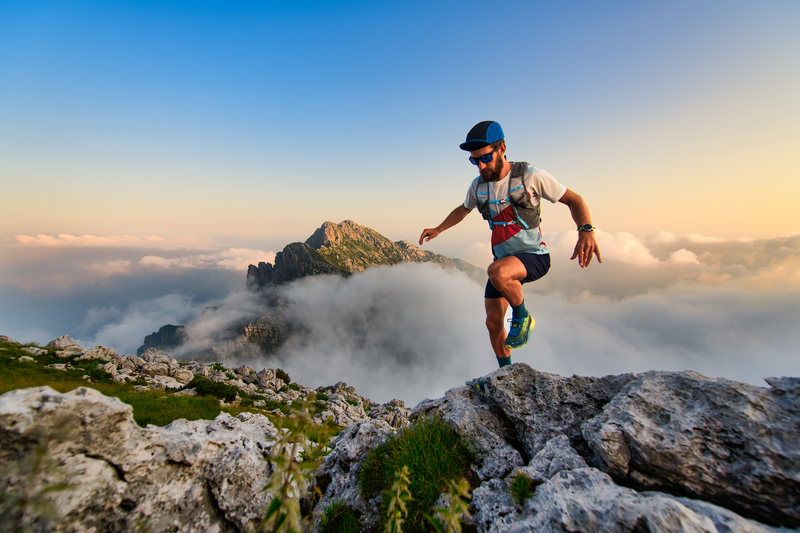
94% of researchers rate our articles as excellent or good
Learn more about the work of our research integrity team to safeguard the quality of each article we publish.
Find out more
ORIGINAL RESEARCH article
Front. Nutr.
Sec. Nutritional Epidemiology
Volume 12 - 2025 | doi: 10.3389/fnut.2025.1485456
This article is part of the Research Topic Ultra-Processed Foods: Impact on Dietary Patterns, Health Outcomes, and Policy Interventions View all 3 articles
The final, formatted version of the article will be published soon.
You have multiple emails registered with Frontiers:
Please enter your email address:
If you already have an account, please login
You don't have a Frontiers account ? You can register here
The rising intake of ultra-processed foods (UPFs) has been linked to adverse health outcomes, yet its impact on aging acceleration remains unclear.Objective: This study aimed to examine the association between the percentage of total daily calories (%Kcal) and grams (%Gram) from UPFs and phenotypic age acceleration (PhenoAgeAccel).Methods: Data from 12,079 adults in the NHANES 2005-2010 cycles were analyzed.The relationship between UPFs intake and PhenoAgeAccel was assessed using multivariable linear regression and restricted cubic splines, with adjustments for relevant covariates. The mediating role of body mass index (BMI) was also explored.Results: A significant positive linear association was observed between UPFs intake (%Gram) and PhenoAgeAccel, with the highest quartile showing an increase of 0.60 (95% CI: 0.15, 1.05; p for trend = 0.039), but no association was found between UPFs intake (%Kcal) and PhenoAgeAccel. Mediation analysis indicated that BMI mediated 27.5% of the association between UPFs intake (%Gram) and PhenoAgeAccel.Sensitivity analyses confirmed the robustness of the results.Conclusions: Higher intake of UPFs intake (%Gram) is positively associated with PhenoAgeAccel, with BMI playing a significant mediating role.
Keywords: Ultra-Processed foods, Phenotypic age acceleration, Body Mass Index, NHANES, Aging
Received: 23 Aug 2024; Accepted: 28 Feb 2025.
Copyright: © 2025 Kong, Xie, Chen and Xiong. This is an open-access article distributed under the terms of the Creative Commons Attribution License (CC BY). The use, distribution or reproduction in other forums is permitted, provided the original author(s) or licensor are credited and that the original publication in this journal is cited, in accordance with accepted academic practice. No use, distribution or reproduction is permitted which does not comply with these terms.
* Correspondence:
Weiliang Kong, Ningbo First Hospital, Ningbo, China
Disclaimer: All claims expressed in this article are solely those of the authors and do not necessarily represent those of their affiliated organizations, or those of the publisher, the editors and the reviewers. Any product that may be evaluated in this article or claim that may be made by its manufacturer is not guaranteed or endorsed by the publisher.
Research integrity at Frontiers
Learn more about the work of our research integrity team to safeguard the quality of each article we publish.