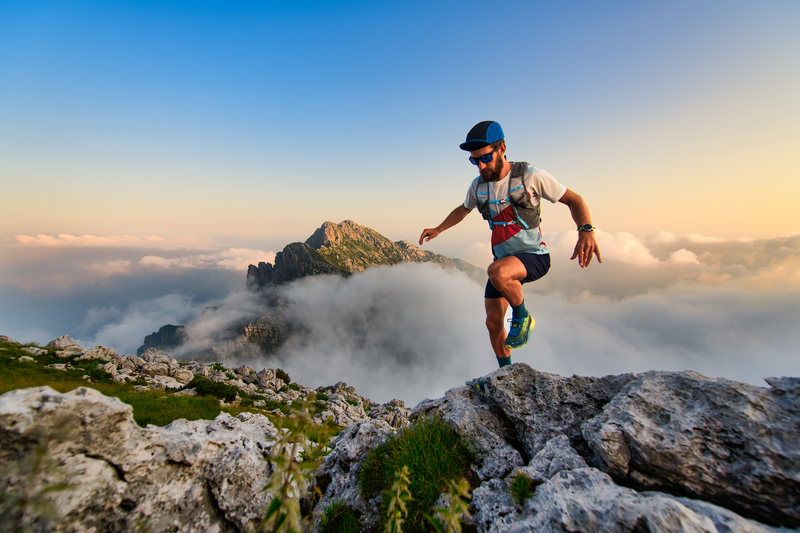
94% of researchers rate our articles as excellent or good
Learn more about the work of our research integrity team to safeguard the quality of each article we publish.
Find out more
ORIGINAL RESEARCH article
Front. Nutr.
Sec. Nutritional Epidemiology
Volume 12 - 2025 | doi: 10.3389/fnut.2025.1519612
This article is part of the Research Topic Preventative Medicine: Nutritional and Lifestyle Interventions for Healthy Ageing and Chronic Diseases View all 36 articles
The final, formatted version of the article will be published soon.
You have multiple emails registered with Frontiers:
Please enter your email address:
If you already have an account, please login
You don't have a Frontiers account ? You can register here
Background: Cardiorenal syndrome (CRS) represents a burgeoning global health concern characterized by its increasing prevalence. Life's Crucial 9 (LC9), an innovative tool for cardiovascular health assessment, and the Dietary Inflammatory Index (DII), which quantifies diet's impact on body inflammation, have not been previously studied in conjunction regarding their association with CRS.Objective: This study aims to explore the relationship between LC9 and CRS, using data from the National Health and Nutrition Examination Survey (NHANES), and to examine whether DII serves as a mediator in this association. Methods: This research included data from 25,792 NHANES participants spanning from 2005 to 2018. The study leverages the dataset's comprehensive representativeness and robust statistical power to ensure generalisable and reliable findings. We employed weighted logistic regression to evaluate the association between LC9 scores and CRS presence, conducted subgroup analyses, and performed mediation analysis to investigate the role of DII. Results: Our analysis demonstrated a significant inverse relationship between LC9 and CRS. Upon controlling for confounders, each 10-point rise in LC9 correlates with a 26% reduction in CRS prevalence (P<0.001). Additionally, stratifying LC9 into tertiles with T1 as the reference group revealed that T2 (OR=0.59, 95% CI=0.48-0.72, P<0.001) and T3 (OR=0.57, 95% CI=0.38-0.88, P<0.001) exhibited a strong negative correlation trend. The dose-response curve illustrates a linear relationship between LC9 and CRS; as LC9 increases, the occurrence of CRS decreases. DII shows a significant positive connection with CRS (P<0.001), but DII indicates a decreasing trend when LC9 rises (β = -0.65, P<0.001). Mediation analysis reveals that DII mediates the association between LC9 and CRS, with a mediation proportion of 12.5% (P<0.001).The findings indicate a robust inverse correlation between LC9 scores and CRS incidence, with DII is associated with this relationship. This suggests potential preventive strategies against CRS through lifestyle modifications guided by LC9.
Keywords: cardiorenal syndrome, Life's Crucial 9, Dietary inflammatory index, Mediation analysis, NHANES
Received: 30 Oct 2024; Accepted: 26 Feb 2025.
Copyright: © 2025 Li and Li. This is an open-access article distributed under the terms of the Creative Commons Attribution License (CC BY). The use, distribution or reproduction in other forums is permitted, provided the original author(s) or licensor are credited and that the original publication in this journal is cited, in accordance with accepted academic practice. No use, distribution or reproduction is permitted which does not comply with these terms.
* Correspondence:
Long Li, Affiliated Hospital of Guizhou Medical University, Guiyang, China
Disclaimer: All claims expressed in this article are solely those of the authors and do not necessarily represent those of their affiliated organizations, or those of the publisher, the editors and the reviewers. Any product that may be evaluated in this article or claim that may be made by its manufacturer is not guaranteed or endorsed by the publisher.
Research integrity at Frontiers
Learn more about the work of our research integrity team to safeguard the quality of each article we publish.