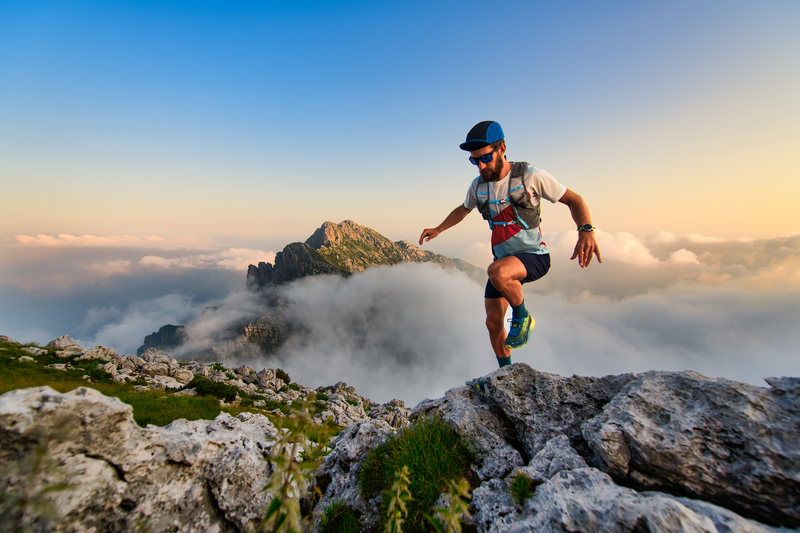
95% of researchers rate our articles as excellent or good
Learn more about the work of our research integrity team to safeguard the quality of each article we publish.
Find out more
ORIGINAL RESEARCH article
Front. Nutr. , 21 March 2025
Sec. Clinical Nutrition
Volume 12 - 2025 | https://doi.org/10.3389/fnut.2025.1564580
This article is part of the Research Topic Immunonutrition: Bridging Precision Nutrition and Modern Medicine View all 9 articles
Background: Previous studies have shown that pro-inflammatory diets increase the risk of coronary heart disease (CHD) and all-cause mortality. The dietary inflammatory index (DII) is a quantitative measure of dietary inflammation, and its accuracy has been validated by several studies.
Methods: This study included 43,842 participants aged ≥18 years from the National Health and Nutrition Examination Survey (NHANES) 1999–2018. The data of CHD was obtained through a questionnaire survey, and the DII was calculated using 24-h dietary recall data. Generalized linear models and logistic regression were used to determine the mediation factors, and subgroup analyses were conducted to evaluate the interaction between DII and CHD. Mean decrease in Gini (MDG) was used to determine the importance of individual dietary components.
Results: The age of the participants was 49.81 ± 18.10 years, with 20,793 (47.4%) being male. A total of 1,892 (4.3%) participants were diagnosed with CHD, and the median DII score was 1.33 (0.11, 2.40). After adjusting for potential confounders, logistic regression analysis revealed that DII independently associated with CHD [OR: 1.049 (1.012–1.087), p = 0.008]. Triglyceride-glucose index, visceral adiposity index, body mass index, waist-to-height ratio, high-density lipoprotein, and glomerular filtration rate (all p < 0.05) may mediate the relationship between DII and CHD. Subgroup analyses showed that DII was more sensitive in participants aged <75 years (p < 0.001), females (p = 0.028), those with low cholesterol levels (p = 0.004), and individuals with low Framingham risk scores (p = 0.005). MDG analysis indicated that carbohydrate, vitamin C and iron intake have the greatest impact on CHD.
Conclusion: This study suggests that various metabolic and lipid indicators play a mediating role in the relationship between DII and CHD. DII may have a greater adverse impact on traditional low-risk CHD populations.
Coronary heart disease (CHD) is characterized by accumulation of lipids and fibrous tissue in the intima of the arterial wall, smooth muscle cell proliferation, and local and systemic inflammatory responses (1). CHD poses a giant threat to health. In the United States, approximately 20.1 million individuals are diagnosed with CHD (2). Despite recent advances in treatment, it remains one of the leading causes of death globally (3). The complex pathophysiological mechanisms of CHD present a significant challenge to improving patient outcomes. Studies have shown that inflammation plays a key role in the onset and progression of CHD (4). It is involved in several stages of atherosclerosis initiation and progression, plaque rupture, ischemia–reperfusion injury, and ventricular remodeling (4).
Healthy dietary habits, such as the Mediterranean diet, high-fiber diets, and plant-based diets, have been shown to be associated with lower systemic inflammation levels. The dietary inflammatory index (DII), first proposed by Cavicchia et al. (5), is used to quantitatively assess the inflammatory potential of diet. The DII is based on 45 different anti-inflammatory and pro-inflammatory food components. A positive DII score indicates a pro-inflammatory effect, while a negative score indicates an anti-inflammatory effect. The higher the score, the more pronounced the pro-inflammatory effect. An increasing body of evidence has described a certain correlation between higher DII and elevated levels of inflammatory markers, such as hypersensitive C-reactive protein (hsCRP), tumor necrosis factor-α (TNF-α) and interleukin-6 (IL-6) (6–8).
Current research suggests that the DII is associated with subclinical atherosclerosis. DII in patients with CHD was significantly higher than in those without CHD (9). A 36-month follow-up study found a positive association between DII and the risk of atherosclerosis-related mortality [HR: 1.36(1.15–1.60)] (10). Additionally, DII was positively associated with all-cause mortality risk in CHD patients, particularly among women (11). However, current studies have not explored the interaction between DII and traditional risk factors, nor have they investigated potential mediating factors between DII and CHD. Due to the inclusion of multiple variables, guiding patients on diet based on DII becomes more complex.
Therefore, this study aims to explore the correlation between DII and CHD, as well as traditional risk factors, further analyze mediating factors and interactions, and rank the importance of dietary components to enhance the practicality in clinical settings.
The National Health and Nutrition Examination Survey (NHANES) is a research project aimed at assessing the health and nutritional status of United States. The survey used scientific sampling methods and annually collected data from approximately 5,000 individuals from 15 counties, representing the national population. The inclusion criteria for this study were: (1) participants in NHANES surveys from 1999 to 2018; (2) age ≥ 18 years. The exclusion criteria were: (1) missing key variables regarding the presence of CHD; (2) missing dietary data required for the calculation of the DII. Ultimately, 43,842 participants were included in this study. The detailed participant inclusion process was shown in Figure 1. The NHANES study was approved by the Institutional Review Board of the National Center for Health Statistics, and all participants provided written informed consent.
The DII was calculated based on individual dietary components, with dietary data collected through 24-h dietary recall interviews in NHANES. For the 1999–2002, only 1 day’s dietary component records were included, and from 2003 to 2018, the average of 2 days of dietary data was used. The two dietary recall data collections were conducted separately: the first was carried out at the Mobile Examination Center, while the second was collected via telephone 3 to 10 days later. This approach helps provide a more comprehensive evaluation of each participant’s dietary habits. The calculation of the DII requires 45 dietary components; however, due to limitations in NHANES data collection, this study included 28 dietary components for DII calculation, which are: alcohol, vitamin B12, vitamin B6, β-carotene, caffeine, carbohydrate, cholesterol, energy, total fat, fiber, folic acid, iron, magnesium, monounsaturated fatty acid, niacin, n-3 fatty acid, n-6 fatty acid, protein, polyunsaturated fatty acid, riboflavin, saturated fat, selenium, thiamine, vitamin A, vitamin C, vitamin D, vitamin E, and zinc. Previous studies have shown that using fewer than 30 dietary components for DII calculation does not significantly affect its accuracy (6, 12). Even a DII calculation that incorporates 28 dietary components has a strong predictive ability for high CRP levels [OR 1.10 (1.02–1.19)] (6). The DII calculation formula is as follows:
Convert the Z-score to a percentile score and then standardize it
The data of CHD was collected from the NHANES interview, where each question was standardized and administered by trained professionals. CHD was defined as “Has a doctor or other health professional ever told you that you had coronary heart disease? (MCQ160C).”
The following variables were extracted from the NHANES: age, sex, race, education level, smoking, hypertension, diabetes, body mass index (BMI), glomerular filtration rate (GFR), total cholesterol, triglycerides, high-density lipoprotein (HDL), low-density lipoprotein (LDL), and hemoglobin A1c. Smoking was defined as having smoked more than 100 cigarettes in one’s lifetime. Biochemical parameters were evaluated using a standardized methodology detailed in the NHANES Laboratory/Medical Technologist Procedure Manual (CDC: NHANES Laboratory/Medical Technologists Procedures Manual, Atlanta, GA, CDC, 2001).
The triglyceride-glucose index (TyG) is defined as ln[triglycerides (mg/dL)/fasting glucose (mg/dL)/2]. The Waist-to-Height Ratio (WHtR) is defined as waist circumference (cm)/height (cm). The Framingham score, first proposed in 2008 to assess cardiovascular disease risk, was updated in 20151 (13). The formula for the visceral adiposity index (VAI) is as follows:
Due to the complexity of NHANES sampling, weighted regression models were used with WTDRD1 and WTDR2D as weights. All participants were divided into three groups based on the tertiles of the DII. Normally distributed continuous data were expressed as means ± standard deviations, and differences between the three groups were examined using one-way ANOVA. Non-normally distributed continuous data were expressed as medians (Q1, Q3) and compared using the Kruskal-Wallis H test. For continuous variables, effect sizes were calculated using Cohen’s F, and post hoc comparisons were performed using the Newman–Keuls method. Categorical data were presented as counts and percentages and were compared using the chi-squared test, with Cramér’s V employed to assess effect sizes and calculating pairwise comparisons between pairs of proportions with bonferroni correction for multiple testing. The relationship between DII and traditional cardiovascular risk factors was assessed using restricted cubic splines (RCS). The association between DII and CHD was explored using multivariable logistic regression models, with DII as both continuous and categorical variable to evaluate the robustness of the results.
In exploring the mediating factors in the association between DII and CHD, two models were constructed. The first is the mediator model, using generalized linear models (GLM) to fit the Gaussian distribution (linear regression with an identity link function) between DII and potential mediators, while controlling for potential confounders. The second is the outcome model, which uses both GLM and logistic regression models, incorporating both exposure factors and mediators. To ensure the reliability of the results, Bootstrap resampling was performed 1,000 time (14). Further subgroup analyses were conducted based on age, sex, cholesterol levels, and Framingham risk scores to assess whether there are interaction effects between DII and these factors. The mean decrease in Gini (MDG) is a commonly used method for measuring feature importance in random forest models, reflecting the contribution of each dietary component to the model’s predictive performance.
Statistical significance was set at p < 0.05. All analyses were performed using R version 4.2.0 (R Foundation for Statistical Computing, Vienna, Austria). The analysis mainly used the ‘survey’, ‘plotRCS’, ‘mediation’, and ‘randomForest’ packages.
A total of 43,842 participants were included in this study, with a mean age of 49.81 ± 18.10 years, of whom 20,793 (47.4%) were male. Among the participants, 15,310 (34.9%) had hypertension, and 5,289(12.1%) had diabetes. The DII score was 1.33 (0.11, 2.40). Participants were divided into three groups based on the DII tertiles, with 14,614 individuals in each group. Significant differences were observed between the groups in terms of age, sex, race, education level, hypertension, diabetes, CHD, BMI, GFR, total cholesterol and HDL (all p < 0.05). The characteristics and effect size data of the three groups were shown in Table 1. Pairwise comparisons between the three groups were performed (Supplementary Table S1). Further comparisons of dietary components between the three groups revealed significant differences across all 28 dietary components included in this study (all p < 0.001) (Supplementary Tables S2, S3).
After adjusting for age, sex, race, and education level, the relationship between DII, CHD, and common risk factors was analyzed using RCS. The knots between 3 and 7 were tested, and the model with the lowest Akaike information criterion value was selected for the RCS analysis. As shown in Figure 2A, there appears to be a J-shaped curve between DII and CHD, with the risk of CHD increasing at an accelerating rate as DII rises. The results indicated a positive correlation between DII and WHtR, BMI, TyG, and the Framingham score (Figures 2B,D–F). DII was negatively correlated with HDL (Figure 2C).
Figure 2. Restricted cubic spline analysis of the association between dietary inflammation index and coronary heart disease and its risk factors (A) coronary heart disease; (B) body mass index; (C) high-density lipoprotein; (D) waist-to-height ratio; (E) triglyceride and glucose index; (F) Framingham score. A restricted cubic spline model was calculated after adjusting for age, sex, race and education level.
For confounder adjustment, multivariable logistic regression was used to analyze the relationship between DII and CHD. DII was analyzed as a continuous variable. Based on previous literature (15) and the covariates included in this study, Model 1 did not adjust for any covariates; Model 2 adjusted for age, sex, race, and education; Model 3 adjusted for hypertension, diabetes, smoking, BMI, GFR and total cholesterol in addition to the covariates in Model 2. In the unadjusted model, a higher DII was significantly associated with an increased risk of CHD [OR: 1.057 (1.026–1.089), p < 0.001]. In Model 2, the association between DII and CHD remained significant [OR: 1.065 (1.031–1.101), p < 0.001]. In Model 3, the correlation between DII and CHD continued to exist [OR: 1.049 (1.012–1.087), p = 0.008]. When DII was categorized into lower, middle, and higher DII groups, the analysis results remained consistent [OR: 1.095 (1.024–1.171), p = 0.008] (Table 2).
Given the correlation between DII and traditional CHD risk factors, further analysis of the mediators between DII and CHD was conducted after adjusting for age, sex, race, and education level. The average direct effect (ADE) represents the direct effect of DII on the outcome variable without mediation, while the average causal mediation effect (ACME) represents the indirect effect of DII on the outcome variable through mediators. The results indicated that DII indirectly increases the risk of CHD by elevating TyG, VAI, BMI, and WHtR. Additionally, DII was found to increase the risk of CHD by lowering HDL and GFR (Figure 3). However, since this study is cross-sectional, causality still needs to be confirmed through further longitudinal research.
Figure 3. Mediators of the association between dietary inflammation index and coronary heart disease. Arrows and rounded heads indicate promotion and inhibition, respectively. The model was calculated after adjusting for age, sex, race and education level. ACME, average causal mediation effects; ADE, average direct effects. TyG, triglyceride-glucose index; HDL, high-density lipoprotein cholesterol; GFR, glomerular filtration rate; VAI, visceral adiposity index; BMI, body mass index; WHtR, waist-to-height ratio. (A) TyG, (B) HDL, (C) GFR, (D) VAI, (E) BMI, (F) WtHR.
To further explore the role of DII in different populations, subgroup analyses were performed based on age (≥75 years vs. <75 years), sex, cholesterol levels (≥5.2 mmol/L vs. <5.2 mmol/L), and Framingham risk score. After adjusting for sex, race, and education level, the subgroup aged <75 years showed higher sensitivity to DII (p for interaction <0.001). When adjusted for age, race, and education level, a stronger association between DII and CHD was observed in females (p for interaction = 0.028). In the Framingham risk groups, individuals with a 10-year heart disease risk >10% were classified as high-risk (men ≥12 points, women ≥20 points). Further analysis showed that, after adjusting for age, sex, race, and education level, individuals with low cholesterol levels (p for interaction = 0.004) and those in the Framingham low-risk group (p for interaction = 0.005) were more sensitive to DII (Figure 4).
Figure 4. Subgroup analysis in the relationship between dietary inflammation index and coronary heart disease. *, adjusting for sex, race and education level; **, adjusting for age, race and education level; ***, adjusting for age, sex, race and education level. (A) Different age group, (B) Sex group, (C) Different cholesterol group, (D) Different framinghan risk group.
To assess the contribution of dietary components to CHD, we used a random forest model to calculate the MDG for each dietary component. The larger the MDG value, the greater the contribution of that dietary component to the model’s performance. The results showed that the top 5 dietary components contributing the most to CHD were carbohydrate, vitamin C, iron, vitamin A and caffeine, while the bottom 3 components were vitamin D, alcohol, and monounsaturated fatty acids (Figure 5).
Figure 5. The importance ranking of 28 dietary components in the dietary inflammation index for CHD using random forest. +, pro-inflammatory; −, anti-inflammatory; GINI, gini index; PUFA, polyunsaturated fatty acids; MUFA, monounsaturated fatty acids.
This study, which included 43,842 participants from the NHANES between 1999 and 2018, showed the association between the DII and CHD, and explored the potential mediating factors and interactions. The results showed that: (1) After adjusting for multiple variables, DII remained independently associated with CHD. (2) DII may be associated with CHD through its impact on factors such as TyG, VAI, BMI, WHtR, HDL and GFR. (3) The association between DII and CHD was more sensitive in individuals aged <75 years, females, those with low cholesterol levels, and those in the low-risk Framingham score group. (4) Among the 28 dietary components analyzed in this study, carbohydrate, vitamin C and iron were found to have the greatest association on CHD.
CHD is a chronic inflammatory disease influenced by various factors. The DII is an inflammation indicator based on dietary components, and studies have shown that DII is correlated with multiple inflammatory markers in the body (6–8, 16). Previous research has also confirmed the association between DII and CHD (9). In this study, despite adjusting for age, sex, race, education level, and traditional cardiovascular risk factors, DII remained independently associated with CHD. Prior studies showed that higher DII in CHD patients is associated with significantly reduced plaque stability, indicating that anti-inflammatory diets may play an important protective role in the pathogenesis of CHD (17).
In this study, TyG, VAI, BMI, WHtR, HDL, and GFR may serve as mediators in the relationship between DII and CHD. TyG index is a well-established indicator of insulin resistance and has been shown to effectively predict the risk of various cardiovascular diseases, including CHD, atherosclerosis, and stroke (18, 19). Pro-inflammatory diets elevate circulating inflammatory cytokines (e.g., TNF-α, IL-6), which impair insulin signaling in adipose tissue, muscle, and liver. Chronic insulin resistance directly damages vascular endothelial cells and smooth muscle cells, promoting atherosclerotic plaque formation (20). Additionally, excessive reactive oxygen species and harmful glycation products generated by hyperglycemia contribute to chronic inflammation, further amplifying the risk of CHD (21). Shu et al. (22) reported that DII was positively correlated with fasting glucose, fasting insulin, and the homeostasis model assessment of insulin resistance. Furthermore, a cohort study further suggested that the relationship between pro-inflammatory diets and nonfatal cardiovascular diseases was partially mediated by TyG (23).
VAI, BMI, and WHtR are indicators of visceral adiposity and obesity, and they exhibit strong associations with CHD and all-cause mortality (24, 25). Pro-inflammatory diets, such as high-fat diets, activate the pro-inflammatory factor NF-κB by inducing endoplasmic reticulum (ER) stress and promoting the interaction of TLR4 and TLR2. Oxidative stress in the ER plays a critical role in initiating inflammation and metabolic disorders, further contributing to visceral fat accumulation and obesity (26). Moreover, dietary inflammation levels may influence gut microbiota composition. Anti-inflammatory diets have been shown to lower the proportion of Gram-negative bacteria in the gut, strengthen intestinal barrier function, and reduce endotoxin levels, thereby mitigating metabolic and inflammatory responses (27).
Moreover, pro-inflammatory diets promote inflammatory markers (TNF-α and NF-κB) and oxidative stress markers (elevated NADPH oxidase activity) in the renal cortex, inducing mesangial cell proliferation and the progression of proteinuria, ultimately leading to a decline in GFR (28). Chronic kidney disease is often associated with lipid metabolism disorders, and the accumulation of toxins in the body can directly damage endothelial cells, promote platelet adhesion, and contribute to the formation of atherosclerotic plaques (29). Due to incomplete and methodologically inconsistent CRP/hsCRP measurements in NHANES (1999–2018), CRP was not analyzed as a mediator in this study.
Subgroup analyses were conducted to examine the relationship between DII and CHD across different CHD risk factors, including age, sex, total cholesterol levels, and Framingham risk score, to evaluate the robustness of the findings. This study found that elevated DII may be more strongly associated with CHD in individuals aged <75 years, females, those with low cholesterol levels, and individuals at low risk group according to the Framingham score. A prospective cohort study involving 155,724 participants, with approximately 10 years of follow-up, revealed that poor dietary quality was more strongly associated with cardiovascular disease in women than in men, and DII was also linked to a higher risk of all-cause mortality in female CHD patients (11, 30). From Figure 4D, it can be observed that when DII is at a low level, the CHD risk in the low-risk group is significantly lower compared to the high-risk group. As DII increases, the CHD risk in the low-risk group rises rapidly, ultimately approaching that of the high-risk group. This suggests that the impact of DII is more sensitive in the low-risk population than in the high-risk group. One possible explanation is that individuals in the low-risk group lack other strong risk factors, making the inflammatory response triggered by diet more prominent. Additionally, high-risk individuals may have access to more health education and lifestyle interventions, thereby reducing their risk of CHD. Finally, DII may exert a greater effect in the low-risk group through interactions with other metabolic and inflammatory markers. Despite adjusting for general demographic characteristics (sex, age, race, and education level), potential confounding factors may still exist, requiring further validation through prospective cohort studies and mechanistic research.
Calculating the DII requires the collection of 45 different dietary components, which poses a challenge for both doctors and patients in clinical practice. This increases the complexity of assessment due to individual differences, dietary habits, and measurement errors. In this study, we used the random forest method to rank the contribution of dietary components to CHD risk based on their DII. However, this is only a preliminary exploration, and further research is needed to assess its feasibility and accuracy.
Our study found that carbohydrate, vitamin C and iron were the three components with the greatest DII contribution to CHD. Carbohydrates are the primary source of energy in the diet. A study involving over 10,000 participants, followed for more than a decade, found a significant association between carbohydrate intake and major adverse cardiovascular events (HR: 1.35; 95%CI: 1.07–1.71; p-trend = 0.001) (31). Another study from 18 countries showed a non-linear relationship between carbohydrate intake and cardiovascular disease mortality (32). Vitamin C is a well-known antioxidant. A research has shown that the intake of vitamin C is negatively correlated with the carotid intima-media thickness in patients with CHD (r = −0.113, p = 0.001) (33). Additionally, vitamin C intake is positively correlated with plaque stability in these patients and may exert its effects by reducing IL-6 and TNF-α, thereby inhibiting the inflammatory response in atherosclerosis (34). Iron’s DII ranked third in contributing to CHD risk. Iron intake has been positively correlated with CRP levels (p trend = 0.03) (35). A meta-analysis of 6 prospective studies indicated that individuals with higher heme iron intake had a 31% increased risk of CHD (36). This suggests that iron intake, particularly heme iron, may need to be controlled to reduce inflammation.
Interestingly, alcohol, typically considered a risk factor for CHD, ranked second to last in DII contribution. This may be related to the relatively low alcohol intake in the study population compared to the upper limits recommended in current guidelines. In the United States, the recommended alcohol intake limit is 196 g per week for men and 98 g per week for women (37). A J-shaped curve relationship exists between alcohol consumption and CHD (38). Recent research has found that reducing alcohol consumption can help consistently lower cardiovascular disease risk, although a clear threshold for alcohol intake remains undefined (39).
This study has several limitations. First, since NHANES is a cross-sectional survey, this study cannot determine a causal relationship between DII and CHD risk, but only an association between the two. Future studies with longitudinal designs are needed to better evaluate the impact of DII on CHD risk. Second, dietary data were collected using recall methods, which may introduce subjective bias and may not accurately reflect long-term daily dietary intake. However, studies have shown a strong correlation between dietary data obtained through food records and 24-h recalls (40). Moreover, 24-h recall methods have been widely used in dietary intervention trials and national surveys (40). Third, the outcome variable in this study was based on self-reported questionnaires, which are subject to recall bias, information bias, and potential misclassification of exposure. Although NHANES implements multiple measures to minimize recall and information bias during data collection, the possibility of data quality issues remains. Lastly, although we adjusted for potential confounding factors, the influence of other unmeasured confounders on the results cannot be entirely excluded.
Higher DII is independently associated with an increased risk of CHD, potentially through pathways involving metabolism, lipid levels, and kidney function. The impact of DII on CHD is more sensitive in individuals with low traditional risk. These findings provide new evidence for the role of dietary interventions in reducing CHD incidence and lay the groundwork for future cohort studies and mechanistic investigations.
Publicly available datasets were analyzed in this study. This data can be found at: https://wwwn.cdc.gov/nchs/nhanes/.
The studies involving humans were approved by Institutional Review Board of the National Center for Health Statistics. The studies were conducted in accordance with the local legislation and institutional requirements. The participants provided their written informed consent to participate in this study.
HX: Formal analysis, Software, Writing – review & editing. PX: Data curation, Methodology, Visualization, Writing – original draft. HL: Resources, Supervision, Writing – review & editing. ZT: Methodology, Validation, Writing – review & editing. RZ: Project administration, Writing – review & editing. MC: Conceptualization, Funding acquisition, Investigation, Project administration, Writing – review & editing.
The author(s) declare that financial support was received for the research and/or publication of this article. This study was funded by the Beijing Research Ward Excellence Clinical Study Program (BRWEP2024W014090201) and the Key Clinical Projects of Peking University Third Hospital (No. 2024003).
We thank all participants, researchers and staff in the NHANES study.
The authors declare that the research was conducted in the absence of any commercial or financial relationships that could be construed as a potential conflict of interest.
The authors declare that no Gen AI was used in the creation of this manuscript.
All claims expressed in this article are solely those of the authors and do not necessarily represent those of their affiliated organizations, or those of the publisher, the editors and the reviewers. Any product that may be evaluated in this article, or claim that may be made by its manufacturer, is not guaranteed or endorsed by the publisher.
The Supplementary material for this article can be found online at: https://www.frontiersin.org/articles/10.3389/fnut.2025.1564580/full#supplementary-material
1. ^https://www.thecalculator.co/health/Framingham-Risk-Score-Calculator-for-Coronary-Heart-Disease-745.html
1. Lechner, K, von Schacky, C, McKenzie, AL, Worm, N, Nixdorff, U, Lechner, B, et al. Lifestyle factors and high-risk atherosclerosis: pathways and mechanisms beyond traditional risk factors. Eur J Prev Cardiol. (2020) 27:394–406. doi: 10.1177/2047487319869400
2. Writing Committee, M, Virani, SS, Newby, LK, Arnold, SV, Bittner, V, Brewer, LC, et al. 2023 AHA/ACC/ACCP/ASPC/NLA/PCNA guideline for the management of patients with chronic coronary disease: a report of the American Heart Association/American College of Cardiology Joint Committee on clinical practice guidelines. J Am Coll Cardiol. (2023) 82:833–955. doi: 10.1016/j.jacc.2023.04.003
3. Tsao, CW, Aday, AW, Almarzooq, ZI, Alonso, A, Beaton, AZ, Bittencourt, MS, et al. Heart disease and stroke statistics-2022 update: a report from the American Heart Association. Circulation. (2022) 145:e153–639. doi: 10.1161/CIR.0000000000001052
4. Punnanithinont, N, Kambalapalli, S, Iskander, B, Ichikawa, K, Krishnan, S, Lakshmanan, S, et al. Anti-inflammatory therapies in atherosclerosis–where are we going? Curr Atheroscler Rep. (2024) 27:19. doi: 10.1007/s11883-024-01267-7
5. Cavicchia, PP, Steck, SE, Hurley, TG, Hussey, JR, Ma, Y, Ockene, IS, et al. A new dietary inflammatory index predicts interval changes in serum high-sensitivity C-reactive protein. J Nutr. (2009) 139:2365–72. doi: 10.3945/jn.109.114025
6. Shivappa, N, Steck, SE, Hurley, TG, Hussey, JR, Ma, Y, Ockene, IS, et al. A population-based dietary inflammatory index predicts levels of C-reactive protein in the seasonal variation of blood cholesterol study (seasons). Public Health Nutr. (2014) 17:1825–33. doi: 10.1017/S1368980013002565
7. Shivappa, N, Hebert, JR, Rietzschel, ER, De Buyzere, ML, Langlois, M, Debruyne, E, et al. Associations between dietary inflammatory index and inflammatory markers in the asklepios study. Br J Nutr. (2015) 113:665–71. doi: 10.1017/S000711451400395X
8. Shivappa, N, Hebert, JR, Marcos, A, Diaz, LE, Gomez, S, Nova, E, et al. Association between dietary inflammatory index and inflammatory markers in the Helena study. Mol Nutr Food Res. (2017) 61:707. doi: 10.1002/mnfr.201600707
9. Agraib, LM, Azab, M, Al-Shudifat, AE, Allehdan, SS, Shivappa, N, Hebert, JR, et al. Dietary inflammatory index and odds of coronary artery disease in a case-control study from Jordan. Nutrition. (2019) 63-64:98–105. doi: 10.1016/j.nut.2018.11.027
10. Bondonno, NP, Lewis, JR, Blekkenhorst, LC, Shivappa, N, Woodman, RJ, Bondonno, CP, et al. Dietary inflammatory index in relation to sub-clinical atherosclerosis and atherosclerotic vascular disease mortality in older women. Br J Nutr. (2017) 117:1577–86. doi: 10.1017/S0007114517001520
11. Wang, E, Fang, C, Zhang, J, and Wang, Y. Association between dietary inflammatory index and all-cause mortality risk in adults with coronary heart disease in the United States. Sci Rep. (2024) 14:23998. doi: 10.1038/s41598-024-75381-6
12. Park, YM, Choi, MK, Lee, SS, Shivappa, N, Han, K, Steck, SE, et al. Dietary inflammatory potential and risk of mortality in metabolically healthy and unhealthy phenotypes among overweight and obese adults. Clin Nutr. (2019) 38:682–8. doi: 10.1016/j.clnu.2018.04.002
13. D'Agostino, RB Sr, Vasan, RS, Pencina, MJ, Wolf, PA, Cobain, M, Massaro, JM, et al. General cardiovascular risk profile for use in primary care: the Framingham heart study. Circulation. (2008) 117:743–53. doi: 10.1161/CIRCULATIONAHA.107.699579
14. Valente, MJ, Rijnhart, JJM, Smyth, HL, Muniz, FB, and MacKinnon, DP. Causal mediation programs in R, Mplus, SAS, SPSS, and Stata. Struct Equ Modeling. (2020) 27:975–84. doi: 10.1080/10705511.2020.1777133
15. Wang, B, and Sun, X. Weight-adjusted waist index shows superior detection of coronary artery disease than body mass index in NHANES 1999-2020. Sci Rep. (2025) 15:7077. doi: 10.1038/s41598-025-90877-5
16. Smidowicz, A, and Regula, J. Effect of nutritional status and dietary patterns on human serum C-reactive protein and Interleukin-6 concentrations. Adv Nutr. (2015) 6:738–47. doi: 10.3945/an.115.009415
17. Zhao, Z, Li, L, Gao, X, Hu, G, Liu, G, Tao, H, et al. High dietary inflammatory index is associated with decreased plaque stability in patients with coronary heart disease. Nutr Res. (2023) 119:56–64. doi: 10.1016/j.nutres.2023.08.007
18. Liu, X, Tan, Z, Huang, Y, Zhao, H, Liu, M, Yu, P, et al. Relationship between the triglyceride-glucose index and risk of cardiovascular diseases and mortality in the general population: a systematic review and meta-analysis. Cardiovasc Diabetol. (2022) 21:124. doi: 10.1186/s12933-022-01546-0
19. Liu, L, Peng, J, Wang, N, Wu, Z, Zhang, Y, Cui, H, et al. Comparison of seven surrogate insulin resistance indexes for prediction of incident coronary heart disease risk: a 10-year prospective cohort study. Front Endocrinol (Lausanne). (2024) 15:1290226. doi: 10.3389/fendo.2024.1290226
20. Laakso, M, and Kuusisto, J. Insulin resistance and hyperglycaemia in cardiovascular disease development. Nat Rev Endocrinol. (2014) 10:293–302. doi: 10.1038/nrendo.2014.29
21. Li, JM, Li, X, Chan, LWC, Hu, R, Zheng, T, Li, H, et al. Lipotoxicity-polarised macrophage-derived exosomes regulate mitochondrial fitness through miro1-mediated mitophagy inhibition and contribute to type 2 diabetes development in mice. Diabetologia. (2023) 66:2368–86. doi: 10.1007/s00125-023-05992-7
22. Shu, Y, Wu, X, Wang, J, Ma, X, Li, H, and Xiang, Y. Associations of dietary inflammatory index with prediabetes and insulin resistance. Front Endocrinol (Lausanne). (2022) 13:820932. doi: 10.3389/fendo.2022.820932
23. Liu, B, Ren, X, and Tian, W. Dietary inflammatory potential and the risk of nonfatal cardiovascular diseases in the China health and nutrition survey. Nutrition. (2024) 124:112469. doi: 10.1016/j.nut.2024.112469
24. Luo, Y, Zhan, X, Liu, Y, Chen, L, Zhu, L, and Cai, W. Predicted visceral adiposity index in relation to risk of coronary heart disease and all-cause mortality: insights from NHANES. Front Endocrinol (Lausanne). (2023) 14:1296398. doi: 10.3389/fendo.2023.1296398
25. Wang, F, Chen, Y, Chang, Y, Sun, G, and Sun, Y. New anthropometric indices or old ones: Which perform better in estimating cardiovascular risks in Chinese adults. BMC cardiovasc. disord. (2018) 18:14. doi: 10.1186/s12872-018-0754-z
26. Sozen, E, and Ozer, NK. Impact of high cholesterol and endoplasmic reticulum stress on metabolic diseases: an updated mini-review. Redox Biol. (2017) 12:456–61. doi: 10.1016/j.redox.2017.02.025
27. Xu, J, Xie, L, Fan, R, Shi, X, Xu, W, Dong, K, et al. The role of dietary inflammatory index in metabolic diseases: the associations, mechanisms, and treatments. Eur J Clin Nutr. (2024). doi: 10.1038/s41430-024-01525-6
28. Duan, Y, Zeng, L, Zheng, C, Song, B, Li, F, Kong, X, et al. Inflammatory links between high fat diets and diseases. Front Immunol. (2018) 9:2649. doi: 10.3389/fimmu.2018.02649
29. Silva, RP, and Diogenes, C. Heart disease and the kidneys. Contrib Nephrol. (2021) 199:71–9. doi: 10.1159/000517704
30. Walli-Attaei, M, Rosengren, A, Rangarajan, S, Breet, Y, Abdul-Razak, S, Sharief, WA, et al. Metabolic, behavioural, and psychosocial risk factors and cardiovascular disease in women compared with men in 21 high-income, middle-income, and low-income countries: an analysis of the Pure study. Lancet. (2022) 400:811–21. doi: 10.1016/S0140-6736(22)01441-6
31. Lim, CGY, Tai, ES, and van Dam, RM. Replacing dietary carbohydrates and refined grains with different alternatives and risk of cardiovascular diseases in a multi-ethnic Asian population. Am J Clin Nutr. (2022) 115:854–63. doi: 10.1093/ajcn/nqab403
32. Dehghan, M, Mente, A, Zhang, X, Swaminathan, S, Li, W, Mohan, V, et al. Associations of fats and carbohydrate intake with cardiovascular disease and mortality in 18 countries from five continents (Pure): a prospective cohort study. Lancet. (2017) 390:2050–62. doi: 10.1016/S0140-6736(17)32252-3
33. Ramezankhani, A, Hadaegh, P, and Hadaegh, F. Association of Novel Dietary and Lifestyle Inflammation Scores with incidence and progression of coronary artery calcification in middle-late adulthood: a longitudinal cohort study. Nutr J. (2024) 23:127. doi: 10.1186/s12937-024-01028-x
34. Li, L, Zhao, Z, Wang, Y, Gao, X, Liu, G, Yu, B, et al. Association between dietary intakes and plaque vulnerability measured by optical coherence tomography in patients with coronary heart disease: a mediation analysis of inflammatory factors. Front Nutr. (2022) 9:920892. doi: 10.3389/fnut.2022.920892
35. de Oliveira Otto, MC, Alonso, A, Lee, DH, Delclos, GL, Jenny, NS, Jiang, R, et al. Dietary micronutrient intakes are associated with markers of inflammation but not with markers of subclinical atherosclerosis. J Nutr. (2011) 141:1508–15. doi: 10.3945/jn.111.138115
36. Yang, W, Li, B, Dong, X, Zhang, XQ, Zeng, Y, Zhou, JL, et al. Is heme Iron intake associated with risk of coronary heart disease? A meta-analysis of prospective studies. Eur J Nutr. (2014) 53:395–400. doi: 10.1007/s00394-013-0535-5
37. Kalinowski, A, and Humphreys, K. Governmental standard drink definitions and low-risk alcohol consumption guidelines in 37 countries. Addiction. (2016) 111:1293–8. doi: 10.1111/add.13341
38. Biddinger, KJ, Emdin, CA, Haas, ME, Wang, M, Hindy, G, Ellinor, PT, et al. Association of habitual alcohol intake with risk of cardiovascular disease. JAMA Netw Open. (2022) 5:e223849. doi: 10.1001/jamanetworkopen.2022.3849
39. Wood, AM, Kaptoge, S, Butterworth, AS, Willeit, P, Warnakula, S, Bolton, T, et al. Risk thresholds for alcohol consumption: combined analysis of individual-participant data for 599 912 current drinkers in 83 prospective studies. Lancet. (2018) 391:1513–23. doi: 10.1016/S0140-6736(18)30134-X
Keywords: dietary inflammatory index, coronary heart disease, National Health and Nutrition Examination Survey, mediating factors, interaction
Citation: Xu H, Xie P, Liu H, Tian Z, Zhang R and Cui M (2025) The relationship between dietary inflammatory index in adults and coronary heart disease: from NHANES 1999–2018. Front. Nutr. 12:1564580. doi: 10.3389/fnut.2025.1564580
Received: 21 January 2025; Accepted: 06 March 2025;
Published: 21 March 2025.
Edited by:
Sofia Viana, University of Coimbra, PortugalReviewed by:
Tania Martins-Marques, University of Coimbra, PortugalCopyright © 2025 Xu, Xie, Liu, Tian, Zhang and Cui. This is an open-access article distributed under the terms of the Creative Commons Attribution License (CC BY). The use, distribution or reproduction in other forums is permitted, provided the original author(s) and the copyright owner(s) are credited and that the original publication in this journal is cited, in accordance with accepted academic practice. No use, distribution or reproduction is permitted which does not comply with these terms.
*Correspondence: Ming Cui, bWluZ2N1aUBiam11LmVkdS5jbg==
†These authors have contributed equally to this work
Disclaimer: All claims expressed in this article are solely those of the authors and do not necessarily represent those of their affiliated organizations, or those of the publisher, the editors and the reviewers. Any product that may be evaluated in this article or claim that may be made by its manufacturer is not guaranteed or endorsed by the publisher.
Research integrity at Frontiers
Learn more about the work of our research integrity team to safeguard the quality of each article we publish.