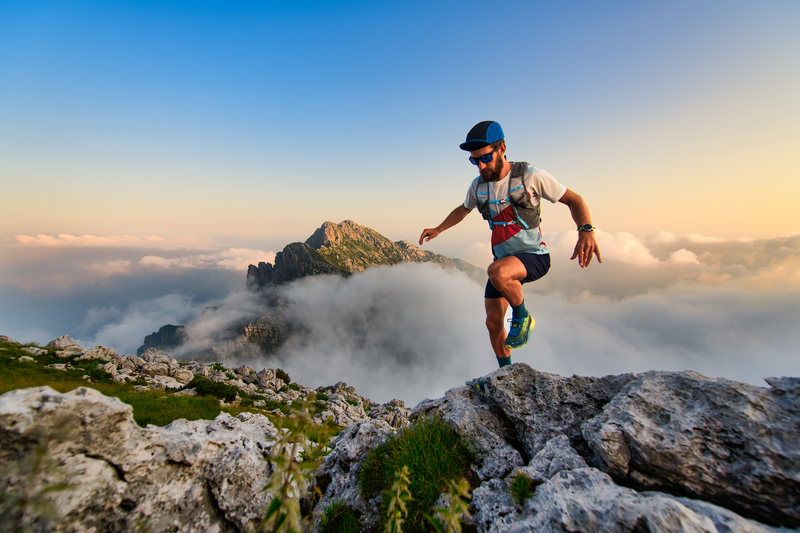
95% of researchers rate our articles as excellent or good
Learn more about the work of our research integrity team to safeguard the quality of each article we publish.
Find out more
BRIEF RESEARCH REPORT article
Front. Nutr. , 05 February 2025
Sec. Nutrition Methodology
Volume 12 - 2025 | https://doi.org/10.3389/fnut.2025.1511230
This article is part of the Research Topic Food-Based Dietary Guidelines View all 9 articles
The United States, and many modern nations, represent assemblies of many cultural groups. Such groups are often influenced, sometimes profoundly, by the culinary traditions of their countries of origin, resulting in a diversity of cultural dietary patterns. Such patterns all derive key elements of nutritional quality from essential food groups—such as vegetables and fruits—but vary in their inclusion of “discretionary” food groups, such as dairy. The application of robust, validated, and standardized diet quality scoring is important in nutrition research, and in the food-as-medicine movement at large if what is being “managed” is to be measured. While robustly validated, the Healthy Eating Index is closely aligned with the Dietary Guidelines for Americans, and thus may not readily account for all multicultural dietary variations. Other diet quality metrics account for deviation from the prevailing American dietary pattern, but none does so in a way that expressly adapts to food components included or excluded so that “credit” for nutritional quality is appropriately assigned in all cases using a standard metric. In this context, we introduce and explain Adaptive Component Scoring as applied to the Healthy Eating Index in the service of advancing fair and universal diet quality scoring. Implications for nutrition research and food-as-medicine initiatives are briefly enumerated.
The Healthy Eating Index (HEI) (1) and the related Alternative Healthy Eating Index (2) are among the most widely used and robustly validated measures of overall diet quality in the United States. These measures have been correlated directly with all-cause mortality and total chronic disease risk in large cohorts (3). Overall diet quality measured accordingly is now recognized as the single leading predictor variable for premature death in the United States (4), and much of the world (5).
Despite these strengths, there are important limitations to the HEI. The metric is closely aligned with the Dietary Guidelines for Americans (6), and accordingly confers credit for food groups that prevail in the American diet, including dairy, meat, poultry, fish, seafood, and grains. Whereas meat, poultry, and fish are assigned to a “protein” category in the HEI scoring construct, for which legumes may substitute, the omission of dairy or grains from a dietary pattern reduces the total, achievable HEI score.
Of note, an array of traditional East and Southeast Asian diets—including one associated with a Blue Zone population (7, 8) omit dairy (9). While categorizable as an omission relative to the HEI construct, these diets in fact never included dairy historically, and only occasionally do so now as elements of the Western diet are globalized. The long-standing inclusion of dairy by select populations, and its exclusion by others, has resulted in marked, demographic variation in the prevalence of lactose tolerance (10). The native, mammalian condition is lactose intolerance after infancy/weaning, and persistence of lactose tolerance throughout the lifespan represents an adaptation by certain human populations (10, 11).
Along with select, traditional Asian diets, vegan diets also exclude dairy. The traditional Paleo diet excludes dairy, and in many applications excludes grains as well (12, 13). Other diet types, whether for disease management, food intolerance, or personal preference, may exclude select food groups such as meat, poultry, fish, dairy products, and/or grain products. While not all of this impact HEI scores, some of them do.
Across a vast expanse of relevant evidence, there is no indication that health outcomes, including the most definitive—vitality and longevity—are adversely affected by the exclusion of dairy when the overall balance of the diet is sound (14). This is certainly true of meat as well, and the same is likely true for the exclusion of grains, although less evidence and fewer real-world examples pertain here. High quality versions of select Asian diets, vegan diets, and potentially Paleo diets are reasonable contenders when dietary patterns “best” for health are under consideration (15–17).
The USA is a multicultural society with a wide range of dietary practices, many based on heritage (18, 19), and others based on alternative nutrition principles and emphasis (e.g., restricting total carbohydrate intake). While routinely applied in this context, the standard application of the HEI may be ill-suited to score diets fairly across this expanse of practices. To address this limitation, and generalize the utility of routine diet quality scoring with a common metric, we introduce a simple adaptation of the HEI.
To adapt the HEI to dietary patterns that exclude select food groups, an initial determination needed to be made about food groups that could reasonably be deemed “discretionary” in balanced, complete, and sustaining dietary patterns. The determination of “discretionary” versus universal food components was made by consensus of the authors, two registered dietitian nutritionists, and one physician expert in nutrition. That consensus was in turn predicated on: (1) work related to mapping the range of dietary patterns currently prevailing in the U.S.A., and to some extent other regions around the world (20); (2) the range of eating patterns represented in worldwide dietary guidelines (21) and clinical practice guidelines (22); (3) the range of dietary patterns saliently associated with longevity and health span (14, 15, 18, 23); and (4) the range of natively adapted human dietary practices (24). Across this breadth of considerations, fruits, vegetables, nuts and seeds were universal; meat, seafood, dairy, grains, and legumes were discretionary. Of note, the characterization of a given food group as discretionary depends partly on other elements in a given dietary pattern. As an example, legumes may be discretionary in a Paleolithic diet that includes meat, seafood, and/or fish, but would not be discretionary in a vegan diet excluding these alternative protein sources. Adaptations were made to the standard HEI scoring construct (1) as shown in Table 1.
The approach to Adaptive Component Scoring was developed to adjust the HEI denominator based on the food groups available to contribute “credit” to the numerator. To create the adapted formula, terms and categories were established as shown in Table 2.
For any given diet, the adjusted scores may be established based on the a priori exclusion of discretionary components, e.g., Asian diets may exclude dairy; Paleo diets may exclude dairy, grains and legumes. See Figure 1 for the adapted formula.
In practice, HEI scoring allows for full protein credit from a range of sources not excluded collectively from any balanced diet, namely: meat, poultry, fish and seafood, and plants (i.e., legumes). Thus, no diet identified required adjustment in this area. A number of diets defined by both cultural parameters and nutritional parameters require adjustment for dairy. See examples in Table 3. Select expressions of certain diets, notably Paleo and low-carb, require adjustment for grains. The maximum HEI score that can be achieved is 90 due to no credit for whole grains. See Table 3 for sample score adjustments.
Table 3. A representative sampling of Healthy Eating Index 2020 scores, with and without Adaptive Component Scoring applied, for optimized versions (i.e., highest achievable HEI score) of select dietary patterns that exclude one or more discretionary food groups.
When stratifying dietary patterns into 10 evenly spaced tiers (deciles) using the HEI-2020, application of Adaptive Component Scoring elevated the scores of the higher tiers for diets excluding dairy and/or grains (see Table 3). This enabled the formulation of an “optimal” diet quality tier for various East Asian diets, and high-fidelity versions of the Paleolithic diet, comparable to scores for dietary patterns with all food groups represented. Absent use of Adaptive Component Scoring, a range of cultural diets, some expressly associated with optimal health outcomes, could not achieve optimal HEI scores.
The quality of a given dietary pattern derives from the quality of health effects it imparts: disease prevention; health promotion; contributions to vitality and longevity. (N.B. Contributions to planetary health are of noteworthy importance, but beyond the scope of the current focus) Invoking such considerations, there is more than one way to achieve a “high quality” diet (15, 19, 25), and no one culture owns a monopoly on the formula. A universally applicable standard for high diet quality predicated on key health outcomes must allow for cultural variations, including the exclusion of a food group that has a traditional place in some cultures, but not others. Adaptive Component Scoring respects the fundamental construct of the Healthy Eating Index, while making this crucial accommodation for cultural variations.
Some food groups are clearly discretionary. There are entire human populations that have no long-standing tradition of dairying, for instance, in which lactose intolerance and the exclusion of dairy from the cultural diet both prevail. There are other populations with long exposure to dairy, and obvious adaptation to it as indicated by widespread lactose tolerance, courtesy of a genetic mutation (26). Of note, both of these groups are represented among the world’s Blue Zones, famous for their healthy life span (25, 27). This salient example illustrates the potential to achieve the same high quality of overall dietary pattern with, and without, dairy. The simple adaptation of the HEI introduced here serves as a quantitative translation of that important principle.
While there are food groups that may be deemed “discretionary” based on modern science, evolutionary biology, and the range of cultural practices, there are clearly food groups that are not. While short-term adjustments might allow for the exclusion of vegetables, fruits, or legumes from the diet, there is no discernible signal across the expanse of evidence sources noted above that such patterns are conducive to optimal health outcomes across the human lifespan. Adaptive Component Scoring was thus directed at those components of an overall dietary pattern that both (a) actually do come and go across an expanse of cultural diversity and prevailing behavior; and (b) can reliably be associated with the same set of health outcomes, summarized as years in life (i.e., longevity), and life in years (i.e., vitality). In practice, this directs the adjustments preferentially to dairy and grains. There is no need to make adjustments for the exclusion of meat, poultry, fish, or seafood, not because these do not occur, but because the HEI already accounts for this by allowing for full credit from plant-derived protein sufficient in quantity and quality (17).
Unadjusted, the HEI can present challenges when applied to dietary patterns that exclude dairy (and/or fortified soy products, which also allow quality points in the HEI) or minimize grain consumption, even if those diets are otherwise nutrient-dense and aligned with health outcomes. While it offers a robust framework for assessing diet quality, it may not fully account for dietary variations that limit these food groups, despite evidence that such diets can still promote optimal health and reduce disease risk. Several alternative scoring systems, including the Mediterranean Diet Score [MDS] (28), Alternative Healthy Eating Index [AHEI] (2), and Plant-Based Diet Index [PDI] (29), allow for reduced or absent intake of dairy or grains while still achieving high scores. These systems acknowledge that diets rich in vegetables, fruits, legumes, nuts, and healthy fats—without necessarily relying on grains or dairy—can still reduce the risk of chronic diseases such as cardiovascular disease and cancer (30, 31). However, each of these metrics is ‘fixed’ rather than adaptive in response to intrinsic dietary variation. The signature distinction of Adaptive Component Scoring is that it is, indeed, “adaptive,” giving credit as it’s due for those food components contributing to overall diet quality. As an example, whole grains are an important contributor to high-quality flexitarian diets, but may be omitted entirely from select expressions of a high-quality Paleolithic diet. Dairy is a signature element in the DASH diet, but is absent from the traditional Okinawan diet.
Other types of scoring systems, such as the Dietary Inflammatory Index [DII] (32) and NOVA classification [NOVA] (33), focus on the processing and inflammatory potential of foods rather than specific food groups, reflecting a more global, multi-cultural perspective. These approaches, too, are fixed, and not directly responsive to variation in the sources of key dietary inputs. This highlights the value of developing adaptive scoring methods that better accommodate diverse dietary patterns, including those that exclude or minimize dairy or grains, without compromising the ability to measure diet quality across various cultural and nutritional styles. Such flexibility can enhance inclusivity while maintaining the strengths of established tools like the HEI.
Attention to the diverse means of elevating overall dietary quality for a multicultural society is increasing, but has historically been limited (34). Among the important implications of this focus is the opportunity to standardize diet quality without standardizing diet type in intervention studies and food-as-medicine initiatives. The food-as-medicine movement (35) is directed to the level of population, and in particular, to population groups that are most food—and nutrition-insecure. Such groups are particularly multicultural. Familiarity is well established as a key driver of dietary preference, and adherence to prescribed diets predicated on a “one-size-fits-all” approach for a diverse population is known to be rate-limiting in their impacts; long-term adherence is a particular limitation (36).
An adaptation of the HEI for multicultural deployments offers the promise of innovations in nutrition research and service that could reduce attrition, enhance adherence, improve satisfaction, and generalize far more readily. In the food-as-medicine movement, efforts directed at the improvement of health outcomes by means of improved diet quality call for routine and standardized measurement of what is being managed. For diet quality assessment to be practiced fairly across such an expanse, it must be adapted to diverse, cultural patterns of dietary intake.
As an example of application of ACS under real-world conditions, our work involves both assessing current diet (habitual intake, rather than per-day intake), and providing guidance toward a personalized goal diet. The “improvement” in diet both intended, and achieved, is measured by change in HEI score—both for individuals, and the population. This, in turn, requires the attachment of HEI scores to goal diets. As our work involves culturally diverse populations, the personalization of goal diets also involves a multicultural array of dietary patterns. Empirically, we observed that optimized versions of select culturally diets, such as various Asian diets that omit dairy, garnered lower HEI scores than comparably, wholesome dietary patterns in other cultural lanes, inclusive of all food groups. We apply ACS when exclusion of a given HEI food group pertains because of high-fidelity adherence to a dietary type that omits that food group, generally at the higher levels (e.g., top 3 deciles) of the HEI scoring range. Application of ACS in this context serves as intended to “level the playing field,” generating comparable quality (HEI) scores for multicultural goal diets satisfying comparable nutritional parameters, while varying slightly in the food groups from which such nutrients are derived. The application of ACS to current dietary intake, along with dietary goal-setting, correspondingly pertains when (a) measurement is of habitual, not per-day, intake; and (b) that same high-fidelity adherence to optimized (i.e., upper HEI deciles) has been achieved.
We note that the generation of HEI scores predicated on dietary intake assessment presupposes, and indeed requires, that the dietary intake assessment methods applied are reliable, valid, and pertain to habitual rather than episodic intake. The same constraints pertain to the application of ACS, for which the generation of HEI scores is prerequisite.
Objective measures of diet quality are useful at both the individual and population level in risk stratification (2); in translating risk into projected costs (37); and in gauging the progress achieved in any given clinical nutrition or food-as-medicine intervention (38). Diet quality, measured objectively, has been cited as the single leading predictor variable for total chronic disease risk and premature death in developed countries around the world (5), with notable attention to that association in the United States (39). Change in overall diet quality, using a standard measure, is a useful outcome measure in nutrition research (40). Finally, overall diet quality is an important parameter to consider for both individuals and populations when establishing dietary goals. The application of ACS expands the array of dietary patterns that can meet or achieve a given quality threshold, thus expanding opportunities to tailor nutrition prescriptions to culture and native preference and measure diet quality improvement in both individuals and populations across an expanse of cultural diversity.
The introduction of Adaptive Component Scoring is intended to advance such objectives. The utility of this innovation will best be tested and affirmed in just such context.
The datasets presented in this article are not readily available because use of the ACS is intended for the public domain, and pertinent data sets are accessible via public access to the Health Eating Index 2020 scoring construct. Requests to access the datasets should be directed to Lauren Q. Rhee,bGF1cmVuLnJoZWVAam9pbnRhbmdlbG8uY29t.
DK: Conceptualization, Methodology, Writing – original draft, Writing – review & editing. LR: Data curation, Writing – review & editing. DA: Writing – review & editing.
The author(s) declare that no financial support was received for the research, authorship, and/or publication of this article.
DK, LR, and DA were employed by Diet ID/Tangelo (Intend, Inc.).
The author(s) declare that no Gen AI was used in the creation of this manuscript.
All claims expressed in this article are solely those of the authors and do not necessarily represent those of their affiliated organizations, or those of the publisher, the editors and the reviewers. Any product that may be evaluated in this article, or claim that may be made by its manufacturer, is not guaranteed or endorsed by the publisher.
1. Shams-White, MM, Pannucci, TE, Lerman, JL, Herrick, KA, Zimmer, M, Meyers Mathieu, K, et al. Healthy Eating Index-2020: review and update process to reflect the Dietary Guidelines for Americans, 2020-2025. J Acad Nutr Diet. (2023) 123:1280–8. doi: 10.1016/j.jand.2023.05.015
2. Chiuve, SE, Fung, TT, Rimm, EB, Hu, FB, McCullough, ML, Wang, M, et al. Alternative dietary indices both strongly predict risk of chronic disease. J Nutr. (2012) 142:1009–18. doi: 10.3945/jn.111.157222
3. Shan, Z, Li, Y, Baden, MY, Bhupathiraju, SN, Wang, DD, Sun, Q, et al. Association between healthy eating patterns and risk of cardiovascular disease. JAMA Intern Med. (2020) 180:1090–100. doi: 10.1001/jamainternmed.2020.2176
4. Wang, DD, Li, Y, Afshin, A, Springmann, M, Mozaffarian, D, Stampfer, MJ, et al. Global improvement in dietary quality could lead to substantial reduction in premature death. J Nutr. (2019) 149:1065–74. doi: 10.1093/jn/nxz010
5. GBD 2017 Diet Collaborators. Health effects of dietary risks in 195 countries, 1990-2017: a systematic analysis for the Global Burden of Disease study 2017. Lancet. (2019) 393:1958–72. doi: 10.1016/S0140-6736(19)30041-8
6. Dietary Guidelines for Americans (2020). Available at: https://www.dietaryguidelines.gov/ (Accessed September 23, 2024).
7. Huang, Y, and Mark, JG. Identification of a Blue Zone in a typical Chinese longevity region. Int J Environ Res Public Health. (2017) 14:571. doi: 10.3390/ijerph14060571
8. Okinawa, Japan (2024). Secrets of the world's longest-living women. Available at: https://www.bluezones.com/explorations/okinawa-japan/. (Accessed September 23, 2024).
9. Asian Heritage Diet (2024). Oldways. Available at: https://oldwayspt.org/traditional-diets/asian-heritage-diet (Accessed September 23, 2024).
10. Tsai, PM, and Duggan, C. Malabsorption syndromes: nutritional management In: Benjamin Caballero, editor. Encyclopedia of human nutrition. Third ed. San Diego, CA: Elsevier Academic Press (2013). 136–42. doi: 10.1016/B978-0-12-375083-9.00178-1
11. Kaufman, EJ, and Tan, C. White as milk: biocentric bias in the framing of lactose intolerance and lactase persistence. Sociol Health Illn. (2022) 44:1533–50. doi: 10.1111/1467-9566.13528
12. Singh, A, and Singh, D. The Paleolithic diet. Cureus. (2023) 5:e34214. doi: 10.7759/cureus.34214
13. Harvard School of Public Health (2024). The Nutrition Source. Diet review: Paleo diet for weight loss. Available at: https://nutritionsource.hsph.harvard.edu/healthy-weight/diet-reviews/paleo-diet/ (Accessed September 23, 2024).
14. Katz, DL, Friedman, RS, Essel, KD, Joshi, S, and Levitt, J. Nutrition in clinical practice (2022). Available at: https://advisor.lwwhealthlibrary.com/book.aspx?bookid=3126§ionid=0 (Accessed September 23, 2024).
15. Katz, DL, and Meller, S. Can we say what diet is best for health? Annu Rev Public Health. (2014) 35:83–103. doi: 10.1146/annurev-publhealth-032013-182351
16. Hess, JM, and Comeau, ME. Application of dairy-free vegetarian and vegan USDA food pattern models for non-pregnant, non-lactating healthy adults. J Food Sci. (2022) 87:4703–13. doi: 10.1111/1750-3841.16314
17. Hertzler, SR, Lieblein-Boff, JC, Weiler, M, and Allgeier, C. Plant proteins: assessing their nutritional quality and effects on health and physical function. Nutrients. (2020) 12:3704. doi: 10.3390/nu12123704
18. LeBlanc, KE, Baer-Sinnott, S, Lancaster, KJ, Campos, H, Lau, KHK, Tucker, KL, et al. Perspective: beyond the Mediterranean diet-exploring Latin American, Asian, and African Heritage Diets as cultural models of healthy eating. Adv Nutr. (2024) 15:100221. doi: 10.1016/j.advnut.2024.100221
19. Traditional Diets (2024). Oldways. Available at: https://oldwayspt.org/explore-heritage-diets/why-traditional-diets/ (Accessed September 23, 2024).
20. Katz, DL, and Rhee, L. (2022). Diet mapping processes and systems to optimize diet quality and/or minimize environmental impact. (U.S. patent number: 11328810). U.S. Patent and Trademark Office.
21. Cámara, M, Giner, RM, González-Fandos, E, López-García, E, Mañes, J, Portillo, MP, et al. Food-based dietary guidelines around the world: a comparative analysis to update AESAN Scientific Committee dietary recommendations. Nutrients. (2021) 13:3131. doi: 10.3390/nu13093131
22. Cara, KC, Goldman, DM, Kollman, BK, Amato, SS, Tull, MD, and Karlsen, MC. Commonalities among dietary recommendations from 2010 to 2021 clinical practice guidelines: a meta-epidemiological study from the American College of Lifestyle Medicine. Adv Nutr. (2023) 14:500–15. doi: 10.1016/j.advnut.2023.03.007
23. Buettner, D. (2017). The Blue Zones solution. Eating and Living Like the World’s Healthiest People. National Geographic. Washington, D.C.
24. De La, OV, Zazpe, I, Martínez, JA, Santiago, S, Carlos, S, Zulet, MÁ, et al. Scoping review of Paleolithic dietary patterns: a definition proposal. Nutr Res Rev. (2021) 34:78–106. doi: 10.1017/S0954422420000153
25. Blue Zones (2024). Food Guidelines. Available at: https://www.bluezones.com/recipes/food-guidelines/ (Accessed September 23, 2024).
26. Comerford, KB, and Pasin, G. Gene-dairy food interactions and health outcomes: a review of nutrigenetic studies. Nutrients. (2017) 9:710. doi: 10.3390/nu9070710
27. Buettner, D, and Skemp, S. Blue Zones: lessons from the world's longest lived. Am J Lifestyle Med. (2016) 10:318–21. doi: 10.1177/1559827616637066
28. Trichopoulou, A, Costacou, T, Bamia, C, and Trichopoulos, D. Adherence to a Mediterranean diet and survival in a Greek population. N Engl J Med. (2003) 348:2599–608. doi: 10.1056/NEJMoa025039
29. Satija, A, Bhupathiraju, SN, Rimm, EB, Spiegelman, D, Chiuve, SE, Borgi, L, et al. Plant-based dietary patterns and incidence of type 2 diabetes in US men and women: results from three prospective cohort studies. PLoS Med. (2016) 13:e1002039. doi: 10.1371/journal.pmed.1002039
30. Schwingshackl, L, Hoffmann, G, Kalle-Uhlmann, T, Arregui, M, Buijsse, B, and Boeing, H. Fruit and vegetable consumption and changes in anthropometric variables in adult populations: a systematic review and meta-analysis of prospective cohort studies. PLoS One. (2015) 10:e0140846. doi: 10.1371/journal.pone.0140846
31. Satija, A, Bhupathiraju, SN, Spiegelman, D, Chiuve, SE, Manson, JAE, Willett, W, et al. Healthful and unhealthful plant-based diets and the risk of coronary heart disease in U.S. adults. J Am Coll Cardiol. (2017) 70:411–22. doi: 10.1016/j.jacc.2017.05.047
32. Shivappa, N, Steck, SE, Hurley, TG, Hussey, JR, and Hébert, JR. Designing and developing a literature-derived, population-based dietary inflammatory index. Public Health Nutr. (2014) 17:1689–96. doi: 10.1017/S1368980013002115
33. Monteiro, CA, Cannon, G, Levy, RB, Moubarac, JC, Louzada, ML, Rauber, F, et al. Ultra-processed foods: what they are and how to identify them. Public Health Nutr. (2019) 22:936–41. doi: 10.1017/S1368980018003762
34. Krishna, P. (2020). Is American dietetics a white-bread world? These dietitians think so. The New York Times. Available at: https://www.nytimes.com/2020/12/07/dining/dietitian-diversity.html. (Accessed September 23, 2024).
35. Food is Medicine: Current Initiative (2024). The Rockefeller Foundation. Available at: https://www.rockefellerfoundation.org/initiative/food-is-medicine/ (Accessed September 23, 2024).
36. Vilaro, MJ, Staub, D, Xu, C, and Mathews, AE. Theory-based interventions for long-term adherence to improvements in diet quality: an in-depth review. Am J Lifestyle Med. (2016) 10:369–76. doi: 10.1177/1559827616661690
37. Katz, DL, Govani, R, Anderson, K, Rhee, LQ, and Aronson, DL. The financial case for food as medicine: introduction of a ROI calculator. Am J Health Promot. (2022) 36:768–71. doi: 10.1177/08901171211070751
38. Vadiveloo, M, Lichtenstein, AH, Anderson, C, Aspry, K, Foraker, R, Griggs, S, et al. Rapid diet assessment screening tools for cardiovascular disease risk reduction across healthcare settings: a scientific statement from the American Heart Association. Circ Cardiovasc Qual Outcomes. (2020) 13:e000094. doi: 10.1161/HCQ.0000000000000094
39. Mozaffarian, D, and Glickman, D. (2019). Opinion: our food is killing too many of us. The New York Times. Available at: https://www.nytimes.com/2019/08/26/opinion/food-nutrition-health-care.html (Accessed December 30, 2024).
Keywords: diet quality, dietary index, diet score, Healthy Eating Index, dietary patterns, multicultural diets, nutrition, food groups
Citation: Katz DL, Rhee LQ and Aronson DL (2025) Application of the Healthy Eating Index in a multicultural population: introduction of Adaptive Component Scoring. Front. Nutr. 12:1511230. doi: 10.3389/fnut.2025.1511230
Received: 14 October 2024; Accepted: 20 January 2025;
Published: 05 February 2025.
Edited by:
Norman Temple, Athabasca University, CanadaReviewed by:
Diane Mitchell, Texas A&M AgriLife Research, United StatesCopyright © 2025 Katz, Rhee and Aronson. This is an open-access article distributed under the terms of the Creative Commons Attribution License (CC BY). The use, distribution or reproduction in other forums is permitted, provided the original author(s) and the copyright owner(s) are credited and that the original publication in this journal is cited, in accordance with accepted academic practice. No use, distribution or reproduction is permitted which does not comply with these terms.
*Correspondence: David L. Katz, a2F0emRsQGF5YS55YWxlLmVkdQ==; ZGF2aWQua2F0ekBqb2ludGFuZ2Vsby5jb20=
Disclaimer: All claims expressed in this article are solely those of the authors and do not necessarily represent those of their affiliated organizations, or those of the publisher, the editors and the reviewers. Any product that may be evaluated in this article or claim that may be made by its manufacturer is not guaranteed or endorsed by the publisher.
Research integrity at Frontiers
Learn more about the work of our research integrity team to safeguard the quality of each article we publish.