- 1Department of Urology, The Eighth Affiliated Hospital, Sun Yat-sen University, Shenzhen, Guangdong, China
- 2School of Medicine, Sun Yat-sen University, Shenzhen, China
Background: Kidney stone disease (KSD) is a widespread problem in urology. But the associations between the Geriatric Nutritional Risk Index (GNRI), an important indicator for assessing the nutritional status of elderly hospitalized patients, and KSD are understudied.
Objective: Utilizing data from the National Health and Nutrition Examination Survey (NHANES) spanning 2007–2018, our study analyzed the correlation between the GNRI and KSD prevalence at cross-sectional level. The aim of the study was to explore the association between GNRI and the prevalence of KSD to identify potential risk factors and inform prevention and management strategies for KSD.
Methods: This cross-sectional study analyzed data focusing on 26,803 adults (20–80 years) after screening for complete data. It evaluated GNRI, a formula involving albumin, present, and ideal body weight, stratifying participants into quartiles. The primary outcome was the history of KSD, based on self-reports. Covariates included demographic, health, and lifestyle factors. Statistical analysis employed t-tests, ANOVA, Wilcoxon, and Kruskal-Wallis tests, with logistic regression modeling GNRI’s impact on KSD prevalence, assessing odds ratios and potential multicollinearity, and sensitivity analyses excluding individuals with low eGFR and adjusting cycle years.
Results: Significant disparities are found in GNRI distributions between individuals with and without kidney stones. Higher GNRI levels are more common in kidney stone patients, with 39.249% in the highest quartile versus 33.334% in those without stones. Notably, those in the highest GNRI quartile (Q4) show a lower prevalence of kidney stone disease (KSD) than those in the lowest (Q1), with rates of 11.988% versus 8.631%, respectively (P < 0.0001). Adjusted model results reveal that higher GNRI quartiles (Q3-Q4) correlate with reduced KSD prevalence, with odds ratios of 0.85 (95% CI [0.72, 1.00]) and 0.76 (95% CI [0.65, 0.89]). A nonlinear inverse relationship exists between GNRI levels and KSD prevalence across the population (P < 0.001), confirming that higher GNRI lowers KSD prevalence. Subgroup and sensitivity analyses support these findings.
Conclusion: The study underscores a significant, albeit nonlinear, association between elevated GNRI levels and decreased KSD prevalence. This relationship highlights the importance of nutritional assessment and management in KSD prevention strategies.
1 Introduction
Kidney Stone Disease (KSD) represents a prevalent issue within urology, affecting approximately 11% of the population and boasting an prevalence rate of 14.8% (1, 2). This condition frequently results in renal colic, urinary tract infections, and kidney damage. Advances in diagnostic and surgical techniques, including Percutaneous Nephrolithotomy (PNL), Retrograde Intrarenal Surgery (RIRS), and Extracorporeal Shock Wave Lithotripsy (ESWL), have significantly enhanced the management of KSD. However, despite these advancements, the post-surgery recurrence rate of KSD remains alarmingly high, with up to 50% of patients experiencing recurrence within 5 years (2). This high recurrence rate underscores the necessity of investigating KSD formation’s risk factors and devising strategies to mitigate the risk of new or recurring instances, which is crucial for alleviating the considerable health and economic impacts associated with this condition.
Since its inception by Bouillanne et al. in 2005, the Geriatric Nutritional Risk Index (GNRI) has become a pivotal instrument for evaluating the nutritional status of hospitalized elderly patients, proving effective in predicting morbidity and mortality risks (3). Its simplicity and efficacy have broadened its application across various medical fields, including digestive system diseases, orthopedics, and urology (4–6). Grinstead et al. emphasize the importance of GNRI in monitoring the nutritional status to enhance cancer patients’ survival rates (7). It is documented that GNRI is a prognostic tool, which can predict severe complications and mortality of elderly patients undergoing colorectal cancer surgery (8). Further, Haas et al. have proposed GNRI as a potential predictor of immunotherapy response in patients with squamous cell carcinoma of the head and neck (9). Additionally, epidemiological studies identify GNRI as an independent risk factor for older people with osteoporosis, linking it to the condition’s severity (10). In the context of urologic diseases, Nakagawa et al. highlighted GNRI’s association with all-cause and cardiovascular mortality (11). Miao et al. found an inverse correlation between GNRI and prostate cancer risk, suggesting a nonlinear relationship (12). Shu et al. demonstrated GNRI’s predictive value for postoperative outcomes in prostate cancer surgery (13). Despite the known correlation between nutritional factors and KSD formation (14), the specific relationship between GNRI and KSD prevalence warrants further investigation.
The National Health and Nutrition Examination Survey (NHANES) is a comprehensive, cross-sectional survey that collects health and nutrition data from households across the United States. Through stratified multistage sampling, NHANES ensures its sample is representative of the U.S. population, covering demographic, socioeconomic, dietary, and health data obtained through household interviews and health examinations. Utilizing this rich database, our study conducted a retrospective analysis to explore the association between the Geriatric Nutritional Risk Index (GNRI) and the prevalence of kidney stone disease (KSD). This investigation aims to deepen our understanding of the risk factors associated with KSD, offering new insights for its prevention and management.
2 Materials and methods
2.1 Data source and study population
This cross-sectional study utilized data from the National Health and Nutrition Examination Survey (NHANES), a resource managed by the National Center for Health Statistics at the Centers for Disease Control and Prevention. The NHANES website’s publicly available data plays a crucial role in epidemiological research and in assessing nutritional health. Our research focused on the 2007–2018 NHANES dataset, encompassing participants aged 20–80 years and providing comprehensive, reliable information across various domains consisting of demographics, dietary and health-related behaviors, physical measurements, and disease information. The initial participant pool of 59,842 was refined to 25,474 eligible subjects for this study. The refinement process included screening for completeness in dataset outcomes and exposures (n = 26,592), and relevant covariates (n = 7776). These covariates encompassed annual household income (1896), marital status (14), education level (29), eGFR (1856), BMI (364), smoking status (13), alcohol use (3220), sitting time (113), cardiovascular disease (1), hypertension (1), diabetes mellitus (269), and moderate recreational activity (0), as illustrated in Figure 1.
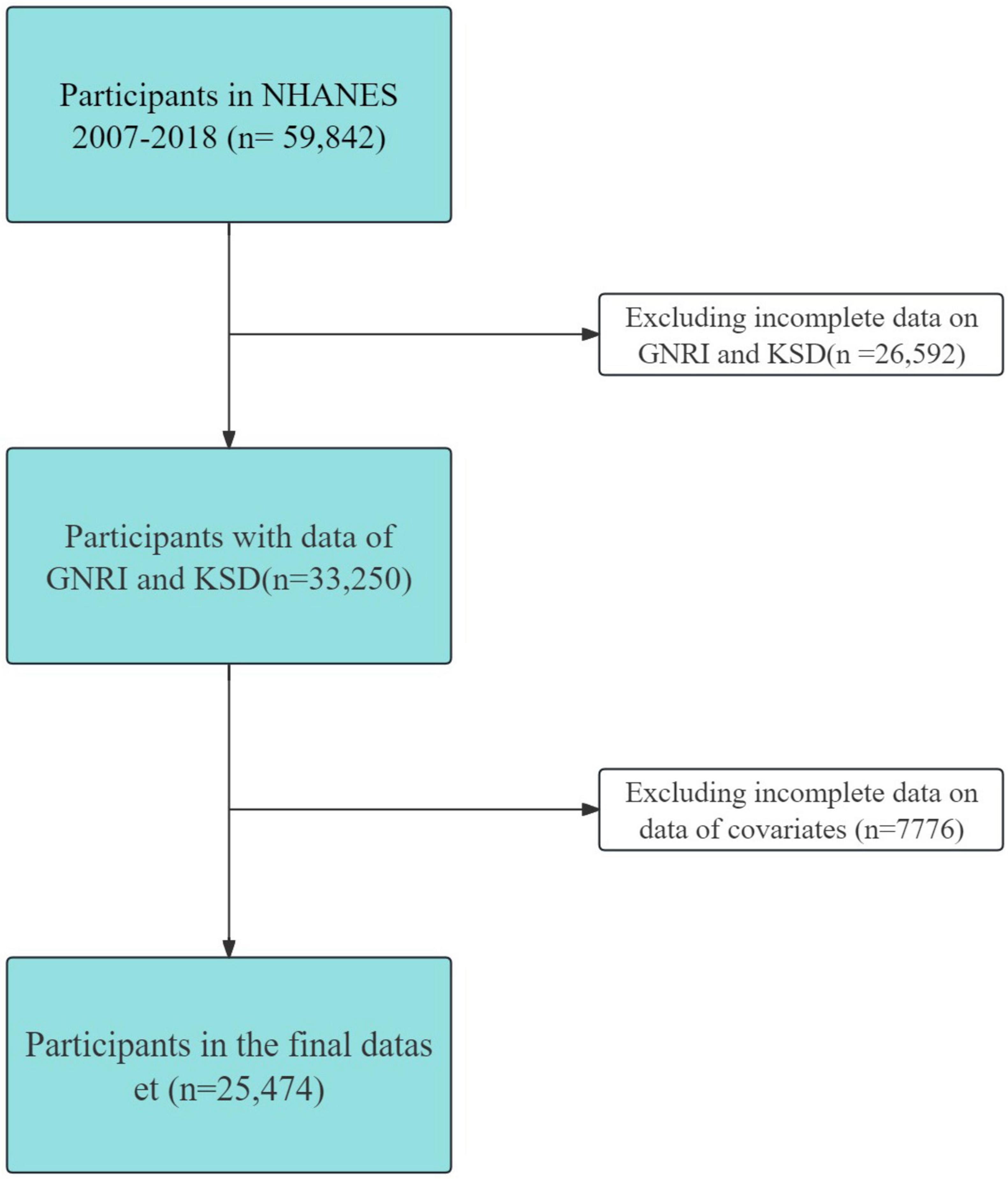
Figure 1. Inclusion and exclusion criteria. NHANES, National Health and Nutrition Examination Survey.
2.2 Assessment of GNRI
The GNRI is recognized as a straightforward yet effective score for nutritional assessment. The GNRI formula incorporates serum albumin levels (expressed in g/L), present body weight (PBW, in kg), and ideal body weight (IBW), where IBW is calculated as the square of height in meters (m2) multiplied by 22. The GNRI is calculated using the following equation: GNRI = 1.489 × albumin ++ 41.7 × (PBW/IBW). In cases where PBW surpasses IBW, the PBW/IBW ratio is set to 1. For our study, participants were stratified into four equal groups based on their GNRI scores: Q1 (61.96–101.26), Q2 (101.26–104.24), Q3 (104.24–107.22), and Q4 (107.22–125.08). This stratification facilitated a comprehensive analysis of nutritional risk across different levels.
2.3 Assessment of KSD
In the NHANES survey, the primary outcome assessed was the presence of a history of KSD disease, dichotomously categorized as “yes” or “no.” This information was garnered through a structured questionnaire survey. Participants affirmatively responding to the query “Have you ever had KSD?” were categorized as having a history of KSD disease. This method of classification was based on self-reported data (15).
2.4 Assessment of covariates
In this study, we meticulously adjusted for a broad range of confounding factors to clarify the relationship between the prevalence of KSD and the GNRI. The adjustment included sociodemographic characteristics, Body Mass Index (BMI, in kg/m2), history of chronic diseases, physical activity levels, cardiovascular diseases, glomerular filtration rate (eGFR), and cycle years. The sociodemographic variables comprised age, gender (male or female), race (White or other), marital status (unmarried or married), annual household income (below $20,000 or above $20,000), and educational level (below high school, high school graduate, or above high school). Chronic diseases considered were cardiovascular diseases, hypertension, and diabetes, with options for response as “no” or “yes”. Smoking and drinking habits were also included, categorized as “more” or “less” for smoking, and “no” or “yes” for drinking. BMI was classified into two categories: less than 25, and 25 or above. Physical activity assessment encompassed moderate recreational activities and sedentary time, with responses of “no” or “yes”. The occurrence of KSD was another covariate, with “no” or “yes” responses. The Chronic Kidney Disease Epidemiology Collaboration (CKD-EPI) equation (19414839) was used to estimate eGFR. Our sensitivity analyses involved considering eGFR as a covariate. The study spanned six biennial cycles from 2007-2008 to 2017-2018, providing a temporal framework for our analysis (16, 17).
2.5 Statistical analysis
This study delineates the presentation and examination of various data types. Continuous variables that show normal or near-normal distribution are described by mean values (standard error, SE), with the application of t-tests for two-group comparisons while one-way ANOVA for scenarios involving three or more groups. In contrast, Continuous variables that are not normally distributed are depicted as median values (interquartile range, IQR) while evaluated using Wilcoxon tests for two groups and Kruskal-Wallis tests for three or more groups. Categorical variables are represented as actual sample numbers (weighted percentages) while Chi-square tests are used to identify significant differences in distribution.
For the investigation of GNRI we categorized these variables into quartiles and implemented a weighted binary logistic regression model to examine its association with the prevalence of KSD. This approach facilitated the presentation of associations as odds ratios (OR) accompanied by 95% confidence intervals (95% CI). To address potential multicollinearity, we utilized variance inflation factors, with values exceeding 10 indicating significant multicollinearity. Dummy variables were introduced for the effective handling of categorical variables.
We also conducted subgroup analyses to evaluate interaction effects, using weighted likelihood ratio tests. The fully adjusted model was used to analyze multiplicative interaction terms for each covariate, except for the stratified covariate. To ensure the robustness of our findings, the study incorporated several sensitivity analyses. These included excluding individuals with an estimated glomerular filtration rate (eGFR) lower than 60 mL/min/1.73m2 and adjusting for cycle years.
R software, version 4.2.1 (R Core Team), was used for all statistical procedures. A 2-tailed P-value threshold of lower than 0.05 was applied to determine statistical significance.
3 Results
This study included 25,474 participants after excluding individuals whose information was incomplete and who had missing data on GNRI, KSD and covariates (Figure 1).
3.1 Characteristics of the study population according to the KSD
In the preliminary analysis (Table 1), significant differences were observed between the groups regarding variables including age, sex, race, marital status, cardiovascular disease, hypertension, diabetes mellitus, smoke, BMI, moderate recreational activity, GNRI.
The prevalence of KSD in the group aged over 65 was significantly above the prevalence in the group aged under 65. Also, the KSD group had a wider proportion of males and white people than the without KSD group.
With regard to social factors, the KSD group exhibited a larger percentage of married individuals, but there was no noteworthy discrepancy with respect to annual household income and educational level. Otherwise, the KSD group had a higher proportion of individuals with regards to cardiovascular disease, hypertension, diabetes mellitus, more smoking and moderate recreational activity (P < 0.0001) as well as the higher BMI score and lower eGFR (P < 0.001). Moreover, alcohol user and sitting time were not statistically different (P > 0.05; Table 1). Overall, the KSD group tends to have a higher proportion of low GNRI scores.
3.2 Characteristics of the study population according to GNRI
Table 2 present the cohort characteristics distribution categorized according to GNRI level. In the preliminary analysis, as the GNRI score increases, the proportion of people aged over 65 years decreases. Regarding social factors, the proportion of people who are males, white, annual household income > 20,000, education level > High School, alcohol users as well as having moderate recreational activity increased while the GNRI increased from Q1 to Q4. However, as for the systemic disease factors, as the GNRI score increases, the proportion of people with cardiovascular disease, hypertension, diabetes mellitus went on a decreased trend (P < 0.05). Interestingly, while the GNRI increased, the BMI of participants dropped but the eGFR of participants elevated. Moreover, the prevalence of KSD among the participants decreased from 11.988 to 8.631% while the GNRI increased, (P < 0.0001).
3.3 Associations between PTN and KSD based on the outcome for KSD status
Taking Quartile 1 as a reference for GNRI, a multifactorial logistic regression analysis was conducted to investigate the potential association between high-GNRI and the prevalence of KSD (Table 3). In the relationship equation, height and weight parameters are crucial for calculating Body Mass Index (BMI) and similarly critical in the GNRI formula. Considering this, we have made suitable adjustments to all analysis models to control for potential confounding factors.
Model 1 has no adjustment for variables apart from BMI. Quartile 2 and 3 for GNRI showed no association with the prevalence of KSD. However, quartile 4 (OR = 0.78, 95% CI 0.68,0.89) demonstrated a decreased prevalence of KSD.
In addition, both adjusted Model 2 and 3 revealed that quartile 3 and 4 of GNRI was negatively associated with the prevalence of KSD. After adjusting for age, sex, ethnicity, marital status, annual household income, education, smoked status, alcohol use, recreational activity, sitting time and BMI, cardiovascular disease, hypertension, diabetes mellitus, it was found that in the fully adjusted model 3, [Q3, OR and 95% CI: 0.85(0.72,1.00); Q4, OR and 95% CI: 0.76(0.65,0.89)] was shown to have a more potent relationship that elevated GNRI linked to a lower prevalence of KSD (P < 0.0001).
3.4 Nonlinear association between GNRI and predicted KSD
A substantial non-linear association between the prevalence of KSD and GNRI in the NHANES cohort was confirmed by restricted cubic spline analysis, obtained by analyzing the 3 knots selected with the lowest AIC score (P = 0.001). As GNRI increased over 77.9, the overall prevalence of KSD dropped steadily (P < 0.05) (P < 0.05) (Figure 2).
3.5 Subgroup analysis
The interactive effect between GNRI and KSD was examined in our subgroup analyses using restricted cubic spline models (Supplementary Figures 1–3). The results indicate a nonlinear negative association between GNRI and the prevalence of KSD in subgroups including those younger than 65 years, males, white individuals, married participants, and those with higher economic status, higher education levels, hypertension, alcohol use, moderate recreational activity, and sedentary time ≥5 h (P < 0.05).
Conversely, no nonlinear correlation was observed between GNRI and KSD prevalence in subgroups characterized by lower education levels, absence of cardiovascular disease, hypertension, or diabetes, and varying smoking and drinking habits, as well as lack of moderate recreational activities. However, a negative linear correlation was present in these subgroups (P < 0.05). Notably, in the diabetes subgroup, a positive association with KSD prevalence was detected when GNRI was below 102.7.
3.6 Sensitivity analysis
On the basis of the original Model 3, two sensitivity analyses were conducted to validate the robustness of the results. These analyses consistently indicated that the relationship between KSD prevalence and the GNRI was significant, as detailed in Supplementary Tables 1, 2. The first sensitivity analysis excluded participants with an estimated glomerular filtration rate (eGFR) less than 60 mL/min, with results shown in Supplementary Table 1. The second analysis accounted for annual variations in KSD risk by adjusting for NHANES cycle years, detailed in Supplementary Table 2. Across these sensitivity analyses, the initial association found between GNRI and KSD in this study remained consistent and unaltered.
4 Discussion
Our large-scale sample study based on the NHANES database found that an elevation in the GNRI is associated with an increased prevalence of KSD, revealing a significant nonlinear relationship between the two. Our finding emphasizes the complexity of the interaction between nutritional status and the prevalence of KSD, indicating that lower GNRI values—typically indicative of inferior nutritional status—could, in a paradoxical twist, augment the prevalence of KSD formation under specific circumstances.
Current research underscores the significant correlation between metabolic and nutritional factors and the prevalence of KSD formation. A comprehensive study by Chang et al., involving over 121,000 participants, highlighted that metabolic syndrome components, such as hypertension, obesity, hyperlipidemia, and hyperglycemia, markedly elevate KSD risk (18). Nutritional factors, vital for metabolic processes by providing substrates, have garnered attention in KSD research. Investigations into dietary impacts reveal that potassium and calcium-rich foods, alongside a balanced vegetarian and dairy diet, as suggested by researchers like Chewcharat and Ferraro, could offer protective benefits against KSD (19, 20). Liu et al.’s work on the dietary inflammation index further established a positive link with KSD prevalence (21). Contrarily, antioxidant content in diets appears to have a negligible impact on stone formation rates (22, 23). The intersection of nutrition and metabolism has prompted a focus on physiological indicators, such as obesity-related measures like waist circumference, as potential predictors for KSD (24). Moreover, some studies have examined dietary patterns using 28 food parameters, such as proteins, carbohydrates, and fiber, to assess participants’ dietary inflammatory index. These studies found a positive correlation between higher dietary inflammatory index scores and an increased prevalence of kidney stones, underscoring the significant influence of dietary patterns on kidney stone prevalence (21). Despite these efforts, there remains a scarcity of research exploring the direct link between individual nutritional status and kidney stone risk. To address this gap, our study utilizes the Geriatric Nutritional Risk Index (GNRI), which integrates serum albumin and body weight, to investigate how individual nutritional levels might correlate with kidney stone risk.
As one of the indices included in the Geriatric Nutritional Risk Index (GNRI), the association between body weight and kidney stones has been extensively studied (25). Excessive body weight is widely considered one of the risk factors for the formation of kidney stones. Kemal-Sarica et al. note that being overweight is a substantial risk factor for the formation of kidney stones, and another meta-analysis confirms that being overweight significantly increases the risk of kidney stone recurrence (26, 27). A meta-analysis of observational studies conducted from 2005 to 2022 found that obese individuals had a higher odds ratio (OR) for kidney stones compared to non-obese individuals, at 1.35 (95% CI 1.20–1.52, P < 0.001) (28). Additionally, studies by Lee et al. have established Body Mass Index (BMI) as a significant risk factor for KSD, attributing to obesity, abdominal obesity, and abnormal fat distribution (24). Likewise, research by Zheng and his team involving more than 3000 participants demonstrated that body fat percentage is a dependable predictor of kidney stone disease, underscoring the strong connection between markers of obesity and kidney stone risk (29). However, it should be noted that in calculating the GNRI, if an individual’s actual weight exceeds their ideal weight, the GNRI score does not increase with increasing weight, which may limit its responsiveness to levels of overweight.
Serum albumin levels are a critical component of the Geriatric Nutritional Risk Index (GNRI) scoring, influencing the total score positively. Our research indicates a potential association between higher serum albumin levels and a reduced prevalence of kidney stones, though the direct relationship remains somewhat elusive. Serum albumin, known for its ability to bind calcium, plays a significant role in calcium homeostasis (30). When albumin levels decrease, there is a reduction in calcium ions bound to albumin, leading to an increase in free serum calcium (31). This condition can elevate urinary calcium excretion in the kidneys, thereby promoting stone formation (32). During the acute inflammatory phase, serum albumin levels are inversely correlated with systemic inflammation, which is supported by findings that hypoalbuminemia is associated with elevated inflammatory markers (33, 34). These markers are positively correlated with the prevalence of kidney stones, suggesting an indirect link between low albumin levels and stone formation (35). Additionally, albumin serves as a vital non-enzymatic antioxidant with free radical scavenging properties, playing a central role in maintaining plasma redox status and influencing oxidative stress levels, which are factors in kidney stone formation (36, 37). As the most abundant protein in human plasma, albumin’s roles extend beyond metabolic functions to include acting as a carrier for hormones, sterols, fatty acids, and drugs, and it is involved in antioxidant activity, immune regulation, and the inflammatory response (38). High serum albumin levels generally reflect good nutritional status and may correlate with higher socioeconomic status, where better access to healthcare and proactive disease prevention could contribute to lower prevalence of kidney stone disease.
This study utilizes a large and representative dataset from the National Health and Nutrition Examination Survey (NHANES), ensuring a broad and diverse sample that enhances the generalizability of the findings. Through an in-depth analysis of the relationship between the Geriatric Nutritional Risk Index (GNRI) and the prevalence of kidney stones, the research provides detailed insights into this association. Advanced statistical methods, specifically complex weighted multivariable logistic regression analysis, allow for robust adjustment of various confounding variables, thereby strengthening the validity of the results. Furthermore, conducting sensitivity analyses in both the general population and specific subgroups increases the reliability and robustness of the findings, ensuring they are applicable across different segments of the population. The study’s significant findings reveal a nonlinear relationship between increased GNRI levels and a reduced prevalence of kidney stones. This contributes valuable knowledge to the field and underscores the potential importance of nutritional risk assessment in the prevention of kidney stones.
Despite the strengths of this study, it is not without limitations. Firstly, while the use of NHANES data ensures a broad representation, it may still carry inherent limitations that could restrict the general applicability of our conclusions. Thus, our findings require external validation in other populations to confirm their robustness. Secondly, the cross-sectional design of the study precludes us from establishing causality. Moreover, despite the thorough design of the questionnaire, the potential for recall bias and variability in participants’ comprehension abilities could introduce further biases into the data. Lastly, the covariates adjusted in the model might not be true confounders, potentially affecting the observed associations. Moreover, the reliance on self-reported KSD could introduce recall bias and misclassification, further influencing the results. Lastly, additional epidemiological studies across diverse conditions and populations, as well as prospective cohort studies, are necessary to provide more comprehensive guidance for the clinical prevention of kidney stones.
In summary, this study’s exploration of the association between GNRI and KSD prevalence highlights the importance of weight management and maintaining serum albumin levels in the prevention of KSD. Using GNRI as a metric to assess individual KSD risk allows healthcare providers to identify those at higher risk due to nutritional or weight-related factors. Future research should focus on clarifying the direct influence of GNRI on KSD risk and investigating the potential of nutritional interventions in reducing this risk.
5 Conclusion
This study emphasizes the association between elevated GNRI levels and a reduced prevalence of KSD, revealing a significant nonlinear relationship between them. Such insights are crucial for formulating targeted prevention strategies for KSD, underscoring the importance of nutritional assessment and management in mitigating this risk.
Data availability statement
Publicly available datasets were analyzed in this study. This data can be found here: https://www.cdc.gov/nchs/nhanes/index.htm.
Author contributions
ZT: Conceptualization, Data curation, Formal analysis, Methodology, Writing – original draft, Writing – review and editing. HZ: Conceptualization, Data curation, Formal analysis, Methodology, Writing – original draft, Writing – review and editing. QZ: Conceptualization, Data curation, Formal analysis, Writing – original draft, Writing – review and editing. YC: Writing – original draft, Writing – review and editing. JZ: Writing – original draft, Writing – review and editing, Software, Validation, Visualization. ZbL: Supervision, Writing – review and editing. ZL: Supervision, Writing – review and editing. CL: Conceptualization, Visualization, Writing – review and editing. FT: Conceptualization, Funding acquisition, Supervision, Writing – review and editing. ZH: Conceptualization, Funding acquisition, Supervision, Writing – review and editing. XS: Conceptualization, Funding acquisition, Supervision, Writing – review and editing.
Funding
The author(s) declare that financial support was received for the research, authorship, and/or publication of this article. This study was supported by the National Key Research and Development Program of China (contract no. 2018YFA0902801), Public Health Research Project in Futian District, Shenzhen (grant no. FTWS2023070), and Shenzhen Futian District Clinical Key Specialty (QZDZK-202414 and ZDXKJF-008).
Acknowledgments
During the preparation of this work the authors used chatGPT (Version 4.0) in order to retouch manuscript. After using this service, the authors reviewed and edited the content as needed and take full responsibility for the content of the publication.
Conflict of interest
The authors declare that the research was conducted in the absence of any commercial or financial relationships that could be construed as a potential conflict of interest.
Publisher’s note
All claims expressed in this article are solely those of the authors and do not necessarily represent those of their affiliated organizations, or those of the publisher, the editors and the reviewers. Any product that may be evaluated in this article, or claim that may be made by its manufacturer, is not guaranteed or endorsed by the publisher.
Supplementary material
The Supplementary Material for this article can be found online at: https://www.frontiersin.org/articles/10.3389/fnut.2024.1430668/full#supplementary-material
Abbreviations
NHANES, National Health and Nutrition Examination Survey; GNRI, Geriatric Nutritional Risk Index; KSD, Kidney Stone Disease; PNL, Percutaneous Nephrolithotomy; RIRS, Retrograde Intrarenal Surgery; ESWL, Extracorporeal Shock Wave Lithotripsy; PBW, present body weight; IBW, ideal body weight; BMI, Body Mass Index; eGFR, glomerular filtration rate; CKD-EPI, The Chronic Kidney Disease Epidemiology Collaboration; OR, odds ratios; 95% CI, 95% confidence intervals; eGFR, estimated glomerular filtration rate; BFP, body fat percentage.
References
1. Hill A, Basourakos S, Lewicki P, Wu X, Arenas-Gallo C, Chuang D, et al. Incidence of kidney stones in the United States: The continuous national health and nutrition examination survey. J Urol. (2022) 207(4):851–6. doi: 10.1097/JU.0000000000002331
2. Khan S, Pearle M, Robertson W, Gambaro G, Canales B, Doizi S, et al. Kidney stones. Nat Rev Dis Primers. (2016) 2(1):16008. doi: 10.1038/nrdp.2016.8
3. Bouillanne O, Morineau G, Dupont C, Coulombel I, Vincent J, Nicolis I, et al. Geriatric nutritional risk index: A new index for evaluating at-risk elderly medical patients. Am J Clin Nutr. (2005) 82(4):777–83. doi: 10.1093/ajcn/82.4.777
4. Tsutsui T, Fujiwara T, Matsumoto Y, Kimura A, Kanahori M, Arisumi S, et al. Geriatric nutritional risk index as the prognostic factor in older patients with fragility hip fractures. Osteoporos Int. (2023) 34(7):1207–21. doi: 10.1007/s00198-023-06753-3
5. Zhang S, Chen N, Huang Z, Yan N, Ma L, Gao X. Geriatric nutritional risk index is associated with the occurrence of acute kidney injury in critically ill patients with acute heart failure. Ren Fail. (2024) 46(1):2349122. doi: 10.1080/0886022X.2024.2349122
6. Hisada H, Tsuji Y, Obata M, Cho R, Nagao S, Miura Y, et al. The impact of sarcopenia on short- and long-term outcomes of endoscopic submucosal dissection for early gastric cancer. J Gastroenterol. (2022) 57(12):952–61. doi: 10.1007/s00535-022-01923-2
7. Grinstead C, George T, Han B, Yoon S. Associations of overall survival with geriatric nutritional risk index in patients with advanced pancreatic cancer. Nutrients. (2022) 14(18):3800. doi: 10.3390/nu14183800
8. Sasaki M, Miyoshi N, Fujino S, Ogino T, Takahashi H, Uemura M, et al. The Geriatric nutritional risk index predicts postoperative complications and prognosis in elderly patients with colorectal cancer after curative surgery. Sci Rep. (2020) 10(1):10744. doi: 10.1038/s41598-020-67285-y
9. Haas M, Lein A, Fuereder T, Brkic F, Schnoell J, Liu D, et al. The Geriatric nutritional risk index (GNRI) as a Prognostic biomarker for immune checkpoint inhibitor response in recurrent and/or metastatic head and neck cancer. Nutrients. (2023) 15(4):880. doi: 10.3390/nu15040880
10. Huang W, Xiao Y, Wang H, Li K. Association of geriatric nutritional risk index with the risk of osteoporosis in the elderly population in the NHANES. Front Endocrinol. (2022) 13:965487. doi: 10.3389/fendo.2022.965487
11. Nakagawa N, Maruyama K, Hasebe N. Utility of geriatric nutritional risk index in patients with chronic kidney disease: A mini-review. Nutrients. (2021) 13(11):3688. doi: 10.3390/nu13113688
12. Miao S, Bao C, Zhang Y, Wang L, Jin X, Huang B, et al. Associations of the geriatric nutritional risk index with high risk for prostate cancer: A cross-sectional study. Nutrition. (2023) 115:112164. doi: 10.1016/j.nut.2023.112164
13. Shu W, Tao W, Chunyan H, Jie F, Yuan L, Yan X, et al. Preoperative nutritional evaluation of prostate cancer patients undergoing laparoscopic radical prostatectomy. Chen RJ, ed. PLoS One. (2022) 17(2):e0262630. doi: 10.1371/journal.pone.0262630
14. Siener R. Nutrition and kidney stone disease. Nutrients. (2021) 13(6):1917. doi: 10.3390/nu13061917
15. Di XP, Gao XS, Xiang LY, Wei X. The association of dietary intake of riboflavin and thiamine with kidney stone: A cross-sectional survey of NHANES 2007-2018. BMC Public Health. (2023) 23(1):964. doi: 10.1186/s12889-023-15817-2
16. Weinberg A, Patel C, Chertow G, Leppert J. Diabetic severity and risk of kidney stone disease. Eur Urol. (2014) 65(1):242–7. doi: 10.1016/j.eururo.2013.03.026
17. Li W, Wu H, Xu X, Zhang Y. Environmental exposure to perchlorate, nitrate, and thiocyanate in relation to chronic kidney disease in the general US population, NHANES 2005-2016. Chin Med J (Engl). (2023) 136(13):1573–82. doi: 10.1097/CM9.0000000000002586
18. Chang C, Ke H, Lee J, Lee Y, Jhan J, Wang H, et al. Metabolic syndrome increases the risk of kidney stone disease: A cross-sectional and longitudinal cohort study. J Pers Med. (2021) 11(11):1154. doi: 10.3390/jpm11111154
19. Ferraro P, Bargagli M, Trinchieri A, Gambaro G. Risk of kidney stones: Influence of dietary factors, dietary patterns, and vegetarian-vegan diets. Nutrients. (2020) 12(3):779. doi: 10.3390/nu12030779
20. Chewcharat A, Thongprayoon C, Vaughan L, Mehta R, Schulte P, O’Connor H, et al. Dietary risk factors for incident and recurrent symptomatic kidney stones. Mayo Clin Proc. (2022) 97(8):1437–48. doi: 10.1016/j.mayocp.2022.04.016
21. Liu N, Feng Y, Li J, Ma X, Ma F. Relationship between the dietary inflammatory index and kidney stone prevalence. World J Urol. (2022) 40(6):1545–52. doi: 10.1007/s00345-022-03998-1
22. Moludi J, Tandorost A, Kamari N, Abdollahzad H, Pakzad R, Najafi F, et al. Dietary total antioxidant capacity and its association with renal function and kidney stones: Results of a RaNCD cohort study. Food Sci Nutr. (2022) 10(5):1442–50. doi: 10.1002/fsn3.2753
23. Jian Z, Wang M, Jin X, Li H, Wang K. Diet-derived antioxidants and risk of kidney stone disease: Results from the NHANES 2007-2018 and mendelian randomization study. Front Nutr. (2021) 8:738302. doi: 10.3389/fnut.2021.738302
24. Lee M, Ke H, Huang J, Huang S, Geng J. Obesity-related indices and its association with kidney stone disease: A cross-sectional and longitudinal cohort study. Urolithiasis. (2022) 50(1):55–63. doi: 10.1007/s00240-021-01288-w
25. Peerapen P, Thongboonkerd V. Kidney stone prevention. Adv Nutr. (2023) 14(3):555–69. doi: 10.1016/j.advnut.2023.03.002
26. Sarica K. Obesity and stones. Curr Opin Urol. (2019) 29(1):27–32. doi: 10.1097/MOU.0000000000000557
27. Wang K, Ge J, Han W, Wang D, Zhao Y, Shen Y, et al. Risk factors for kidney stone disease recurrence: A comprehensive meta-analysis. BMC Urol. (2022) 22(1):62. doi: 10.1186/s12894-022-01017-4
28. Emami E, Heidari-Soureshjani S, Oroojeni Mohammadjavad A, Sherwin C. Obesity and the risk of developing kidney stones: A systematic review and meta-analysis. Iran J Kidney Dis. (2023) 1(2):63–72.
29. Zheng X, Chen Q, Wu Y, Xiong J. Association of body fat percentage with kidney stone disease: A cross-sectional and longitudinal study among bus drivers. BMC Public Health. (2023) 23(1):2174. doi: 10.1186/s12889-023-17128-y
30. Jin M, Zhu S, Hou Y. Insight on serum albumin: From structure and biological properties to functional biomaterials for bone repair. ACS Biomater Sci Eng. (2023) 9(5):2235–50. doi: 10.1021/acsbiomaterials.2c01553
31. Grzych G, Pekar J, Durand G, Deckmyn B, Maboudou P, Lippi G. Albumin-adjusted calcium and ionized calcium for assessing calcium status in hospitalized patients. Clin Chem. (2019) 65(5):703–5. doi: 10.1373/clinchem.2018.300392
32. Eyre K, Lewis F, Cui H, Grout E, Mihai R, Turney B, et al. Utility of blood tests in screening for metabolic disorders in kidney stone disease. BJU Int. (2021) 127(5):538–43. doi: 10.1111/bju.15250
33. Sheinenzon A, Shehadeh M, Michelis R, Shaoul E, Ronen O. Serum albumin levels and inflammation. Int J Biol Macromol. (2021) 184:857–62. doi: 10.1016/j.ijbiomac.2021.06.140
34. Remer T, Kalotai N, Amini A, Lehmann A, Schmidt A, Bischoff-Ferrari H, et al. Protein intake and risk of urolithiasis and kidney diseases: An umbrella review of systematic reviews for the evidence-based guideline of the German Nutrition Society. Eur J Nutr. (2023) 62(5):1957–75. doi: 10.1007/s00394-023-03143-7
35. Di X, Liu S, Xiang L, Jin X. Association between the systemic immune-inflammation index and kidney stone: A cross-sectional study of NHANES 2007-2018. Front Immunol. (2023) 14:1116224. doi: 10.3389/fimmu.2023.1116224
36. Roche M, Rondeau P, Singh N, Tarnus E, Bourdon E. The antioxidant properties of serum albumin. FEBS Lett. (2008) 582(13):1783–7. doi: 10.1016/j.febslet.2008.04.057
37. Soriani M, Pietraforte D, Minetti M. Antioxidant potential of anaerobic human plasma: Role of serum albumin and thiols as scavengers of carbon radicals. Arch Biochem Biophys. (1994) 312(1):180–8. doi: 10.1006/abbi.1994.1297
Keywords: Geriatric Nutritional Risk Index (GNRI), Kidney Stone Disease (KSD), National Health and Nutrition Examination Survey (NHANES), nutritional status, risk factors
Citation: Tang Z, Zhong H, Zhi Q, Chen Y, Zhang J, Li Z, Lu Z, Liu C, Tang F, He Z and Sun X (2024) The association between Geriatric Nutritional Risk Index and KSD disease: results from National Health and Nutrition Examination Survey 2007–2018. Front. Nutr. 11:1430668. doi: 10.3389/fnut.2024.1430668
Received: 22 May 2024; Accepted: 09 October 2024;
Published: 06 November 2024.
Edited by:
Biagio Barone, A.S.L. Napoli 1 Centro, ItalyReviewed by:
Luigi Napolitano, University of Naples Federico II, ItalyBenito Fabio Mirto, University of Naples Federico II, Italy
Copyright © 2024 Tang, Zhong, Zhi, Chen, Zhang, Li, Lu, Liu, Tang, He and Sun. This is an open-access article distributed under the terms of the Creative Commons Attribution License (CC BY). The use, distribution or reproduction in other forums is permitted, provided the original author(s) and the copyright owner(s) are credited and that the original publication in this journal is cited, in accordance with accepted academic practice. No use, distribution or reproduction is permitted which does not comply with these terms.
*Correspondence: Fucai Tang, dGFuZ2ZjQG1haWwuc3lzdS5lZHUuY24=; Zhaohui He, aGVjaGg5QG1haWwuc3lzdS5lZHUuY24=; Xuan Sun, aGVpamlsaW5AMTYzLmNvbQ==
†These authors have contributed equally to this work and share first authorship