- 1Arthritis Clinic and Research Center, Peking University People’s Hospital, Peking University, Beijing, China
- 2School of Biomedical Engineering, Faculty of Engineering and IT, University of Technology Sydney, Sydney, NSW, Australia
Background: To understand the impact of individual preferences for specific dietary items on OA, and to help inform the development of effective and targeted OA prevention and management strategies, we performed a Mendelian randomization analysis between dietary preferences and osteoarthritis.
Methods: This study utilized genetic data from the UK Biobank to investigate the association between OA and 21 different common dietary items. Instrumental variables representing European populations were carefully selected based on their genetic significance and linkage disequilibrium. In cases where a dietary item had few relevant genetic markers, a more lenient selection threshold was applied. To prevent bias, the analysis excluded single nucleotide polymorphisms (SNPs) associated with factors such as body mass index (BMI) and cholesterol. Using inverse-variance weighting (IVW) and Mendelian randomization, significant associations were detected between certain dietary items and OA.
Results: Using Mendelian randomization to examine the relationship between 21 different dietary items and OA, significant associations were found for coffee, peas, watercress, and cheese, where the first two had a promoting effect and the last two an inhibiting effect on OA. Due to heterogeneity in the test results for cheese, a random IVW representation was used. The results of sensitivity analysis showed no significant heterogeneity or horizontal pleiotropy in the selected SNPS, demonstrating the reliability of Mendelian randomization analysis.
Conclusion: This study identified coffee, peas, watercress, and cheese as food items that may have significant dietary effects on osteoarthritis. This information may be useful to consider in the development of OA management strategies.
1 Introduction
Osteoarthritis (OA) is a prevalent joint disorder characterized by clinical symptoms such as chronic pain, crepitus, joint stiffness, and structural changes including radiographic alterations and joint-wide tissue degradation (1). The socioeconomic influences of OA are globally recognized as imposing a staggering long-term burden on both individuals and healthcare systems due to the absence of a curative treatment and need for chronic management (2, 3). The pathogenesis of OA is complex, involving a variety of genetic, lifestyle, and other factors (4), and is not fully understood. Certain lifestyle and health factors are found to be definitive risk factors for OA, such as excessive physical activity, alcohol consumption, smoking, type 2 diabetes, and obesity (5). Current studies have outlined a role of diet on OA progression, although mostly centered on the association between diet and obesity and consequent effects on OA (6, 7), or specific types of diet (8).
Research into the effects of diet on OA has yielded interesting, sometimes conflicting results (6, 9–11). There is good evidence to suggest that certain dietary components such as dietary fiber, lipids, and vitamins have a significant impact on OA progression (12–14). These nutrients may influence key molecular pathways implicated in OA pathogenesis, by changing serum lipid concentrations, expression of inflammatory biomarkers, oxidative stress responses, and the activity of matrix metalloproteinases, among other mechanisms (12, 15). There is a gap in research regarding the comprehensive impact of specific foods on osteoarthritis. This study aims to explore the association between specific food items, including coffee, tea, fruits, dairies, meats, vegetables, and nuts, and osteoarthritis using genetic data from the UK Biobank.
The UK Biobank is an invaluable resource for biomedical research, providing a vast repository of detailed genetic, lifestyle, and health information from around 500,000 participants in the UK (16). This allows multifaceted analysis particularly regarding the associations and risk factors of common health problems such as OA. Of particular significance, the database contains genome-wide association analysis data for various dietary intake preferences. The IEU open GWAS database is a comprehensive platform that integrates genome-wide association study data from various sources. The data for our GWAS study on diet were sourced from the summary data of the most recent UK Biobank GWAS available in the IEU Open GWAS database. Mendelian randomization (MR) is an analytical method that conducts causal inferences by using ancestral genetic variations as instrumental variables for exposures (such as dietary preference for milk) and outcomes (such as OA) (17). It takes advantage of the fact that single nucleotide polymorphisms (SNPs) are randomly allocated at conception, avoiding issues of reverse causality and reducing residual confounding. Mendelian randomization is grounded on three key assumptions: (i) Correlation assumption: A robust correlation exists between SNP and exposure factors (ii) Independence assumption: SNP and confounding factors are independent (iii) Exclusion hypothesis: SNP can solely impact the outcome through exposure factors. In this study, we conducted a two-sample Mendelian analysis to investigate the causal relationship between specific dietary preferences and OA. We selected representative food items as exposures and used OA as the outcome. This approach should allow an accurate assessment of the impact of dietary choices on OA risk. The study findings may enhance the current understanding of how common food items affect OA, and inform the development of more effective dietary strategies for disease prevention and its chronic management, particularly in predisposing populations such as those who are overweight or have had prior joint injury.
2 Materials and methods
2.1 Selection of instrumental variables and data source
The genetic variation in dietary intake was obtained from publicly available data from the UK Biobank cohort, consisting of approximately half a million people (16). From the original list of dietary items, we selected 21 by adaptation of published methods (18), namely coffee, tea, cheese, cereal, pork, fresh fruit, dried fruit, cooked vegetable, salad/raw vegetable, bread, peas, unsalted peanuts, salted peanuts, milk, yogurt, beef, unsalted nuts, salted nuts, lamb, Indian snacks, and watercress (Table 1). The foods we selected considered a wide range of common dietary categories including drinks, dairy products, meat, vegetables, fruits, nuts, snacks, and staple foods.
To select valid instrumental variables (IVs), we included SNPs that reached the genome-wide significant level (p < 5 × 10−8) and applied strict cutoff values (R2 < 0.01; region size = 5,000 kb) to remove SNPs in linkage disequilibrium. The threshold criteria we selected were based on previous studies (19, 20). For dietary items with less than 5 SNPs meeting the strict threshold (p < 5 × 10−8), namely peas, salted peanuts, milk, yogurt, unsalted nuts, salted nuts, lamb, Indian snacks, and watercress, we chose to use a relaxed threshold (p < 1 × 10−5; R2 < 0.01; region size = 5,000 kb) to select SNPs. Additionally, SNPs with a minimum allele frequency (MAF) below 0.05 were excluded due to their unstable association with dietary intake. To fulfill the second critical hypothesis, we evaluated the sub-phenotype of the selected SNPs using the PhenoScanner database (p < 1 × 10−5). We also excluded SNPs associated with body mass index (BMI) and cholesterol, as well as SNPs directly related to OA, to avoid violating the third critical hypothesis that the IVs should not directly relate to the outcome. Furthermore, we ruled out SNPs associated with multiple diets to reduce potential pleiotropy across the SNPs. The OA data came from a previous genome-wide association study (GWAS) (21).
2.2 Statistical analysis
This study utilized SNPs to represent the genetic prediction level of dietary intake and investigated their association with the risk of OA. The primary method used was the fixed-effects inverse-variance weighted (IVW) method, which combines Wald utilizes a fixed-effects meta-analysis model to integrate ratio estimates from multiple genetic variants, providing a comprehensive effect estimate of dietary influence on OA (19). By combining Wald estimates for each SNP through a meta-analysis, the IVW method generates an overarching assessment of the diet’s impact on OA. The IVW method can provide unbiased estimations if there is no horizontal pleiotropy imbalance (22).
The weighted median approach, for instance, allowed for the inclusion of half the weight from invalid genetic variants while providing a consistent point estimate. The MR-Egger method, based on the InSIDE hypothesis, which allows for the possibility of pleiotropy under certain conditions where it posits that the strength of the association between the genetic variants (used as IVs) and the exposure is independent of any direct effect these variants might have on the outcome, enables a valid test of the null associational hypothesis and a consistent estimation of associational effects even if all genetic variants are invalid IVs (18, 23).
2.3 Sensitivity analysis
In Mendelian Randomization (MR) analysis, conducting heterogeneity and pleiotropy tests is essential to ensure the validity and reliability of causal inferences derived from genetic variants used as instrumental variables. Heterogeneity in MR refers to significant variations in the effects of different genetic variants on the exposure variable, which may signal issues like inappropriate instrumental variables or unaccounted confounding factors. Heterogeneity tests aim to evaluate the consistency of genetic instruments in influencing the outcome, thereby enhancing the accuracy of MR analyses. On the other hand, pleiotropy occurs when a genetic variant impacts multiple traits, potentially influencing the outcome through pathways unrelated to the exposure of interest. Pleiotropy tests strive to detect and adjust for genetic variants that could bias results by affecting the outcome through multiple mechanisms. Together, these tests play a crucial role in minimizing bias, enhancing the precision and reliability of causal estimates in MR studies, and ultimately increasing confidence in the conclusions drawn regarding causal relationships.
Sensitivity analysis was conducted to explore various potential effects in the final model. In each analysis of association between dietary intake and OA, Cochran’s Q statistics were used to measure the heterogeneity between independent variables (24). If heterogeneity was detected (PCochran’s Q < 0.05), then the multiplicative random-effects IVW model was applied to avoid bias toward weaker instrument exposure associations. The MR-Egger intercept test was used to evaluate pleiotropy by comparing the intercept term to zero. A significant difference suggested the presence of horizontal pleiotropy between IVs. Additionally, forest plots, scatter plots, funnel plots, and leave-one-out analysis plots were used to visualize the results with high confidence.
Dietary items with statistically significant IVW results were selected for further study. The p-value of IVW results obtained from four items, namely coffee, cheese, peas, and watercress, was found to be less than 0.05. However, the p-value of the heterogeneity test for cheese was lower than 0.05. Therefore, we conducted Mendelian randomization using non-fixed model IVW analysis with IVs of cheese. The non-fixed model IVW considers the variability of instrumental variable effects, allowing for differences in these effects among various genetic mutations. This method typically results in broader confidence intervals that better represent the uncertainty in estimates, thus minimizing the potential for drawing misleading conclusions.
3 Results
3.1 Statistically significant dietary preferences
At the onset of the study, the instrumental variables for 21 dietary exposure factors were individually screened (Supplementary Table S1). MR analysis across 21 different food items in relation to OA indicated statistically significant associations for four dietary preferences, namely coffee, cheese, peas, and watercress. Significant differences were determined based on p-values less than 0.05 during data analysis. The inverse-variance weighted (IVW) p-values were found to be 0.004, 0.003, 0.045, and 0.008, respectively (Table 1). The 95% confidence interval for the ratio of coffee was (1.145, 2.079), for cheese was (0.605, 0.901), for peas was (1.003, 1.295), and for watercress was (0.510, 0.903). Coffee and peas were found to have a promoting effect on OA, while cheese and watercress were found to have an inhibiting effect (Figure 1). The results of Mendelian randomization analysis for other food intakes were displayed in the graph. However, the statistical analysis did not reveal a significant relationship between other food groups and osteoarthritis.
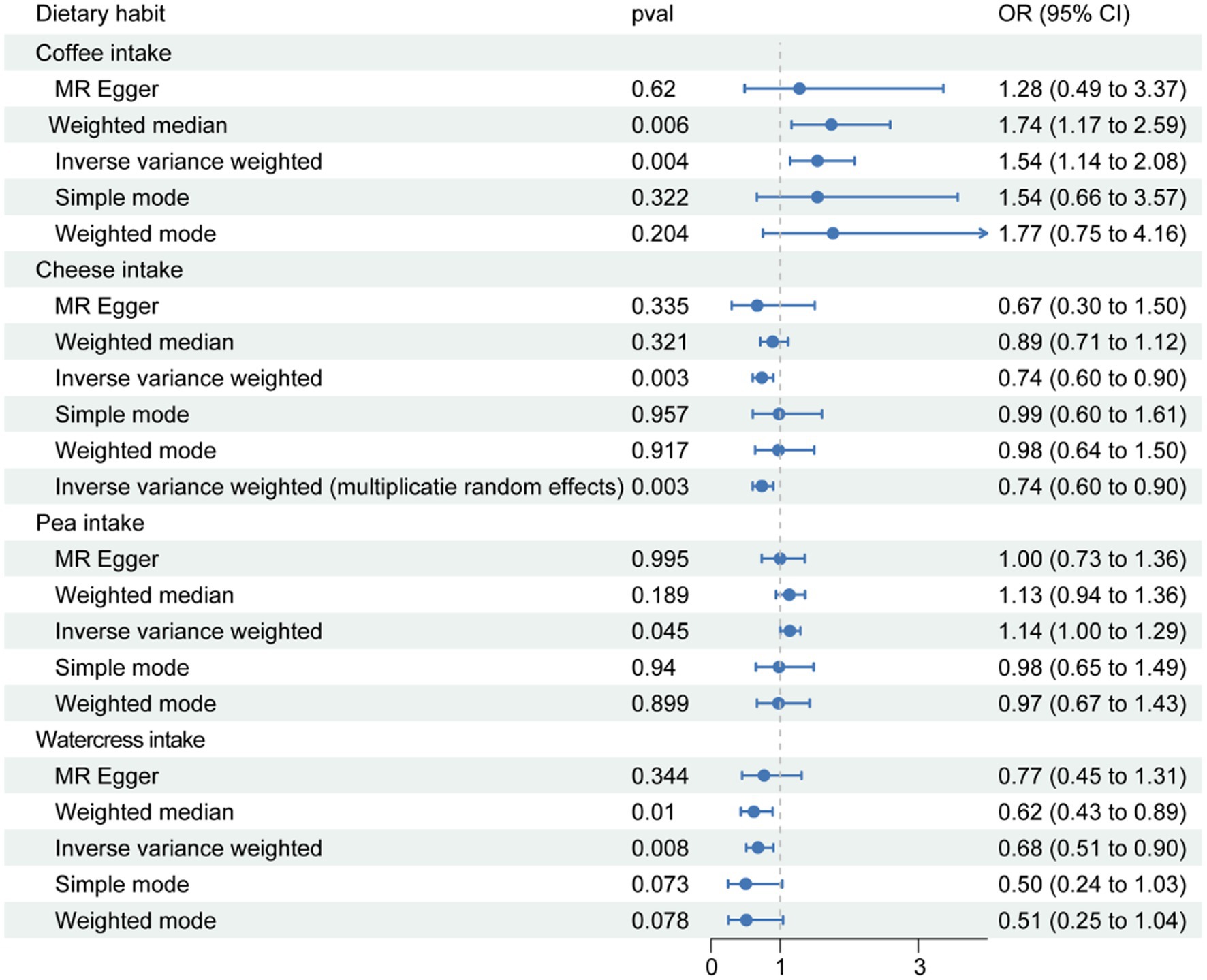
Figure 1. The results of Mendelian randomization analysis of statistically significant dietary preferences were screened, and the results were displayed using forest maps. The term ‘pval’ refers to the p-value obtained from the corresponding Mendelian randomization analysis method. The 95% Odds Ratio (OR) refers to the range of values within which we are 95% confident that the true OR lies, indicating the strength of association between a genetic variant and a health outcome.
3.2 Results of sensitivity analysis
The sensitivity analysis results of the 21 dietary items were tabulated (Table 2), showing the heterogeneity and pleiotropy test results of the chosen IVs. Our main focus was on the IVW method and its application to the four specific dietary items that showed significant associations with OA. Furthermore, the heterogeneity test for cheese yielded a p-value of 0.002, which was represented using random IVW.
The fitting results of different MR analyses were presented through scatter plots (Figure 2), while funnel plots were used to visually assess the heterogeneity of IVs (Figure 3). Figure 2 displayed the outcomes of an MR analysis investigating the association between exposure factors and outcome factors. The different colors of the lines corresponded to distinct algorithms utilized in the analysis. The results revealed a consistent pattern across the lines for four types of food intake examined by various algorithms, demonstrating a positive relationship between coffee and pea consumption and osteoarthritis. In contrast, cheese and watercress intake were associated with a negative impact on the condition. The funnel plot in Figure 3 illustrated the heterogeneity of the selected SNPs through individual black dots. The symmetrical distribution of these dots around the IVW method in the plot indicated the robustness of the chosen SNPs for our analysis. This symmetry implied that the selected SNPs offered a fair and impartial estimation of the relationship between exposure factors and the outcome, thereby strengthening the credibility of our findings within the realm of Mendelian Randomization studies.
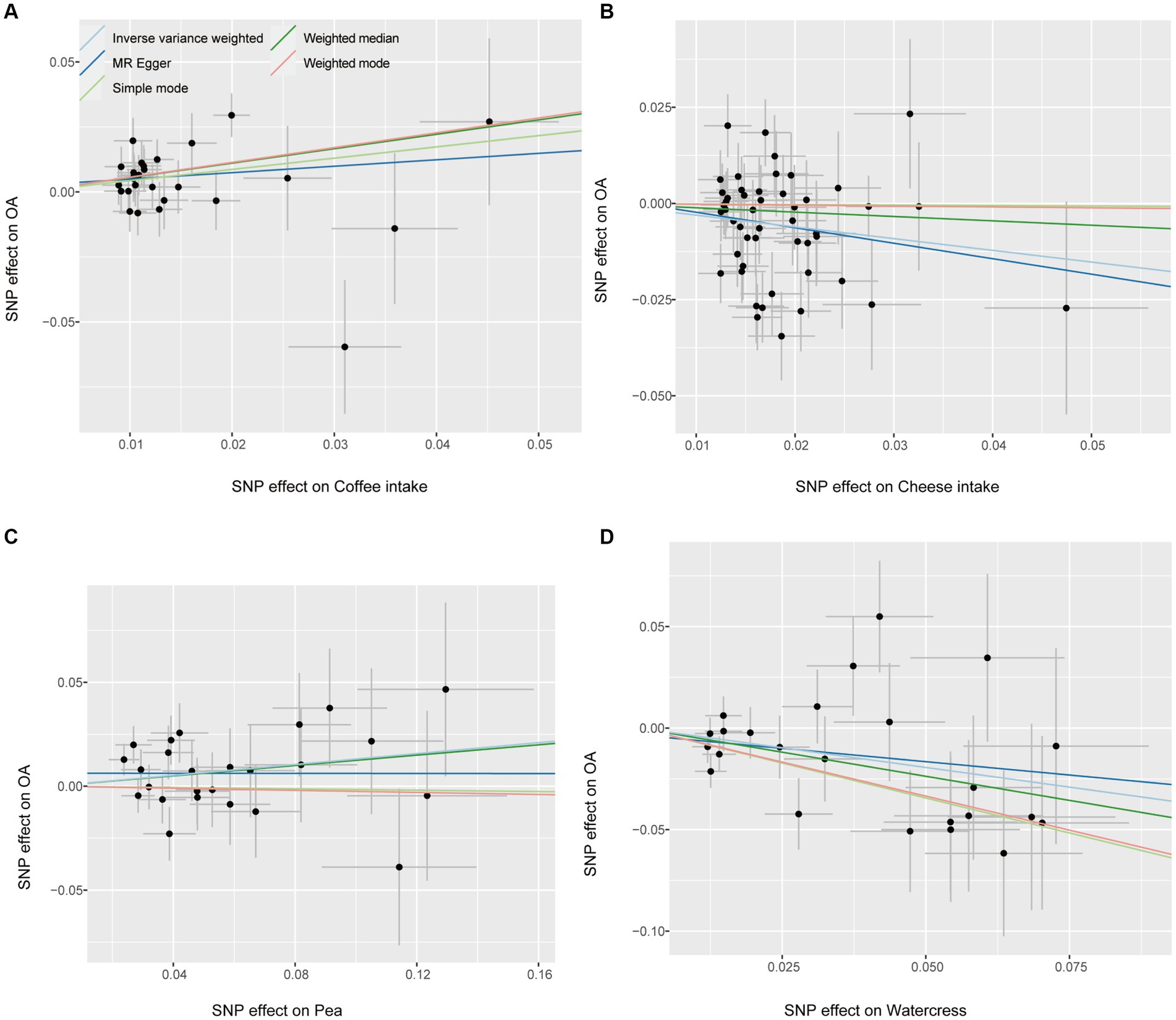
Figure 2. Scatter plot. Each point on the graph represents an IV, and the lines at each point represent the 95% confidence interval, with the horizontal coordinate showing the SNP effect on diet, the vertical coordinate showing the SNP effect on OA, and the colored lines showing the MR Fit. (A) Coffee intake (B) Cheese intake (C) Pea intake (D) Watercress intake.
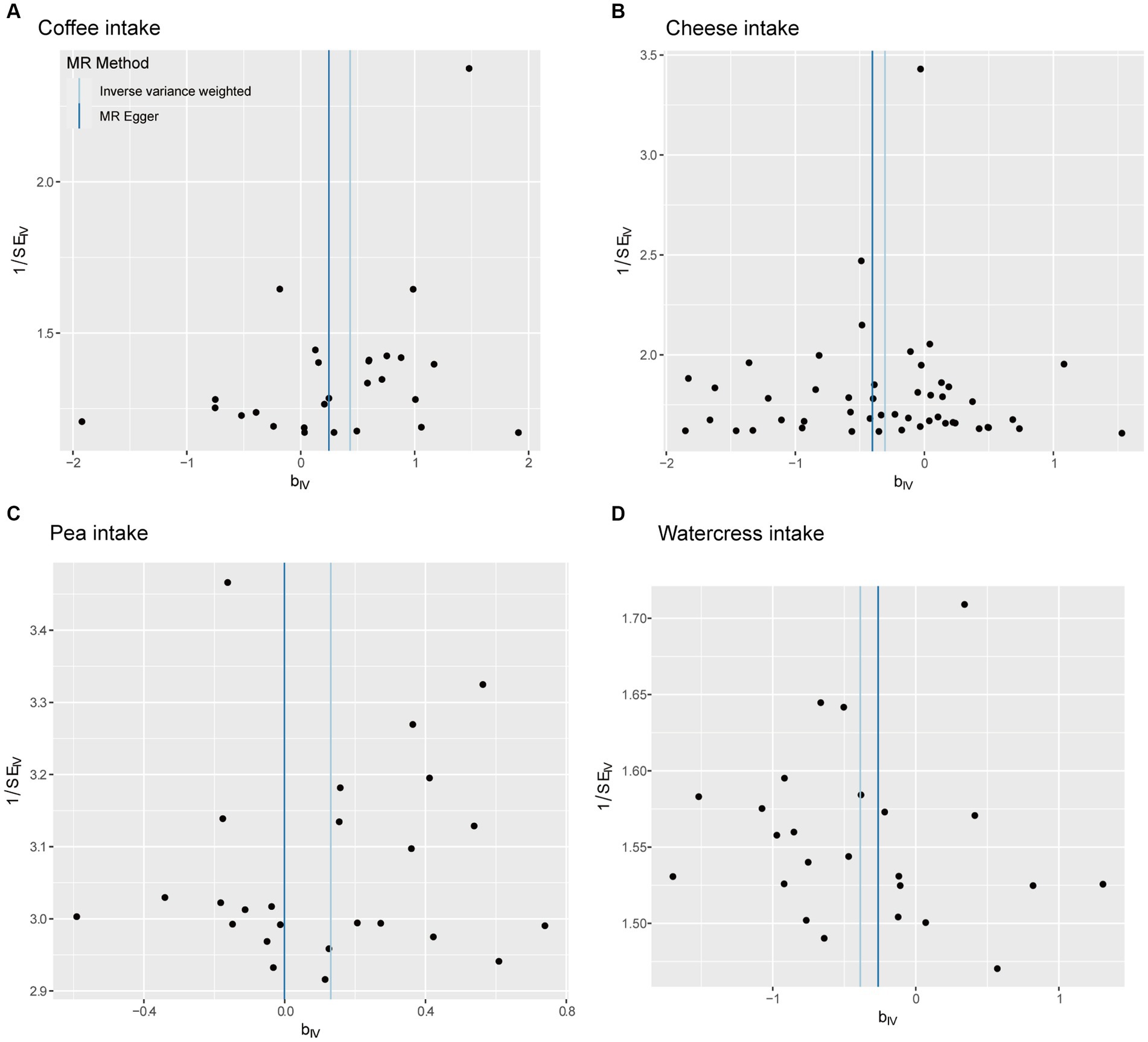
Figure 3. Funnel diagram. The points on the graph reflect the overall distribution of the selected IV. (A) Coffee intake, (B) cheese intake, (C) pea intake, (D) watercress intake.
To assess the robustness of these results, we performed sensitivity testing using the leave-one-out analysis (Figure 4). The leave-one-out plot revealed that the four IVs of dietary preference identified in IVW exhibited a relatively strong level of consistency. Additionally, the effect of each SNP variable aligned closely with the overall effect. Each point in the leave-one-out plot represents the total effect of other SNPs on osteoarthritis after excluding this particular SNP. Consistency in the impact of excluding each SNP suggested that the effects of the SNPs are relatively consistent. Our findings revealed that the overall effect remains relatively consistent even after excluding each SNP. The results suggested that each SNP related to food intake has an effect on osteoarthritis, with most effects showing consistency, thereby enhancing confidence in our research findings.
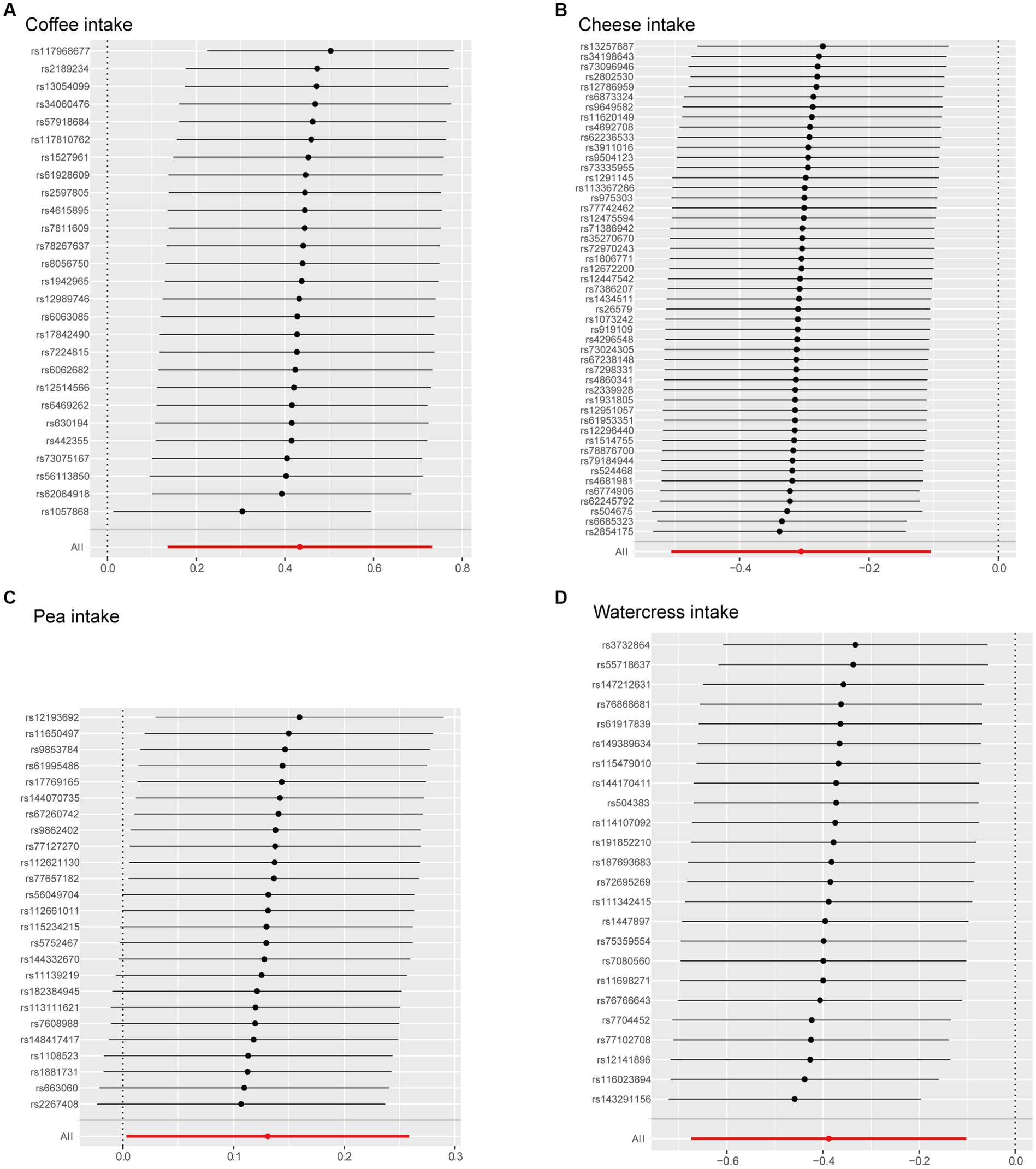
Figure 4. Leave-one-out plot. The Mendelian effect after removing every instrumental variable. (A) Coffee intake, (B) cheese intake, (C) pea intake, (D) watercress intake.
4 Discussion
Mendelian randomization provides a robust framework to differentiate between correlation and causation, offering more reliable insights compared to conventional observational studies. In this study, we used MR to analyze genetic variants associated with common dietary habits, and inferred the potential causal effects of these dietary items on OA. This research may help inform the development of dietary recommendations and preventive strategies for OA. Interestingly, our findings identified four dietary preferences which may impact OA, namely coffee, peas, watercress, and cheese, where the first two had a promoting effect and the last two an inhibiting effect.
Several studies have pointed to the relationship between coffee and OA (25–27). A cross-sectional study found that consuming more than 7 cups of coffee per day was linked to a higher risk of OA in men, and this risk increased with the amount of coffee consumption (26). A recent review also summarized experimental as well as clinical evidence on the negative effects of caffeine on hyaline cartilage, including its catabolic effects on articular and growth plate cartilage (28). Other studies have suggested an indirect effect of caffeine on the relationship between inflammatory factors and articular cartilage, with caffeine intake being associated with the expression of inflammatory cytokines IL-1 and TNF-α (29).
Pea consumption was found to contribute to OA in our study. Very limited research has explored this association in the literature, with only one study suggesting that alcoholic drinks produced using peas was an independent risk factor for OA (30). It should be noted however that this study set out to investigate the relation of alcohol and specific alcoholic drinks with OA risk, and that high consumption of alcohol itself (>14 standard drinks per week) was significantly associated with incident knee surgery due to OA (30). Further research is needed to determine whether there are specific components in peas that may trigger or enhance molecular pathways leading to OA progression.
Interestingly, our findings suggested an inhibitory effect of watercress on OA, which might be related to several mechanisms. Firstly, watercress contains high levels of beneficial compounds such as isothiocyanates, which have shown strong anti-inflammatory properties (31). This may help reduce inflammation in joint tissues and retard associated pro-inflammatory mechanisms contributing to OA development (32). Secondly, watercress is rich in antioxidants such as vitamin C and beta-carotene, which may counteract the harmful effects of free radicals (31), known to be implicated in the degradation of joint cartilage leading to OA progression (33). Furthermore, the nutrients present in watercress may help protect the integrity of cartilage, maintaining normal cartilage metabolism and inhibiting or slowing catabolic processes (34).
Among dairy products, cheese is naturally nutrient dense and provides high levels of compounds that help maintain bone density and strength, which can be beneficial in OA management especially given the bone weakening associated with OA (35) and the critical role of the subchondral bone in OA (36). Cheese provides a rich source of calcium and is often fortified with vitamin D, both of which are essential for maintaining bone health (37). Some cheeses, especially those made from the milk of grass-fed animals, contain conjugated linoleic acid (CLA), a fatty acid shown to possess anti-inflammatory properties (38). Other cheeses from grass-fed animals can have high levels of omega-3 fatty acids (39), also known for their potent anti-inflammatory properties (40). Both of these compounds may provide benefits in reducing the inflammation involved in the pathogenesis of joint diseases including OA.
In this study, we employed a dual-sample MR approach to investigate potential associations between four specific dietary items and the onset of OA. It is important to acknowledge that certain limitations may exist in our work. Firstly, the observational nature of our study may introduce potential biases, as the establishment of a causative relationship would require randomized controlled trials. Additionally, the genetic instruments used for MR may not fully capture the complexity of dietary influences on OA, considering its multifactorial nature as well as the wide-ranging effects of diet on other factors associated with OA such as exercise, obesity, and co-morbidities including cardiovascular disease (41). Secondly, our findings may have been influenced by unmeasured confounding factors. It only includes a range of genetic markers directly associated with the items investigated in this study. To gain an enhanced and more accurate understanding of the relationship between diet and OA, further studies incorporating a wider array of genetic markers and consideration of environmental factors are necessary, particularly given an emerging understanding of the role of epigenetics in OA progression (42). Ideally, these studies should involve diverse populations to comprehend the intricate interplay among genetics, diet, and lifestyle factors in the context of OA. Due to the utilization of summary GWAS data, the demographic information is restricted, with the population solely reflecting individuals of European descent. Consequently, the generalization of the research findings is constrained. In an investigation into the correlation between 21 dietary intakes and osteoarthritis, our study employed Mendelian randomization to evaluate causality, using p-values for statistical significance. Despite its innovative approach, this methodology encounters challenges when dealing with multiple comparisons, especially given the numerous dietary factors involved, which increases the risk of Type I errors. The absence of adjustments for these multiple comparisons represents a notable limitation of our study, underscoring the need for cautious interpretation of our results. Nevertheless, our findings offer valuable insights into the link between diet and osteoarthritis. Future research should consider incorporating correction methods like the Bonferroni or Benjamini-Hochberg procedures to improve result reliability and explore alternative statistical techniques, such as Bayesian approaches, to more precisely evaluate the genuine relationship between diet and osteoarthritis while addressing the issue of multiple comparisons. Moreover, relaxing the threshold for selecting SNPs related to certain dietary items due to a limited number of SNPs meeting the stringent genome-wide significance level may introduce bias and potentially impact the outcomes of our study. Lastly, it is important to note that while the potential mechanisms discussed in this study in relation to the identified food items with significant influence on OA are supported by preliminary research, further studies are necessary to fully understand the extent of the impact of these dietary preferences on OA.
Data availability statement
The original contributions presented in the study are included in the article/Supplementary material, further inquiries can be directed to the corresponding author.
Author contributions
LC: Formal analysis, Methodology, Writing – original draft, Writing – review & editing. YS: Formal analysis, Methodology, Writing – review & editing. HL: Methodology, Writing – review & editing. ZY: Methodology, Writing – review & editing. JL: Writing – review & editing. DX: Funding acquisition, Methodology, Supervision, Writing – review & editing.
Funding
The author(s) declare financial support was received for the research, authorship, and/or publication of this article. This work was funded by grants from Natural Science Foundation of Beijing Municipality (L222087) and Peking University People’s Hospital Scientific Research Develop- ment Funds (RDGS2023-04).
Acknowledgments
We express our gratitude to the UK Biobank public GWAS database and the providers of summary GWAS data on osteoarthritis that we utilized.
Conflict of interest
The authors declare that the research was conducted in the absence of any commercial or financial relationships that could be construed as a potential conflict of interest.
Publisher’s note
All claims expressed in this article are solely those of the authors and do not necessarily represent those of their affiliated organizations, or those of the publisher, the editors and the reviewers. Any product that may be evaluated in this article, or claim that may be made by its manufacturer, is not guaranteed or endorsed by the publisher.
Supplementary material
The Supplementary material for this article can be found online at: https://www.frontiersin.org/articles/10.3389/fnut.2024.1373850/full#supplementary-material
References
1. Abramoff, B, and Caldera, FE. Osteoarthritis: pathology, diagnosis, and treatment options. Med Clin North Am. (2020) 104:293–311. doi: 10.1016/j.mcna.2019.10.007
2. Martel-Pelletier, J, Barr, AJ, Cicuttini, FM, Conaghan, PG, Cooper, C, Goldring, MB, et al. Osteoarthritis. Nat Rev Dis Primers. (2016) 2:16072. doi: 10.1038/nrdp.2016.72
3. Litwic, A, Edwards, MH, Dennison, EM, and Cooper, C. Epidemiology and burden of osteoarthritis. Br Med Bull. (2013) 105:185–99. doi: 10.1093/bmb/lds038
4. Palazzo, C, Nguyen, C, Lefevre-Colau, M-M, Rannou, F, and Poiraudeau, S. Risk factors and burden of osteoarthritis. Ann Phys Rehabil Med. (2016) 59:134–8. doi: 10.1016/j.rehab.2016.01.006
5. Blümel, JE, Aedo, S, Arteaga, E, Vallejo, MS, and Chedraui, P. Risk factors for the development of osteoarthritis in middle-aged women. Rev Med Chile. (2022) 150:46–53. doi: 10.4067/S0034-98872022000100046
6. Liu, Q, Hebert, JR, Shivappa, N, Guo, J, Tao, K, Zeng, C, et al. Inflammatory potential of diet and risk of incident knee osteoarthritis: a prospective cohort study. Arthritis Res Ther. (2020) 22:209. doi: 10.1186/s13075-020-02302-z
7. Mooney, RA, Sampson, ER, Lerea, J, Rosier, RN, and Zuscik, MJ. High-fat diet accelerates progression of osteoarthritis after meniscal/ligamentous injury. Arthritis Res Ther. (2011) 13:R198. doi: 10.1186/ar3529
8. Morales-Ivorra, I, Romera-Baures, M, Roman-Viñas, B, and Serra-Majem, L. Osteoarthritis and the Mediterranean diet: a systematic review. Nutrients. (2018) 10:1030. doi: 10.3390/nu10081030
9. Thomas, S, Browne, H, Mobasheri, A, and Rayman, MP. What is the evidence for a role for diet and nutrition in osteoarthritis? Rheumatology (Oxford). (2018) 57:iv61–74. doi: 10.1093/rheumatology/key011
10. Mazzei, DR, Ademola, A, Abbott, JH, Sajobi, T, Hildebrand, K, and Marshall, DA. Are education, exercise and diet interventions a cost-effective treatment to manage hip and knee osteoarthritis? A systematic review. Osteoarthr Cartil. (2021) 29:456–70. doi: 10.1016/j.joca.2020.10.002
11. Ameye, LG, and Chee, WSS. Osteoarthritis and nutrition. From nutraceuticals to functional foods: a systematic review of the scientific evidence. Arthritis Res Ther. (2006) 8:R127. doi: 10.1186/ar2016
12. Gkretsi, V, Simopoulou, T, and Tsezou, A. Lipid metabolism and osteoarthritis: lessons from atherosclerosis. Prog Lipid Res. (2011) 50:133–40. doi: 10.1016/j.plipres.2010.11.001
13. Dai, Z, Niu, J, Zhang, Y, Jacques, P, and Felson, DT. Dietary intake of fibre and risk of knee osteoarthritis in two US prospective cohorts. Ann Rheum Dis. (2017) 76:1411–9. doi: 10.1136/annrheumdis-2016-210810
14. Chin, K-Y . The relationship between vitamin K and osteoarthritis: a review of current evidence. Nutrients. (2020) 12:1208. doi: 10.3390/nu12051208
15. Ansari, MY, Ahmad, N, and Haqqi, TM. Oxidative stress and inflammation in osteoarthritis pathogenesis: role of polyphenols. Biomed Pharmacother. (2020) 129:110452. doi: 10.1016/j.biopha.2020.110452
17. Bowden, J, and Holmes, MV. Meta-analysis and Mendelian randomization: a review. Res Synth Methods. (2019) 10:486–96. doi: 10.1002/jrsm.1346
18. Yan, H, Jin, X, Zhang, C, Zhu, C, He, Y, Du, X, et al. Associations between diet and incidence risk of lung cancer: a Mendelian randomization study. Front Nutr. (2023) 10:1149317. doi: 10.3389/fnut.2023.1149317
19. Burgess, S, Butterworth, A, and Thompson, SG. Mendelian randomization analysis with multiple genetic variants using summarized data. Genet Epidemiol. (2013) 37:658–65. doi: 10.1002/gepi.21758
20. Liu, Z, Wang, H, Yang, Z, Lu, Y, Wang, J, and Zou, C. Genetically predicted mood swings increased risk of cardiovascular disease: evidence from a Mendelian randomization analysis. J Affect Disord. (2024) 354:463–72. doi: 10.1016/j.jad.2024.03.076
21. Tachmazidou, I, Hatzikotoulas, K, Southam, L, Esparza-Gordillo, J, Haberland, V, Zheng, J, et al. Identification of new therapeutic targets for osteoarthritis through genome-wide analyses of UK Biobank data. Nat Genet. (2019) 51:230–6. doi: 10.1038/s41588-018-0327-1
22. Burgess, S, Small, DS, and Thompson, SG. A review of instrumental variable estimators for Mendelian randomization. Stat Methods Med Res. (2017) 26:2333–55. doi: 10.1177/0962280215597579
23. Burgess, S, and Thompson, SG. Interpreting findings from Mendelian randomization using the MR-egger method. Eur J Epidemiol. (2017) 32:377–89. doi: 10.1007/s10654-017-0255-x
24. Bowden, J, Del Greco, MF, Minelli, C, Davey Smith, G, Sheehan, N, and Thompson, J. A framework for the investigation of pleiotropy in two-sample summary data Mendelian randomization. Stat Med. (2017) 36:1783–802. doi: 10.1002/sim.7221
25. Zhang, Y, Fan, J, Chen, L, Xiong, Y, Wu, T, Shen, S, et al. Causal Association of Coffee Consumption and Total, knee, hip and self-reported osteoarthritis: a Mendelian randomization study. Front Endocrinol (Lausanne). (2021) 12:768529. doi: 10.3389/fendo.2021.768529
26. Bang, CH, Kim, C, Kim, J-H, Choi, SJ, Song, GG, and Jung, JH. Is knee osteoarthritis related to coffee drinking? A nationwide cross-sectional observational study. Clin Rheumatol. (2019) 38:817–25. doi: 10.1007/s10067-018-4354-1
27. Lee, YH . Investigating the possible causal association of coffee consumption with osteoarthritis risk using a Mendelian randomization analysis. Clin Rheumatol. (2018) 37:3133–9. doi: 10.1007/s10067-018-4252-6
28. Guillán-Fresco, M, Franco-Trepat, E, Alonso-Pérez, A, Jorge-Mora, A, López-Fagúndez, M, Pazos-Pérez, A, et al. Caffeine, a risk factor for osteoarthritis and longitudinal bone growth inhibition. J Clin Med. (2020) 9:1163. doi: 10.3390/jcm9041163
29. Kuo, C-L, Liu, S-T, Chang, Y-L, Wu, C-C, and Huang, S-M. Zac1 regulates IL-11 expression in osteoarthritis. Oncotarget. (2018) 9:32478–95. doi: 10.18632/oncotarget.25980
30. Huang, X, Zhang, J, Zheng, Y, Liu, X, Xu, Y, Fang, Y, et al. Alcoholic drink produced by pea is a risk factor for incident knee surgery in patients with knee osteoarthritis. Front Nutr. (2023) 10:1264338. doi: 10.3389/fnut.2023.1264338
31. Klimek-Szczykutowicz, M, Szopa, A, and Ekiert, H. Chemical composition, traditional and professional use in medicine, application in environmental protection, position in food and cosmetics industries, and biotechnological studies of Nasturtium officinale (watercress) – a review. Fitoterapia. (2018) 129:283–92. doi: 10.1016/j.fitote.2018.05.031
32. Liu, S, Deng, Z, Chen, K, Jian, S, Zhou, F, Yang, Y, et al. Cartilage tissue engineering: from proinflammatory and anti-inflammatory cytokines to osteoarthritis treatments (review). Mol Med Rep. (2022) 25:12615. doi: 10.3892/mmr.2022.12615
33. Lu, H, Wei, J, Liu, K, Li, Z, Xu, T, Yang, D, et al. Radical-scavenging and subchondral bone-regenerating nanomedicine for osteoarthritis treatment. ACS Nano. (2023) 17:6131–46. doi: 10.1021/acsnano.3c01789
34. Messina, OD, Vidal Wilman, M, and Vidal Neira, LF. Nutrition, osteoarthritis and cartilage metabolism. Aging Clin Exp Res. (2019) 31:807–13. doi: 10.1007/s40520-019-01191-w
35. Goggs, R, Vaughan-Thomas, A, Clegg, PD, Carter, SD, Innes, JF, Mobasheri, A, et al. Nutraceutical therapies for degenerative joint diseases: a critical review. Crit Rev Food Sci Nutr. (2005) 45:145–64. doi: 10.1080/10408690590956341
36. Li, G, Yin, J, Gao, J, Cheng, TS, Pavlos, NJ, Zhang, C, et al. Subchondral bone in osteoarthritis: insight into risk factors and microstructural changes. Arthritis Res Ther. (2013) 15:223. doi: 10.1186/ar4405
37. Rozenberg, S, Body, J-J, Bruyère, O, Bergmann, P, Brandi, ML, Cooper, C, et al. Effects of dairy products consumption on health: benefits and beliefs--a commentary from the Belgian bone Club and the European Society for Clinical and Economic Aspects of osteoporosis, osteoarthritis and musculoskeletal diseases. Calcif Tissue Int. (2016) 98:62. doi: 10.1007/s00223-015-0062-x
38. Gorissen, L, Leroy, F, De Vuyst, L, De Smet, S, and Raes, K. Bacterial production of conjugated linoleic and linolenic acid in foods: a technological challenge. Crit Rev Food Sci Nutr. (2015) 55:1561–74. doi: 10.1080/10408398.2012.706243
39. Stergiadis, S, Berlitz, CB, Hunt, B, Garg, S, Ian Givens, D, and Kliem, KE. An update to the fatty acid profiles of bovine retail milk in the United Kingdom: implications for nutrition in different age and gender groups. Food Chem. (2019) 276:218–30. doi: 10.1016/j.foodchem.2018.09.165
40. Djuricic, I, and Calder, PC. Beneficial outcomes of Omega-6 and Omega-3 polyunsaturated fatty acids on human health: an update for 2021. Nutrients. (2021) 13:2421. doi: 10.3390/nu13072421
41. Biver, E, Berenbaum, F, Valdes, AM, Araujo de Carvalho, I, Bindels, LB, Brandi, ML, et al. Gut microbiota and osteoarthritis management: an expert consensus of the European society for clinical and economic aspects of osteoporosis, osteoarthritis and musculoskeletal diseases (ESCEO). Ageing Res Rev. (2019) 55:100946. doi: 10.1016/j.arr.2019.100946
Keywords: osteoarthritis, dietary preferences, Mendelian randomization, causality, SNP
Citation: Chen L, Su Y, Li H, Yang Z, Li JJ and Xing D (2024) The role of dietary preferences in osteoarthritis: a Mendelian randomization study using genome-wide association analysis data from the UK Biobank. Front. Nutr. 11:1373850. doi: 10.3389/fnut.2024.1373850
Edited by:
Han Feng, Tulane University, United StatesReviewed by:
Chenguang Zhang, University of Texas Health Science Center at Houston, United StatesMayana Bsoul, Tulane University, United States
Yuxuan Gu, University of Texas Health Science Center at Houston, United States
Copyright © 2024 Chen, Su, Li, Yang, Li and Xing. This is an open-access article distributed under the terms of the Creative Commons Attribution License (CC BY). The use, distribution or reproduction in other forums is permitted, provided the original author(s) and the copyright owner(s) are credited and that the original publication in this journal is cited, in accordance with accepted academic practice. No use, distribution or reproduction is permitted which does not comply with these terms.
*Correspondence: Dan Xing, xingdan@bjmu.edu.cn