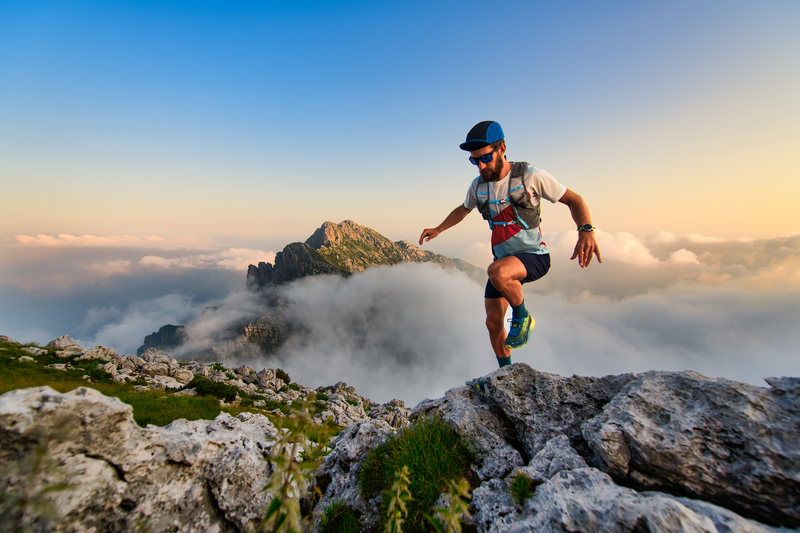
94% of researchers rate our articles as excellent or good
Learn more about the work of our research integrity team to safeguard the quality of each article we publish.
Find out more
ORIGINAL RESEARCH article
Front. Nutr.
Sec. Nutritional Epidemiology
Volume 12 - 2025 | doi: 10.3389/fnut.2025.1530846
This article is part of the Research Topic Analysis of Innovations in Food Development: Improving Nutritional Value, Flavor and Texture in Food Products View all 4 articles
The final, formatted version of the article will be published soon.
You have multiple emails registered with Frontiers:
Please enter your email address:
If you already have an account, please login
You don't have a Frontiers account ? You can register here
Background: Carbohydrates are the major contributor to the energy intake of worldwide population. There is established evidence of links of carbohydrate quality with human health. Knowledge of specific carbohydrate in packaged food, such as added and free sugars, could help further investigate this link, however this information is generally not available Objective: To develop an algorithm to predict the content of free sugars in a global database of packaged foods and beverages; and test the applicability of the algorithm to assess carbohydrate quality in packaged food products from different countries and monitor the evolution over time. Carbohydrate quality was defined using a 10:1|1:2 ratio for carbohydrate, fibers and free sugar i.e. for every 10 g of total carbohydrates in a diet or product, there is at least 1 g of dietary fibers, and less than 2 g of free sugars for every 1 g of dietary fibers.Methods: we used a machine learning approach to predict added and free sugars, which enabled us to predict the carbohydrate quality of products from a global database of packaged food. Our predictions were tested by splitting the dataset into training, validation, and test sets, using US data.Results: we were able to predict free sugars and carbohydrate quality for 424,543 products in the U.S. and in 14 countries. The overall mean absolute error on the test set was 0.96g/100g of product. The predictions generalized with a high accuracy to non-US countries, and we were able to effectively predict the proportion of products meeting the 10:1|1:2 criteria in the food supply of 15 countries Conclusions: our methodology achieved high accuracy and is fully automated; it may be applied to other databases of packaged products and can be easily applied for continuous monitoring of the carbohydrate quality of the global supply of packaged food.
Keywords: Food Supply, free sugar, missing value imputation, Carbohydrate quality, machine learning, Added sugar
Received: 19 Nov 2024; Accepted: 19 Feb 2025.
Copyright: © 2025 Scuccimarra, Arnaud, Tassy, Lê and Mainardi. This is an open-access article distributed under the terms of the Creative Commons Attribution License (CC BY). The use, distribution or reproduction in other forums is permitted, provided the original author(s) or licensor are credited and that the original publication in this journal is cited, in accordance with accepted academic practice. No use, distribution or reproduction is permitted which does not comply with these terms.
* Correspondence:
Fabio Mainardi, Nestlé Research Center, Lausanne, Switzerland
Disclaimer: All claims expressed in this article are solely those of the authors and do not necessarily represent those of their affiliated organizations, or those of the publisher, the editors and the reviewers. Any product that may be evaluated in this article or claim that may be made by its manufacturer is not guaranteed or endorsed by the publisher.
Research integrity at Frontiers
Learn more about the work of our research integrity team to safeguard the quality of each article we publish.