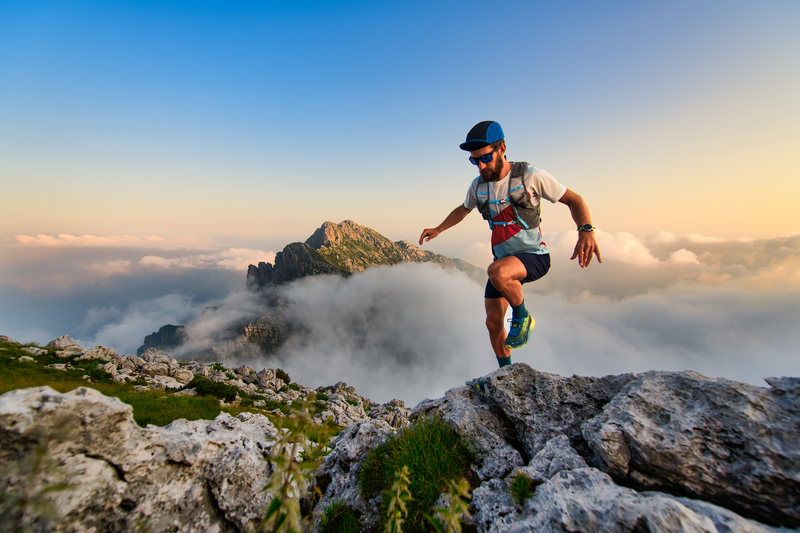
95% of researchers rate our articles as excellent or good
Learn more about the work of our research integrity team to safeguard the quality of each article we publish.
Find out more
ORIGINAL RESEARCH article
Front. Nutr. , 13 May 2024
Sec. Nutrition and Metabolism
Volume 11 - 2024 | https://doi.org/10.3389/fnut.2024.1379390
Introduction: The branched-chain amino acids (BCAAs) are essential to mammalian growth and development but aberrantly elevated in obesity and diabetes. Each BCAA has an independent and specific physio-biochemical effect on the host. However, the exact molecular mechanism of the detrimental effect of valine on metabolic health remains largely unknown.
Methods and results: This study showed that for lean mice treated with valine, the hepatic lipid metabolism and adipogenesis were enhanced, and the villus height and crypt depth of the ileum were significantly increased. Transcriptome profiling on white and brown adipose tissues revealed that valine disturbed multiple signaling pathways (e.g., inflammation and fatty acid metabolism). Integrative cecal metagenome and metabolome analyses found that abundances of Bacteroidetes decreased, but Proteobacteria and Helicobacter increased, respectively; and 87 differential metabolites were enriched in several molecular pathways (e.g., inflammation and lipid and bile acid metabolism). Furthermore, abundances of two metabolites (stercobilin and 3-IAA), proteins (AMPK/pAMPK and SCD1), and inflammation and adipogenesis-related genes were validated.
Discussion: Valine treatment affects the intestinal microbiota and metabolite compositions, induces gut inflammation, and aggravates hepatic lipid deposition and adipogenesis. Our findings provide novel insights into and resources for further exploring the molecular mechanism and biological function of valine on lipid metabolism.
The essential branched-chain amino acids (BCAAs: leucine, isoleucine, and valine) supplied in dietary protein are of functional importance to the metabolic health of mammals (1, 2). In metabolic diseases, such as obesity and diabetes, the level of BCAAs is increased and becomes a circulating hallmark of insulin resistance (2–5). As BCAAs accelerate lipid deposition in metabolic tissues (6), dietary protein restriction was proposed recently to reestablish metabolic health, through multiple nutrient-sensing signaling pathways, especially the mammalian target of the rapamycin complex 1 (mTORC1) pathway (7–9). However, the exact role of BCAAs in health and lifespan remains conflicting (10, 11).
BCAAs have been examined originally on their systematic effects and biological and physiological functions, focusing on metabolic organs. In brown adipose tissue, BCAAs were found to be transported into mitochondria by SLC25A44 and utilized for heat production (12). When cross-talk between the BCAA metabolism and the mitochondrial pyruvate carrier is inhibited, the plasma BCAA level is reduced by activating the mTOR axis (13). During insulin resistance (IR), the BCAA flux is affected by the adipocyte-specific neutral amino acid transporter SLC7A10 to enhance lipogenesis and elevate the circulating level of valine-derived metabolite 3-hydroxyisobutyrate (3-HIB) (14). Recent studies shift to the separate or specific role of each BCAA in adipogenesis and systemic metabolism. Leucine can relieve IR and the browning of white adipose tissue, but isoleucine showed contradictory results (15, 16). Isoleucine is a key regulator in the adverse metabolic response to BCAA (17), and its restriction increases the health span and longevity in mice (18). As for valine, it is detrimental to glucose uptake and IR, with negative impacts on metabolic homeostasis (19, 20). However, functional results on valine in humans and mice were largely obtained based on high-fat diet (HFD) feeding or obesity models. Without HFD intervention or in lean animals, valine is also demonstrated to be vital to growth, immunity, and reproduction (21, 22). We previously discovered that valine affected the tissue structure and induced apoptosis in mouse testis (23). However, the molecular mechanism underlying the detrimental effect of valine on metabolic health remains less explored.
The integrative omics method can be effectively and efficiently employed to study the molecular changes underlying complex traits of agricultural importance (24–26) and also the biochemical and physiological function of bioactive substances (27). In particular, the combined metagenome and metabolome analysis can help understand the intricate interaction between microbe and metabolite, and their involvement in BCAAs biosynthesis and metabolism. In humans with IR, the main bacterial species (Prevotella copri and Bacteroides vulgatus) can impact BCAA biosynthesis and augment circulating BCAA levels (28, 29). Metabolome analysis found that BCAA supplementation significantly increased the oxidation of valine, but not that of leucine (30). The intestinal mucosal integrity is compromised by BCAAs, and the microbial composition is also disturbed by valine (31). However, the relationship between the specific detrimental effect of valine on metabolic health and the composition of gut microbiome and metabolites in lean mice remains unknown (21).
In order to explore the molecular mechanism underlying the detrimental effect of valine on metabolic health, in the present study, we employed first transcriptome sequencing on both white and brown adipose tissues and then combined cecal metagenome and metabolome analyses on lean mice treated with L-valine. We identified that valine induced inflammation and compromised structure integrity of the small intestine and enhanced adipogenesis and hepatic lipid deposition. Moreover, for the composition of microbial species, Bacteroidetes were decreased, but those of Proteobacteria and Helicobacter were increased.
Animal care and experimental procedures were conducted in accordance with the Animal Research Committee guidelines of Yangtze University, Hubei Province, China (YZU-2018-0031).
KunMing (KM) mice (male, 3-week-old) were purchased from the China Three Gorges University Experimental Animal Center [Yichang, China, SCXK (E)2022–0012]. Mice were kept in the independent ventilation cages (IVC) system (Suhang Tech, Suzhou, China) (24 ± 2°C, humidity 50–60%) on a 12 h:12 h light–dark cycle. After 1-week of adaptation, mice (body weight range: 23.97 ± 1.33 g) were randomly divided into three groups (eight mice per group and four mice per cage): chow diet (control or 0 V), chow diet +0.30% L-valine (w/v) (0.3 V), and chow diet +0.45% L-valine (0.45 V). The mice fodder formula is shown in Supplementary Table S1. L-valine (purity >99%, V0010, Solarbio and Aladine, China) was supplemented to mice via drinking water (w/v) and made freshly every 3 days. We defined the doses of L-valine in preliminary experiments and used the same protocol to examine the effect of valine on mice testis development as published recently (23). All mice showed normal growth rates, and no death of animals occurred during all experiments. Mice in different groups were caged separately and treated for 30 days, with free access to a chow diet and drinking water. For each group, the amount of water and feed intake were measured each day and divided by four to get a value for each mouse. The body weights of each mouse were measured every 3 days. Stool samples of mice were collected and stored at −80°C for subsequent experiments.
After treatment for 30 days and fasting for 12 h, mice were euthanized by cervical dislocation, and the blood samples were collected and centrifuged in heparin tubes. The tissue samples of the liver, adipose tissues [epididymal WAT, interscapular BAT, and inguinal beige adipose tissue (BeAT)], the gut, and different segments of the small intestine (the duodenum, the jejunum, the ileum, and the cecum) were excised, and relevant phenotypic measures were collected. All samples collected were immediately stored at −80°C.
For histological analysis, the liver, the duodenum, the jejunum, and the ileum tissues collected for mice in each group were fixed in the 4% paraformaldehyde at room temperature overnight and then embedded in paraffin and sliced into 5 μm sections. The sections were dehydrated with 75, 85, 95, and 100% ethanol, sealed with Neutral Balsam (G8590, Solarbio) after transparent xylene, followed by the hematoxylin and eosin staining (HE staining kit, G1120, Solarbio). Slides were finally visualized, and images, villus height, and crypt depth of the small intestine were observed under an inverted microscope (OLYMPUS, IX73, Japan).
The levels of triglycerides (TG) and total cholesterol (TC) in the serum and the liver were determined using commercial assay kits (Nanjing Jiancheng Bioengineering Institute, Nanjing, China) according to the instructions of the manufacturer.
Transcriptome sequencing (RNAseq) included a total of 12 samples: for epididymal white adipose tissue (WAT), three controls (CW1–CW3) and three from the 0.45% valine group (VW1–VW3) were included; for interscapular brown adipose tissue (BAT), three controls (CB1–CB3) and three from the 0.45% valine group (VB1–VB3) were included. Total RNAs for white and brown adipose tissues were extracted using 1 mL TRIzol Reagent (Invitrogen Life Technologies, Carlsbad, CA, United States) according to the manufacturer’s instructions. After quality control (Supplementary Table S2), a total of 3 μg RNA per sample was used as the input materials for transcriptome sequencing (RNAseq). Sequencing libraries were constructed by the NEBNext® Ultra™ Directional RNA Library Prep Kit for Illumina® (NEB, United States) following the recommendations of the manufacturer. Library quality was assessed on the Agilent Bioanalyzer 2,100 system and was sequenced on the Illumina NovaSeq 6,000 platform to generate 150-bp paired-end reads.
Clean reads were obtained by removing raw reads containing adapter and N ratio greater than 0.002, and the reads with low-quality bases exceeding 50% of the length ratio of the reads in single-ended reads, by fastp-0.23.2. After filtering, the sequencing error rate (Q20 and Q30) and GC content distribution were calculated. Reference genome and transcript annotation files (GRCm39 Ensembl release 107) were downloaded directly from Ensembl.1 Bowtie2 and TopHat v2.0.9 were used to align the paired-end clean reads to the reference genome (32, 33). The mapped reads were assembled by Cufflinks (v2.1.1) in a reference-based approach (34), and genes with zero expression levels in each sample in the matrix were deleted. Deseq2 (1.36.0) was used to determine transcripts or genes with a P-adjust<0.05 and |log2foldchange|>1 to be significantly differentially expressed, after gene expression normalization. Gene ontology (GO) and Kyoto Encyclopedia of Genes and Genomes (KEGG) were used for gene enrichment analysis and functional annotation by clusterProfiler package in R (the significance threshold set at P-adjust<0.05).
The total RNA of the liver, adipose tissues (the WAT, the BAT, and the BeAT), and the small intestine (the duodenum, the jejunum, and the ileum) tissues were extracted using the TRIzol reagent (Takara, Japan) according to the instructions of the manufacturer. Reverse transcription was performed, and qRT-PCR was carried out on a 7,500 Real-Time PCR System (Applied Biosystems, Carlsbad, CA, United States), using the Roche FastStart Universal SYBR Green Master Mix (Roche Molecular Systems, Basel, Switzerland). β-Actin was chosen as the internal control and analyzed using the 2−ΔΔCT method (primer sequences are provided in Supplementary Table S3).
A total of six samples (control: C1-3, 0.45% valine: V1-3) were selected for shotgun metagenomics sequencing. According to the routine process of Beijing Novogene Technology Co., Ltd. (Beijing, China), the raw data obtained from the Illumina HiSeq sequencing platform using Readfq (V8, https://github.com/cjfields/readfq) were processed to obtain the clean data for subsequent analysis. The specific steps were as follows: (a) Remove reads with low-quality bases (default quality threshold is <38) that exceed a certain proportion (default length is 40 bp); (b) Remove reads with N bases reaching a certain proportion (default length is 10 bp); and (c) Remove reads whose overlaps with adapters exceed a certain threshold (default length is 15 bp). Considering the possibility of host contamination in samples, clean data needed to be BLASTed to the host database to filter out reads that may come from host origin. MEGAHIT software (v1.0.4-beta) was used for assembly analysis of clean data, with assembly parameter settings:--presets meta-large (−-end-to-end,--sensitive,-I 200,-X 400), and Scaftigs without N were obtained by breaking the resulted Scaffolds from the N junction. Clean data of each sample were aligned to the initial gene catalog by using Bowtie2 (Bowtie2.2.4) to calculate the number of reads of the genes on each sample alignment, with parameter settings: --end-to-end,--sensitive,-I 200, and -x 400. Genes with reads≤2 in each sample are filtered out to finally determine the gene catalog (Unigenes) for subsequent analysis.
DIAMOND software (V0.9.9.110, https://github.com/bbuchfink/diamond/) (35) was used for the alignment of Unigenes sequences with those of bacteria, fungi, archaea, and viruses extracted from the NR database of NCBI (Version 2018-01-02, https://www.ncbi.nlm.nih.gov/), with parameter settings:-blastp,-e 1e-5, and Evalue≤min. Evalue *10 of each sequence was selected. Meanwhile, DIAMOND software was used to align Unigenes with those in the functional database, with parameter settings: blastp,-e 1e-5. Functional databases include the KEGG database (Version 2018-01-01) (36), the eggNOG database (Version 4.5) (37), and the Carbohydrate-Active EnZymes (CAZy, Version 201,801) database (38). From the alignment results of each sequence, the best Blast hit results were selected. MetaStat and linear discriminant effect size analysis (LEfSe) were used to count the differential bacteria and metabolic pathways between the two groups.
A total of 12 samples (control: C1-6, 0.45% valine: V1-6) were selected for untargeted metabolomics. According to the process of Beijing Novogene Technology Co., Ltd. (Beijing, China), UHPLC–MS/MS analyses were performed using a Vanquish UHPLC system (Thermo Fisher, Germany), coupled with an Orbitrap Q ExactiveTM HF mass spectrometer (Thermo Fisher, Germany). The raw data files generated by UHPLC–MS/MS were processed using Compound Discoverer 3.1 (CD3.1, Thermo Fisher) to perform peak alignment, peak picking, and quantitation for each metabolite.
The metabolites were annotated using the KEGG database,2 the HMDB database,3 and the LIPID MAPS database.4 Principal components analysis (PCA) and partial least squares discriminant analysis (PLS-DA) were performed to verify the quality of the obtained data by metaX (39). The metabolites with VIP > 1 and a p-value of <0.05 and a fold change of ≥1.2 or an FC of ≤0.833 were considered to be differential metabolites. Volcano plots were produced by ggplot2, and the clustering heat maps were plotted by the Pheatmap package in R language. The functions of these metabolites and metabolic pathways were studied using the KEGG database after the enrichment of differential metabolites.
The top 10 differential bacteria in the metagenome and the top 20 differential metabolites in the metabolomic were selected for correlation analysis. Pearson’s correlation coefficient R was calculated (scipy.stats.pearsonr in python SciPy) between the relative abundance of each different genus and the quantitative values of different metabolites at the genus level. The heatmap of correlation analysis was drawn by using the corrplot package in R.
Levels of adenosine 5′-monophosphate (AMP)-activated protein kinase (AMPK), pAMPK, and stearoyl-coenzyme A desaturase 1 (SCD1) (Enzyme-linked Biotechnology Co., Ltd., Shanghai, China) in the hepatic and adipose tissues, and two metabolites, stercobilin (Cloud-Clone Corp., Houston, United States) and indole-3-acetic acid (3-IAA) (Enzyme-linked Biotechnology Co., Ltd., Shanghai, China), in the small intestine (the duodenum, the jejunum, and the ileum) and stool, were assessed using commercial ELISA kits, respectively.
Data analyzed by Student’s t-test, a one-way analysis of variance (ANOVA), and the least significant difference (LSD) for gene expression, protein, and metabolite abundances were expressed as mean ± SEM. Statistical analysis was performed using Prism 5.0 (GraphPad Software, Inc.) and SPSS Statistics 25 (IBM) software.
The detrimental effect of valine on metabolic homeostasis was mainly demonstrated in HFD-treated or obese animal or human subjects (19–23). Thus, we began with the experiment on the effect of valine on the growth and metabolism of lean mice. No significant differences were observed for the body weight and daily growth rate (Supplementary Figures S1A,B) and also for the feed and water intake (Supplementary Figures S1C,D) between the 0 V and valine treatment (0.3 V and 0.45 V) groups. After tolerance tests for glucose (GTT), pyruvate (PTT), and insulin (ITT) (Supplementary Figures S1E–G), it showed that mice treated with valine were slightly less sensitive to insulin. Furthermore, we assayed the levels of TG and TC. Significant differences did not exist in serum (Figure 1A). However, in the liver, both TG and TC levels were different between the 0.3 V and 0.45 V groups (p < 0.05) (Figure 1B).
Figure 1. Disorganization of hepatic and intestinal tissue structure by valine treatment. TG and TC levels in (A) serum; and (B) liver; (C) hepatic histology staining; the villus height, crypt depth, villus/crypt ratio, and HE staining for (D) and (G) duodenum; (E) and (H) jejunum; (F) and (I) ileum. *p < 0.05, **p < 0.01, ***p < 0.001.
Histology staining on the liver and small intestine tissues showed that compared to the 0 V group, 0.3 V and 0.45 V groups disturbed the hepatic structure, with more disoriented and scarce hepatic cords, narrower sinusoid, and more vacuoles (Figure 1C). As for the small intestine, valine treatment significantly reduced the crypt depth for the duodenum (p < 0.05) (Figures 1D,G), but without effect on the jejunum (Figures 1E,H). Notably, for the ileum, the villus height (p < 0.05 or p < 0.001), crypt depth (p < 0.05 or p < 0.01), and their ratio (p < 0.001) were increased between the 0 V and valine treatment (0.3 V and 0.45 V) groups (Figures 1F,I).
Next, we profiled the expression levels of genes related to β-oxidation [lipoprotein lipase (Lpl), acyl-coenzyme A oxidase 1 (Acox1), peroxisome proliferator-activated receptor α (Pparα), and carnitine palmitoyltransferase 1 (Cpt1)] and adipogenesis [CCAAT/enhancer binding protein α (Cebpα), Scd1, sterol regulatory element binding transcription factor 1 (Srebp-1c), fatty acid synthase (Fas), and acetyl-CoA carboxylase 1 (Acaca)] in hepatic and adipose tissues. For β-oxidation genes (Figures 2A–D), LPl and Acox1 were enhanced in the liver (p < 0.01 or p < 0.001), WAT (p < 0.05 or p < 0.01 or p < 0.001), and BAT (p < 0.05 or p < 0.01) for 0.3 V and 0.45 V groups, except for the BeAT. Pparα was inhibited in the liver (p < 0.01), BeAT (p < 0.001), and BAT (p < 0.05) for the 0.45 V group, but elevated in WAT (p < 0.01) for the 0.3 V group. Similarly, Cpt1 was inhibited in the liver (p < 0.01) and BeAT (p < 0.01) for the 0.45 V group but enhanced in BAT (p < 0.01) for the 0.3 V group.
Figure 2. Enhanced hepatic lipid deposition and adipogenesis by valine. Expression profiling on genes related to β-oxidation and adipogenesis. (A,E) Liver; (B,F) WAT; (C,G) BeAT; (D,H) BAT; Protein abundances of (I) AMPK; (J) p-AMPK; (K) p-AMPK/AMPK ratio; (L) SCD1 in the vertical panel, for the liver (up), WAT (middle), and BAT (down), respectively. *p < 0.05, **p < 0.01, ***p < 0.001.
As for adipogenesis genes, they were all significantly enhanced in WAT (Cebpα, p < 0.01; Scd1, Srebp-1c, Fas and Acaca, p < 0.05) for the 0.45 V group. Scd1 was disturbed in all tissues for the 0.45 V group, enhanced in the liver (p < 0.05), WAT (p < 0.05), and BAT (p < 0.01), but inhibited in BeAT (p < 0.01), respectively (Figures 2E–H). For the other genes, Cebpα was elevated in the liver (p < 0.001) and WAT (p < 0.001) of the 0.45 V group (Figures 2E,F); Fas was elevated in WAT (p < 0.05) of the 0.45 V group (Figure 2F); Acaca was elevated in WAT (p < 0.05) but inhibited in BAT (p < 0.05) of the 0.45 V group, respectively (Figures 2F,H).
The protein abundances of AMPK, pAMPK, and SCD1 were further assayed in the liver, WAT, and BAT. As AMPK was elevated in the liver and WAT (p < 0.05) (Figure 2I) and pAMPK was reduced in WAT (p < 0.05) (Figure 2J), leading to the decrease in the pAMPK/AMPK ratios (p < 0.05, liver; p < 0.01, WAT) (Figure 2K). In WAT and BAT, SCD1 abundances were all increased (p < 0.05) (Figure 2L), consistent with its transcription levels.
As lipid deposition in the liver and adipose tissues was affected by valine treatment (0.45 V), we next performed WAT and BAT transcriptome sequencing (RNAseq), to check whether valine reprogrammed the global transcriptome. Raw reads were filtered to obtain clean reads of good quality for downstream analysis (Supplementary Table S4). The distribution of gene expression levels showed the transcriptional dynamics for each sample (Supplementary Figures S2A,B), and after PCA, samples could be clustered into two separate groups for WAT and BAT, respectively (Supplementary Figures S2C,D).
After multiple significance testing, 740 and 980 significantly differentially expressed genes (DEGs) were found for WAT (up, 534; down, 206) and BAT (up, 517; down, 465), respectively (Figures 3A,B, 4A,B). For WAT, gene enrichment analysis based on DEGs found the following GO terms (CC: transmembrane transporter complex, high-density lipoprotein particle; BP: protein maturation, regulation of reproductive process, hormone metabolic process; MF: heparin binding, serine hydrolase activity, steroid binding) (Figure 3C). The top-ranked DEGs (normalized counts ≥10, framed in red for CC, BP, and MF) were mostly downregulated, except for Hp and Ccn2 (Figure 3D). For BAT, the GO terms were enriched as follows (CC: protein kinase complex; BP: regulation of inflammatory response, lipid localization, fat cell differentiation, regulation of lipid metabolic process, regulation of protein secretion, regulation of autophagy, and circadian rhythm; MF: carbohydrate binding) (Figure 4C). The top-ranked DEGs in protein kinase complex, regulation of protein secretion, circadian rhythm, and carbohydrate binding were upregulated, whereas those in the regulation of inflammatory response and lipid localization were downregulated (Figure 4D).
Figure 3. White adipose tissue transcriptome sequencing. (A) volcano plots; (B) heatmap of DEGs; (C) gene ontology (GO); (D) top three genes in each GO term; (E) KEGG pathways; (F) top three genes in each signaling pathway.
Figure 4. Brown adipose tissue transcriptome sequencing. (A) volcano plots; (B) heatmap of DEGs; (C) gene ontology (GO); (D) top three genes in each GO term; (E) KEGG pathways; (F) top three genes in each signaling pathway.
KEGG pathway analysis was then performed. For WAT, the WNT signaling pathway, bile secretion, circadian entrainment, mineral absorption, and cholesterol metabolism were found (Figure 3E). Nearly all DEGs were downregulated, except for the Per1 gene (Figure 3F). For BAT, the MAPK signaling pathway, ribosome, type I diabetes mellitus, circadian rhythm, and T-cell receptor signaling pathway were found (Figure 4E). DEGs were upregulated in circadian rhythm and downregulated in ribosome and type I diabetes mellitus (Figure 4F). Venn diagram showed that 57 common DEGs existed for WAT and BAT, but were not enriched for any signaling pathways (Supplementary Figure S3 and Supplementary Table S5).
To validate RNAseq results, nine DEGs were selected for WAT [ATPase Na+/K+ transporting subunit α 1 (Atp1a1), apolipoprotein D (Apod), haptoglobin (Hp), neutral cholesterol ester hydrolase 1 (Nceh1), calcium voltage-gated channel subunit α 1 H (Cacna1h), PRKC apoptosis WT1 regulator (Pawr), secreted frizzled-related protein 1 (Sfrp1), cellular communication network factor 2 (Ccn2) and period circadian clock 1 (Per1)], and BAT [complement component 3 (C3), Hp, amylo-1, 6-glucosidase, 4-α-glucanotransferase (Agl), Per2, protein kinase, AMP-activated, α 2 catalytic subunit (Prkaa2), fatty acid binding protein 5, epidermal (Fabp5), nuclear receptor subfamily 1, group D, member 1 (Nr1d1), and zinc finger CCCH type containing 12A (Zc3h12a) and Per1]. For WAT, all nine DEGs had similar expression patterns to those of RNAseq (Figures 5A,B). For BAT, seven DEGs showed the same pattern, except for Fabp5 and Zc3h12a (Figures 5C,D).
Figure 5. Transcriptional profiling of DEGs in white and brown adipose tissues. RNAseq and transcriptional validation of DEGs, for (A,B) WAT; (C,D) BAT, respectively. *p < 0.05, **p < 0.01, ***p < 0.001.
Gut microbiota is inextricably linked to obesity and IR (29), and we found that valine induced insulin insensitivity, disrupted intestinal and hepatic tissue structure and enhanced lipid deposition. Thus, we examined the cecal microbial composition and metabolites by integrating metagenome sequencing and untargeted metabolomics. First, PCoA clearly clustered the 0 V and 0.45 V samples into two distinct groups (Supplementary Figure S4A). Two groups had 992,469 common genera (Venn diagram), and 82,151 and 101,009 were specific to the 0 V and 0.45 V groups, respectively (Supplementary Figure S4B). Relative microbial abundance showed that at the phylum level, 0.45% valine reduced the proportion of Bacteroidetes, but increased that of Proteobacteria (p < 0.05) (Figure 6A); and at the genus level, Bacteroidetes and Helicobacter were reduced and increased, respectively (p < 0.05) (Figure 6B).
Figure 6. Gut metagenome sequencing. Dynamic changes of different proportions of microbe at the levels of (A) phylum; (B) genus; (C) LDA of different microbes; (D) heat map of KEGG signaling pathways (level 2); (E) LEfSe analysis identified 22 tertiary signaling pathways (LDA Score ≥ 2); (F) Signaling pathways enriched.
After microbial linear discriminant analysis (LDA) (Figure 6C) and KEGG pathway analysis (Figure 6D), the endocrine and metabolic diseases secondary signaling pathway and the immune system pathway in human disease were found to be enriched. Moreover, after LEfSe analysis, 22 tertiary signaling pathways (LDA Score ≥ 2) (Figure 6E) were found, including non-alcoholic fatty liver disease (ko04932), MAPK (ko04016), and glycerophospholipid metabolism (ko00564), as well as the neural system degeneration (ko05010 and ko05012) (Figure 6F).
Non-targeted metabolomics was performed to observe how valine modulated intestinal metabolism. PCA did not separate samples into two different groups (Supplementary Figures S5A,D), but after the PLS-DA analysis, for both positive and negative modes, the 0 V and 0.45 V groups were clearly separated (Supplementary Figures S5B,E), and the model for the relationship between the metabolite and sample class was not over-fit (R2 > Q2, bias <0) (Supplementary Figures S5C,F). After differential analysis (criteria: VIP > 1.0 and FC > 1.2, or FC < 0.833 and p < 0.05), 87 metabolites (20 up- and 67 down-regulated) were identified (Figures 7A,C and Supplementary Figure S6). Enrichment analysis based on differential metabolites showed that multiple pathways, such as protein digestion and absorption, and those related to lipid metabolism (steroid hormone biosynthesis, biosynthesis of unsaturated fatty acids, glycerophospholipid metabolism, and primary bile acid biosynthesis) were affected (Figures 7B,D).
Figure 7. Gut metabolome and integrative analysis. (A,C) Volcano plots of differential metabolites (positive and negative modes); (B,D) enriched signaling pathways. Integrative analysis: (E,F) correlation between significant metabolites and microbes (positive and negative modes). Blue and red colors indicate negative and positive correlations, respectively. *p < 0.05.
Further combined metagenome and metabolome analysis showed the correlation between microbes (top 10 genera) and differential metabolites (positive and negative) (top 20, starting from the largest |log2(FoldChange)|), respectively (Figures 7E,F). Interestingly, there existed similar strong correlation patterns between two metabolites (17-AAG and tauroursodeoxycholic acid) and different microbes (Figure 7F).
Combined RNAseq, metagenomics, and metabolomics analysis highlighted the fact that inflammation and lipid metabolism pathways in the small intestine and metabolic tissues could be affected by valine supplementation. The intestinal microbial metabolite, stercobilin, previously reported to be associated with chronic inflammation (40), was significantly altered by valine treatment. Therefore, we assayed its level and that of another metabolite (3-IAA) by ELISA. In duodenum and stool, stercobilin was significantly inhibited and elevated by valine treatment (0.45 V), respectively (p < 0.05) (Figure 8A). Stercobilin content in stool was consistent with the metabolomics result. In the ileum, stercobilin was also enhanced, though not significantly different. However, 3-IAA was not significantly different (Figure 8B).
Figure 8. Validation of metabolites and the inflammation pathway. Two metabolites: (A) stercobilin; (B) 3-IAA. Expression profiling on genes related to inflammation and gap junction: (C) liver; (D) duodenum; (E) jejunum; (F) ileum. *p < 0.05, **p < 0.01, ***p < 0.001.
In addition, expression levels of inflammation-related genes were evaluated in the liver. However, no significant difference was found (Figure 8C). Furthermore, we profiled the expression levels of genes related to neutral amino acid transferring [solute carrier family 6 (neurotransmitter transporter), member 19 (Slc6a19)], inflammation, and gap junction in the small intestine (the duodenum, the jejunum, and the ileum). With 0.45% valine supplementation, inflammation genes were increased significantly in the duodenum [interleukin 6 (Il-6), p < 0.05; interleukin 1β (Il-1β), p < 0.01] (Figure 8D). However, in the jejunum, they were inhibited significantly [Il-6, Il-1β, and nitric oxide synthase 2, inducible (Inos) was, p < 0.05] (Figure 8E), and especially in the ileum, which is the most significantly inhibited [tumor necrosis factor α (Tnf-α), p < 0.01; Il-6, p < 0.001; Il-1β, p < 0.05 and Inos, p < 0.01] (Figure 8F).
Furthermore, gap junction genes, tight junction protein 1 (Zo-1) and Occludin, were enhanced in the duodenum and ileum (p < 0.01) of the 0.45 V group, respectively (Figures 8D,F). Expression of Slc6a19 only increased in the ileum (p < 0.05) of the 0.45 V group (Figure 8F).
As each member of BCAAs is found to play either a beneficial or detrimental role to host metabolism (15–22), in the current study, we aimed to examine the specific role of valine in metabolic health. Here, by a multi-Omics approach, we showed that valine can disturb the cecal microbiota and metabolite compositions, with Bacteroidetes decreased, but Proteobacteria and Helicobacter increased. The inflammation in the duodenum was induced, and lipid metabolism in hepatic and adipose tissues was also enhanced.
Originally, valine was discovered to be related to BAT thermogenesis and affected glucose intake and IR of metabolic diseases (19, 20). The metabolite product of valine, 3-HIB, was elevated and considered a circulating biomarker in obese and diabetic patients (12, 14). We found that reduced insulin sensitivity was incurred, and hepatic TG and TC levels were found to be increased by 0.30% post valine treatment. A recent study also showed that in pig intestinal epithelial cells (IPEC-J2), valine and 3-HIB promoted fatty acid transport and enhanced TG synthesis, lipid droplet formation, and unsaturated fatty acid concentration (41). We further demonstrated that hepatic de novo lipogenesis and adipogenesis in WAT were increased, and β-oxidation in WAT and BAT was inhibited by valine. Thus, valine and 3-HIB could be directly involved in the enhancement of fatty acid and lipid metabolism.
The influence of BCAAs on individual metabolism is closely related to the gut microbiome. Studies have shown that the gut microbiome in insulin-resistant individuals has more Prevotella copri and Bacteroides vulgatus species, with the tendency to synthesize more BCAAs (28, 42, 43). P. copri was demonstrated to be capable of inducing IR, aggravating glucose intolerance and augmenting circulating levels of BCAAs (28). In addition, BCAA catabolism in BAT is important for alleviating IR (19, 20). Feeding Bacteroides dorei and vulgatus to mice improved BAT BCAA catabolism, decreased circulating BCAA levels and body weight gain (44). Moreover, Bacteroides could be used as probiotics for treating aberrant metabolism, which is currently under active research (44–46). We found here that Bacteroides decreased in cecum, which could be related to the detrimental effect of valine on lipid metabolism in hepatic and adipose tissues.
Further assessment revealed that valine increased inflammation and reduced crypt depth in the duodenum. On the contrary, it seems that valine could be absorbed mainly and reduce inflammation in the ileum, as indicated by the local high expression levels of Slc6a19 and reduced expression of inflammation-related genes (Tnf-α and interleukins) (40). Moreover, the structural integrity of the small intestine and systemic inflammation are reported to be linked to IR (40, 43). Adipose tissue transcriptome sequencing also indicated the existence of adipose inflammation. Signaling pathways related to lipid metabolism and adipogenesis were also found to be disturbed by valine supplementation, including bile acid and circadian rhythm. Interestingly, the core circadian clock member Per1 regulates directly the phosphorylation of bile acid synthases through PKA and, in turn, affects fat absorption and accumulation (47). Per1 also interacts with GPX1 to mediate mitochondrial function and metabolic homeostasis in response to the oxidation process (48). Moreover, intestinal metabolic dysregulation and hepatic inflammation could be induced by disrupted circadian rhythmic stasis (49). It is worthwhile to investigate further how valine affects the molecular clock and metabolic health.
Microbes directly digest, absorb, and metabolize food nutrients and produce metabolites to affect inter-organ and holistic metabolism (50–54). Among them, stercobilin is the metabolite directly involved in bile acid metabolism and associated with chronic inflammation of obese mice (40). Here, valine induced the increased stercobilin level in cecal content, which could contribute to inflammation and enhanced lipid metabolism, as observed in small intestine and adipose tissues. The diet, metabolite, and microbiota axis is of functional importance to human health, as also shown previously by a multi-omic analysis (53). Furthermore, in the present study, valine was supplemented via drinking water. Nowadays, functional beverages, containing different bioactive substances (for instance, BCAAs and vitamins in sports drinks), are consumed daily by humans (55, 56). However, valine can have adverse effects on metabolic health as found here and reproductive function as reported previously (23), serving as a caution to its use in functional drinks. Certainly, our study is limited, and a detailed investigation is warranted to understand the exact role and effect of valine on human metabolic health.
Taken together, the multi-Omics analysis revealed that valine treatment enhances hepatic lipid metabolism and adipogenesis, by affecting the intestinal microbiota and inflammation in gut and adipose tissues. Our findings provide novel insights and resources for further investigation on the molecular mechanism and biological function of valine on lipid metabolism.
The data presented in the study are deposited in the NCBI repository, accession number SRR27778108–SRR27778119, SRR28172663–SRR28172668.
The animal study was approved by Animal Research Committee guidelines of Yangtze University. The study was conducted in accordance with the local legislation and institutional requirements.
H-YZ: Data curation, Formal analysis, Methodology, Validation, Writing – original draft. LW: Data curation, Formal analysis, Methodology, Validation, Writing – original draft. RZ: Software, Writing – original draft. RD: Methodology, Writing – original draft. C-XY: Funding acquisition, Methodology, Project administration, Supervision, Writing – original draft, Writing – review & editing. Z-QD: Funding acquisition, Methodology, Project administration, Supervision, Writing – original draft, Writing – review & editing.
The author(s) declare that financial support was received for the research, authorship, and/or publication of this article. We appreciate the help from other laboratory members and funding support from the Joint Research on Improved Livestock and Poultry Breeds in Anhui Province (2021-2025) and the Startup grants from Yangtze University.
The authors declare that the research was conducted in the absence of any commercial or financial relationships that could be construed as a potential conflict of interest.
All claims expressed in this article are solely those of the authors and do not necessarily represent those of their affiliated organizations, or those of the publisher, the editors and the reviewers. Any product that may be evaluated in this article, or claim that may be made by its manufacturer, is not guaranteed or endorsed by the publisher.
The Supplementary material for this article can be found online at: https://www.frontiersin.org/articles/10.3389/fnut.2024.1379390/full#supplementary-material.
Valine treatment on mice growth and metabolism. (A) Body weights; (B) weight gains; (C) feed intake; (D) water intake; (E) glucose tolerance test (GTT); (F) pyruvate tolerance test (PTT); (G) insulin tolerance test (ITT). *P < 0.05, **P < 0.01.
Transcriptome sequencing on adipose tissues. Transcriptional dynamics of genes for (A) White adipose tissue (WAT); (B) brown adipose tissue (BAT); PCA sample clustering for (C) WAT; (D) BAT.
Venn diagram of common differentially expressed genes (DEGs) for WAT and BAT.
Cecal metagenome sequencing. (A) Principal co-ordinates analysis (PCoA); (B) Venn diagram.
Non-targeted metabolomics. For the positive and negative modes, (A,D) PCA clustering; (B,E) PLS-DA analysis; (C,F) The fitted model parameters.
Metabolites significantly different. (A) Positive mode; (B) negative mode.
1. ^http://www.ensembl.org/index.html
2. ^https://www.genome.jp/kegg/pathway.html
1. Tremblay, F, Lavigne, C, Jacques, H, and Marette, A. Role of dietary proteins and amino acids in the pathogenesis of insulin resistance. Annu Rev Nutr. (2007) 27:293–310. doi: 10.1146/annurev.nutr.25.050304.092545
2. Bloomgarden, Z . Diabetes and branched-chain amino acids: what is the link? J Diabetes. (2018) 10:350–2. doi: 10.1111/1753-0407.12645
3. White, PJ, McGarrah, RW, Herman, MA, Bain, JR, Shah, SH, and Newgard, CB. Insulin action, type 2 diabetes, and branched-chain amino acids: a two-way street. Mol Metab. (2021) 52:101261. doi: 10.1016/j.molmet.2021.101261
4. Holeček, M . Role of impaired glycolysis in perturbations of amino acid metabolism in diabetes mellitus. Int J Mol Sci. (2023) 24:1724. doi: 10.3390/ijms24021724
5. Tanase, DM, Gosav, EM, Botoc, T, Floria, M, Tarniceriu, CC, Maranduca, MA, et al. Depiction of branched-chain amino acids (BCAAs) in diabetes with a focus on diabetic microvascular complications. J Clin Med. (2023) 12:6053. doi: 10.3390/jcm12186053
6. Newgard, CB . Interplay between lipids and branched-chain amino acids in development of insulin resistance. Cell Metab. (2012) 15:606–14. doi: 10.1016/j.cmet.2012.01.024
7. Fontana, L, Cummings, NE, Arriola Apelo, SI, Neuman, JC, Kasza, I, Schmidt, BA, et al. Decreased consumption of branched-chain amino acids improves metabolic health. Cell Rep. (2016) 16:520–30. doi: 10.1016/j.celrep.2016.05.092
8. Cummings, NE, Williams, EM, Kasza, I, Konon, EN, Schaid, MD, Schmidt, BA, et al. Restoration of metabolic health by decreased consumption of branched-chain amino acids. J Physiol. (2018) 596:623–45. doi: 10.1113/JP275075
9. Green, CL, Lamming, DW, and Fontana, L. Molecular mechanisms of dietary restriction promoting health and longevity. Nat Rev Mol Cell Biol. (2022) 23:56–73. doi: 10.1038/s41580-021-00411-4
10. Yao, H, Li, K, Wei, J, Lin, Y, and Liu, Y. The contradictory role of branched-chain amino acids in lifespan and insulin resistance. Front Nutr. (2023) 10:1189982. doi: 10.3389/fnut.2023.1189982
11. van Dijk, AM, Bruins Slot, AS, Portincasa, P, Siegerink, SN, Chargi, N, Verstraete, CJR, et al. Systematic review with meta-analysis: branched-chain amino acid supplementation in liver disease. Eur J Clin Investig. (2023) 53:e13909. doi: 10.1111/eci.13909
12. Yoneshiro, T, Wang, Q, Tajima, K, Matsushita, M, Maki, H, Igarashi, K, et al. BCAA catabolism in brown fat controls energy homeostasis through SLC25A44. Nature. (2019) 572:614–9. doi: 10.1038/s41586-019-1503-x
13. Ferguson, D, Eichler, SJ, Yiew, NKH, Colca, JR, Cho, K, Patti, GJ, et al. Mitochondrial pyruvate carrier inhibition initiates metabolic crosstalk to stimulate branched chain amino acid catabolism. Mol Metab. (2023) 70:101694. doi: 10.1016/j.molmet.2023.101694
14. Jersin, RÅ, Sri Priyanka Tallapragada, D, Skartveit, L, Bjune, MS, Muniandy, M, Lee-Ødegård, S, et al. Impaired adipocyte SLC7A10 promotes lipid storage in association with insulin resistance and altered BCAA metabolism. J Clin Endocrinol Metab. (2023) 108:2217–29. doi: 10.1210/clinem/dgad148
15. Ma, Q, Zhou, X, Hu, L, Chen, J, Zhu, J, and Shan, A. Leucine and isoleucine have similar effects on reducing lipid accumulation, improving insulin sensitivity and increasing the browning of WAT in high-fat diet-induced obese mice. Food Funct. (2020) 11:2279–90. doi: 10.1039/c9fo03084k
16. Zhou, X, Chen, J, Sun, B, Wang, Z, Zhu, J, Yue, Z, et al. Leucine, but not isoleucine or valine, affects serum lipid profiles and browning of WAT in mice. Food Funct. (2021) 12:6712–24. doi: 10.1039/d1fo00341k
17. Yu, D, Richardson, NE, Green, CL, Spicer, AB, Murphy, ME, Flores, V, et al. The adverse metabolic effects of branched-chain amino acids are mediated by isoleucine and valine. Cell Metab. (2021) 33:905–22.e6. doi: 10.1016/j.cmet.2021.03.025
18. Green, CL, Trautman, ME, Chaiyakul, K, Jain, R, Alam, YH, Babygirija, R, et al. Dietary restriction of isoleucine increases healthspan and lifespan of genetically heterogeneous mice. Cell Metab. (2023) 35:1976–95.e6. doi: 10.1016/j.cmet.2023.10.005
19. Bishop, CA, Schulze, MB, Klaus, S, and Weitkunat, K. The branched-chain amino acids valine and leucine have differential effects on hepatic lipid metabolism. FASEB J. (2020) 34:9727–39. doi: 10.1096/fj.202000195R
20. Bishop, CA, Machate, T, Henning, T, Henkel, J, Püschel, G, Weber, D, et al. Detrimental effects of branched-chain amino acids in glucose tolerance can be attributed to valine induced glucotoxicity in skeletal muscle. Nutr Diabetes. (2022) 12:20. doi: 10.1038/s41387-022-00200-8
21. Wang, C, Peng, Y, Zhang, Y, Xu, J, Jiang, S, Wang, L, et al. The biological functions and metabolic pathways of valine in swine. J Anim Sci Biotechnol. (2023) 14:135. doi: 10.1186/s40104-023-00927-z
22. Sun, Y, Sun, B, Wang, Z, Lv, Y, and Ma, Q. Short-term decreasing and increasing dietary BCAA have similar, but not identical effects on lipid and glucose metabolism in lean mice. Int J Mol Sci. (2023) 24:5401. doi: 10.3390/ijms24065401
23. Wu, ZW, Wang, L, Mou, Q, Wang, F, Wang, Y, Fang, T, et al. L-valine supplementation disturbs vital molecular pathways and induces apoptosis in mouse testes. Theriogenology. (2023) 215:31–42. doi: 10.1016/j.theriogenology
24. Cao, XK, Cheng, J, Huang, YZ, Wang, XG, Ma, YL, Peng, SJ, et al. Growth performance and meat quality evaluations in three-way cross cattle developed for the Tibetan plateau and their molecular understanding by integrative omics analysis. J Agric Food Chem. (2019) 67:541–50. doi: 10.1021/acs.jafc.8b05477
25. Meng, Y, Qiu, N, Guyonnet, V, and Mine, Y. Omics as a window to unravel the dynamic changes of egg components during chicken embryonic development. J Agric Food Chem. (2021) 69:12947–55. doi: 10.1021/acs.jafc.1c05883
26. Zhao, X, Jia, W, Wang, J, Wang, S, Zheng, Q, and Shan, T. Identification of a candidate gene regulating intramuscular fat content in pigs through the integrative analysis of transcriptomics and proteomics data. J Agric Food Chem. (2023) 71:19154–64. doi: 10.1021/acs.jafc.3c05806
27. Zhang, Y, Cheng, L, Liu, Y, Zhang, R, Wu, Z, Cheng, K, et al. Omics analyses of intestinal microbiota and hypothalamus clock genes in circadian disturbance model mice fed with green tea polyphenols. J Agric Food Chem. (2022) 70:1890–901. doi: 10.1021/acs.jafc.1c07594
28. Pedersen, HK, Gudmundsdottir, V, Nielsen, HB, Hyotylainen, T, Nielsen, T, Jensen, BA, et al. Human gut microbes impact host serum metabolome and insulin sensitivity. Nature. (2016) 535:376–81. doi: 10.1038/nature18646
29. Gojda, J, and Cahova, M. Gut microbiota as the link between elevated BCAA serum levels and insulin resistance. Biomol Ther. (2021) 11:1414. doi: 10.3390/biom11101414
30. Lee, J, Vijayakumar, A, White, PJ, Xu, Y, Ilkayeva, O, Lynch, CJ, et al. BCAA supplementation in mice with diet-induced obesity alters the metabolome without impairing glucose homeostasis. Endocrinology. (2021) 162:bqab062. doi: 10.1210/endocr/bqab062
31. Kim, WK, Singh, AK, Wang, J, and Applegate, T. Functional role of branched chain amino acids in poultry: a review. Poult Sci. (2022) 101:101715. doi: 10.1016/j.psj.2022.101715
32. Langdon, WB . Performance of genetic programming optimised Bowtie2 on genome comparison and analytic testing (GCAT) benchmarks. BioData Min. (2015) 8:1. doi: 10.1186/s13040-014-0034-0
33. Trapnell, C, Pachter, L, and Salzberg, SL. TopHat: discovering splice junctions with RNA-Seq. Bioinformatics. (2009) 25:1105–11. doi: 10.1093/bioinformatics/btp120
34. Trapnell, C, Roberts, A, Goff, L, Pertea, G, Kim, D, Kelley, DR, et al. Differential gene and transcript expression analysis of RNA-seq experiments with TopHat and cufflinks. Nat Protoc. (2012) 7:562–78. doi: 10.1038/nprot.2012.016
35. Huson, DH, Mitra, S, Ruscheweyh, HJ, Weber, N, and Schuster, SC. Integrative analysis of environmental sequences using MEGAN4. Genome Res. (2011) 21:1552–60. doi: 10.1101/gr.120618.111
36. Kanehisa, M, Goto, S, Sato, Y, Kawashima, M, Furumichi, M, and Tanabe, M. Data, information, knowledge and principle: back to metabolism in KEGG. Nucleic Acids Res. (2014) 42:D199–205. doi: 10.1093/nar/gkt1076
37. Powell, S, Forslund, K, Szklarczyk, D, Trachana, K, Roth, A, Huerta-Cepas, J, et al. eggNOG v4. 0: nested orthology inference across 3686 organisms. Nucleic Acids Res. (2014) 42:D231–9. doi: 10.1093/nar/gkt1253
38. Cantarel, BL, Coutinho, PM, Rancurel, C, Bernard, T, Lombard, V, and Henrissat, B. The carbohydrate-active EnZymes database (CAZy): an expert resource for Glycogenomics. Nucleic Acids Res. (2009) 37:D233–8. doi: 10.1093/nar/gkn663
39. Wen, B, Mei, Z, Zeng, C, and Liu, S. metaX: a flexible and comprehensive software for processing metabolomics data. BMC Bioinformat. (2017) 18:183. doi: 10.1186/s12859-017-1579-y
40. Sanada, S, Suzuki, T, Nagata, A, Hashidume, T, Yoshikawa, Y, and Miyoshi, N. Intestinal microbial metabolite stercobilin involvement in the chronic inflammation of Ob/Ob mice. Sci Rep. (2020) 10:6479. doi: 10.1038/s41598-020-63627-y
41. Xu, M, Che, L, Niu, L, Wang, L, Li, M, Jiang, D, et al. Molecular mechanism of valine and its metabolite in improving triglyceride synthesis of porcine intestinal epithelial cells. Sci Rep. (2023) 13:2933. doi: 10.1038/s41598-023-30036-w
42. Palmas, V, Pisanu, S, Madau, V, Casula, E, Deledda, A, Cusano, R, et al. Gut microbiota markers associated with obesity and overweight in Italian adults. Sci Rep. (2021) 11:5532. doi: 10.1038/s41598-021-84928-w
43. Sun, Y, Nie, Q, Zhang, S, He, H, Zuo, S, Chen, C, et al. Parabacteroides distasonis ameliorates insulin resistance via activation of intestinal GPR109a. Nat Commun. (2023) 14:7740. doi: 10.1038/s41467-023-43622-3
44. Yoshida, N, Yamashita, T, Osone, T, Hosooka, T, Shinohara, M, Kitahama, S, et al. Bacteroides spp. promotes branched-chain amino acid catabolism in brown fat and inhibits obesity. iScience. (2021) 24:103342. doi: 10.1016/j.isci.2021.103342
45. Chu, C, Yu, L, Li, Y, Guo, H, Zhai, Q, Chen, W, et al. Lactobacillus plantarum CCFM405 against rotenone-induced Parkinson's disease mice via regulating gut microbiota and branched-chain amino acids biosynthesis. Nutrients. (2023) 15:1737. doi: 10.3390/nu15071737
46. Song, H, Xue, H, Zhang, Z, Wang, J, Li, A, Zhang, J, et al. Amelioration of type 2 diabetes using four strains of Lactobacillus probiotics: effects on gut microbiota reconstitution-mediated regulation of glucose homeostasis, inflammation, and oxidative stress in mice. J Agric Food Chem. (2023) 71:20801–14. doi: 10.1021/acs.jafc.3c04665
47. Ge, W, Sun, Q, Yang, Y, Ding, Z, Liu, J, and Zhang, J. Circadian PER1 controls daily fat absorption with the regulation of PER1-PKA on phosphorylation of bile acid synthetase. J Lipid Res. (2023) 64:100390. doi: 10.1016/j.jlr.2023.100390
48. Sun, Q, Yang, Y, Wang, Z, Yang, X, Gao, Y, Zhao, Y, et al. PER1 interaction with GPX1 regulates metabolic homeostasis under oxidative stress. Redox Biol. (2020) 37:101694. doi: 10.1016/j.redox.2020.101694
49. Zhen, Y, Xi, Z, Hu, L, Chen, Y, Ge, L, Wei, W, et al. Impacts of circadian gene Period2 knockout on intestinal metabolism and hepatic antioxidant and inflammation state in mice. Oxidative Med Cell Longev. (2022) 2022:7896371–19. doi: 10.1155/2022/7896371
50. Van Hul, M, and Cani, PD. The gut microbiota in obesity and weight management: microbes as friends or foe? Nat Rev Endocrinol. (2023) 19:258–71. doi: 10.1038/s41574-022-00794-0
51. Ganesan, R, Gupta, H, Jeong, JJ, Sharma, SP, Won, SM, Oh, KK, et al. Characteristics of microbiome-derived metabolomics according to the progression of alcoholic liver disease. Hepatol Int. (2023) 18:486–99. doi: 10.1007/s12072-023-10518-9
52. Xourafa, G, Korbmacher, M, and Roden, M. Inter-organ crosstalk during development and progression of type 2 diabetes mellitus. Nat Rev Endocrinol. (2024) 20:27–49. doi: 10.1038/s41574-023-00898-1
53. Palacios-González, B, Ramírez-Salazar, EG, Rivera-Paredez, B, Quiterio, M, Flores, YN, Macias-Kauffer, L, et al. A multi-omic analysis for low bone mineral density in postmenopausal women suggests a RELATIONSHIP between diet, metabolites, and microbiota. Microorganisms. (2020) 8:1630. doi: 10.3390/microorganisms8111630
54. Moran-Ramos, S, Ocampo-Medina, E, Gutierrez-Aguilar, R, Macías-Kauffer, L, Villamil-Ramírez, H, López-Contreras, BE, et al. An amino acid signature associated with obesity predicts 2-year risk of hypertriglyceridemia in school-age children. Sci Rep. (2017) 7:5607. doi: 10.1038/s41598-017-05765-4
55. Martinho, DV, Nobari, H, Faria, A, Field, A, Duarte, D, and Sarmento, H. Oral branched-chain amino acids supplementation in athletes: a systematic review. Nutrients. (2022) 14:4002. doi: 10.3390/nu14194002
Keywords: adipose tissue, metabolite, metagenome, small intestine, valine
Citation: Zheng H-Y, Wang L, Zhang R, Ding R, Yang C-X and Du Z-Q (2024) Valine induces inflammation and enhanced adipogenesis in lean mice by multi-omics analysis. Front. Nutr. 11:1379390. doi: 10.3389/fnut.2024.1379390
Received: 31 January 2024; Accepted: 12 April 2024;
Published: 13 May 2024.
Edited by:
Arpita Mukhopadhyay, St. John's Research Institute, IndiaReviewed by:
Ornella Cominetti, Nestlé Research Center, SwitzerlandCopyright © 2024 Zheng, Wang, Zhang, Ding, Yang and Du. This is an open-access article distributed under the terms of the Creative Commons Attribution License (CC BY). The use, distribution or reproduction in other forums is permitted, provided the original author(s) and the copyright owner(s) are credited and that the original publication in this journal is cited, in accordance with accepted academic practice. No use, distribution or reproduction is permitted which does not comply with these terms.
*Correspondence: Cai-Xia Yang, Y2FpeGlheWFuZ0B5YW5ndHpldS5lZHUuY24=; Zhi-Qiang Du, emhxZHVAeWFuZ3R6ZXUuZWR1LmNu
†These authors have contributed equally to this work and share first authorship
Disclaimer: All claims expressed in this article are solely those of the authors and do not necessarily represent those of their affiliated organizations, or those of the publisher, the editors and the reviewers. Any product that may be evaluated in this article or claim that may be made by its manufacturer is not guaranteed or endorsed by the publisher.
Research integrity at Frontiers
Learn more about the work of our research integrity team to safeguard the quality of each article we publish.