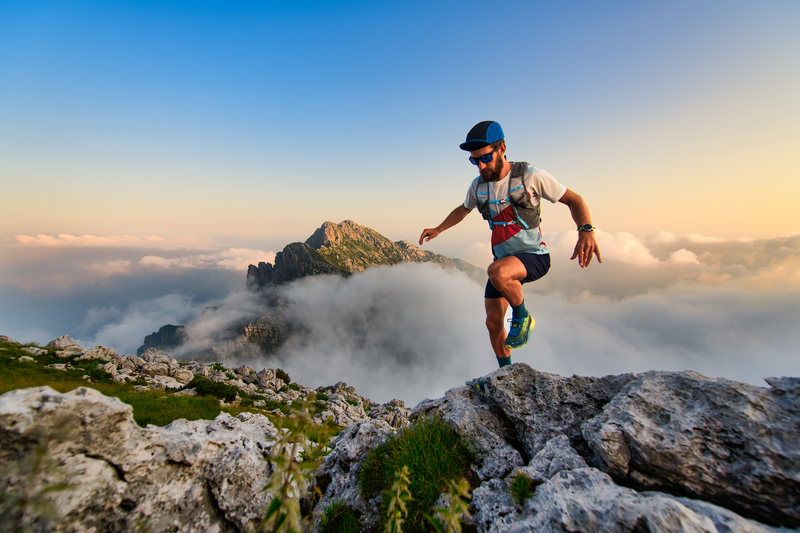
94% of researchers rate our articles as excellent or good
Learn more about the work of our research integrity team to safeguard the quality of each article we publish.
Find out more
ORIGINAL RESEARCH article
Front. Nutr.
Sec. Nutrition and Metabolism
Volume 12 - 2025 | doi: 10.3389/fnut.2025.1525105
This article is part of the Research Topic Subjective Sensations in Obesity and Related Chronic Diseases View all 4 articles
The final, formatted version of the article will be published soon.
You have multiple emails registered with Frontiers:
Please enter your email address:
If you already have an account, please login
You don't have a Frontiers account ? You can register here
Background and objectives: Osteoporosis (OP) has emerged as one of the most rapidly escalating complications associated with diabetes mellitus. However, the potential risk factors contributing to OP in patients with type 2 diabetes mellitus (T2DM) remain controversial. The aim of this study was to explore the relationship between triglyceride glucose-body mass index (TyG-BMI), a marker of insulin resistance calculated as Ln [triglyceride (TG, mg/dL)×fasting plasma glucose (mg/dL)/2]×BMI,and the risk of OP in T2DM patients.This retrospective cross-sectional study enrolled 386 inpatients with T2DM, comprising both male and postmenopausal female participants aged 40 years or older. Individuals with significant medical histories or medications known to influence bone mineral density were excluded. Machine learning algorithms were employed to rank factors affecting OP risk. Logistic regression analysis was performed to identify independent influencing factors for OP, while subgroup analysis was conducted to evaluate the impact of TyG-BMI on OP across different subgroups. Restricted cubic spline (RCS) analysis was used to explore the dose-response relationship between TyG-BMI and OP. Additionally, the receiver operating characteristic (ROC) curve was utilized to assess the predictive efficiency of TyG-BMI for OP.Results: Machine learning analysis identified TyG-BMI as the strongest predictor for type 2 diabetic osteoporosis in middle-aged and elderly patients. After adjusting for confounding factors, multivariate logistic regression analysis revealed that age, osteocalcin, and uric acid were independent influencing factors for OP. Notably, TyG-BMI also emerged as an independent risk factor for OP (95%CI 1.031-1.054, P < 0.01). Subgroup analysis demonstrated a consistent increase in OP risk with higher TyG-BMI levels across all subgroups. RCS analysis indicated a threshold effect, with the risk of OP gradually increasing when TyG-BMI exceeded 191.52. Gender-specific analysis showed increasing the risk of OP when TyG-BMI surpassed 186.21 in males and 198.46 in females, with a more pronounced trend observed in females. ROC suggested that TyG-BMI index has significant discriminative power for type 2 diabetic osteoporosis.TyG-BMI has been identified as a robust predictive biomarker for assessing OP risk in middle-aged and elderly populations with T2DM.
Keywords: type 2 diabetes mellitus, Osteoporosis, Insulin Resistance, triglyceride, Glucose, Body Mass Index, bone mineral density
Received: 08 Nov 2024; Accepted: 26 Feb 2025.
Copyright: © 2025 Chen, Zhang, Qin, Fadong, Ni and Zhong. This is an open-access article distributed under the terms of the Creative Commons Attribution License (CC BY). The use, distribution or reproduction in other forums is permitted, provided the original author(s) or licensor are credited and that the original publication in this journal is cited, in accordance with accepted academic practice. No use, distribution or reproduction is permitted which does not comply with these terms.
* Correspondence:
Jian Zhong, Department of Endocrinology, The Third Affiliated Hospital of Chongqing Medical University, Chongqing, China
Disclaimer: All claims expressed in this article are solely those of the authors and do not necessarily represent those of their affiliated organizations, or those of the publisher, the editors and the reviewers. Any product that may be evaluated in this article or claim that may be made by its manufacturer is not guaranteed or endorsed by the publisher.
Research integrity at Frontiers
Learn more about the work of our research integrity team to safeguard the quality of each article we publish.