng, Department of Pulmonary and Critical Care Medicine, Key Laboratory of Interventional Pulmonology of Zhejiang Province, The First Affiliated Hospital of Wenzhou Medical University, Wenzhou, China
Disclaimer: All claims expressed in this article are solely those of the authors and do not necessarily represent those of their affiliated organizations, or those of the publisher, the editors and the reviewers. Any product that may be evaluated in this article or claim that may be made by its manufacturer is not guaranteed or endorsed by the publisher.
Research integrity at Frontiers
Learn more about the work of our research integrity team to safeguard the quality of each article we publish.