- 1Department of Gastrointestinal Surgery, the First Affiliated Hospital of Chongqing Medical University, Chongqing, China
- 2Department of Clinical Nutrition, The First Affiliated Hospital of Chongqing Medical University, Chongqing, China
Aim: The aim of this study was to develop a validated nomogram to predict the risk of postoperative complications in colorectal cancer (CRC) patients by analyzing the factors that contribute to these complications.
Methods: We retrospectively collected clinical information on patients who underwent CRC surgery at a single clinical center from January 2021 to December 2021. Univariate and multivariate logistic regression analysis to identify independent risk factors for postoperative complications and to develop a predictive model. A receiver operating characteristic (ROC) curve was used to calculate the area under the curve (AUC) to assess the predicted probability. Calibration curve was drawn to compare the predicted probability of the nomogram with the actual probability, and decision curve analysis (DCA) was employed to evaluate the clinical utility of the nomogram.
Results: A total of 190 CRC patients were included in this study. We retrospectively collected baseline information, clinical information, surgical information, and nutrition-related indicators for all patients. Through multivariate logistic regression analysis, preoperative albumin (p = 0.041, OR = 0.906, 95% CI = 0.824–0.996), surgical time (p = 0.009, OR = 1.006, 95% CI = 1.001–1.010), waistline (p = 0.049, OR = 1.011, 95% CI = 1.002–1.020) and phase angle (PA) (p = 0.022, OR = 0.615, 95% CI = 0.405–0.933) were identified as independent risk factors for postoperative complications in CRC, and a nomogram prediction model was established using the above four variables. The AUC of 0.706 for the ROC plot and the high agreement between predicted and actual probabilities in the calibration curves suggested that the prediction model has good predictive power. The DCA also confirmed the good clinical performance of the nomogram.
Conclusion: This study developed a nomogram to predict the risk of postoperative complications in CRC patients, providing surgeons with a reliable reference to personalized patient management in the perioperative period and preoperative nutritional interventions.
Introduction
According to Global Cancer Data 2020, colorectal cancer (CRC) has become the third most common new cancer diagnosis and the second leading cause of cancer-related deaths worldwide (1–3). In 2020, approximately 1,931,590 people were diagnosed with CRC and 935,173 people died from CRC (4, 5). The World Health Organization (WHO) estimates that by 2030, new cases of CRC will increase by 77% and deaths from CRC will increase by 80% (6, 7). The main treatments for CRC include surgery, local radiotherapy and systemic treatments such as chemotherapy and targeted therapy (8, 9).
Currently, radical surgery remains the cornerstone of CRC treatment (10, 11). Several previous studies have shown that postoperative complications affect the prognosis of CRC patients (12–14). Because CRC affects the intake and absorption of food and nutrients, it often leads to malnutrition in patients (15, 16). Malnutrition can cause changes in immune, respiratory and renal function and impairs wound healing (17, 18). Therefore, comprehensive preoperative assessment of the patient’s nutritional status and timely intervention should receive the full attention of clinicians.
Various objective measures are often used to assess the nutritional status of patients, mainly including anthropometric measures, Subjective Gross Assessment (SGA), Nutritional Risk Score 2002 (NRS2002) and Bioelectrical impedance analysis (BIA) related indicators (19, 20). Anthropometric measurements mainly include body mass index (BMI), waistline, hipline, calf line and triceps skin fold (TSF).
SGA is a nutritional assessment of patients based on subjective patient interviews combined with objective measurement data. Patient-generated SGA (PG-SGA) is an adaptation of SGA and is specifically used as a reference method to assess nutritional status in cancer patients (21, 22). Several studies have shown that PG-SGA is associated with a poorer clinical prognosis in cancer patients (23–25). The PG-SGA primarily assesses the patient’s weight change over a 2-week period, changes in dietary intake, symptoms of nutritional effects, activity and function, and physical examination findings (26).
NRS-2002 is a screening tool for malnutrition, endorsed by the European Society for Clinical Nutrition and Metabolism (ESPEN) for used in hospitalized patients (27, 28). The NRS-2002 is divided into two sections: the patient’s nutritional status and current illness and severity, which are assessed on the basis of the patient’s age, weight loss, body mass index (BMI), food intake and severity of illness (29).
The aim of this study was to collect clinical information and preoperative nutrition-related indicators from CRC patients, analyze the factors leading to postoperative complications, and to establish a valid nomogram to predict the risk of these complications.
Materials and methods
Patient selection
We retrospectively collected information on patients who underwent CRC surgery from January 2021 to December 2021 at a single clinical center, including baseline information, clinical information, and surgical information. Inclusion criteria were patients with preoperative pathologically confirmed colorectal malignancy who underwent laparoscopic radical surgery. Exclusion criteria were as follows: 1. Patients who underwent CRC surgery after recurrence; 2. Patients with metastatic CRC; and 3. Patients with incomplete baseline or surgical information. Finally, 190 patients were included.
This study was reviewed by the Ethics Committee of the First Affiliated Hospital of Chongqing Medical University (2022–135-2). It complied with the principles of medical ethics and the Declaration of Helsinki, and all patients participating in the study signed an informed consent form.
Data elements
We retrospectively collected baseline information, clinical information, surgical information, and nutrition-related indicators from patients. Baseline information included age, sex, BMI, smoking and drinking history, and preoperative comorbidities. Clinical information included preoperative albumin and hemoglobin, tumor location, tumor stage and tumor size. Surgical information included surgical time, blood loss and postoperative complications. Nutrition-related indicators included waistline, hipline, calf line, TSF, NRS2002, PG-SGA and BIA related indicators.
Preoperative comorbidities included hypertension and type 2 diabetes mellitus (T2DM). The tumor was comprehensively staged according to the 8th edition of the AJCC guidelines and was classified as stage I-IV (30).
BIA
BIA is a non-invasive, practical, objective and simple method of assessing body composition and measuring nutritional status that can be applied to both healthy and patient populations (18, 19, 31–34). BIA uses the measurement of impedance as low amplitude (800 mA) and high frequency (50 kHz) electrical currents pass through the body to calculate indicators of body composition such as muscle, fat, cell mass and volumetric loading status (35, 36).
BIA is a body composition analysis technique that quantifies various body tissue components and directly measures impedance, phase angle (PA) and other metrics (37, 38). The indices measured by BIA in this study included: appendicular skeletal muscle mass index (ASMMI), body cell mass (BCM), body cell mass index (BCMI), extracellular water (ECW), fat free mass (FFM), fat free mass index (FFMI), fat mass (FM), fat mass index (FMI), PA, skeletal muscle index (SMI), skeletal muscle mass (SMM), total body water (TBW) (Figure 1).
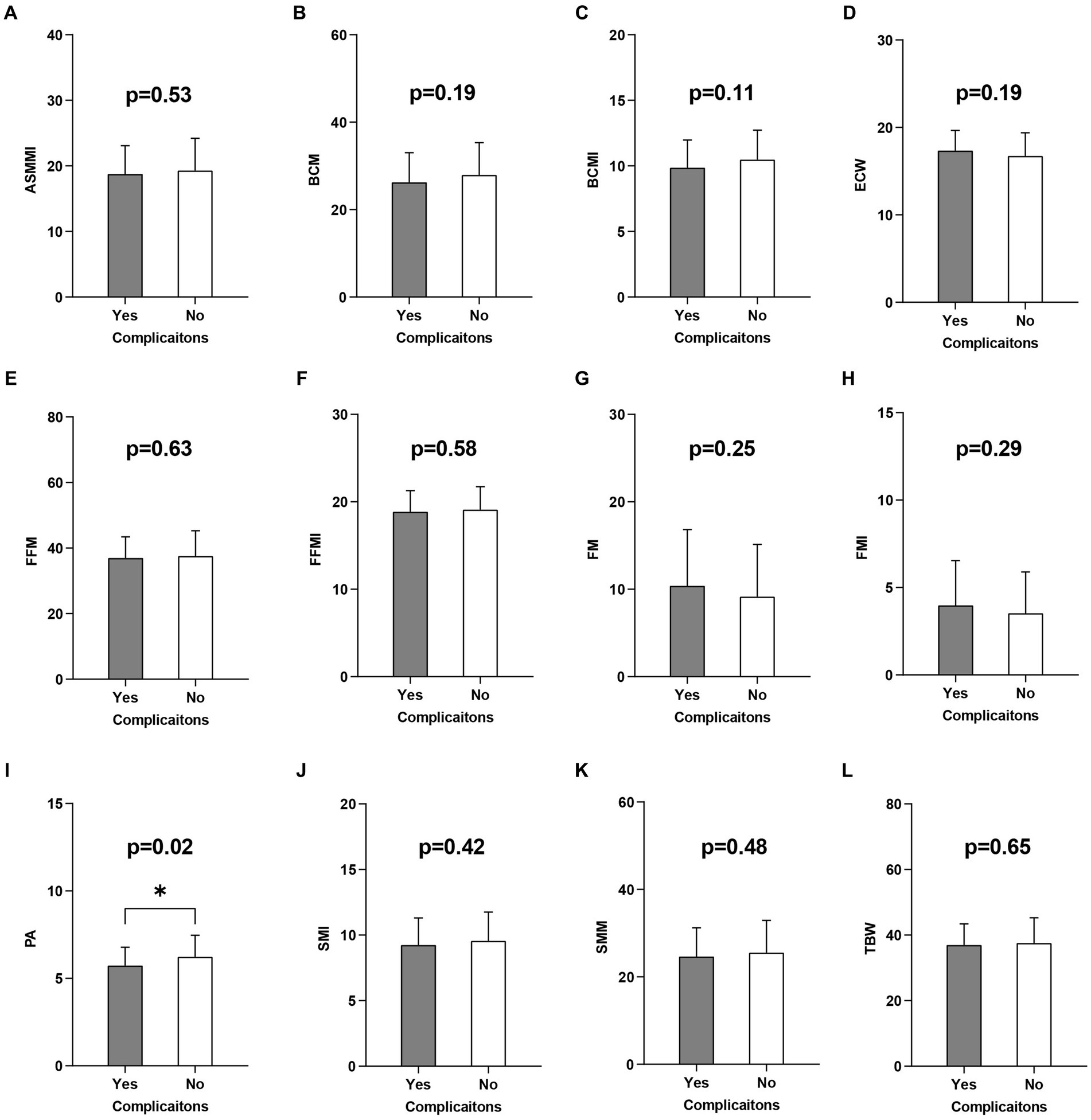
Figure 1. Univariate logistic regression analysis of the BIA related indicators. BIA, Bioelectrical impedance analysis; ASMMI, appendicular skeletal muscle mass index; BCM, body cell mass; BCMI, body cell mass index; ECW, extracellular water; FFM, fat free mass; FFMI, fat free mass index; FM, fat mass; FMI, fat mass index; PA, phase angle; SMI, skeletal muscle index; SMM, skeletal muscle mass; TBW, total body water. (A-L) are the results of a univariate logistic analysis of the above indicators of the development of postoperative complications.
Statistical analysis
All data in this study were processed using SPSS (version 22.0) and R (version 4.1.2). Continuous variables that followed a normal distribution were expressed as mean ± standard deviation (SD) and comparisons were made using t-test; continuous variables that did not follow a normal distribution were expressed as median and interquartile range (IQR) and assessed using chi-squared or Fisher’s exact test; and categorical variables were expressed as numbers and percentages. Univariate logistic regression analysis was performed on all variables, and variables with p < 0.05 were considered potential risk factors for the occurrence of postoperative complications in CRC patients. The screened potential risk factors were then subjected to multivariate logistic regression analysis to identify independent predictors of complications after CRC surgery. Finally, variables with p < 0.05 in the multivariate logistic regression analysis were included and a nomogram was created to predict the risk of postoperative complications in CRC.
The predictive model was evaluated in three ways. First, the predictive value of the risk factors was verified using the receiver operating characteristic (ROC) curve, and the area under the curve (AUC) was calculated to assess the performance of the nomogram. The AUC took values between 0 and 1, with 1 indicating perfect agreement, 0.5 indicating no better than chance, and greater than 0.7 indicating that the model has relatively good predictive power (39, 40). Secondly, prediction curves were plotted to test the correction of the postoperative complication risk map, comparing the predicted and actual probabilities of the nomogram, using the 45 degree line as a perfect model with 100% accuracy (41). Finally, decision curve analysis (DCA) was used to analyze the net benefit based on different threshold probabilities to determine the clinical applicability of the nomogram (42, 43).
Results
Baseline information
Based on the above inclusion and exclusion criteria, a total of 190 patients who underwent CRC surgery were included in this study. This included 42 patients in the complication group and 148 patients in the no complication group. Comparing the baseline information of the two groups of patients, we found a statistical difference (p < 0.05), including preoperative albumin (p = 0.005), surgical time (p = 0.004), blood loss (p = 0.010), and waistline (p = 0.043; Table 1).
Nomogram variable screening
Univariate and multivariate logistic regression analysis were performed on a total of 32 potential factors, including baseline information, clinical information, surgical information, and nutrition-related indicators, to find the risk factors affecting the occurrence of complications after CRC surgery. The results of univariate logistic regression analysis showed that preoperative albumin (p = 0.005, OR = 0.879, 95% CI = 0.804–0.962), surgical time (p = 0.009, OR = 1.006, 95% CI = 1.001–1.010), waistline (p = 0.046, OR = 1.042, 95% CI = 1.001–1.084) and PA (p = 0.016, OR = 0.622, 95% CI = 0.424–0.914) were the potential risk factors for postoperative complications of CRC (Table 1; Figure 1). Further multivariate logistic regression analysis of the four potential risk factors showed that preoperative albumin (p = 0.041, OR = 0.906, 95% CI = 0.824–0.996), surgical time (p = 0.009, OR = 1.006, 95% CI = 1.001–1.010), waistline (p = 0.049, OR = 1.011, 95% CI = 1.002–1.020) and PA (p = 0.022, OR = 0.615, 95% CI = 0.405–0.933) were independent risk factors for the occurrence of postoperative complications in CRC (Table 2).
Nomogram construction and validation
A nomogram model for predicting the risk of postoperative complications in CRC patients was constructed using four independent risk factors identified by multivariate logistic regression analysis. As shown in Figure 2, the corresponding scores of each factor were obtained according to the patients’ own actual situation, and the total score was obtained by adding the four scores, and the final predicted risk of postoperative complications was the probability corresponding to an individual patient’s total score.
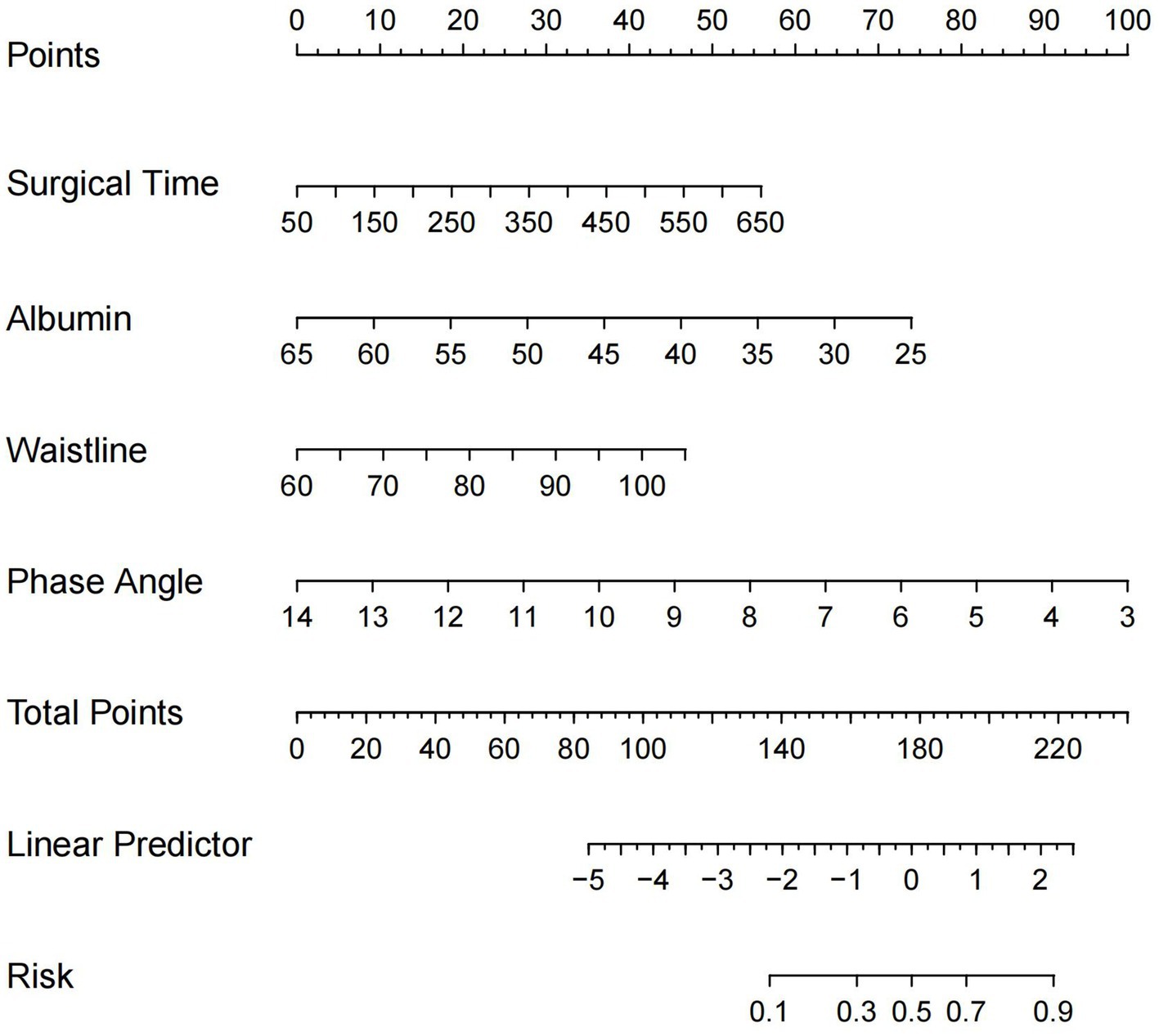
Figure 2. Nomogram for predicting the risk of postoperative complications after CRC surgery. CRC, colorectal cancer.
By ROC analysis, AUC of the nomogram over time was 0.706, indicating that the model has good predictive performance (Figure 3). The calibration curve showed that there was a high degree of agreement between the predicted and observed results of the nomogram model constructed in this study (Figure 4). Finally, DCA was used to evaluate the clinical application value of the prediction model, as shown in Figure 5.
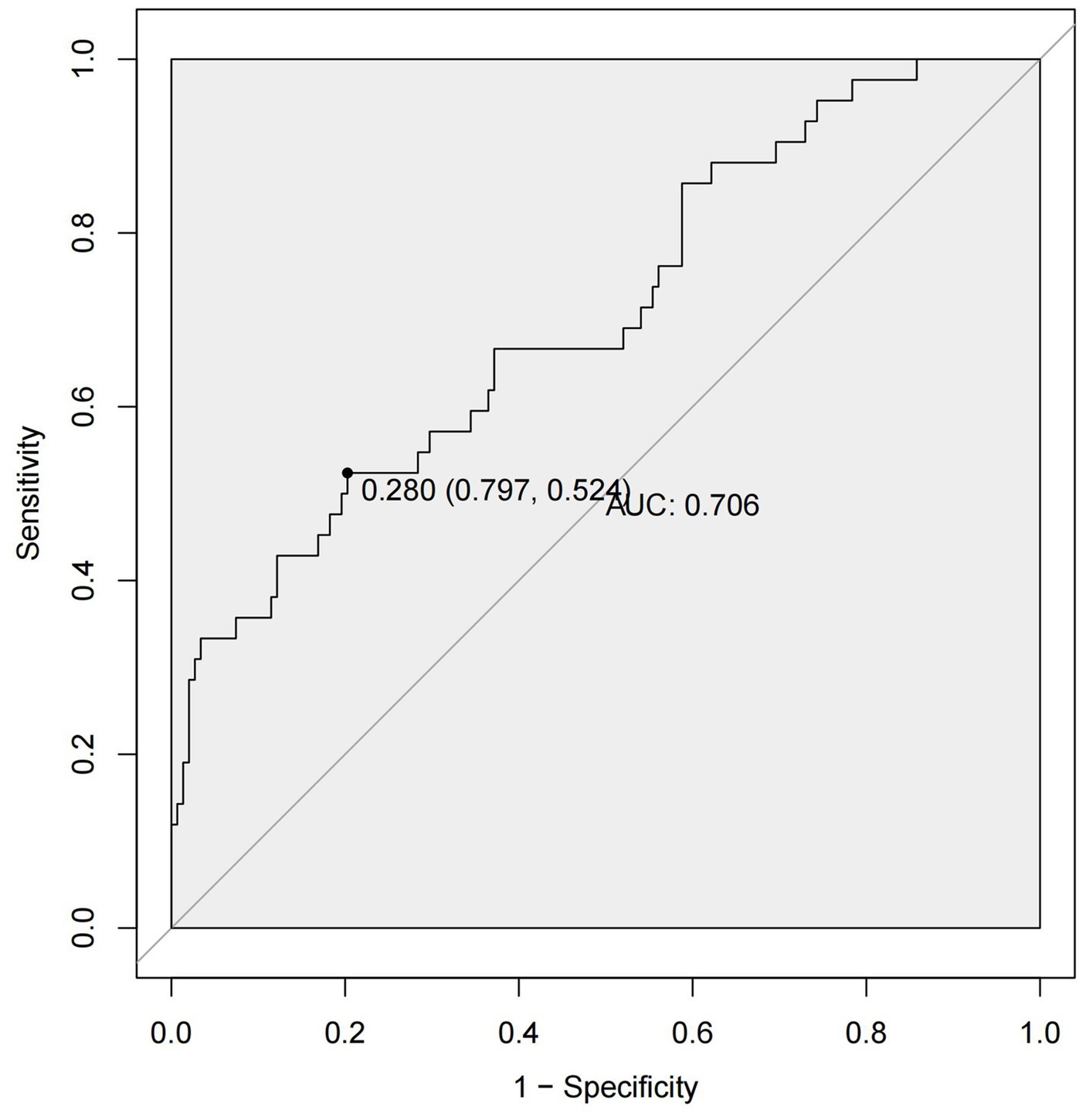
Figure 3. ROC curves of the nomogram. ROC, receiver operating characteristic; AUC, area under the curve.
Discussion
A total of 190 patients undergoing CRC surgery were included in this study, including 42 patients in the complication group and 148 patients in the no complication group. Multivariate logistic regression analysis revealed that preoperative albumin, surgical time, waistline and PA were independent risk factors for postoperative complications of CRC. Using these four independent risk factors, we constructed a nomogram model to predict the risk of postoperative complications in CRC patients.
The results of this study suggested that lower preoperative albumin, longer surgical time, larger waistline and smaller PA were independent risk factors for postoperative complications in CRC. Several previous studies have shown that low preoperative albumin levels were a risk factor for postoperative complications and survival in several types of cancer, including CRC (44–47). This might be because low preoperative albumin was a marker of malnutrition, cancer cachexia and chronic inflammatory activity, among other factors (48, 49).
The results of the multivariate logistic regression analysis showed that the duration of surgery was an independent risk factor for the development of postoperative complications in CRC, which was consistent with previous literature (50, 51). Prolonged surgical time not only increased tissue contraction, causing tissue ischaemia, desiccation and necrosis, but also led to coagulation, hemostasis and endothelial damage, thereby increasing the risk of venous thromboembolism (52, 53). In addition, the effectiveness of antimicrobial prophylaxis decreased over time and the likelihood of aseptic technique violations increases (54). Longer surgical time also meant longer anesthesia time and increased fatigue for the surgical team (55).
Waistline was an indicator of central obesity and was often considered a more useful perioperative risk assessment tool than BMI in CRC surgery (56, 57). Increased waistline affected the incidence of postoperative complications in CRC, which might be due to prolonged surgical time, relative wound hypoxia due to reduced subcutaneous tissue oxygenation, impaired collagen synthesis and immune system function (58, 59). Moreover, abdominal obesity was often associated with high levels of inflammatory mediators such as interleukin-6, c-reactive protein and tumor necrosis factor-α, which often led to insulin resistance and postoperative complications (60, 61).
PA was a BIA correlated that was directly related to the quality and integrity of cell membranes, and was often considered a marker of cellular health (62, 63). PA was closely related to the level of inflammation in the body, and inflammation and low albumin were associated with altered fluid distribution in the extracellular space, which could reduce the PA (64, 65). In addition, PA was a marker of oxidative stress, whereby an imbalance between oxidants and antioxidants produced oxidative stress, leading to the destruction of cellular components such as proteins or lipids, which could lead to cellular damage and rupture of cell membranes (65–67).
However, this study still has some limitations. First, this was a single-center retrospective study. Secondly, the nomogram prediction model developed in this study was not internally validated, and we will continue to collect clinical information and nutritional indicators from the patients concerned to improve internal validation. The application of deep learning algorithms has the potential to improve the accuracy and effectiveness of CRC detection (68, 69), however, due to the small sample size of this study, the application of deep learning algorithms was not carried out and it is expected that in the future appropriate validation can be carried out when large data sets are available.
In conclusion, this study created a nomogram to predict the risk of postoperative complications in CRC patients, providing surgeons with a reliable reference to personalized patient management in the perioperative period and preoperative nutritional interventions.
Data availability statement
The raw data supporting the conclusions of this article will be made available by the authors, without undue reservation.
Ethics statement
The studies involving humans were approved by this study was reviewed by the Ethics Committee of the First Affiliated Hospital of Chongqing Medical University (2022-135-2), and all patients in this study signed an informed consent form. The studies were conducted in accordance with the local legislation and institutional requirements. Written informed consent for participation was not required from the participants or the participants' legal guardians/next of kin because the present study was performed according to the World Medical Association Declaration of Helsinki. Ethical approval from the institutional review board (K2024-002-01) was obtained. In addition, we applied for a waiver of informed consent.
Author contributions
X-YL: Conceptualization, Data curation, Methodology, Writing – original draft. QL: Data curation, Investigation, Software, Writing – original draft, Project administration. BK: Conceptualization, Investigation, Project administration, Software, Writing – original draft. Z-WW: Formal analysis, Software, Validation, Writing – review & editing, Methodology.
Funding
The author(s) declare that no financial support was received for the research, authorship, and/or publication of this article.
Acknowledgments
We acknowledge all the authors whose publications are referred in our article.
Conflict of interest
The authors declare that the research was conducted in the absence of any commercial or financial relationships that could be construed as a potential conflict of interest.
Publisher’s note
All claims expressed in this article are solely those of the authors and do not necessarily represent those of their affiliated organizations, or those of the publisher, the editors and the reviewers. Any product that may be evaluated in this article, or claim that may be made by its manufacturer, is not guaranteed or endorsed by the publisher.
References
1. Xia, C, Dong, X, Li, H, Cao, M, Sun, D, He, S, et al. Cancer statistics in China and United States, 2022: profiles, trends, and determinants. Chin Med J. (2022) 135:584–90. doi: 10.1097/CM9.0000000000002108
2. Miller, KD, Nogueira, L, Mariotto, AB, Rowland, JH, Yabroff, KR, Alfano, CM, et al. Cancer treatment and survivorship statistics, 2019. CA Cancer J Clin. (2019) 69:363–85. doi: 10.3322/caac.21565
3. Liu, XY, Zhang, B, Cheng, YX, Tao, W, Yuan, C, Wei, ZQ, et al. Does chronic kidney disease affect the complications and prognosis of patients after primary colorectal cancer surgery? World J Gastrointest Oncol. (2022) 14:1199–209. doi: 10.4251/wjgo.v14.i6.1199
4. Siegel, RL, Wagle, NS, Cercek, A, Smith, RA, and Jemal, A. Colorectal cancer statistics, 2023. CA Cancer J Clin. (2023) 73:233–54. doi: 10.3322/caac.21772
5. Engholm, G, Storm, HH, Ferlay, J, Christensen, N, Johannesen, TB, Klint, Å, et al. NORDCAN: Cancer incidence, mortality, prevalence and survival in the Nordic countries, version 8.2 (26.03.2019). (2019).
6. Sung, H, Ferlay, J, Siegel, RL, Laversanne, M, Soerjomataram, I, Jemal, A, et al. Global Cancer statistics 2020: GLOBOCAN estimates of incidence and mortality worldwide for 36 cancers in 185 countries. CA Cancer J Clin. (2021) 71:209–49. doi: 10.3322/caac.21660
7. Binefa, G, Rodríguez-Moranta, F, Teule, A, and Medina-Hayas, M. Colorectal cancer: from prevention to personalized medicine. World J Gastroenterol. (2014) 20:6786–808. doi: 10.3748/wjg.v20.i22.6786
8. Liu, XY, Li, ZW, Zhang, B, Liu, F, Zhang, W, and Peng, D. Effects of preoperative bicarbonate and lactate levels on short-term outcomes and prognosis in elderly patients with colorectal cancer. BMC Surg. (2023) 23:127. doi: 10.1186/s12893-023-02039-x
9. Kuipers, EJ, Grady, WM, Lieberman, D, Seufferlein, T, Sung, JJ, Boelens, PG, et al. Colorectal cancer. Nat Rev Dis Primers. (2015) 1:15065. doi: 10.1038/nrdp.2015.65
10. Chern, YJ, You, JF, Cheng, CC, Jhuang, JR, Yeh, CY, Hsieh, PS, et al. Decreasing postoperative pulmonary complication following laparoscopic surgery in elderly individuals with colorectal Cancer: a competing risk analysis in a propensity score-weighted cohort study. Cancers (Basel). (2021) 14:131. doi: 10.3390/cancers14010131
11. Dekker, E, Tanis, PJ, Vleugels, JLA, Kasi, PM, and Wallace, MB. Colorectal cancer. Lancet. (2019) 394:1467–80. doi: 10.1016/S0140-6736(19)32319-0
12. Liu, XY, Li, ZW, Kang, B, Cheng, YX, Tao, W, Zhang, B, et al. Does preoperative waiting time affect the short-term outcomes and prognosis of colorectal Cancer patients? A retrospective study from the west of China. Can J Gastroenterol Hepatol. (2022) 2022:8235736. doi: 10.1155/2022/8235736
13. Nowakowski, M, Pisarska, M, Rubinkiewicz, M, Torbicz, G, Gajewska, N, Mizera, M, et al. Postoperative complications are associated with worse survival after laparoscopic surgery for non-metastatic colorectal cancer - interim analysis of 3-year overall survival. Wideochir Inne Tech Maloinwazyjne. (2018) 13:326–32. doi: 10.5114/wiitm.2018.76179
14. Liu, XY, Zhang, B, Kang, B, Cheng, YX, Yuan, C, Tao, W, et al. The effect of complications on oncological outcomes of colorectal Cancer patients after primary surgery: a propensity score matching analysis. Front Oncol. (2022) 12:857062. doi: 10.3389/fonc.2022.857062
15. Vandewoude, M. Nutritional assessment in geriatric cancer patients. Support Care Cancer. (2010) 18:S51–6. doi: 10.1007/s00520-009-0755-7
16. Dewys, WD, Begg, C, Lavin, PT, Band, PR, Bennett, JM, Bertino, JR, et al. Prognostic effect of weight loss prior to chemotherapy in cancer patients. Eastern cooperative oncology group. Am J Med. (1980) 69:491–7. doi: 10.1016/s0149-2918(05)80001-3
17. Cavagnari, MAV, Silva, TD, Pereira, MAH, Sauer, LJ, Shigueoka, D, Saad, SS, et al. Impact of genetic mutations and nutritional status on the survival of patients with colorectal cancer. BMC Cancer. (2019) 19:644. doi: 10.1186/s12885-019-5837-4
18. Barbosa-Silva, MC, and Barros, AJ. Bioelectric impedance and individual characteristics as prognostic factors for post-operative complications. Clin Nutr. (2005) 24:830–8. doi: 10.1016/j.clnu.2005.05.005
19. Gupta, D, Lammersfeld, CA, Burrows, JL, Dahlk, SL, Vashi, PG, Grutsch, JF, et al. Bioelectrical impedance phase angle in clinical practice: implications for prognosis in advanced colorectal cancer. Am J Clin Nutr. (2004) 80:1634–8. doi: 10.1093/ajcn/80.6.1634
20. Bauer, J, Capra, S, and Ferguson, M. Use of the scored patient-generated subjective global assessment (PG-SGA) as a nutrition assessment tool in patients with cancer. Eur J Clin Nutr. (2002) 56:779–85. doi: 10.1038/sj.ejcn.1601412
21. Barao, K, Abe Vicente Cavagnari, M, Silva Fucuta, P, and Manoukian Forones, N. Association between nutrition status and survival in elderly patients with colorectal Cancer. Nutr Clin Pract. (2017) 32:658–63. doi: 10.1177/0884533617706894
22. Ottery, FD. Definition of standardized nutritional assessment and interventional pathways in oncology. Nutrition. (1996) 12:S15–9. doi: 10.1016/0899-9007(96)90011-8
23. Mota, AP, Aredes, MA, De Oliveira Miguel, J, and Chaves, GV. Nutritional status assessed by Patient-Generated Subjective Global Assessment is associated with toxicity to chemoradiotherapy in women with cervical cancer: a prospective study. Eur J Clin Nutr. (2022) 76:1740–7. doi: 10.1038/s41430-022-01180-9
24. Ge, T, Lin, T, Yang, J, and Wang, M. Nutritional status and related factors of patients with advanced lung cancer in northern China: a retrospective study. Cancer Manag Res. (2019) 11:2225–31. doi: 10.2147/CMAR.S193567
25. Kim, HS, Lee, JY, Lim, SH, Cho, J, Kim, SJ, Jang, JH, et al. Patient-generated subjective global assessment as a prognosis tool in patients with multiple myeloma. Nutrition. (2017) 36:67–71. doi: 10.1016/j.nut.2016.06.009
26. Jager-Wittenaar, H, and Ottery, FD. Assessing nutritional status in cancer: role of the patient-generated subjective global assessment. Curr Opin Clin Nutr Metab Care. (2017) 20:322–9. doi: 10.1097/MCO.0000000000000389
27. Kyle, UG, Kossovsky, MP, Karsegard, VL, and Pichard, C. Comparison of tools for nutritional assessment and screening at hospital admission: a population study. Clin Nutr. (2006) 25:409–17. doi: 10.1016/j.clnu.2005.11.001
28. Velasco, C, García, E, Rodríguez, V, Frias, L, Garriga, R, Álvarez, J, et al. Comparison of four nutritional screening tools to detect nutritional risk in hospitalized patients: a multicentre study. Eur J Clin Nutr. (2011) 65:269–74. doi: 10.1038/ejcn.2010.243
29. Bakkaloglu, OK, Bektas, M, Ince, B, Amikishiyev, S, Tor, YB, Altınkaynak, M, et al. Malnutrition risk in hospitalized patients measured with nutrition risk screening 2002 tool and its association with in-hospital mortality. Eur Rev Med Pharmacol Sci. (2023) 27:5812–21. doi: 10.26355/eurrev_202306_32820
30. Weiser, MR. AJCC 8th edition: colorectal Cancer. Ann Surg Oncol. (2018) 25:1454–5. doi: 10.1245/s10434-018-6462-1
31. Paiva, SI, Borges, LR, Halpern-Silveira, D, Assunção, MCF, Barros, AJD, and Gonzalez, MC. Standardized phase angle from bioelectrical impedance analysis as prognostic factor for survival in patients with cancer. Support Care Cancer. (2010) 19:187–92. doi: 10.1007/s00520-009-0798-9
32. Gupta, D, Lis, CG, Dahlk, SL, King, J, Vashi, PG, Grutsch, JF, et al. The relationship between bioelectrical impedance phase angle and subjective global assessment in advanced colorectal cancer. Nutr J. (2008) 7:19. doi: 10.1186/1475-2891-7-19
33. Kroc, Ł, Fife, E, Piechocka-Wochniak, E, Sołtysik, B, and Kostka, T. Comparison of nutrition risk screening 2002 and subjective global assessment form as short nutrition assessment tools in older hospitalized adults. Nutrients. (2021) 13:225. doi: 10.3390/nu13010225
34. Barbosa-Silva, MC, Barros, AJ, Wang, J, Heymsfield, SB, and Pierson, RN. Bioelectrical impedance analysis: population reference values for phase angle by age and sex. Am J Clin Nutr. (2005) 82:49–52. doi: 10.1093/ajcn.82.1.49
35. Kyle, UG, Bosaeus, I, de Lorenzo, AD, Deurenberg, P, Elia, M, Gómez, JM, et al. Bioelectrical impedance analysis--part I: review of principles and methods. Clin Nutr. (2004) 23:1226–43. doi: 10.1016/j.clnu.2004.06.004
36. Wang, W, Meng, X, Liu, J, Lou, X, Zhang, P, He, P, et al. Study on the correlation between bioelectrical impedance analysis index and protein energy consumption in maintenance dialysis patients. Nutr J. (2023) 22:56. doi: 10.1186/s12937-023-00890-5
37. Ruperto, M, and Barril, G. Extracellular mass to body cell mass ratio as a potential index of wasting and fluid overload in hemodialysis patients. Clin Nutr. (2020) 39:316–7. doi: 10.1016/j.clnu.2019.11.019
38. Rymarz, A, Bartoszewicz, Z, Szamotulska, K, and Niemczyk, S. The associations between body cell mass and nutritional and inflammatory markers in patients with chronic kidney disease and in subjects without kidney disease. J Ren Nutr. (2016) 26:87–92. doi: 10.1053/j.jrn.2015.09.005
39. Liu, Q, Zhou, Q, Song, M, Zhao, F, Yang, J, Feng, X, et al. A nomogram for predicting the risk of sepsis in patients with acute cholangitis. J Int Med Res. (2020) 48:66100. doi: 10.1177/0300060519866100
40. Yu, P, Kan, R, Meng, X, Wang, Z, Xiang, Y, Mao, B, et al. A nomogram for predicting the risk of CKD based on Cardiometabolic risk factors. Int J Gen Med. (2023) 16:4143–54. doi: 10.2147/IJGM.S425122
41. Lin, Z, Li, Y, Wu, J, Zheng, H, and Yang, C. Nomogram for prediction of prolonged postoperative ileus after colorectal resection. BMC Cancer. (2022) 22:1273. doi: 10.1186/s12885-022-10377-x
42. Shi, B, Shen, L, Huang, W, Cai, L, Yang, S, Zhang, Y, et al. A nomogram for predicting surgical timing in neonates with necrotizing Enterocolitis. J Clin Med. (2023) 12:3062. doi: 10.3390/jcm12093062
43. Huai, J, Ye, X, and Ding, J. Nomogram for the prediction of delayed colorectal post-polypectomy bleeding. Turk J Gastroenterol. (2021) 32:727–34. doi: 10.5152/tjg.2021.20842
44. Uppal, S, al-Niaimi, A, Rice, LW, Rose, SL, Kushner, DM, Spencer, RJ, et al. Preoperative hypoalbuminemia is an independent predictor of poor perioperative outcomes in women undergoing open surgery for gynecologic malignancies. Gynecol Oncol. (2013) 131:416–22. doi: 10.1016/j.ygyno.2013.08.011
45. Lee, KG, Lee, HJ, Yang, JY, Oh, SY, Bard, S, Suh, YS, et al. Risk factors associated with complication following gastrectomy for gastric cancer: retrospective analysis of prospectively collected data based on the Clavien-Dindo system. J Gastrointest Surg. (2014) 18:1269–77. doi: 10.1007/s11605-014-2525-1
46. Kudsk, KA, Tolley, EA, DeWitt, RC, Janu, PG, Blackwell, AP, Yeary, S, et al. Preoperative albumin and surgical site identify surgical risk for major postoperative complications. JPEN J Parenter Enteral Nutr. (2003) 27:1–9. doi: 10.1177/014860710302700101
47. Lai, CC, You, JF, Yeh, CY, Chen, JS, Tang, R, Wang, JY, et al. Low preoperative serum albumin in colon cancer: a risk factor for poor outcome. Int J Color Dis. (2011) 26:473–81. doi: 10.1007/s00384-010-1113-4
48. Braga, M, Ljungqvist, O, Soeters, P, Fearon, K, Weimann, A, Bozzetti, F, et al. ESPEN guidelines on parenteral nutrition: surgery. Clin Nutr. (2009) 28:378–86. doi: 10.1016/j.clnu.2009.04.002
49. Ataseven, B, du Bois, A, Reinthaller, A, Traut, A, Heitz, F, Aust, S, et al. Pre-operative serum albumin is associated with post-operative complication rate and overall survival in patients with epithelial ovarian cancer undergoing cytoreductive surgery. Gynecol Oncol. (2015) 138:560–5. doi: 10.1016/j.ygyno.2015.07.005
50. Cheng, H, Clymer, JW, Po-Han Chen, B, Sadeghirad, B, Ferko, NC, Cameron, CG, et al. Prolonged operative duration is associated with complications: a systematic review and meta-analysis. J Surg Res. (2018) 229:134–44. doi: 10.1016/j.jss.2018.03.022
51. Haddock, NT, Wen, YE, Steppe, C, Shang, Z, and Teotia, SS. Operative time predicts postoperative outcomes in bilateral DIEP flap reconstruction: multivariate 1000 flap analysis. Plast Reconstr Surg Glob Open. (2022) 10:e4713. doi: 10.1097/GOX.0000000000004713
52. Bekelis, K, Labropoulos, N, and Coy, S. Risk of venous thromboembolism and operative duration in patients undergoing neurosurgical procedures. Neurosurgery. (2017) 80:787–92. doi: 10.1093/neuros/nyw129
53. Kim, JY, Khavanin, N, Rambachan, A, McCarthy, RJ, Mlodinow, AS, de Oliveria, GS, et al. Surgical duration and risk of venous thromboembolism. JAMA Surg. (2015) 150:110–7. doi: 10.1001/jamasurg.2014.1841
54. Korol, E, Johnston, K, Waser, N, Sifakis, F, Jafri, HS, Lo, M, et al. A systematic review of risk factors associated with surgical site infections among surgical patients. PLoS One. (2013) 8:e83743. doi: 10.1371/journal.pone.0083743
55. Shtarbanov, P, Ioannidi, L, Hamilton, S, Ghali, S, Mosahebi, A, Ahmed, Z, et al. Prolonged operative time is a risk factor for adverse postoperative outcomes in the unilateral deep inferior epigastric perforator (DIEP) flap surgery: a retrospective cohort study. J Plast Reconstr Aesthet Surg. (2023) 87:180–6. doi: 10.1016/j.bjps.2023.07.048
56. Kartheuser, AH, Leonard, DF, Penninckx, F, Paterson, HM, Brandt, D, Remue, C, et al. Waist circumference and waist/hip ratio are better predictive risk factors for mortality and morbidity after colorectal surgery than body mass index and body surface area. Ann Surg. (2013) 258:722–30. doi: 10.1097/SLA.0b013e3182a6605a
57. Balentine, CJ, Robinson, CN, Marshall, CR, Wilks, J, Buitrago, W, Haderxhanaj, K, et al. Waist circumference predicts increased complications in rectal cancer surgery. J Gastrointest Surg. (2010) 14:1669–79. doi: 10.1007/s11605-010-1343-3
58. Pierpont, YN, Dinh, TP, Salas, RE, Johnson, EL, Wright, TG, Robson, MC, et al. Obesity and surgical wound healing: a current review. ISRN Obes. (2014) 2014:638936. doi: 10.1155/2014/638936
59. Kurmann, A, Vorburger, SA, Candinas, D, and Beldi, G. Operation time and body mass index are significant risk factors for surgical site infection in laparoscopic sigmoid resection: a multicenter study. Surg Endosc. (2011) 25:3531–4. doi: 10.1007/s00464-011-1753-7
60. Weisberg, SP, McCann, D, Desai, M, Rosenbaum, M, Leibel, RL, Ferrante, AW, et al. Obesity is associated with macrophage accumulation in adipose tissue. J Clin Invest. (2003) 112:1796–808. doi: 10.1172/JCI19246
61. Gurunathan, U, Rapchuk, IL, Dickfos, M, Larsen, P, Forbes, A, Martin, C, et al. Association of Obesity with Septic Complications after Major Abdominal Surgery: a secondary analysis of the RELIEF randomized clinical trial. JAMA Netw Open. (2019) 2:e1916345. doi: 10.1001/jamanetworkopen.2019.16345
62. Dittmar, M. Reliability and variability of bioimpedance measures in normal adults: effects of age, gender, and body mass. Am J Phys Anthropol. (2003) 122:361–70. doi: 10.1002/ajpa.10301
63. Cimmino, F, Petrella, L, Cavaliere, G, Ambrosio, K, Trinchese, G, Monda, V, et al. A bioelectrical impedance analysis in adult subjects: the relationship between phase angle and body cell mass. J Funct Morphol Kinesiol. (2023) 8:107. doi: 10.3390/jfmk8030107
64. Lukaski, HC, Kyle, UG, and Kondrup, J. Assessment of adult malnutrition and prognosis with bioelectrical impedance analysis: phase angle and impedance ratio. Curr Opin Clin Nutr Metab Care. (2017) 20:330–9. doi: 10.1097/MCO.0000000000000387
65. Lee, GR, and Kim, EY. Usefulness of phase angle on bioelectrical impedance analysis as a surveillance tool for postoperative infection in critically ill patients. Front Med (Lausanne). (2023) 10:1111727. doi: 10.3389/fmed.2023.1111727
66. Da Silva, BR, Gonzalez, MC, Cereda, E, and Prado, CM. Exploring the potential role of phase angle as a marker of oxidative stress: a narrative review. Nutrition. (2022) 93:111493. doi: 10.1016/j.nut.2021.111493
67. Rosas-Carrasco, O, Núñez-Fritsche, G, López-Teros, MT, Acosta-Méndez, P, Cruz-Oñate, JC, Navarrete-Cendejas, AY, et al. Low muscle strength and low phase angle predicts greater risk to mortality than severity scales (APACHE, SOFA, and CURB-65) in adults hospitalized for SARS-CoV-2 pneumonia. Front Nutr. (2022) 9:965356. doi: 10.3389/fnut.2022.965356
68. Bousis, D, Verras, GI, Bouchagier, K, Antzoulas, A, Panagiotopoulos, I, Katinioti, A, et al. The role of deep learning in diagnosing colorectal cancer. Prz Gastroenterol. (2023) 18:266–73. doi: 10.5114/pg.2023.129494
69. Chlorogiannis, DD, Verras, G, Tzelepi, V, Chlorogiannis, A, Filippiadis, DK, Kelekis, A, et al. Tissue classification and diagnosis of colorectal cancer histopathology images using deep learning algorithms. Is the time ripe for clinical practice implementation? Prz Gastroenterol. (2023) 18:353–67. doi: 10.5114/pg.2023.130337
Keywords: colorectal cancer, complications, surgery, phase angle, nomogram
Citation: Liu X-Y, Kang B, Lv Q and Wang Z-W (2024) Phase angle is a predictor for postoperative complications in colorectal cancer. Front. Nutr. 11:1446660. doi: 10.3389/fnut.2024.1446660
Edited by:
Francesk Mulita, General University Hospital of Patras, GreeceReviewed by:
Nallely Bueno-Hernández, General Hospital of Mexico, MexicoAngelis Peteinaris, University of Patras, Greece
Andreas Antzoulas, General University Hospital of Patras, Greece
Copyright © 2024 Liu, Kang, Lv and Wang. This is an open-access article distributed under the terms of the Creative Commons Attribution License (CC BY). The use, distribution or reproduction in other forums is permitted, provided the original author(s) and the copyright owner(s) are credited and that the original publication in this journal is cited, in accordance with accepted academic practice. No use, distribution or reproduction is permitted which does not comply with these terms.
*Correspondence: Zi-Wei Wang, wangziwe571@sina.com
†These authors have contributed equally to this work