- 1The Wujin Clinical College of Xuzhou Medical University, Changzhou, Jiangsu, China
- 2Department of Orthopedics, Wujin Hospital Affiliated with Jiangsu University, Changzhou, Jiangsu, China
- 3Jiangsu Province Key Laboratory of Anesthesiology, Xuzhou Medical University, Xuzhou, Jiangsu, China
- 4Department of Pharmacy, Wujin Hospital Affiliated with Jiangsu University, Changzhou, Jiangsu, China
- 5Department of Outpatient and Emergency, First Affiliated Hospital of Soochow University, Suzhou, Jiangsu, China
- 6Department of Good Clinical Practice, Wujin Hospital Affiliated with Jiangsu University, Changzhou, Jiangsu, China
Background: This study delves into the complex interplay between genetics, 25-hydroxyvitamin D (25OHD), and schizophrenia (SCZ). It leverages extensive sample data derived from Genome-Wide Association Studies (GWAS) to uncover genetic correlations.
Methods: Employing Linkage Disequilibrium Score Regression (LDSC) and S-LDSC, this study investigates genetic connections between 25OHD and SCZ. It examines Single Nucleotide Polymorphism (SNP) heritability in specific tissues and incorporates diverse immune cell datasets for genetic enrichment analysis. Local genetic correlations were analyzed using HESS software, and pleiotropy analysis identified shared genetic loci in brain tissues. Hyprcoloc analysis was used to explore shared genetic factors between 25OHD, immune cells, and SCZ, complemented by a bidirectional Mendelian Randomization (MR) to probe potential causal links.
Results: We identified a significant negative genetic correlation between 25OHD levels and SCZ. PLACO analysis revealed 35 pleiotropic loci with strong enrichment in brain regions, particularly the cerebellum, frontal cortex, and hippocampus. Eight loci (1p34.2, 2p23.3, 3p21.1, 5q31.2, 12q23.2, 14q32.33, 16p13.3, and 16q24.3) exhibited strong colocalization, highlighting potential drug targets. Gene and tissue enrichment analyses emphasized neurological and immune-related mechanisms, including hyaluronan metabolism. Bidirectional MR analysis supported a causal effect of SCZ on 25OHD levels.
Conclusion: Our study identifies NEK4 as a potential therapeutic target and highlights the involvement of hyaluronan metabolism in the genetic association between 25OHD and SCZ. These findings provide valuable insights into shared genetic pathways, immune-related connections, and causal interactions in the context of SCZ.
1 Introduction
Schizophrenia (SCZ) is a complex mental disorder that affects about 21 million people worldwide (1). Although the pathogenesis of SCZ is unclear, SCZ has been shown to have etiological links to events early in life, at birth and even in utero (2, 3). Epidemiological evidence has demonstrated that a person's risk of developing SCZ is influenced by factors such as childhood trauma, environmental displacement, social isolation, advancing urbanization, and substance abuse (4). In more detail, the prevalence is higher in children born in winter, living at high latitudes and growing up in cities (5–7). All of these factors are associated with reduced sunlight exposure, so low 25OHD levels are considered a risk factor for SCZ (8).
Most vitamin D is synthesized in the skin with the help of sunlight, and only a small portion is taken in through the diet (9). The amount of vitamin D synthesized depends on age, skin color, season, duration of sun exposure, and latitude of residence (10). Vitamin D3 is first hydroxylated in the liver to produce 25OHD, and then the physiologically active 1, 25 dihydroxyvitamin 2D (1, 25OH2D) is produced in the kidneys, so serum 25OHD levels are the best parameter to represent vitamin D levels (11). Recent studies have found that 25OHD, in addition to its involvement in the regulation of calcium and phosphorus metabolism in the body and the maintenance of bone health, is potentially linked to psychiatric disorders such as SCZ, depression and anxiety (12, 13). The association may be predicated on the fact that both 25OHD and 1, 25OH2D can cross the blood-brain barrier, and that vitamin D receptors and vitamin D metabolizing enzymes are present in human and rodent brain tissue (14–17). 25OHD increases the number of mature macrophages, raises the expression levels of CD36 and PPAR-γ in the brain, benefits neurological recovery and promotes the clearance of blood masses in mice after cerebral hemorrhage (18). Imaging studies have found a significant positive correlation between 25OHD levels and hippocampal volume in patients with SCZ (19). 25OHD has an important protective effect on the hippocampus.
Low levels of 25OHD in fetuses or newborns increase risk of SCZ (20). A Danish case-control study (N = 868) found a significant association between low 25OHD levels in newborns and the risk of SCZ later in life (21). Another case-control study (N = 2,602) with a larger sample size replicated the association of neonatal 25OHD deficiency with a significantly increased risk of SCZ (22). Such evidence points to the hypothesis that maternal 25OHD deficiency is a risk factor for SCZ (8). The meta-analysis found that people with SCZ were more likely to be 25OHD deficient (23, 24). However, this does not confirm an independent association: people with SCZ generally have reduced exercise and diet and do not spend enough time in the sun, which are known to contribute to lower 25OHD levels. In conclusion, controversy with regard to the true link between 25OHD and SCZ has been unsettled.
Traditional observational epidemiologic studies have many limitations in establishing causal relationships between disease exposures and outcomes, including reverse causal associations with potential confounders that bias results and render them unreliable (25). Well-designed randomized controlled trials are the gold standard for determining the association between 25OHD levels and the risk of SCZ, but are difficult to conduct for large samples followed over decades. By integrating genetic epidemiological methods such as Mendelian randomization (MR), pleiotropy, and genetic correlation analyses, potential associations in complex phenotypes can be explored more reliably. This approach has demonstrated its advantages in research across multiple fields (26–28). Numerous studies have shown that 25OHD is involved in evolutionary processes associated with SCZ pathogenesis, and genetic variation may influence such neurobiological processes directly or indirectly through 25OHD levels. Therefore, it is important to further investigate potential overlapping genetic structures. Based on these factors, we systematically evaluated the complex relationship between 25OHD and SCZ in terms of genetic correlation, pleiotropy, and causality (Figure 1).
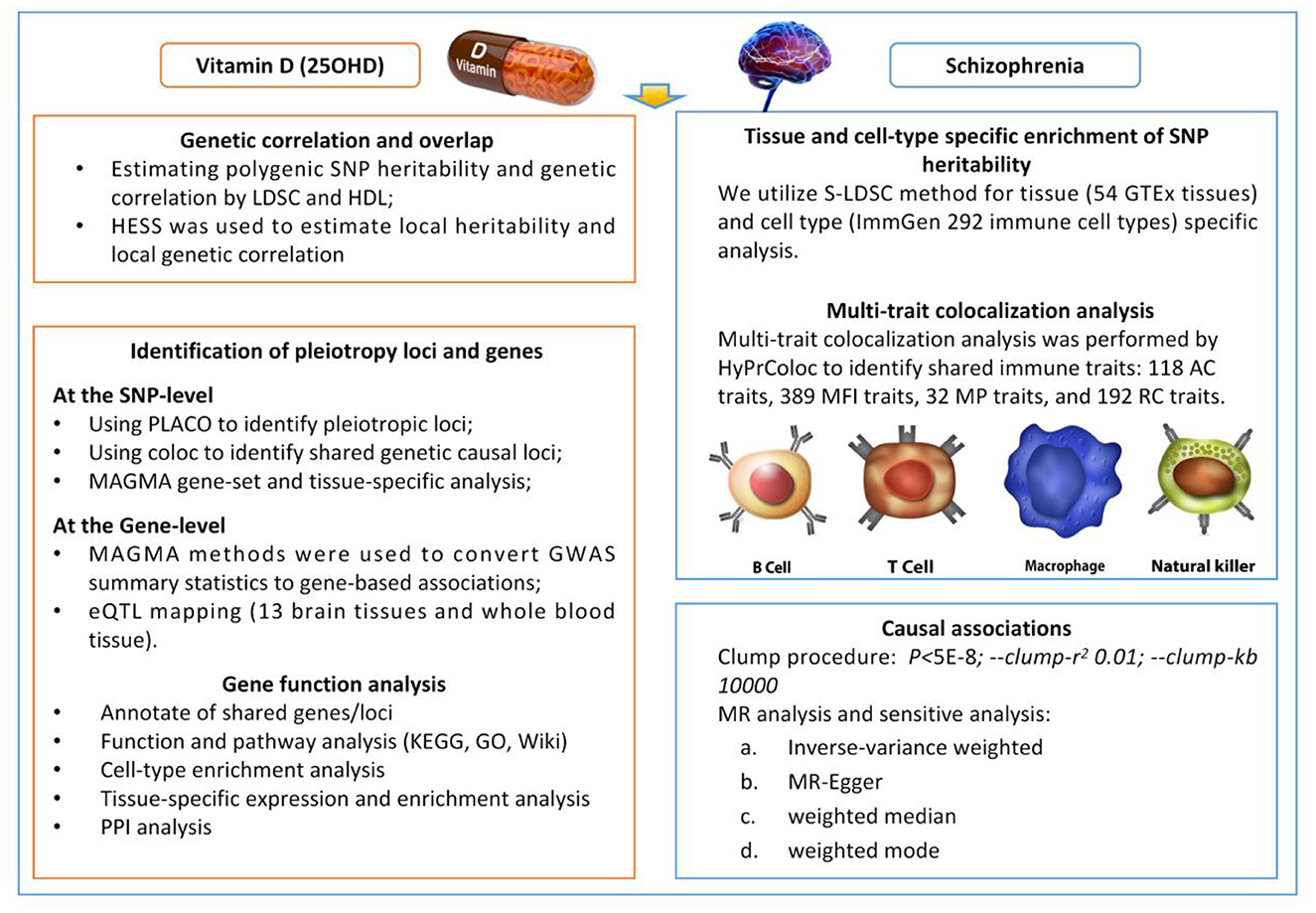
Figure 1. Overview of statistical analyses performed in the study. SNP, Single Nucleotide Polymorphism; LDSC, Linkage Disequilibrium Score Regression; HDL, high-definition likelihood; HESS, Heritability Estimation from Summary Statistics; PLACO, Protein–Ligand Affinity by Comparable Co-Evolution; MAGMA, Multi-marker Analysis of GenoMic Annotation; GWAS, Genome-Wide Association Study; eQTL, Expression Quantitative Trait Locus; S-LDS, Stratified Linkage Disequilibrium Score.
2 Materials and methods
2.1 Study design
In order to assess the complex genetic relationship between 25OHD and SCZ, this present study evaluated the genetic correlation between them using the linkage disequilibrium score regression (LDSC) method, and identified the corresponding pleiotropic loci and genes between the two phenotypes using the composite null hypothesis (PLACO). Finally we assessed the causal associations between 25OHD and SCZ using two-sample bidirectional MR method.
2.2 25OHD data
25OHD data were obtained from a genome-wide association study (GWAS) of 25OHD levels in 401,460 white British samples (29). The study measured 25OHD levels in twice-collected blood samples in a linear mixed model GWAS of standardized log-transformed 25OHD levels with covariates including age, sex, season, and vitamin D supplementation. A meta-analysis of GWAS results from 42,274 European samples was also performed, resulting in the detection of 138 conditionally independent Single Nucleotide Polymorphisms (SNPs, 63 novel) and an estimated SNP heritability of 16.1% for the 25OHD.
2.3 Schizophrenia data
The GWAS summary data for SCZ were derived from a two-stage genome-wide association study involving 55,365 European SCZ patients and 219,884 control individuals (30). This study reported 287 common variants associated with SCZ. In our study, we selected only European ancestry samples to align with the 25OHD dataset. In each individual cohort, association tests were performed using an additive logistic regression model with PLINK, and the results were finally meta-analyzed using an inverse variance-weighted fixed-effects model.
2.4 Linkage disequilibrium score regression
The Linkage Disequilibrium Score Regression (LDSC) method was used to analyze genetic correlations, showing how different traits might share common genetic factors (31). This method calculates the extent of shared genetic structures between traits, which helps identify their potential genetic links. LD scores in LDSC were calculated using European ancestry samples from the 1,000 Genomes Project and the HapMap3 project as reference panels (32). Additionally, high-definition likelihood (HDL) analysis was performed to increase the precision of these estimates and enhance statistical power (33), using a reference panel from approximately 300,000 individuals with European ancestry in the UK Biobank. For SNPs, stringent quality control measures were implemented: (1) Exclusion of non-biallelic SNPs and SNPs with ambiguous strand information; (2) Exclusion of SNPs without rs tags; (3) Removal of duplicate SNPs or those not present in the 1,000 Genomes Project or with mismatched alleles; (4) SNPs located within the major histocompatibility complex (chr6: 28.5–33.5 Mb) region were excluded from LDSC analysis due to their complex LD structure. (5) SNPs with a minor allele frequency (MAF) >0.01 were retained. Missing data were handled by excluding SNPs with incomplete information to prevent potential biases in the analysis.
2.5 Stratified linkage disequilibrium score regression
We used Stratified Linkage Disequilibrium Score Regression (S-LDSC) to explore whether genetic heritability for 25OHD and SCZ is enriched in specific cell types and tissues, such as the brain or immune cells. This method helps determine if certain tissues or cell types show stronger genetic influences related to these traits, providing insights into where these genetic effects are most active. We obtained human tissue data from GTEx consortium, including 54 different tissues (comprising 13 distinct brain tissues) (34), and obtained data on 292 immune cell types from the ImmGen consortium (35). After adjusting for baseline models and all gene sets, we used the p-values of the regression coefficient z-scores to evaluate the significance of SNP heritability enrichment estimates in each tissue and immune cell type.
2.6 Local genetic correlation analysis
We applied the Heritability Estimation from Summary Statistics (HESS) to estimate local genetic correlation and assessed the genetic overlap in distinct phenotypes within locally independent genomic regions (36). This approach helps identify areas in the genome where genetic overlap between 25OHD and SCZ may be concentrated, providing more detailed insights into specific genetic regions. A total of 1,702 independent LD regions were included in the analysis. In the first step, HESS computed the eigenvalues of the LD matrix, as well as the squared projection of the GWAS effect size vectors onto the eigenvectors of the LD matrix. In the second step, HESS utilized the output from step 1 to estimate local SNP heritability and its standard error. In the third step, HESS used the output from step 2 to obtain estimates of local genetic covariance and their standard errors. The reference dataset was constructed based on the hg19 genome using samples from the 1,000 Genomes European population.
2.7 Pleiotropic analysis under composite null hypothesis
SNP-Level PLACO is a novel method used to study the polygenic loci underlying complex traits using only summary-level genotype-phenotype association statistics (28, 37, 38). The purpose of PLACO analysis is to identify genetic regions that may be shared between 25OHD and SCZ. This type of pleiotropy analysis helps us pinpoint genetic loci that are linked to both traits, revealing potential shared genetic foundations and biological mechanisms. Specifically, we computed the square of the Z-scores for each variant and removed SNPs with extremely high Z2 (>80). Considering the potential correlation between 25OHD and SCZ, we estimated the correlation matrix of Z-scores. Subsequently, we used a level-α intersection-union test (IUT) method to assess the hypothesis of no pleiotropy. The final p-value for the IUT test was determined by the maximum p-value between H0 and H1.
Based on the PLACO results, we further mapped the identified loci to nearby genes to explore the shared biological mechanisms of these polygenic sites. We conducted a Generalized Gene-Set Analysis of GWAS Data (MAGMA) analysis on genes located at or overlapping with the polygenic loci identified based on PLACO output and single-trait GWAS to identify candidate pathways associated with polygenicity and tissue enrichment of polygenic genes (39). Functional Mapping and Annotation (FUMA) using genome-wide association study data were utilized to determine the biological functions of the polygenic loci (40). Pathway enrichment analysis was performed based on a range of pathways using the Molecular Signatures Database (MSigDB) to determine the functions of the mapped genes (41). eQTL analysis incorporated SNP-gene association data from whole blood tissues.
2.8 Colocalization analysis
To identify whether shared genetic regions between 25OHD and SCZ are likely due to common causal variants, we conducted Bayesian colocalization analysis using the R package “coloc.” This method helps determine if two traits have a true shared genetic cause at a given locus or if they simply overlap by chance. Colocalization analysis relies on the assumption of a single causal variant, and for each multi-trait locus, it yields posterior probabilities (PP) for five hypotheses: H0: Neither of the two traits has a genetic association in this region; H1: Only trait 1 has a genetic association in this region; H2: Only trait 2 has a genetic association in this region; H3: Both traits are associated but have different causal variants; H4: Both traits are associated and share a common causal variant. The “coloc.abf” function was employed for colocalization analysis (P1 = P2 = 1 × 10−4, P12 = 1 × 10−5).
2.9 Hypothesis prioritization for multi-trait colocalization analysis
We utilized the Hypothesis Prioritization for Multi-Trait Colocalization (HyPrColoc) method (42) to conduct multi-trait colocalization analysis by integrating GWAS summary statistics for 731 immune cells from the GWAS catalog (ranging from GCST0001391 to GCST0002121) (43). This analysis aimed to underscore the pivotal role of immune cells in the progression of 25OHD and SCZ. The immune cell traits encompassed various aspects, including 118 absolute cell counts (AC) traits, 389 median fluorescence intensity (MFI) reflecting surface antigen levels traits, 32 morphological parameters (MP) traits, and 192 relative cell counts (RC) traits. Notably, MFI, AC, and RC features covered a range of immune cell subsets, such as B cells, CDC, Ts cell maturation stages, mononuclear cells, bone marrow cells, TBNK, and Treg panels. The MP features were specific to CDC and TBNK panels. Primary GWAS analyses for 731 Immune traits were conducted on a cohort of 3,757 individuals of European descent, with covariates including sex, age, and age2. Furthermore, a reference panel based on European sequences was leveraged to estimate approximately 22 million SNPs genotyped using high-density arrays.
2.10 Mendelian randomization analysis
MR analysis in this study aims to investigate whether there is a potential causal relationship between 25OHD and SCZ. By using genetic variants as instrumental variables (IVs), MR helps estimate causal effects in a way that minimizes bias from other confounding factors. In this study, significant genetic loci are carefully selected, and sensitivity analyses ensure the reliability of the results, even when different assumptions are tested. This approach allows us to infer causality rather than just correlation, making it a powerful tool for understanding complex relationships in genetic data. In our analysis, we employed the clumping procedure in the PLINK software to select independently significant genetic loci as instrumental variables for two traits (P < 5 × 10−8) (41). The r2 threshold for instrumental variables was set at 0.001, with a physical distance of 10,000 kb window. We also calculated the r2 and F-statistic for each of them to ensure the strength of the instrumental variables (44) and the formula for calculating the F-statistic is as follows:
Here, r2 represents the proportion of variance explained by the instrumental variable, n is the sample size, and k is the number of SNPs.
The primary method used for Mendelian randomization (MR) was the Inverse Variance Weighted (IVW) method, which requires IVs to satisfy three assumptions: (1) IVs should be associated with the exposure; (2) IVs should not be associated with confounding factors related to both the exposure and the outcome; (3) The effect of IVs on the outcome is entirely mediated through the exposure. Several sensitivity analyses were conducted. First, the heterogeneity Q test of IVW and MR-Egger was used to detect potential violations of the assumptions through heterogeneity among the individual IVs (45). Second, intercept of MR-Egger was applied to estimate the horizontal pleiotropy, ensuring that genetic variation is independently associated with exposure and outcome (46). Additional analyses using different modeling assumptions and robust MR methods (weighted median and weighted mode) were employed to enhance the stability and robustness of the results.
All statistical analyses were performed using R version 3.5.3 software, and Mendelian randomization analyses utilized the Mendelian Randomization package (47).
3 Results
3.1 Genetic correlation between 25OHD and SCZ
In this study, we observed a significant genetic correlation between 25OHD and SCZ (rg = −0.083, P = 1 × 10−4) by LDSC. The intercept was −0.008 (with a standard error of 0.007), excluding the possibility of sample overlap between 25OHD and SCZ data. The LDSC method without constraining the intercept yielded consistent results (rg = −0.091, P = 3.48 × 10−9). Additionally, the HDL method also provided nearly identical results (rg = −0.103, P = 2.96 × 10−4). Supplementary Table S1 provides a detailed breakdown of the genetic correlations between 25OHD and SCZ, showing the consistency of results across different methods such as LDSC and HDL. The table highlights the significant negative genetic correlation observed and further supports the robustness of these findings. By using HESS, we estimated significant local genetic correlations in some genomic regions and observed negative genetic overlaps in these overlapping regions. But no significant difference was observed in respective local genetic heritability estimated for SCZ and 25OHD (Figure 2 and Supplementary Table S2).
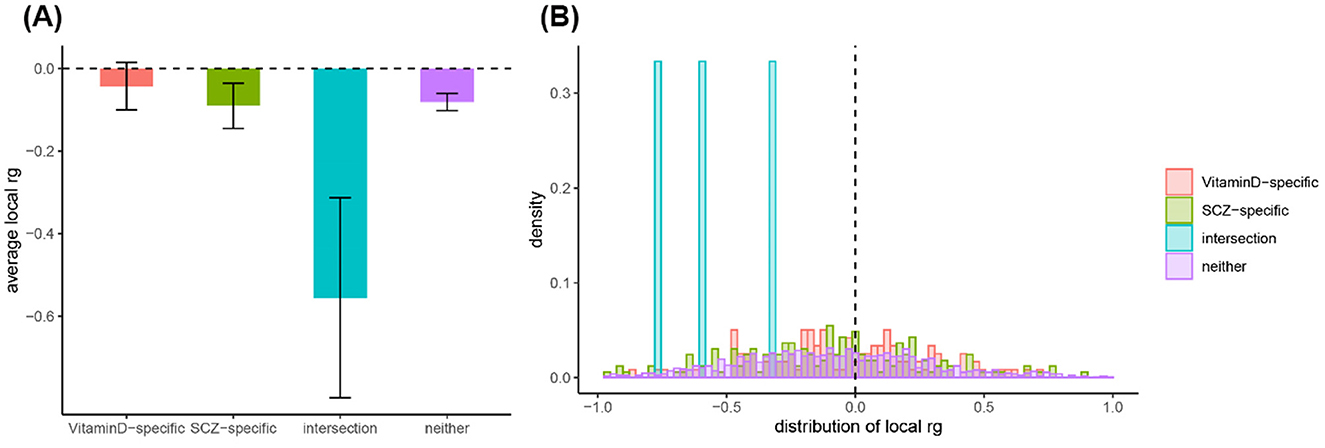
Figure 2. Analysis of local genetic correlation and heritability distribution across different genomic regions. (A) Average local genetic correlations (rg) for Vitamin D-specific, SCZ-specific, and interaction regions, highlighting the significant negative correlation in the interaction regions. (B) Frequency distribution of local genetic correlations, showing a concentration of negative correlations in interaction regions, suggesting a complex negative genetic relationship between Vitamin D and SCZ in these areas.
3.2 Pleiotropic GeneLoci identified for 25OHD and SCZ
By using PLACO analysis, we identified a total of 35 independent pleiotropic loci between 25OHD and SCZ, with eight genomic regions (FOXO6, GCKR, NEK4, CTB-35F21.1, RP11-328J6.1, PPP1R13B, CDIP1, and FANCA) having PP.H4 values greater than 0.7, indicating their significance in the association between 25OHD and SCZ. Figure 3A is the Manhattan plot of the identified signals, and detailed information on the identified pleiotropic loci was presented in Table 1 and Supplementary Table S3, including the genomic positions and their associated genes. We didn't find any evidence of genome inflation in the QQ Plot (Supplementary Figure S1), indicating that the results are free from systematic biases. Additionally, Supplementary Figure S2 provides essential information on each genomic risk locus, offering a detailed overview of the genetic architecture and variability across loci in the study. Supplementary Figure S3 shows significant enrichment of pleiotropic SNPs in intronic and intergenic regions, particularly in introns, suggesting a key role for non-coding regions in the genetic overlap between 25OHD and SCZ. Other regions, such as downstream and exonic areas, also show some enrichment but to a lesser extent. Regional plots for each risk locus are presented in Supplementary Figures S4–S11. Gene set enrichment analysis using MAGMA was performed based on the results of pleiotropy (Table 2), and the top 3 pathways were statins inhibit cholesterol production, plasma lipoprotein remodeling in responder group and vitamin D Metabolism. Furthermore, we identified nominal significant enrichment in the top six tissues (P < 1 × 10−3) using MAGMA tissue-specific analysis (Figure 4A), with five of these significantly enriched tissues being of cerebral origin, including the cerebellar hemisphere, cerebellum, and frontal cortex (BA9). These brain regions exhibited the highest enrichment levels, underscoring their potential involvement in the genetic relationship between 25OHD and SCZ (Supplementary Table S4). Additionally, the pituitary gland was also among the significantly enriched tissues, further highlighting the role of brain-related tissues in this association. It is worth noting that this section of the MAGMA gene set and tissue-specific analysis was conducted using the complete distribution of SNP p-values.
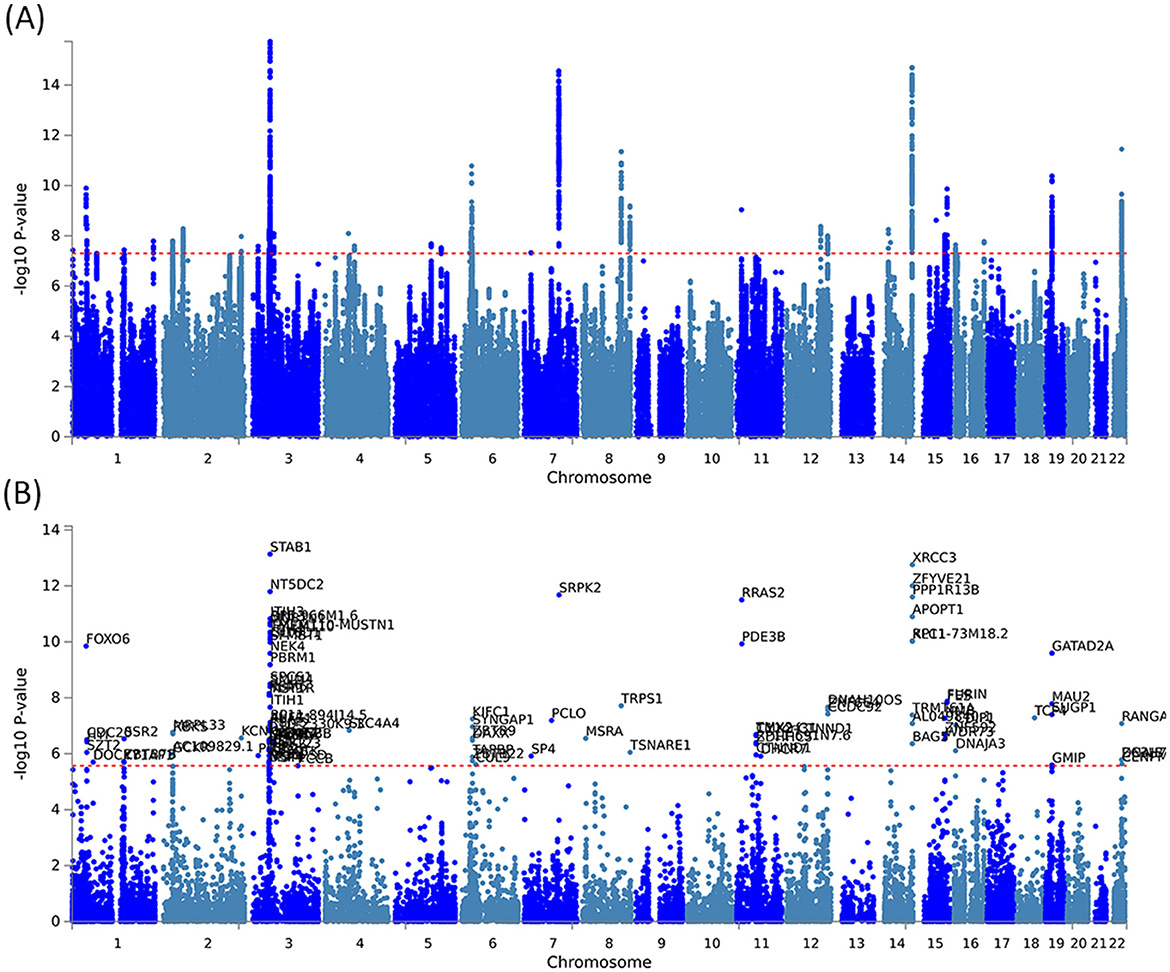
Figure 3. Manhattan plots illustrating pleiotropic associations between 25OHD and SCZ. (A) Manhattan plot showing pleiotropic SNP signals between 25OHD and SCZ, with significant associations observed on chromosomes 3, 7, 8, 14, and 19. (B) Manhattan plot highlighting pleiotropic genes identified by the MAGMA method, such as STAB1 and NT5DC2, which are significantly associated with both 25OHD and SCZ.
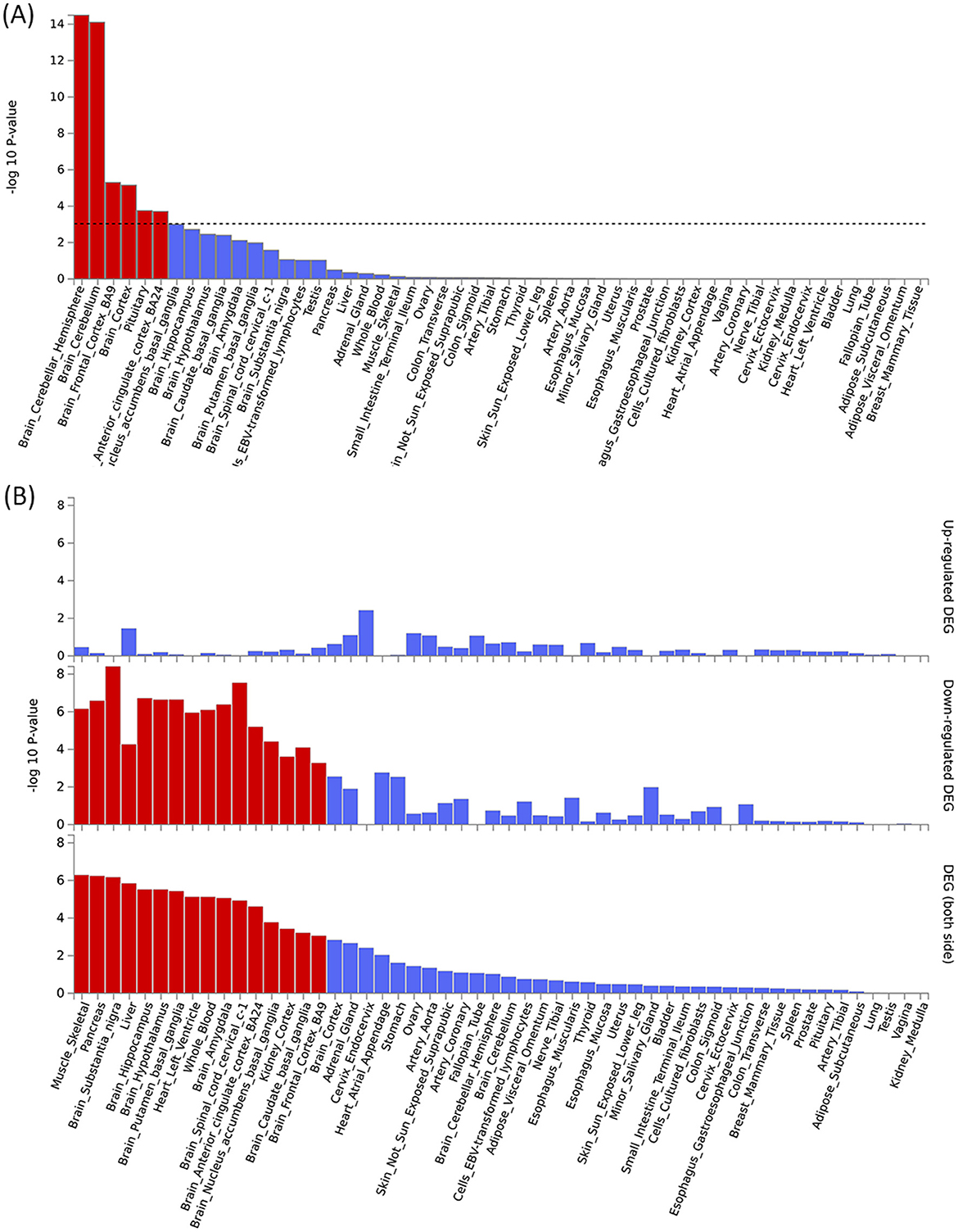
Figure 4. Tissue-specific analysis and gene enrichment of pleiotropic signals between 25OHD and SCZ. (A) Tissue-specific analysis reveals significant enrichment of pleiotropic signals in brain regions such as the cortex, frontal cortex, and cerebellum. (B) The enrichment of pleiotropic MAGMA genes is particularly pronounced in brain tissues, with both upregulated and downregulated genes showing significant associations, highlighting the central role of these regions in the genetic relationship between 25OHD and SCZ.
3.3 Identification of priority pleiotropic genes and functional enrichment
The pleiotropic genes were mapped using three different approaches, including the mapping of nearby genes based on the physical position of lead SNPs, MAGMA gene-level associations, and eQTL analysis (Supplementary Table S5). Supplementary Figure S12 and Supplementary Table S6 provide a comprehensive heatmap of gene expression levels across various tissues, with notable expression observed in the brain cortex, hippocampus, and cerebellum. Supplementary Figure S13 and Supplementary Table S7 illustrate the enrichment patterns of gene expression across multiple tissues. Significant enrichment is observed in regions such as the brain cortex, frontal cortex, and hippocampus. These findings suggest that these genes may have important roles in neurological functions, potentially linked to the genetic relationship between 25OHD and SCZ. MAGMA gene analysis identified a total of 105 pleiotropic genes (P < 2.69 × 10−6 = 0.05/18,565) (Figure 3B and Supplementary Table S8). Supplementary Figure S14 presents the QQ plot, showing a clear deviation from the expected line, particularly in the upper tail. This suggests the presence of significant genetic associations that contribute to the relationship between 25OHD and SCZ. The expression values of these genes across 54 different tissues can be found in Supplementary Figure S15 and Supplementary Table S9. Many genes were observed to be differentially expressed across the 13 brain tissues (e.g., STAB1, GLYCTK, and FOXO6), while several genes (e.g., CDC20, CENPM, and KIFC1) exhibited high expression in EBV-transformed lymphocytes, testis, liver, and whole blood. Tissue-specific enrichment analysis revealed the enrichment of these pleiotropic genes in skeletal muscle and various brain tissues (Supplementary Table S10 and Figure 4B). We further conducted pathway analysis (KEGG, Wiki, GO) after integrating the gene mapping based on positional information and MAGMA gene analysis (Supplementary Figure S16). We observed that these genes were significantly enriched in pathways such as 15q25 copy number variation, presynaptic active zone cytoplasmic component, and hyaluronan metabolic process. Through cell-specific enrichment analysis, the enrichment was primarily observed in three cell phenotypes: “Descartes fetal spleen afp alb positive cells”, “Lake adult kidney c3 proximal tubule epithelial cells s1 s2” and “Fan embryonic ctx nsc 2” (Supplementary Figure S17). eQTL mapping analysis was further conducted based on 13 brain and whole blood tissues, identifying a total of 329 pleiotropic genes. For detailed information, please refer to Supplementary Table S5. The overlap of genes between different methods is illustrated in Supplementary Figure S18 and a total of 11 (28%) pleiotropic genes were identified in all three mapping approaches (Supplementary Table S11).
3.4 Immune-related mechanisms shared between 25OHD and SCZ
Through S-LDSC, we observed significant enrichment of SNP heritability in 27 brain tissues and four immune cell phenotypes, which was significantly associated with the pleiotropic results between 25OHD and SCZ (P < 0.05) (Figure 5 and Supplementary Table S12). When analyzing the enrichment of immune traits from ImmGen, we also observed two cell phenotypes enriched in the T-cell panel: Tgd.vg2-.Sp and T.4SP24-.Th. Furthermore, within the innate lymphocyte panel, ILC3.NKp46-0.4-.SI, and within the B-cell panel, B.FrE.BM, were identified, suggesting potential shared immune mechanisms.
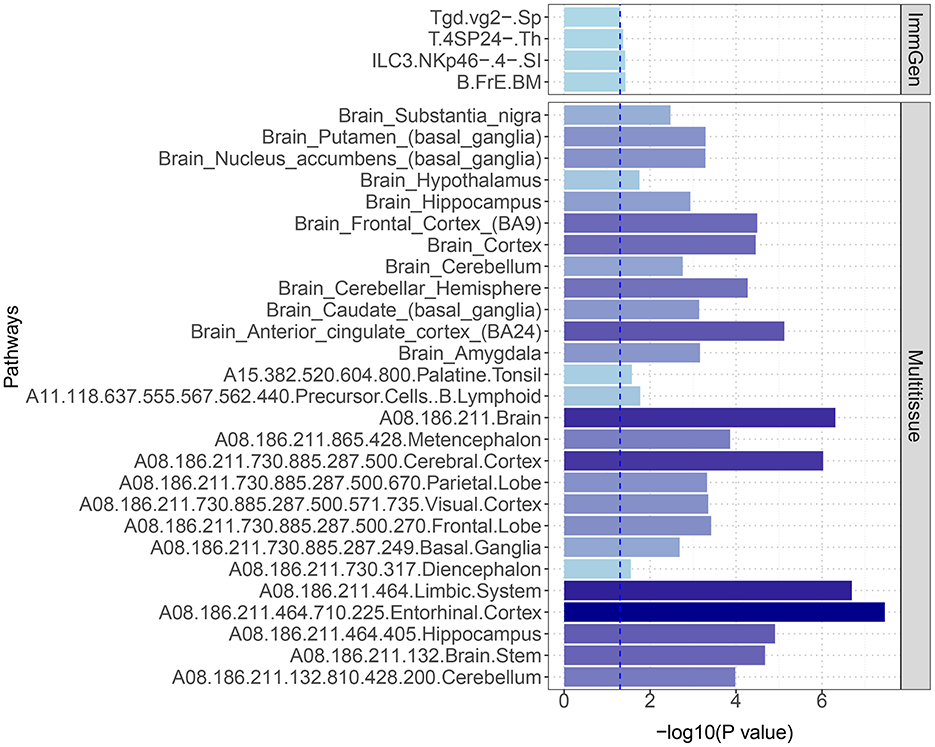
Figure 5. S-LDSC estimates of heritability enrichment across different immune cells and brain tissues. The figure highlights significant heritability enrichment in specific immune cells, such as Tgd.vg2-.Sp and T.4SP24-.Th, as well as in key brain regions including the entorhinal cortex, limbic system, and cerebral cortex. These findings suggest critical roles for both immune cells and these specific brain regions in the genetic relationship between 25OHD and SCZ.
3.5 Multi-trait colocalization analysis to pinpoint critical immune traits
Our multi-trait colocalization analysis, conducted using the HyPrColoc method, unveiled shared genetic loci among a diverse array of immune cell phenotypes, 25OHD and SCZ. Within the realm of immune cells, we observed significant genetic associations with several traits, such as CD80 on myeloid DCs, CD80 on CD62L+ myeloid DCs, and CD28 on CD39+ activated Treg cells (Supplementary Table S13). Notably, these findings included traits from different panels like cDC, Treg, B cells, and TBNK, which play pivotal roles in the immune system.
3.6 Causal association between 25OHD and SCZ
We employed a bidirectional two-sample MR approach to infer causality between exposure and outcome. The results provided support for a significant causal effect of SCZ on 25OHD levels, but failed to reveal a causal relationship from 25OHD to SCZ (Table 3). We employed both the global test of MR-PRESSO and the intercept term of MR-Egger to detect variants with disproportional effects and potential directional pleiotropy. Additionally, we utilized scatter plots and funnel plots to evaluate the stability of the association (Supplementary Figure S19). The scatter plot revealed the absence of multi-effect outliers that could influence causal inference. Meanwhile, the funnel plot displayed a balanced distribution of causal estimates around the effect estimates, confirming the robustness of the results. Supplementary Table S14 presents the information for the IVs.
4 Discussion
This study, based on extensive sample data from GWAS research, employed the LDSC method and identified a pronounced genetic correlation between 25OHD and SCZ (rg = −0.083, P = 1 × 10−4). S-LDSC extended this by assessing whether specific tissues, displayed significant enrichment of heritability, helping to localize the genetic influence to biologically relevant tissues. Using HESS, we estimated local genetic correlations, which allowed us to identify specific genomic regions where overlapping genetic effects are concentrated. Pleiotropy analysis then identified pleiotropic loci and found notable gene enrichment in brain tissues, suggesting a potential shared biological basis. To examine immune-related effects, HyPrColoc analysis integrated immune cell data to uncover shared genetic information across 25OHD, immune traits, and SCZ. Finally, bidirectional MR was used to explore potential causal effects between SCZ and 25OHD, allowing us to infer the directionality of their association.
Our study confirmed a significant genetic correlation between 25OHD and SCZ. Bidirectional two-sample MR analysis revealed that SCZ is a risk factor for 25OHD deficiency. Worldwide, the prevalence of 25OHD deficiency is as high as 37.6% in newborns and the elderly (48). A meta-analysis of 25OHD deficiency prevalence revealed that the overall prevalence of 25OHD deficiency in SCZ patients is 65.3%. Individuals with 25OHD deficiency are 2.16 times more likely to develop SCZ compared to those with sufficient levels of 25OHD (24). A more recent meta-analysis found that this prevalence has increased to 70% (23). Two studies based on Danish biobanks have confirmed the association between neonatal 25OHD deficiency and an increased risk of SCZ (21, 22). There is evidence to suggest that genetic variations associated with psychiatric disorders, including major depressive disorder (MDD), bipolar disorder (BIP), and SCZ, are related to lower concentrations of 25OHD. People with risk allele genes associated with psychiatric disorders may have lower 25OHD levels, regardless of clinical diagnosis (49). Most evidence suggests an association between 25OHD deficiency and cardiovascular diseases (CVD) (50). A recent randomized controlled trial involving 21,315 participants found a lower incidence of major cardiovascular events in the group receiving vitamin D3 supplementation (60,000 IU/month) compared to the placebo group, suggesting that vitamin D supplementation may reduce the occurrence of major CVD events (51). Our research findings suggest that individuals with a family history of SCZ should be vigilant about 25OHD deficiency to prevent the later development of CVD events.
Furthermore, a PLACO pleiotropy analysis of both disorders revealed 35 pleiotropic loci, with eight genomic regions (FOXO6, GCKR, NEK4, CTB-35F21.1, RP11-328J6.1, PPP1R13B, CDIP1, and FANCA) having PP.H4 values >0.7, indicating their significance in the association between 25OHD and SCZ. NEK4 has been reported as a shared genetic signal between BIP and SCZ (52). The study identified NEK4 as one of the risk genes associated with neuropsychiatric and substance use disorders. Pathway enrichment analysis revealed an enrichment of XWAS signals in 25OHD gene sets. This suggests that NEK4 may play a significant role in vitamin D metabolic pathways, potentially influencing the risk of disorders such as SCZ. A study identified that alternative splicing of NEK4 is regulated by sQTLs, which are significantly enriched in schizophrenia-associated loci. This suggests that dysregulation of NEK4 splicing may be a key genetic mechanism contributing to SCZ risk (53). A Mendelian randomization study identified a total of 31 promising drug targets for psychiatric disorders, with NEK4 being one of the significant genes specifically associated with SCZ (54). A summary data-based Mendelian randomization (SMR) analysis found that risk alleles in the chromosome 3p21.1 region are associated with NEK4 mRNA expression, and these alleles are significantly linked to SCZ (55). Another study involving 133 first-episode SCZ patients observed a significant association between the severity of SCZ negative symptoms and the risk gene FOXO6, which is associated with BIP (56). A study identifies FOXO6 as a novel candidate gene for SCZ through its pleiotropic effects on educational attainment (EA) and SCZ, revealed by using EA as a proxy phenotype (57). GCKR has been identified as one of the genes associated with alcohol use disorders. Moreover, it exhibits a positive genetic correlation with SCZ, indicating a potential connection between GCKR and SCZ within the framework of alcohol use disorders (58). In summary, among the 35 pleiotropic loci identified, NEK4 shows the strongest evidence and is considered a potential target for the association between 25OHD and SCZ. While there are some studies reporting the relationship between FOXO6 and GCKR with SCZ, there are no reported associations with 25OHD, warranting further investigation.
Tissue-specific analysis revealed significant enrichment of these pleiotropic genes in six tissues, with five originating from brain tissues (P < 1 × 10−3). Our multi-effect results were further subjected to gene analysis using MAGMA, identifying 105 pleiotropic genes. Enrichment analysis demonstrated that these genes are enriched in skeletal muscle and various brain tissues. This emphasizes the potential roles of these gene loci in brain-related processes and their functional impact on skeletal muscle. We further conducted pathway enrichment analysis and found significant enrichment in five pathways: “15q25 copy number variation,” “Presynaptic active zone cytoplasmic component,” “Hyaluronan metabolic process,” “Glyoxylate and dicarboxylate metabolism,” and “Oxidoreductase activity, acting on a sulfur group of donors.” In a large-scale GWAS meta-analysis investigating the genetic determinants of smoking quantity and their relevance to SCZ, multiple loci were identified. Notably, among these loci, the 15q25 region emerged as a common genetic locus associated with these traits. This locus has been linked to alterations in CHRNA5 expression in the brain (59). Perineuronal nets (PNNs) are highly organized mesh-like networks composed of extracellular matrix molecules surrounding neuronal cell bodies and proximal dendrites. The main components of PNNs include hyaluronan synthase, cartilage link protein-1, and chondroitin sulfate proteoglycans (60). It has been reported that PNN deficiencies can lead to frontal cortex dysfunction in individuals with SCZ, and abnormal PNN formation may increase the risk of SCZ episodes (61). In the context of liver fibrosis induced by sodium arsenite, 25OHD intervention using calcitriol significantly reduces hyaluronic acid levels, suggesting a potential protective effect of vitamin D on liver fibrosis by modulating hyaluronan metabolism (62). 25OHD deficiency may lead to reduced PNN function, resulting in abnormal cortical gamma band oscillations, thereby increasing the risk of SCZ and worsening the cognitive symptoms of SCZ (63). These observations indicate that the hyaluronan metabolism pathway could be a crucial link between 25OHD and the pathophysiology of SCZ. The synthesis and activation of vitamin D involve multiple enzymes, with some of these enzymes participating in redox reactions involving sulfur atoms within the organism. They alter the structure of vitamin D by introducing oxygen atoms into the molecule, thereby activating it (64). In conclusion, among the five significantly enriched pathways identified, “Hyaluronan metabolic process” shows the strongest evidence, indicating its potential role as a pathway linking 25OHD and SCZ. “15q25 copy number variation” and “Oxidoreductase activity” have some reported associations with SCZ or 25OHD, suggesting they are worthy of further investigation.
25OHD possesses potential immunomodulatory effects (65). Some epidemiological evidence suggests a significant association between 25OHD deficiency and an increased risk or exacerbation of infectious diseases and autoimmune conditions (66–68). A MR study has demonstrated a significant association between various immune cell phenotypes and the risk of SCZ (69). Many immune-related genes and pathways have been shown to be involved in neural development and neuronal function (70). Studies suggest that elevated neutrophil counts and C-reactive protein levels may be more strongly associated with the severity of SCZ (71). Through multi-trait colocalization analysis of GWAS data from 731 immune cell phenotypes, we conducted an in-depth investigation and observed shared genetic loci between multiple immune traits and both 25OHD deficiency and SCZ. We identified 19 immune cell phenotypes with significant genetic overlap (PP > 0.5), including cDC, Treg, B cell, and TBNK panels, among others. These molecules play critical roles in the immune system, deepening our genetic understanding of SCZ and underscoring the importance of the immune system in the disease's pathogenesis.
25OHD has properties that promote differentiation, inhibit proliferation, and prevent apoptosis in brain cells. Offspring of mice with 25OHD deficiency have been found to exhibit increased cell proliferation in the brain (72). Cell proliferation is indeed associated with brain structural abnormalities, and 25OHD deficiency has been shown to reduce the expression of neurotrophic factors such as nerve growth factor (NGF), glial cell line-derived neurotrophic factor (GDNF), and brain-derived neurotrophic factor in the brains of neonatal rats. This reduction could potentially lead to early brain dysfunction (73). Although MR results suggest a unidirectional effect of SCZ on 25OHD deficiency, this does not eliminate the possibility of a bidirectional relationship between these two factors. Given the intricate network of genetic correlations and pleiotropy, it is likely that the relationship between 25OHD and SCZ is multifaceted. One plausible explanation for this could be the complexity of SCZ etiology, which involves multiple genetic and environmental factors. SCZ pathogenesis is influenced by a wide range of environmental and lifestyle factors, including sunlight exposure, diet, and other socio-environmental elements, which play essential roles in vitamin D synthesis and metabolism (74–76). However, the potential positive effects of these factors on SCZ risk remain speculative (77). These factors add layers of complexity to SCZ etiology, making it unlikely that a single nutrient or metabolic factor could fully account for the disorder's development. In addition, vitamin D levels may play a more indirect or compensatory role rather than a primary causal role in SCZ. While our analysis found no direct causal link between 25OHD and SCZ, the inverse relationship where SCZ appears to influence 25OHD levels suggests that psychiatric conditions, lifestyle factors, or medication use in SCZ patients could negatively affect vitamin D metabolism or absorption. For instance, individuals with SCZ often have limited outdoor exposure and may exhibit poor dietary habits, both of which can lead to vitamin D deficiency (8, 78). Furthermore, antipsychotic medications, frequently prescribed for SCZ, have been shown to alter metabolic pathways, potentially affecting vitamin D levels (8). Several clinical randomized controlled trials have examined whether supplemental 25OHD levels improve symptoms of SCZ, but the results have been inconsistent. One study showed that vitamin D supplementation (50,000 IU vitamin D per week for 12 weeks) improved positive or negative symptoms of SCZ (79). Conversely, another study found no improvement in symptoms with vitamin D supplementation in patients using antipsychotic medications (80). Other small open-label studies have also shown inconsistent results (81, 82). The inconsistency of these trials may be attributed to varying baseline 25OHD levels, differences in patient profiles, or the impact of vitamin D on drug metabolism. Evidence suggests that vitamin D has a direct effect on drug metabolism by inducing CYP3A4, which may reduce the efficacy of antipsychotic medications that are CYP3A4 substrates (83). Studies have shown a negative correlation between serum vitamin D levels and the concentrations of such antipsychotics, indicating that while vitamin D may contribute to symptom relief (83), this effect could be counteracted by its impact on medication levels. Thus, while vitamin D supplementation remains important for overall health, particularly for those at risk of deficiency, current findings do not support its use as a primary or standalone treatment for SCZ. Instead, comprehensive approaches that consider the multifactorial nature of SCZ—including genetic, environmental, and lifestyle factors—are essential. For individuals at high risk of SCZ, strategies such as maintaining sufficient vitamin D levels through diet and sunlight exposure may offer general health benefits (9, 77), although their potential role in specifically reducing SCZ risk remains uncertain and requires further investigation. These lifestyle adjustments can help ensure adequate vitamin D, which may indirectly support health without the complexities introduced by direct supplementation in combination with antipsychotic treatment (84).
5 Limitation
This study has several limitations that should be considered. First, due to the use of summary-level data, we were unable to perform stratified analyses by key factors such as sex, age, or disease subtypes. While sex-specific effects were not explored, age-related differences and variations across SCZ subtypes could also influence the association between serum 25OHD levels and SCZ risk. Future studies utilizing individual-level data are necessary to assess potential interactions across these factors. Second, our analysis assumed a linear relationship between 25OHD and SCZ, as current MR methodologies primarily focus on linear associations. Although non-linear MR methods are emerging, they are still in their early stages and have significant limitations, particularly when applied to summary-level data. As a result, we were unable to robustly assess non-linear effects, which may provide deeper insights into the 25OHD-SCZ relationship. Methodological advancements and access to individual-level data could help address these issues in the future. Furthermore, the study predominantly included individuals of European ancestry, which may limit the generalizability of the findings to other ethnic populations. Genetic variations and environmental factors, such as differences in vitamin D metabolism, may vary across ethnic groups. We have noted that while several associations in MAGMA gene-set analysis were initially observed, they did not retain statistical significance after adjustment for multiple comparisons. We caution readers to interpret these findings conservatively, recognizing that the non-significant adjusted p-values may indicate that these associations are not robust to stringent statistical correction. Additionally, we have highlighted the need for further validation in independent cohorts to confirm the findings. Future research should include more diverse populations to enhance the external validity of the results. Lastly, while the MR approach strengthens causal inference, it is not without limitations. Our analysis may be subject to horizontal pleiotropy, where genetic variants influence SCZ risk through pathways independent of 25OHD. Although sensitivity analyses were performed to address this, residual pleiotropy cannot be entirely ruled out. Additionally, MR relies on the assumption that the genetic instruments are valid proxies for the exposure, which may not always hold true in complex traits like SCZ. Ultimately, the robustness of our results requires further validation through experimental and mechanistic studies to fully confirm the causal pathways.
6 Conclusions
Our study has unveiled the genetic correlation between 25OHD levels and SCZ, further supporting the hypothesis that 25OHD deficiency and SCZ have shared genetic mechanisms. We have identified pleiotropic genetic loci and pathways that link these two conditions. Importantly, our Mendelian randomization analysis suggests that SCZ influences 25OHD levels. This research may serve as a foundation for future investigations into shared genetic mechanisms and molecular interactions.
Data availability statement
The original contributions presented in the study are included in the article/Supplementary material, further inquiries can be directed to the corresponding authors.
Ethics statement
Ethical approval was not required for the study involving humans in accordance with the local legislation and institutional requirements. Written informed consent to participate in this study was not required from the participants or the participants' legal guardians/next of kin in accordance with the national legislation and the institutional requirements.
Author contributions
G-WR: Conceptualization, Data curation, Formal analysis, Investigation, Methodology, Validation, Visualization, Writing – original draft. X-ML: Investigation, Validation, Visualization, Writing – original draft, Formal analysis. H-ML: Formal analysis, Investigation, Validation, Visualization, Writing – original draft. M-ZS: Formal analysis, Investigation, Validation, Visualization, Writing – original draft. YJ: Investigation, Validation, Visualization, Writing – original draft, Conceptualization, Funding acquisition, Methodology, Project administration, Supervision, Writing – review & editing.
Funding
The author(s) declare financial support was received for the research, authorship, and/or publication of this article. This work was supported by the Open Funding Project of Jiangsu Provincial Key Laboratory of Anesthesiology, Xuzhou Medical University (Project ID: XZSYSKF2023011) and the Changzhou Sci & Tech Program (Project ID: CJ20242012) to YJ.
Acknowledgments
We thank all the studies for making the summary association statistics data publicly available.
Conflict of interest
The authors declare that the research was conducted in the absence of any commercial or financial relationships that could be construed as a potential conflict of interest.
The reviewer Y-QY declared a shared parent affiliation with the author H-ML to the handling editor at the time of review.
Publisher's note
All claims expressed in this article are solely those of the authors and do not necessarily represent those of their affiliated organizations, or those of the publisher, the editors and the reviewers. Any product that may be evaluated in this article, or claim that may be made by its manufacturer, is not guaranteed or endorsed by the publisher.
Supplementary material
The Supplementary Material for this article can be found online at: https://www.frontiersin.org/articles/10.3389/fnut.2024.1415132/full#supplementary-material
Abbreviations
25OHD, 25-hydroxyvitamin D; SCZ, schizophrenia; CNS, central nervous system; PLACO, pleiotropic locus colocalization analysis; MR, Mendelian randomization; LDSC, linkage disequilibrium score regression; HDL, high-definition likelihood; HESS, heritability estimation from summary statistics; SNP, single nucleotide polymorphism; eQTL, expression quantitative trait loci; S-LDSC, stratified linkage disequilibrium score regression; cDC, conventional dendritic cells; TBNK, T cells, B cells, natural killer cells; MFI, median fluorescence intensity; BA9, Brodmann Area 9; MR-PRESSO, Mendelian randomization pleiotropy residual sum and outlier; QQ, quantile-quantile; GWAS, genome-wide association study; IVs, instrumental variables; FDR, false discovery rate; GO, Gene Ontology; KEGG, Kyoto Encyclopedia of Genes and Genomes; MAGMA, Multi-marker Analysis of Genomic Annotation; ILC3, group 3 innate lymphoid cells; NKp46, natural killer cell p46-related protein; ICD, International Classification of Diseases; NEK4, NIMA-related kinase 4; MSigDB, Molecular Signatures Database; PP, posterior probability; MDD, major depressive disorder; BIP, bipolar disorder; CVD, cardiovascular disease.
References
1. Charlson FJ, Ferrari AJ, Santomauro DF, Diminic S, Stockings E, Scott JG, et al. Global epidemiology and burden of schizophrenia: findings from the global burden of disease study 2016. Schizophr Bull. (2018) 44:1195–203. doi: 10.1093/schbul/sby058
2. Jauhar S, Johnstone M, McKenna PJ. Schizophrenia. Lancet. (2022) 399:473–86. doi: 10.1016/S0140-6736(21)01730-X
3. Kotlicka-Antczak M, Pawełczyk A, Rabe-Jabłońska J, Smigielski J, Pawełczyk T. Obstetrical complications and Apgar score in subjects at risk of psychosis. J Psychiatr Res. (2014) 48:79–85. doi: 10.1016/j.jpsychires.2013.10.004
4. Stilo SA, Murray RM. Non-genetic factors in schizophrenia. Curr Psychiatry Rep. (2019) 21:100. doi: 10.1007/s11920-019-1091-3
5. Torrey EF, Miller J, Rawlings R, Yolken RH. Seasonality of births in schizophrenia and bipolar disorder: a review of the literature. Schizophr Res. (1997) 28:1–38. doi: 10.1016/S0920-9964(97)00092-3
6. Saha S, Chant D, McGrath J. Meta-analyses of the incidence and prevalence of schizophrenia: conceptual and methodological issues. Int J Methods Psychiatr Res. (2008) 17:55–61. doi: 10.1002/mpr.240
7. McGrath J, Scott J. Urban birth and risk of schizophrenia: a worrying example of epidemiology where the data are stronger than the hypotheses. Epidemiol Psichiatr Soc. (2006) 15:243–6. doi: 10.1017/S1121189X00002104
8. Cui X, McGrath JJ, Burne THJ, Eyles DW. Vitamin D and schizophrenia: 20 years on. Mol Psychiatry. (2021) 26:2708–20. doi: 10.1038/s41380-021-01025-0
9. Neriman A, Hakan Y, Ozge U. The psychotropic effect of vitamin D supplementation on schizophrenia symptoms. BMC Psychiatry. (2021) 21:309. doi: 10.1186/s12888-021-03308-w
10. Kinney DK, Teixeira P, Hsu D, Napoleon SC, Crowley DJ, Miller A, et al. Relation of schizophrenia prevalence to latitude, climate, fish consumption, infant mortality, and skin color: a role for prenatal vitamin d deficiency and infections? Schizophr Bull. (2009) 35:582–95. doi: 10.1093/schbul/sbp023
11. Yüksel RN, Altunsoy N, Tikir B, Cingi Külük M, Unal K, Goka S, et al. Correlation between total vitamin D levels and psychotic psychopathology in patients with schizophrenia: therapeutic implications for add-on vitamin D augmentation. Therap Adv Psychopharmacol. (2014) 4:268–75. doi: 10.1177/2045125314553612
12. Graham KA, Keefe RS, Lieberman JA, Calikoglu AS, Lansing KM, Perkins DO. Relationship of low vitamin D status with positive, negative and cognitive symptom domains in people with first-episode schizophrenia. Early Interv Psychiatry. (2015) 9:397–405. doi: 10.1111/eip.12122
13. Mayne PE, Burne THJ. Vitamin D in Synaptic Plasticity, Cognitive Function, and Neuropsychiatric Illness. Trends Neurosci. (2019) 42:293–306. doi: 10.1016/j.tins.2019.01.003
14. Cui X, Pelekanos M, Liu PY, Burne TH, McGrath JJ, Eyles DW. The vitamin D receptor in dopamine neurons; its presence in human substantia nigra and its ontogenesis in rat midbrain. Neuroscience. (2013) 236:77–87. doi: 10.1016/j.neuroscience.2013.01.035
15. Boontanrart M, Hall SD, Spanier JA, Hayes CE, Olson JK. Vitamin D3 alters microglia immune activation by an IL-10 dependent SOCS3 mechanism. J Neuroimmunol. (2016) 292:126–36. doi: 10.1016/j.jneuroim.2016.01.015
16. Landel V, Stephan D, Cui X, Eyles D, Feron F. Differential expression of vitamin D-associated enzymes and receptors in brain cell subtypes. J Steroid Biochem Mol Biol. (2018) 177:129–34. doi: 10.1016/j.jsbmb.2017.09.008
17. Eyles DW, Liu PY, Josh P, Cui X. Intracellular distribution of the vitamin D receptor in the brain: comparison with classic target tissues and redistribution with development. Neuroscience. (2014) 268:1–9. doi: 10.1016/j.neuroscience.2014.02.042
18. Liu J, Li N, Zhu Z, Kiang KM, Ng ACK, Dong CM, et al. Vitamin D enhances hematoma clearance and neurologic recovery in intracerebral hemorrhage. Stroke. (2022) 53:2058–68. doi: 10.1161/STROKEAHA.121.037769
19. Shivakumar V, Kalmady SV, Amaresha AC, Jose D, Narayanaswamy JC, Agarwal SM, et al. Serum vitamin D and hippocampal gray matter volume in schizophrenia. Psychiatry Res. (2015) 233:175–9. doi: 10.1016/j.pscychresns.2015.06.006
20. Berridge MJ. Vitamin D deficiency: infertility and neurodevelopmental diseases (attention deficit hyperactivity disorder, autism, and schizophrenia). Am J Physiol Cell Physiol. (2018) 314:C135–c51. doi: 10.1152/ajpcell.00188.2017
21. McGrath JJ, Eyles DW, Pedersen CB, Anderson C, Ko P, Burne TH, et al. Neonatal vitamin D status and risk of schizophrenia: a population-based case-control study. Arch Gen Psychiatry. (2010) 67:889–94. doi: 10.1001/archgenpsychiatry.2010.110
22. Eyles DW, Trzaskowski M, Vinkhuyzen AAE, Mattheisen M, Meier S, Gooch H, et al. The association between neonatal vitamin D status and risk of schizophrenia. Sci Rep. (2018) 8:17692. doi: 10.1038/s41598-018-35418-z
23. Zhu JL, Luo WW, Cheng X, Li Y, Zhang QZ, Peng WX. Vitamin D deficiency and schizophrenia in adults: a systematic review and meta-analysis of observational studies. Psychiatry Res. (2020) 288:112959. doi: 10.1016/j.psychres.2020.112959
24. Valipour G, Saneei P, Esmaillzadeh A. Serum vitamin D levels in relation to schizophrenia: a systematic review and meta-analysis of observational studies. J Clin Endocrinol Metab. (2014) 99:3863–72. doi: 10.1210/jc.2014-1887
25. Yu G, Xu M, Chen Y, Ke H. 25(OH)Vitamin D and autism spectrum disorder: genetic overlap and causality. Genes Nutr. (2023) 18:8. doi: 10.1186/s12263-023-00727-0
26. Jin Y, Yu X, Li J, Su M, Li X. Causal effects and immune cell mediators between prescription analgesic use and risk of infectious diseases: a Mendelian randomization study. Front Immunol. (2023) 14:1319127. doi: 10.3389/fimmu.2023.1319127
27. Yu XH, Yang YQ, Cao RR, Cai MK, Zhang L, Deng FY, et al. Rheumatoid arthritis and osteoporosis: shared genetic effect, pleiotropy and causality. Hum Mol Genet. (2021) 30:1932–40. doi: 10.1093/hmg/ddab158
28. Yu X, Chen Y, Chen J, Fan Y, Lu H, Wu D, et al. Shared genetic architecture between autoimmune disorders and B-cell acute lymphoblastic leukemia: insights from large-scale genome-wide cross-trait analysis. BMC Med. (2024) 22:161. doi: 10.1186/s12916-024-03385-0
29. Manousaki D, Mitchell R, Dudding T, Haworth S, Harroud A, Forgetta V, et al. Genome-wide association study for vitamin d levels reveals 69 independent loci. Am J Hum Genet. (2020) 106:327–37. doi: 10.1016/j.ajhg.2020.01.017
30. Trubetskoy V, Pardiñas AF Qi T, Panagiotaropoulou G, Awasthi S, Bigdeli TB, et al. Mapping genomic loci implicates genes and synaptic biology in schizophrenia. Nature. (2022) 604:502–8. doi: 10.1038/s41586-022-04434-5
31. Bulik-Sullivan B, Finucane HK, Anttila V, Gusev A, Day FR, Loh PR, et al. An atlas of genetic correlations across human diseases and traits. Nat Genet. (2015) 47:1236–41. doi: 10.1038/ng.3406
32. Auton A, Brooks LD, Durbin RM, Garrison EP, Kang HM, Korbel JO, et al. A global reference for human genetic variation. Nature. (2015) 526:68–74. doi: 10.1038/nature15393
33. Ning Z, Pawitan Y, Shen X. High-definition likelihood inference of genetic correlations across human complex traits. Nat Genet. (2020) 52:859–64. doi: 10.1038/s41588-020-0653-y
34. Lonsdale J, Thomas J, Salvatore M, Phillips R, Lo E, Shad S, et al. The genotype-tissue expression (GTEx) project. Nat Genet. (2013) 45:580–5. doi: 10.1038/ng.2653
35. ImmGen Consortium Benoist Christophe cbdm@ hms. harvard. edu 1 b. Open-source ImmGen: mononuclear phagocytes. Nat Immunol. (2016) 17:741. doi: 10.1038/ni.3478
36. Shi H, Mancuso N, Spendlove S, Pasaniuc B. Local genetic correlation gives insights into the shared genetic architecture of complex traits. Am J Hum Genet. (2017) 101:737–51. doi: 10.1016/j.ajhg.2017.09.022
37. Ray D, Chatterjee N. A powerful method for pleiotropic analysis under composite null hypothesis identifies novel shared loci between type 2 diabetes and prostate cancer. PLoS Genet. (2020) 16:e1009218. doi: 10.1371/journal.pgen.1009218
38. Hu YQ, Jin XJ, Lei SF Yu XH, Bo L. Inflammatory bowel disease and osteoporosis: common genetic effects, pleiotropy, and causality. Hum Immunol. (2024) 85:110856. doi: 10.1016/j.humimm.2024.110856
39. de Leeuw CA, Mooij JM, Heskes T, Posthuma D. MAGMA generalized gene-set analysis of GWAS data. PLoS Comput Biol. (2015) 11:e1004219. doi: 10.1371/journal.pcbi.1004219
40. Watanabe K, Taskesen E, van Bochoven A, Posthuma D. Functional mapping and annotation of genetic associations with FUMA. Nat Commun. (2017) 8:1826. doi: 10.1038/s41467-017-01261-5
41. Subramanian A, Tamayo P, Mootha VK, Mukherjee S, Ebert BL, Gillette MA, et al. Gene set enrichment analysis: a knowledge-based approach for interpreting genome-wide expression profiles. Proc Natl Acad Sci U S A. (2005) 102:15545–50. doi: 10.1073/pnas.0506580102
42. Foley CN, Staley JR, Breen PG, Sun BB, Kirk PDW, Burgess S, et al. A fast and efficient colocalization algorithm for identifying shared genetic risk factors across multiple traits. Nat Commun. (2021) 12:764. doi: 10.1038/s41467-020-20885-8
43. Orrù V, Steri M, Sidore C, Marongiu M, Serra V, Olla S, et al. Complex genetic signatures in immune cells underlie autoimmunity and inform therapy. Nat Genet. (2020) 52:1036–45. doi: 10.1038/s41588-020-0684-4
44. Burgess S, Thompson SG. Avoiding bias from weak instruments in Mendelian randomization studies. Int J Epidemiol. (2011) 40:755–64. doi: 10.1093/ije/dyr036
45. Thompson SG, Sharp SJ. Explaining heterogeneity in meta-analysis: a comparison of methods. Stat Med. (1999) 18:2693–708. doi: 10.1002/(SICI)1097-0258(19991030)18:20<2693::AID-SIM235>3.0.CO;2-V
46. Burgess S, Thompson SG. Interpreting findings from Mendelian randomization using the MR-Egger method. Eur J Epidemiol. (2017) 32:377–89. doi: 10.1007/s10654-017-0255-x
47. Broadbent JR, Foley CN, Grant AJ, Mason AM, Staley JR, Burgess S. MendelianRandomization v0.50: updates to an R package for performing Mendelian randomization analyses using summarized data. Wellcome Open Res. (2020) 5:252. doi: 10.12688/wellcomeopenres.16374.2
48. Hilger J, Friedel A, Herr R, Rausch T, Roos F, Wahl DA, et al. A systematic review of vitamin D status in populations worldwide. Br J Nutr. (2014) 111:23–45. doi: 10.1017/S0007114513001840
49. Albiñana C, Boelt SG, Cohen AS, Zhu Z, Musliner KL, Vilhjálmsson BJ, et al. Developmental exposure to vitamin D deficiency and subsequent risk of schizophrenia. Schizophr Res. (2022) 247:26–32. doi: 10.1016/j.schres.2021.06.004
50. Pál É, Ungvári Z, Benyó Z, Várbíró S. Role of vitamin D deficiency in the pathogenesis of cardiovascular and cerebrovascular diseases. Nutrients. (2023) 15:334. doi: 10.3390/nu15020334
51. Thompson B, Waterhouse M, English DR, McLeod DS, Armstrong BK, Baxter C, et al. Vitamin D supplementation and major cardiovascular events: D-Health randomised controlled trial. BMJ. (2023) 381:e075230. doi: 10.1136/bmj-2023-075230
52. Gedik H, Nguyen TH, Peterson RE, Chatzinakos C, Vladimirov VI, Riley BP, et al. Identifying potential risk genes and pathways for neuropsychiatric and substance use disorders using intermediate molecular mediator information. Front Genet. (2023) 14:1191264. doi: 10.3389/fgene.2023.1191264
53. Takata A, Matsumoto N, Kato T. Genome-wide identification of splicing QTLs in the human brain and their enrichment among schizophrenia-associated loci. Nat Commun. (2017) 8:14519. doi: 10.1038/ncomms14519
54. Li X, Shen A, Zhao Y, Xia J. Mendelian randomization using the druggable genome reveals genetically supported drug targets for psychiatric disorders. Schizophr Bull. (2023) 49:1305–15. doi: 10.1093/schbul/sbad100
55. Yang Z, Zhou D, Li H, Cai X, Liu W, Wang L, et al. The genome-wide risk alleles for psychiatric disorders at 3p21.1 show convergent effects on mRNA expression, cognitive function, and mushroom dendritic spine. Molecular psychiatry. (2020) 25:48–66. doi: 10.1038/s41380-019-0592-0
56. Shenker JJ, Sengupta SM, Joober R, Malla A, Chakravarty MM, Lepage M. Bipolar disorder risk gene FOXO6 modulates negative symptoms in schizophrenia: a neuroimaging genetics study. J Psychiat Neurosci. (2017) 42:172–80. doi: 10.1503/jpn.150332
57. Bansal V, Mitjans M, Burik CAP, Linnér RK, Okbay A, Rietveld CA, et al. Genome-wide association study results for educational attainment aid in identifying genetic heterogeneity of schizophrenia. Nat Commun. (2018) 9:3078. doi: 10.1038/s41467-018-05510-z
58. Sanchez-Roige S, Palmer AA, Fontanillas P, Elson SL, Adams MJ, Howard DM, et al. Genome-wide association study meta-analysis of the alcohol use disorders identification test (AUDIT) in two population-based cohorts. Am J Psychiatry. (2019) 176:107–18. doi: 10.1176/appi.ajp.2018.18040369
59. Ohi K, Kuwata A, Shimada T, Kataoka Y, Yasuyama T, Uehara T, et al. Genome-wide variants shared between smoking quantity and schizophrenia on 15q25 are associated with CHRNA5 expression in the brain. Schizophr Bull. (2019) 45:813–23. doi: 10.1093/schbul/sby093
60. Sethi MK, Zaia J. Extracellular matrix proteomics in schizophrenia and Alzheimer's disease. Anal Bioanal Chem. (2017) 409:379–94. doi: 10.1007/s00216-016-9900-6
61. Mauney SA, Athanas KM, Pantazopoulos H, Shaskan N, Passeri E, Berretta S, et al. Developmental pattern of perineuronal nets in the human prefrontal cortex and their deficit in schizophrenia. Biol Psychiatry. (2013) 74:427–35. doi: 10.1016/j.biopsych.2013.05.007
62. He R, Fan L, Song Q, Diao H, Xu H, Ruan W, et al. Protective effect of active vitamin D on liver fibrosis induced by sodium arsenite in SD rats. Wei Sheng Yan Jiu. (2022) 51:926–33. doi: 10.19813/j.cnki.weishengyanjiu.2022.06.012
63. Yu S, Park M, Kang J, Lee E, Jung J, Kim T. Aberrant gamma-band oscillations in mice with vitamin D deficiency: implications on schizophrenia and its cognitive symptoms. J Person Med. (2022) 12:318. doi: 10.3390/jpm12020318
64. Christakos S, Dhawan P, Verstuyf A, Verlinden L, Carmeliet G. Vitamin D: metabolism, molecular mechanism of action, and pleiotropic effects. Physiol Rev. (2016) 96:365–408. doi: 10.1152/physrev.00014.2015
65. Ismailova A, White JH. Vitamin D, infections and immunity. Rev Endocr Metab Disord. (2022) 23:265–77. doi: 10.1007/s11154-021-09679-5
66. Ao T, Kikuta J, Ishii M. The effects of vitamin D on immune system and inflammatory diseases. Biomolecules. (2021) 11:1624. doi: 10.3390/biom11111624
67. Martens PJ, Gysemans C, Verstuyf A, Mathieu AC. Vitamin D's effect on immune function. Nutrients. (2020) 12:1248. doi: 10.3390/nu12051248
68. Ahmad S, Arora S, Khan S, Mohsin M, Mohan A, Manda K, et al. Vitamin D and its therapeutic relevance in pulmonary diseases. J Nutr Biochem. (2021) 90:108571. doi: 10.1016/j.jnutbio.2020.108571
69. Wang C, Zhu D, Zhang D, Zuo X, Yao L, Liu T, et al. Causal role of immune cells in schizophrenia: Mendelian randomization (MR) study. BMC Psychiatry. (2023) 23:590. doi: 10.1186/s12888-023-05081-4
70. van Mierlo HC, Schot A, Boks MPM, de Witte LD. The association between schizophrenia and the immune system: Review of the evidence from unbiased 'omic-studies'. Schizophr Res. (2020) 217:114–23. doi: 10.1016/j.schres.2019.05.028
71. Steiner J, Frodl T, Schiltz K, Dobrowolny H, Jacobs R, Fernandes BS, et al. Innate immune cells and c-reactive protein in acute first-episode psychosis and schizophrenia: relationship to psychopathology and treatment. Schizophr Bull. (2020) 46:363–73. doi: 10.1093/schbul/sbz068
72. Eyles D, Brown J, Mackay-Sim A, McGrath J, Feron F. Vitamin D3 and brain development. Neuroscience. (2003) 118:641–53. doi: 10.1016/S0306-4522(03)00040-X
73. Hawes JE, Tesic D, Whitehouse AJ, Zosky GR, Smith JT, Wyrwoll CS. Maternal vitamin D deficiency alters fetal brain development in the BALB/c mouse. Behav Brain Res. (2015) 286:192–200. doi: 10.1016/j.bbr.2015.03.008
74. Chiang M, Natarajan R, Fan X. Vitamin D in schizophrenia: a clinical review. Evid Based Ment Health. (2016) 19:6–9. doi: 10.1136/eb-2015-102117
75. Kimlin MG. Geographic location and vitamin D synthesis. Mol Aspects Med. (2008) 29:453–61. doi: 10.1016/j.mam.2008.08.005
76. Wacker M, Holick MF. Sunlight and Vitamin D: a global perspective for health. Dermatoendocrinol. (2013) 5:51–108. doi: 10.4161/derm.24494
77. Hart PH, Norval M, Byrne SN, Rhodes LE. Exposure to ultraviolet radiation in the modulation of human diseases. Annu Rev Pathol. (2019) 14:55–81. doi: 10.1146/annurev-pathmechdis-012418-012809
78. Itzhaky D, Amital D, Gorden K, Bogomolni A, Arnson Y, Amital H. Low serum vitamin D concentrations in patients with schizophrenia. Israel Med Assoc J. (2012) 14:88–92.
79. Ghaderi A, Banafshe HR, Mirhosseini N, Moradi M, Karimi MA, Mehrzad F, et al. Clinical and metabolic response to vitamin D plus probiotic in schizophrenia patients. BMC Psychiatry. (2019) 19:77. doi: 10.1186/s12888-019-2059-x
80. Sheikhmoonesi F, Zarghami M, Mamashli S, Yazdani Charati J, Hamzehpour R, Fattahi S, et al. Effectiveness of vitamin D supplement therapy in chronic stable schizophrenic male patients: a randomized controlled trial. Iranian J Pharm Res. (2016) 15:941–50. doi: 10.22037/ijpr.2016.1942
81. Fond G, Godin O, Schürhoff F, Berna F, Bulzacka E, Andrianarisoa M, et al. Hypovitaminosis D is associated with depression and anxiety in schizophrenia: results from the national FACE-SZ cohort. Psychiatry Res. (2018) 270:104–10. doi: 10.1016/j.psychres.2018.09.024
82. Thakurathi N, Stock S, Oppenheim CE, Borba CP, Vincenzi B, Seidman LJ, et al. Open-label pilot study on vitamin D3 supplementation for antipsychotic-associated metabolic anomalies. Int Clin Psychopharmacol. (2013) 28:275–82. doi: 10.1097/YIC.0b013e3283628f98
83. Gaebler AJ, Finner-Prével M, Lammertz S, Schaffrath S, Eisner P, Stöhr F, et al. The negative impact of vitamin D on antipsychotic drug exposure may counteract its potential benefits in schizophrenia. Br J Clin Pharmacol. (2022) 88:3193–200. doi: 10.1111/bcp.15223
Keywords: vitamin D, schizophrenia, genetic overlap, Mendelian randomization, genome-wide association study
Citation: Rong G-W, Li X-M, Lu H-M, Su M-Z and Jin Y (2024) Association between 25(OH) vitamin D and schizophrenia: shared genetic correlation, pleiotropy, and causality. Front. Nutr. 11:1415132. doi: 10.3389/fnut.2024.1415132
Received: 10 April 2024; Accepted: 11 November 2024;
Published: 13 December 2024.
Edited by:
L. Joseph Su, University of Texas Southwestern Medical Center, United StatesReviewed by:
Yi-Qun Yang, Soochow University, ChinaArnim Johannes Gaebler, University Hospital RWTH Aachen, Germany
Copyright © 2024 Rong, Li, Lu, Su and Jin. This is an open-access article distributed under the terms of the Creative Commons Attribution License (CC BY). The use, distribution or reproduction in other forums is permitted, provided the original author(s) and the copyright owner(s) are credited and that the original publication in this journal is cited, in accordance with accepted academic practice. No use, distribution or reproduction is permitted which does not comply with these terms.
*Correspondence: Yi Jin, amlueWkmI3gwMDA0MDt3anJteXkuY24=; Xiao-Min Li, bGl4aWFvbWluJiN4MDAwNDA7d2pybXl5LmNu