- 1Tianjin Key Laboratory of Cerebral Vascular and Neurodegenerative Diseases, Tianjin Neurosurgical Institute, Tianjin Huanhu Hospital, Tianjin, China
- 2Tianjin Key Laboratory of Brain Science and Neural Engineering, Tianjin University, Tianjin, China
- 3Tianjin Huanhu Hospital, Tianjin, China
Background: The relationship between vitamin D levels, depressive symptoms, and cognitive function has yet to be definitively understood in the elderly, particularly when considering the impact of chronic diseases. This study focuses on how depression mediates the impact of 25-hydroxyvitamin D3 (25(OH)D3) on cognitive performance in older U.S. adults.
Methods: We analyzed data from 2,745 elderly individuals extracted from the NHANES 2011–2014 cycles, applying weighted processing to account for the complex multi-stage sampling design characteristic of NHANES data. Utilizing weighted data for covariate and model selection, we conducted mediation analyses on both the overall population and subgroup data. Significant mediation pathways were validated using a stratified weighted bootstrap approach. For significant subgroup pathways, we explored interactive mechanisms through interactive mediation analysis.
Results: Mediation analyses, thoroughly accounting for the impact of chronic conditions, revealed significant pathways in both the weighted overall population and the weighted diabetes subgroup. After 1,000 stratified weighted bootstrap replications, the proportion of mediation effects were 10.6% [0.040, 0.268] and 20.9% [0.075, 0.663], respectively. Interactive mediation analysis for diabetes indicated that the interaction between diabetes and depression was not significant in the direct pathway (estimates = 0.050, p = 0.113) but was significant in the mediation pathway, yielding the largest effect size compared to other covariates (estimates = 0.981, p < 0.001).
Conclusion: This study highlights the mediating role of depression in the relationship between vitamin D levels and cognitive function in the elderly, particularly emphasizing diabetes as a key moderator. Our findings suggest targeted interventions addressing both vitamin D sufficiency and depression could significantly benefit cognitive health, especially in diabetic individuals.
1 Introduction
Cognitive dysfunction, increasingly prevalent in geriatric populations, not only escalates healthcare burdens but also severely diminishes their quality of life (1). Vitamin D, a fat-soluble steroid, has been demonstrated to have profound effects on physical and mental health due to its significant roles in the cardiovascular, endocrine, and nervous systems. The relationship between vitamin D levels and depression is supported by both the presence of vitamin D-related components in brain tissue and the plausible biological mechanisms through which vitamin D influences neurological functions (2, 3). These mechanisms include the regulation of neurotransmitter synthesis, which is crucial for neurological functions stabilization, and the modulation of inflammatory responses, which have been implicated in the pathophysiology of depression (2, 3). Two follow-up cohort studies have found that vitamin D deficiency is a risk factor for depression in the elderly (4, 5). Additionally, existing research has established that the relationship between depression and cognitive function is unidirectional, with depression occurring prior to the onset of cognitive impairments and serving as a longitudinal risk factor for cognitive decline (6, 7). Therefore, we posit that there may exist a pathway in elderly individuals where vitamin D influences cognitive function through the mediation of depression. This hypothesis highlights the importance of considering the interplay between nutritional status, mental health, and cognitive health in the aging population, suggesting a sequential and interconnected process that warrants further investigation.
The elderly represent a distinct demographic, often grappling with a greater number of chronic conditions than their younger counterparts. Likewise, various chronic diseases are known to influence the onset of depression and cognitive decline. Evidence indicates that older adults with diabetes are at heightened risk for developing both depression and cognitive dysfunction (8, 9). Clinically significant depression is observed in one out of every four individuals with type 2 diabetes mellitus (10). Evidence indicates that diabetes significantly contributes to cognitive dysfunction and predisposes individuals to cognitive decline, with a 50% increased risk of developing dementia (11). Other common chronic diseases among older adults, such as hypertension, stroke, and heart disease, have also been reported to be associated with the occurrence of depression and cognitive dysfunction (12–17). Without accounting for such covariates as chronic diseases, we might struggle to accurately differentiate between the direct and indirect effects of vitamin D levels on cognition, and to precisely determine the significance of the mediating effect of depression. Neglecting these covariates could obscure the true relationship between vitamin D and cognitive outcomes, as well as the mediating role of depression.
In our research, we extend beyond common demographic and lifestyle factors to particularly emphasize the impact of chronic disease-related covariates on how vitamin D and depression mediate cognitive function. This includes considering the effects of chronic diseases both in mediating pathways and direct pathways. Moreover, we have conducted subgroup mediation effect analyses on chronic disease factors that demonstrated statistical significance in preliminary screenings, using them as grouping variables. All analyses, including variable selection, model fitting, and subgroup analyses, are based on the complex stratified weighted data from the National Health and Nutrition Examination Survey (NHANES), utilizing the official NHANES weights.1 This approach ensures our findings reflect the real situation across the U.S. civilian, non-institutionalized population. Our objective is to delineate and validate the pathways through which vitamin D impacts cognitive function by mediating depression, with a special focus on the role of chronic diseases.
2 Materials and methods
2.1 Study population
Data from the 2011–2014 NHANES cycles are used in our study, as only these cycles include our selected research variables. We excluded all rows with missing values in the key variables of cognitive function, depression, and 25(OH)D3. The remaining dataset, comprising 2,745 individuals aged 60 and over, retained covariates with less than 10% missing values for further analysis. The study population, based on complex multistage probability weighted data, consisted of approximately 46% men and 54% women, with a total weighted sample size of 50,667,046. The median age of the weighted sample was 68 years, with an interquartile range of 63 to 74 years (Supplementary Table S1). This survey design ensures that the sample is representative of the non-institutionalized elderly civilian US population, capturing a broad range of demographic and socioeconomic backgrounds to provide a robust basis for our analysis.
Participants were included based on their willingness and ability to participate in comprehensive health assessments. These assessments were conducted by trained interviewers and healthcare providers, either in participants’ homes or at Mobile Examination Centers (MEC). Ethical approval for the data collection was obtained from the National Center for Health Statistics Ethics Review Board, and informed consent was secured from all participants. Detailed interview protocols and measurement methodologies are described in the NHANES documentation.2
2.2 Cognitive function assessment
The NHANES 2011–2014 cycles included a comprehensive cognitive assessment battery that consisted of three tests: the Consortium to Establish a Registry for Alzheimer’s Disease (CERAD), the Animal Fluency Test (AF), and the Digit Symbol Substitution Test (DSST). The CERAD evaluated immediate and delayed memory function through a set of learning and recall trials involving 10 unrelated words. The assessment was comprised of three Word Learning Subtests and one Delayed Word Recall test. We aggregated these scores to arrive at a total CERAD score (18). The AF assessed categorical verbal fluency by asking participants to name as many animals as possible within a minute, with a preliminary test involving naming three articles of clothing. The DSST gaged processing speed, sustained attention, and working memory by requiring participants to match symbols and numbers on a paper form within 2 min. To create a composite cognitive function score as our dependent variable, we averaged the z-scores of three standardized tests that collectively assess cognitive function (19). Poor cognitive performance was identified as scores falling in the lowest 25th percentile for the composite score, after accounting for NHANES sampling weights, aligning with previous NHANES-based studies (20).
2.3 Depression of patient health questionnaire-9 (PHQ-9)
Depressive symptoms were assessed using the Patient Health Questionnaire-9 (PHQ-9), a widely accepted and validated self-rating scale administered during face-to-face interviews at MEC. The PHQ-9 consists of nine items that evaluate various facets of depression, including anhedonia, depressed mood, sleep disturbance, and suicidal ideation, among others. Respondents rated each item on a scale from 0 to 3, resulting in a total score ranging from 0 to 27, where higher scores indicate more severe depressive symptoms. To achieve analytical consistency with cognitive scores and 25(OH)D3 trends, we transformed the PHQ-9 scores using the formula: Depression Score = 27 − Original Score (actual PHQ-9 score). This transformation aligns the depression scores directionally with cognitive function scores and 25(OH)D3 levels, where higher values consistently represent better outcomes, thereby making the model’s interpretation more straightforward and consistent. We analyzed the depression scores as a continuous mediator variable.
2.4 Laboratory measurement of 25(OH)D3
The concentrations of serum 25(OH)D3, 25(OH)D2 and C3-epi-25(OH)D3 were analyzed using high-performance liquid chromatography tandem mass spectrometry (LC–MS/MS) at the National Center for Environmental Health, CDC, Atlanta, GA. Calibration and quality control followed NHANES laboratory procedures. The total serum 25(OH)D level was computed by summing the levels of 25(OH)D3 and 25(OH)D2. We selected 25(OH)D3 as the independent variable for our mediation analysis for several reasons. First, it is the most metabolically stable and abundant form of vitamin D metabolite (21), with its serum concentrations serving as a reliable biomarker for assessing an individual’s Vitamin D status (22). Measurement of 25(OH)D3 thus represents a more accurate estimation of in vivo vitamin D status (23). Secondly, in the case of the continuous variable 25(OH)D2, the proportion of truncated values is excessively high (74.1%), which compromises its accuracy and, by extension, affects the accuracy of the combined value of total 25(OH)D.
2.5 Covariates
We selected covariates from the NHANES dataset based on their relevance to depression and cognitive function, as determined by literature reviews and expert consultation. These covariates include age, gender, poverty income ratio (PIR), marital status, educational background, Body Mass Index (BMI), waist circumference, smoking status, alcohol consumption, season of exam, and historical chronic diseases such as diabetes, hypertension, heart diseases (including congestive heart failure, coronary heart disease, angina/angina pectoris, and heart attack), emphysema, chronic bronchitis, malignancy, hyperlipidemia, stroke, and sleep disorders. All information was acquired through standardized questioning, physical examinations, and laboratory tests, provided by qualified medical staff.
2.6 Statistical analysis
To ensure our statistical analysis accurately represents the complex structure of NHANES, we adapted all our statistical analysis methods to align with its sophisticated, multistage, probability sampling design, explicitly incorporating complex weights to achieve accurate estimates that reflect the U.S. population. The cognitive score was divided into quartiles, with quantile thresholds determined using survey-weighted quantile analysis. We present the descriptive results in two forms: weighted data, to provide estimates that are generalizable to the population level, and unweighted data, which represent the raw data without considering the survey’s stratification and clustering. For categorical variables, we display frequencies and percentages, denoted as n (%). Continuous variables are described using median values with the interquartile range, indicated as M (Q1, Q3), for both weighted and unweighted data. Variable selection was conducted using weighted data. For comparisons between categorical variables and outcome variable, we applied an adjusted Chi-squared test, which accounts for the design effect in survey data. This adjustment makes the test appropriate for contingency tables, particularly those with small expected frequencies in cells. For continuous variables, the comparison with outcome variable was carried out using a Wilcoxon rank-sum test tailored for complex survey samples. The variables extracted for our study were those with less than 10% missing values. Missing data were filled using Random Forest Imputation via the missForest package. This technique excels at handling nonlinear relationships between variables and offers a robust, high-accuracy approach for imputing both numerical and categorical data without requiring distributional assumptions (24). For subgroup analysis, we selected age, gender, and chronic disease-related variables as grouping factors, ensuring that each subgroup demonstrated a power value greater than 0.8 on the actual number of data points. This criterion guarantees the statistical robustness and representativeness of our analysis, focusing on variables with sufficient statistical power to detect meaningful differences.
From both overall and subgroup perspectives, we employed survey-weighted generalized linear modeling (GLM) with a Gaussian family to assess the effects of the independent variables on the mediator, the mediator on the outcome variable, and the independent variables on the outcome variable. This initial analysis aimed to determine the statistical significance of these relationships, thereby establishing the necessity for mediation analysis. Furthermore, we assessed the interaction effects between 25(OH)D3 and depression within the cohort in a weighted analysis to mitigate the potential influence of interaction effects. This step is critical in ensuring that the mediation analysis is not confounded by unaccounted interaction between variables.
In our analysis, we employed structural equation modeling (SEM) specifically tailored for the complex, weighted survey design. We integrated key aspects of the survey’s methodology, including the designation of primary sampling units (PSUs) and the careful stratification of the data into distinct layers. Each model took into account the hierarchical structure of the data through nesting, alongside the application of survey weights, which are pivotal in ensuring our analyses accurately represent the broader U.S. civilian, non-institutionalized population. By strategically incorporating chronic disease covariates into the mediation and direct effect paths in varying configurations, we constructed five multifactorial mediation analysis models, each designed to explore different aspects of the relationships among chronic conditions, mediators, and outcomes. We conduct a comprehensive evaluation of several fit indices for SEM, including the comparative fit index (CFI), Tucker-Lewis index (TLI), Akaike information criterion (AIC), Bayesian information criterion (BIC), standardized root mean square residual (SRMR), and sample-size adjusted BIC (SABIC), to select the model with the best fit.
The chosen model is then used to perform mediation analysis on both the weighted total population data and weighted subgroup data. The criteria for determining significant pathways were based on the condition that the p-values for direct effects, mediation effects, and total effects were all less than 0.05. For the validation of significant pathways, we developed stratified weighted bootstrap mediation analysis for complex survey data, with an emphasis on maintaining the integrity of the survey’s design, including stratification, clustering, and weighting. This approach meticulously generates 1,000 weighted bootstrap samples, with a unique emphasis on ensuring that each stratum within the data has at least two primary sampling units (PSUs). This requirement is crucial for preserving the representativeness and variability of the survey’s stratified design, thereby ensuring that the integrity of the survey structure is upheld in the analysis. For significant subgroup pathways, we adopt the approach of incorporating depression interaction terms into both the mediation and direct paths. This method allows us to analyze the moderating mechanism of the subgroup on depression. Finally, as a sensitivity analysis, we applied the same mediation analysis model to unweighted NHANES data to examine the stability of significant pathways.
All statistical analyses were completed by R 4.2.3 software and the results were considered statistically significant when the bilateral p-value <0.05.
3 Results
3.1 Baseline characteristics of the study population
Table 1 presents the baseline characteristics of the study population, with statistical descriptions provided for both data adjusted using NHANES’s complex survey weights and for unweighted data from the 2011–2014 NHANES dataset. The medians for continuous variables and the proportions for categorical variables differ between the weighted and unweighted data, underscoring the impact of the NHANES complex weighting. Nevertheless, the directional trends across both the weighted and unweighted data remain similar with respect to the association with cognitive function categories. Individuals with conditions such as hypertension, diabetes, heart disease, and stroke are more prevalent in the cohort with poor cognitive function than in those with better cognitive function.
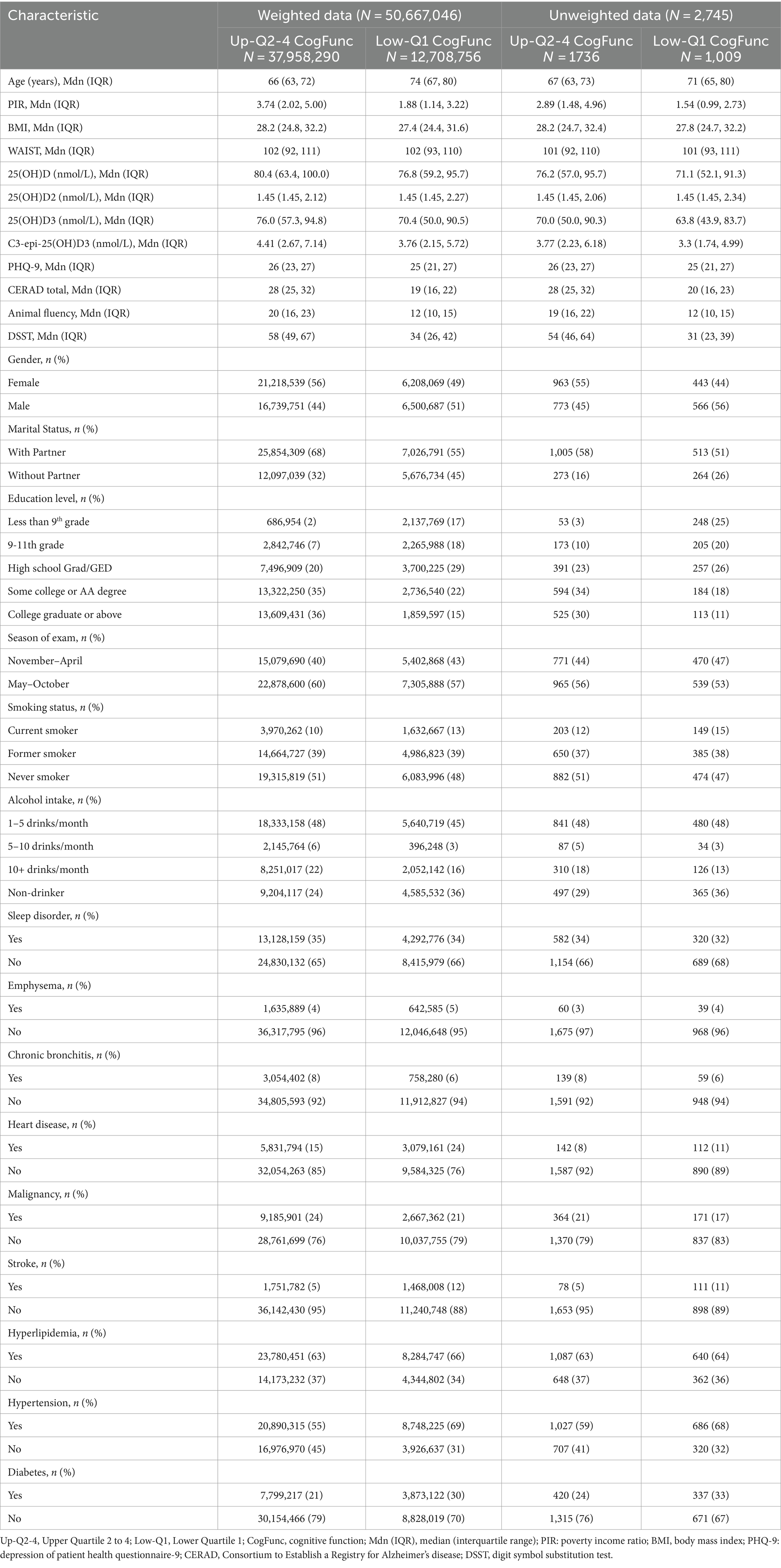
Table 1. Characteristics of adults aged 60 and over, stratified by cognitive function category in the 2011–2014 NHANES.
3.2 Covariable selection and preliminary analysis for mediation analysis
In our analysis, the covariables selected for further study based on the weighted data were stroke, alcohol consumption, education level, age group, gender, income-to-poverty ratio, diabetes, hypertension, heart disease, and marital status (Supplementary Table S1). Preliminary analysis confirmed the statistical significance of the relationships between 25(OH)D3 levels, depression, and cognitive function, which laid the groundwork for the subsequent mediation analysis. Upon controlling for all selected covariates, both 25(OH)D3 (Estimate = 0.049, p = 0.010) and depression (Estimate = 0.066, p = 0.005) were identified as independent predictors of cognitive function. A significant positive association was also established between 25(OH)D3 levels and depression scores (Estimate = 0.098, p = 0.001). This association persisted (Estimate = 0.094, p = 0.001) when taking into account the influence of chronic diseases (stroke, hypertension, diabetes and heart disease). In the results presented in Table 2, the survey-weighted regression analyses demonstrate significant associations between 25(OH)D3 levels, depression scores, and cognitive outcomes across both the entire cohort and most stratified subgroups after adjusting for NHANES complex survey designs. In Figure 1, the analysis reveals no significant interaction between 25(OH)D3 levels and depression scores in influencing cognitive outcomes, both in the univariate model and the multivariate model.
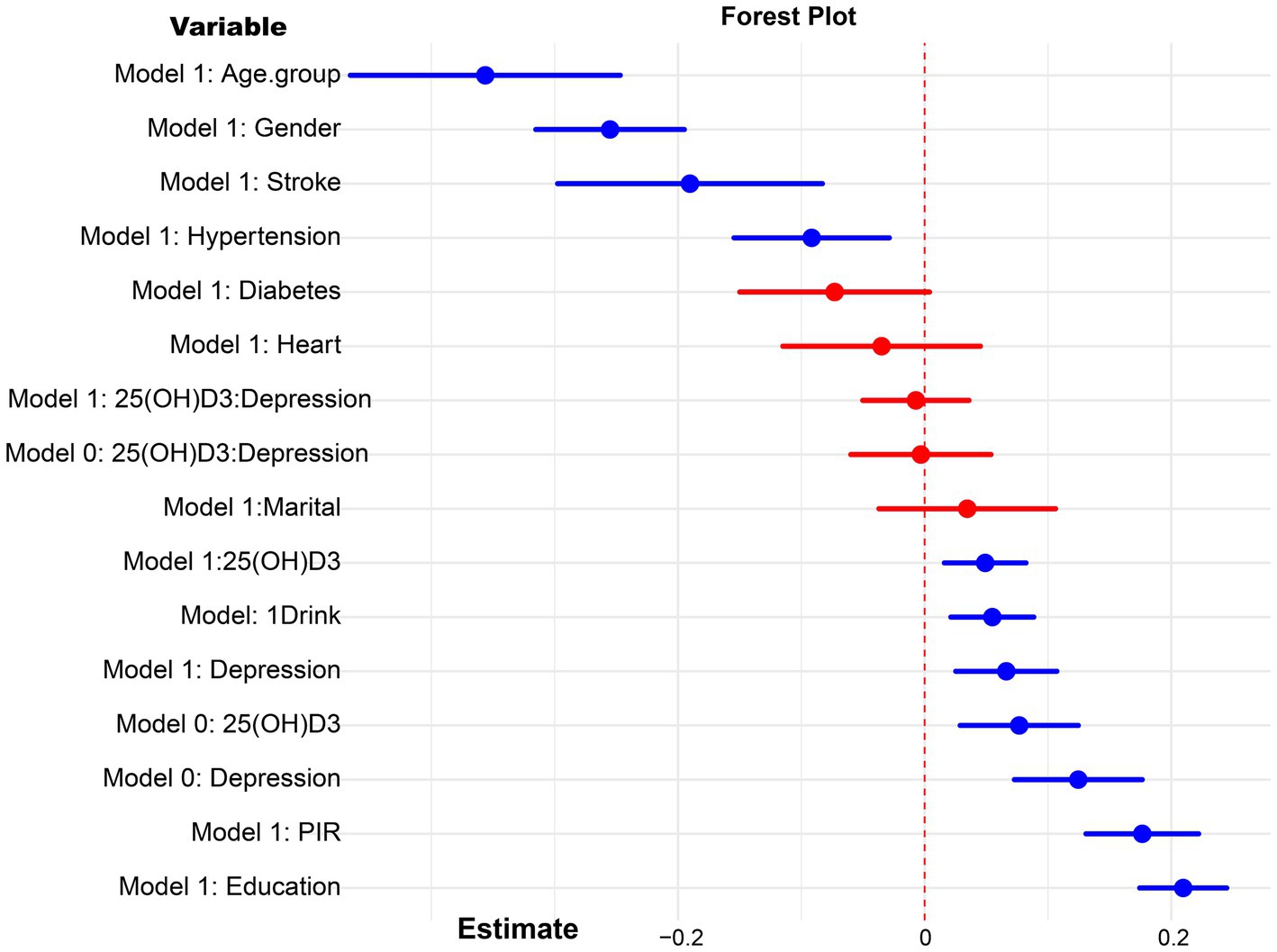
Figure 1. Forest plot for the interaction analysis of 25(OH)D3 and depression on cognitive function. Model 0: crude model, model 1: adjusted for age, gender, education, poverty income ratio (PIR), marital status, drink, stroke, heart disease, diabetes, hypertension.
3.3 Selection of stratified weighted mediation analysis models
Upon evaluating various fit indices such as the Comparative Fit Index (CFI), Tucker-Lewis Index (TLI), Standardized Root Mean Square Residual (SRMR), Akaike Information Criterion (AIC), Bayesian Information Criterion (BIC), and Sample-Size Adjusted BIC (SABIC), Model 1 proved to be the most appropriate. It demonstrated an optimal balance among these measures, suggesting that it best captures the data structure when compared to other models, as detailed in Table 3. The results of the path analysis for Model 1 in the weighted total population are presented in Figure 2.
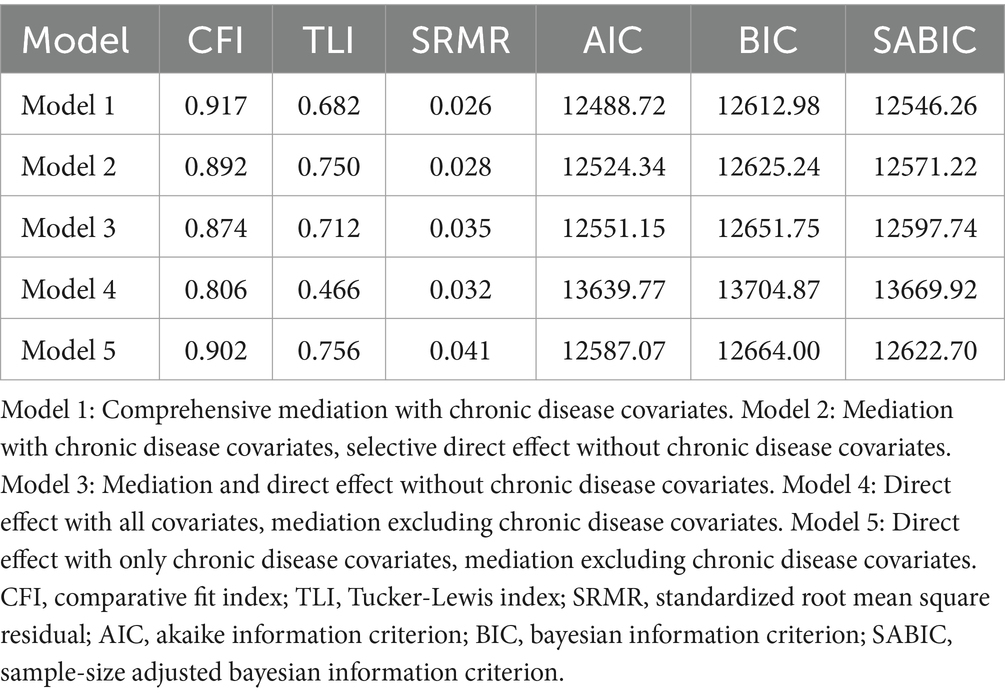
Table 3. Comparative fit indices for weighted SEM models with varied inclusion of chronic disease covariates.
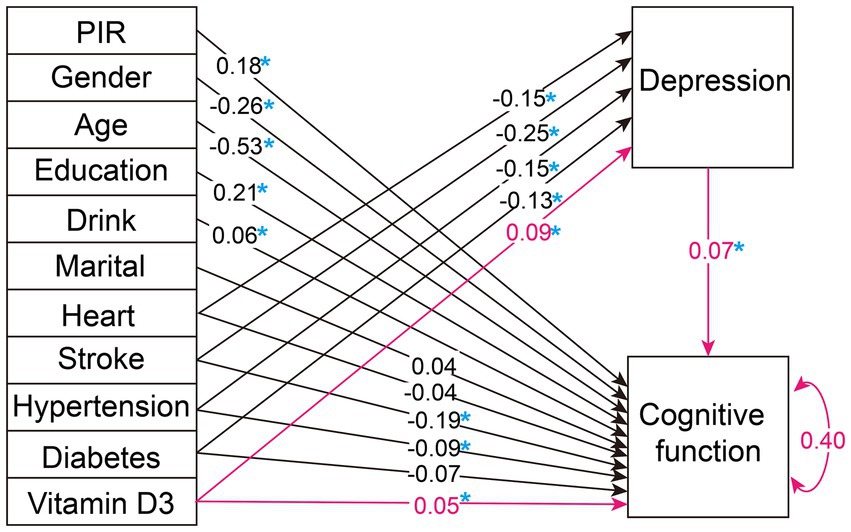
Figure 2. Weighted overall population path analysis diagram. The figure illustrates the path diagram for the variables analyzed in our selected SEM, using the weighted total population. Black lines with associated values represent path estimates on depression and cognitive function, respectively. The red lines highlight the study's focal path estimates. The value of 0.40 adjacent to 'Cognitive function' indicates the estimated residual variance for the dependent variable within the SEM. PIR, poverty income ratio; Heart, heart disease. A blue asterisk indicates statistical significance.
3.4 Mediation analysis for weighted total and subgroup data
Mediation analysis was conducted using Model 1 on both the weighted total population data and weighted subgroup data. As presented in Table 4, significant pathways were identified in the overall data and within the diabetes subgroup. In contrast, other pathways did not show statistical significance either in the mediation effect, direct effect, or total effect.
3.5 Validation of significant pathways
We developed a stratified weighted bootstrap approach to validate the robustness of mediation pathways in both the weighted overall population and the weighted diabetic subgroup. Figure 3, based on 1,000 bootstrap resamples, illustrates that the mediated effect accounts for 20.9% [0.075, 0.663] in the diabetic subgroup. For the general population, the mediated effect is 10.6% [0.040, 0.268]. Both effects were statistically significant, as indicated by their 95% bootstrap confidence intervals not encompassing zero.
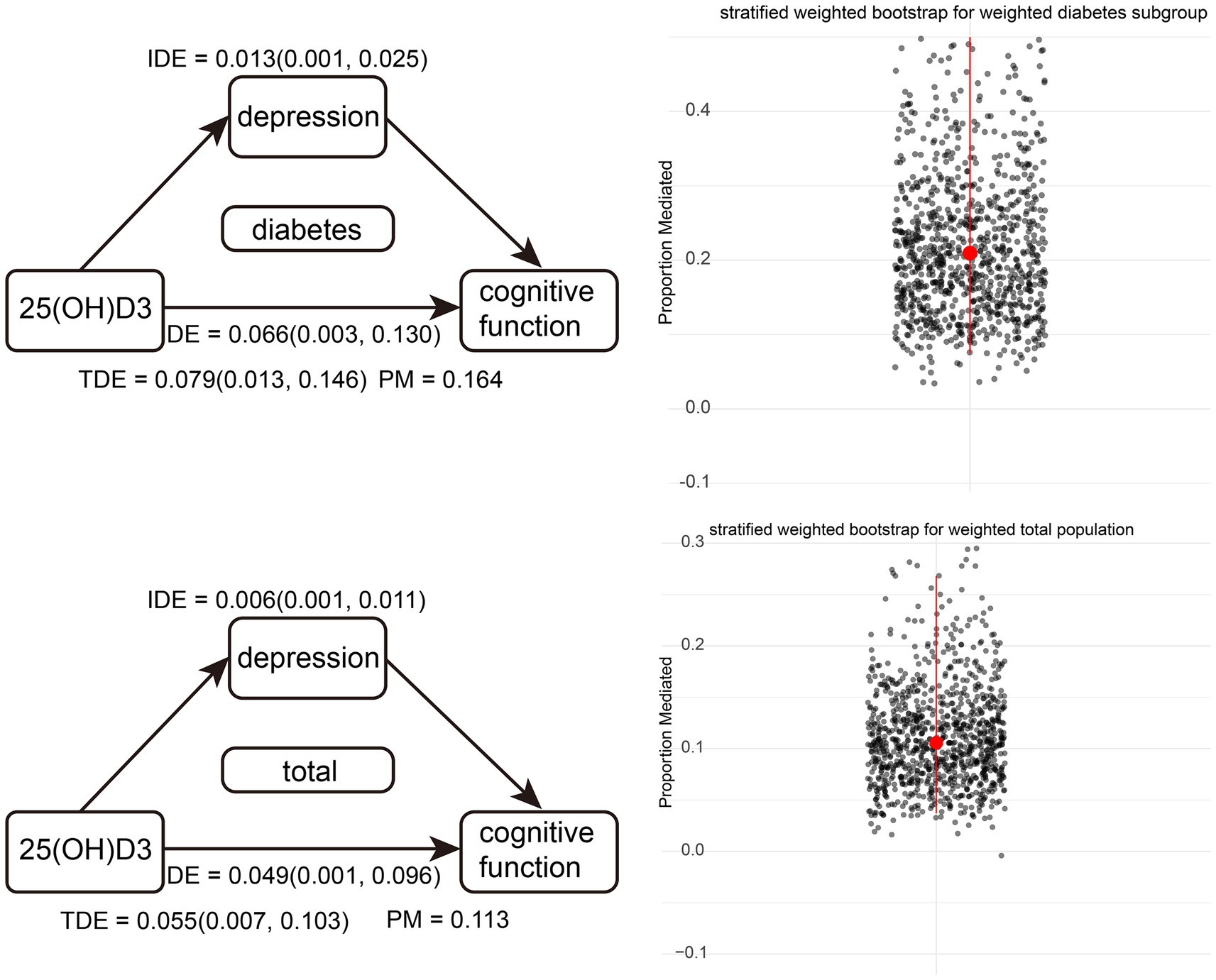
Figure 3. Diagram of weighted overall population and weighted diabetes subgroup pathways, along with stratified weighted bootstrap results. Red dots represent the medians of 1,000 resampling iterations, and red lines indicate confidence intervals.
3.6 Interactive mediation analysis for depression and diabetes
We further explored the moderating role of diabetes in the mediation analysis. Figure 4 presents the path analysis results after incorporating interaction terms into both the direct and mediated pathways. Notably, the introduction of an interaction term between diabetes and depression yields a statistically significant estimate of 0.981 in the mediation pathway, surpassing all other variables in the mediation analysis. However, this interaction term is not significant in the direct pathway. Therefore, we consider diabetes to serve as an important moderator of depression in the mediation pathway.
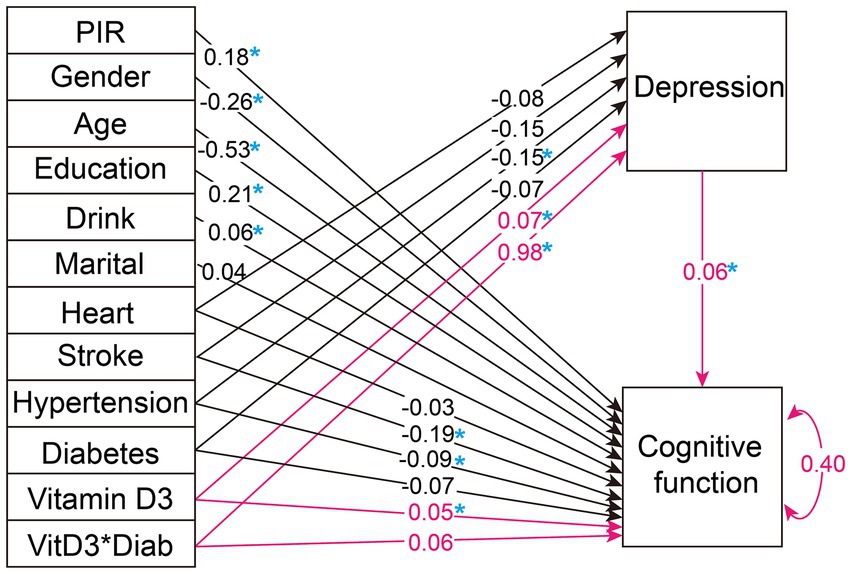
Figure 4. Path analysis diagram for the weighted overall population with the addition of diabetes and depression interaction terms. The figure illustrates the path diagram for the variables analyzed in selected SEM, using the weighted total population. Black lines with associated values represent path estimates on depression and cognitive function, respectively. The red lines highlight the focal path estimates of the mediation effect of depression moderated by diabetes. The value of 0.40 adjacent to 'Cognitive function' indicates the estimated residual variance for the dependent variable within the SEM. PIR, poverty income-ratio; Heart, heart disease. A blue asterisk indicates statistical significance.
3.7 Sensitivity analysis
We applied Model 1 to unweighted data for both overall and subgroup mediation analyses, as shown in Table 4. The results indicate that pathways for the general population and the diabetes subgroup remain significant. Additionally, in the unweighted scenario, numerous other significant pathways emerged, including subgroups of elderly under 70 years, females, non-diabetics, and those with hypertension (Table 5).
4 Discussion
In our study, leveraging the complex multi-stage sampling design of NHANES and fully accounting for the impact of chronic conditions in the elderly, we conducted an in-depth analysis of how vitamin D affects cognitive function through depression as a mediating factor. Several studies across diverse regions have reported that lower vitamin D is associated with cognitive impairment in the elderly (25, 26), which was consistent with our hypothesis. However, other studies showed that there is no association between vitamin D status and cognitive impairment (27–29). Given the conflicting conclusions in existing research, it’s plausible that the relationship between vitamin D and cognitive function is intricate, potentially influenced by mediating factors and different subgroups. Our research, drawing on weighted data from the entire U.S. civilian, non-institutionalized population, confirms the presence of a pathway in which vitamin D impacts cognitive function through depression, both in the general population and specifically within the diabetic subgroup.
Many NHANES-based studies overlook the importance of the survey’s sampling weights in mediation analyses (30, 31). Building on the R survey package, we integrated it with the complex multi-stage sampling design and combined it with the lavaan package, ensuring that weighted data are utilized throughout the mediation analysis process (32). This methodology not only significantly reduces bias in our estimates but also enhances the statistical power of subgroup analyses by improving generalizability. By considering the design effect and employing appropriate weighting methods, our approach effectively addresses the challenges of unbalanced subgroup sizes, thereby ensuring more accurate and representative analysis outcomes (33). For significant mediation pathways, we implemented a validation process using a robust stratified weighted bootstrap approach, which we developed specifically to accommodate the complex multi-stage sampling design of NHANES. This innovative method underscores the stability and replicability of our findings.
Beyond the general population, the diabetes subgroup also exhibited the significant pathway, prompting further investigation into the mechanisms by which diabetes influences the mediation effect of depression. Our analysis revealed that the interaction term between depression and diabetes presented the highest estimate value (0.981) among all covariates within the mediation pathway, positioning diabetes as a crucial conditional moderator in the mediation of depression. This assertion is well-supported theoretically, as depression and diabetes frequently co-occur (34). Both depression and diabetes are intricately linked to disruptions in brain function and neuroplasticity, evidenced by changes in brain chemistry and structure, such as altered prefrontal neurotransmitter levels and hippocampal atrophy, which impact cognition and mood (35). Research indicates that the prevalence of depression is threefold higher in individuals with prediabetes and twofold higher in diabetes patients compared to the general population (36).
The sensitivity analyses, using unweighted NHANES data across subgroups enhanced our study’s confidence. Beyond the significant pathways identified in the general population and the diabetic subgroup, significant pathways also emerged among the elderly under 70, females, non-diabetics, and hypertensive individuals. This outcome suggests that the impact of vitamin D, mediating through depression on cognitive function, may be more extensive than previously thought, underlining its significance across various population segments. The sensitivity analysis also hints at a potential overestimation of effects when the survey design is not considered, thereby emphasizing the importance of incorporating NHANES weighting throughout our research for accurate and meaningful public health insights. Our findings highlight the potential for interventions such as targeted vitamin D supplementation to not only improve vitamin D levels but also address depression as a key intermediary, thereby enhancing cognitive function. This approach holds particular promise for diabetic and elderly populations, where the interplay of vitamin D, depression, and cognitive decline appears most significant. These findings pave the way for future randomized controlled trials designed to evaluate the significant pathways identified in our research. Such trials are crucial for refining and potentially enhancing current public health guidelines and clinical practices for the elderly, particularly in the context of leveraging vitamin D to mediate the impact of depression on cognitive function.
There are several limitations present in the study. Firstly, although our findings are supported by existing literature suggesting potential pathways between vitamin D, depression, and cognitive function, given the cross-sectional nature of our research, it inherently lacks the temporal data necessary to establish causality or the sequence of occurrence among these variables. Secondly, the study is also geographically limited to the U.S. elderly population, and despite controlling for multiple variables, including some collected through self-reported questionnaires which may introduce bias, unmeasured confounders may still exist. Thirdly, since our analysis methods incorporated weights from NHANES’ complex multi-stage sampling design, many machine learning algorithms do not support analyses with complex weights, leading us to employ path analysis. This analytical approach, being a conventional statistical method, is subject to limitations related to p-values, confidence intervals, and statistical power. Fourthly, while this study comprehensively analyzes the mediating role of depression in the relationship between vitamin D levels and cognitive function, it does not directly address factors that might influence vitamin D levels themselves. Future research should explore potential variables that influence vitamin D status, such as BMI, gender, and sun exposure, to expand the research directions and deepen our understanding of the underlying determinants.
5 Conclusion
In conclusion, our study utilizes NHANES data to uncover significant pathways by which vitamin D affects cognitive function through depression, with diabetes serving as a pivotal moderator. Throughout the mediation analysis, we employed NHANES’ complex multi-stage sampling design with weighted data and validated the mediation effect proportion using a stratified weighted bootstrap approach. Our results are representative of the entire U.S. civilian, non-institutionalized elderly population. The findings highlight the need to concurrently address vitamin D sufficiency and mental health in developing cognitive health strategies for the elderly, emphasizing the importance of customized interventions for individuals with diabetes.
Data availability statement
Publicly available datasets were analyzed in this study. This data can be found at: https://www.cdc.gov/nchs/nhanes/index.htm?CDC_AA_refVal=https%3A%2F%2Fwww.cdc.gov%2Fnchs%2Fnhanes.htm.
Ethics statement
The research adhered to the principles of the Declaration of Helsinki and received approval from the Institutional Review Board at the National Centre for Health Statistics.
Author contributions
CS: Conceptualization, Data curation, Formal analysis, Investigation, Methodology, Software, Validation, Visualization, Writing – original draft, Writing – review & editing. CZ: Funding acquisition, Supervision, Writing – original draft. XD: Writing – original draft. DL: Funding acquisition, Supervision, Writing – original draft.
Funding
The author(s) declare that financial support was received for the research, authorship, and/or publication of this article. This work was supported by the Natural Science Foundation of Tianjin (grant number: 20JCZDJC00540) and Tianjin Health and Technology Project (grant number: TJWJ2021MS032).
Acknowledgments
We thank the team at the National Health and Nutrition Examination Survey for their work in gathering and organizing NHANES data and creating the public database.
Conflict of interest
The authors declare that the research was conducted in the absence of any commercial or financial relationships that could be construed as a potential conflict of interest.
Publisher’s note
All claims expressed in this article are solely those of the authors and do not necessarily represent those of their affiliated organizations, or those of the publisher, the editors and the reviewers. Any product that may be evaluated in this article, or claim that may be made by its manufacturer, is not guaranteed or endorsed by the publisher.
Supplementary material
The Supplementary material for this article can be found online at: https://www.frontiersin.org/articles/10.3389/fnut.2024.1356071/full#supplementary-material
Footnotes
References
1. GBD 2019 Dementia Forecasting Collaborators . Estimation of the global prevalence of dementia in 2019 and forecasted prevalence in 2050: an analysis for the global burden of disease study 2019. Lancet Public Health. (2022) 7:e105–25. doi: 10.1016/S2468-2667(21)00249-8
2. Zhao, W, Zhu, DM, Li, Q, Xu, X, Zhang, Y, Zhang, C, et al. Brain function mediates the association between low vitamin D and neurocognitive status in female patients with major depressive disorder. Psychol Med. (2023) 53:4032–45. doi: 10.1017/S0033291722000708
3. Zhao, W, Zhu, DM, Li, S, Cui, S, Jiang, P, Wang, R, et al. The reduction of vitamin D in females with major depressive disorder is associated with worse cognition mediated by abnormal brain functional connectivity. Prog Neuro-Psychopharmacol Biol Psychiatry. (2022) 118:110577. doi: 10.1016/j.pnpbp.2022.110577
4. May, HT, Bair, TL, Lappe, DL, Anderson, JL, Horne, BD, Carlquist, JF, et al. Association of Vitamin D Levels with incident depression among a general cardiovascular population. Am Heart J. (2010) 159:1037–43. doi: 10.1016/j.ahj.2010.03.017
5. Milaneschi, Y, Shardell, M, Corsi, AM, Vazzana, R, Bandinelli, S, Guralnik, JM, et al. Serum 25-Hydroxyvitamin D and depressive symptoms in older women and men. J Clin Endocrinol Metab. (2010) 95:3225–33. doi: 10.1210/jc.2010-0347
6. Wu, Z, Zhong, X, Peng, Q, Chen, B, Zhang, M, Zhou, H, et al. Longitudinal association between cognition and depression in patients with late-life depression: a cross-lagged design study. Front Psych. (2021) 12:577058. doi: 10.3389/fpsyt.2021.577058
7. Bunce, D, Batterham, PJ, Christensen, H, and Mackinnon, AJ. Causal associations between depression symptoms and cognition in a community-based cohort of older adults. Am J Geriatr Psychiatry. (2014) 22:1583–91. doi: 10.1016/j.jagp.2014.01.004
8. Cai, J, Zhang, S, Wu, R, and Huang, J. Association between depression and diabetes mellitus and the impact of their comorbidity on mortality: evidence from a nationally representative study. J Affect Disord. (2024) 354:11–8. doi: 10.1016/j.jad.2024.03.003
9. Moheet, A, Mangia, S, and Seaquist, ER. Impact of diabetes on cognitive function and brain structure. Ann N Y Acad Sci. (2015) 1353:60–71. doi: 10.1111/nyas.12807
10. Semenkovich, K, Brown, ME, Svrakic, DM, and Lustman, PJ. Depression in type 2 diabetes mellitus: prevalence, impact, and treatment. Drugs. (2015) 75:577–87. doi: 10.1007/s40265-015-0347-4
11. Sebastian, MJ, Khan, SK, Pappachan, JM, and Jeeyavudeen, MS. Diabetes and cognitive function: an evidence-based current perspective. World J Diabetes. (2023) 14:92–109. doi: 10.4239/wjd.v14.i2.92
12. Ungvari, Z, Toth, P, Tarantini, S, Prodan, CI, Sorond, F, Merkely, B, et al. Hypertension-induced cognitive impairment: from pathophysiology to public health. Nat Rev Nephrol. (2021) 17:639–54. doi: 10.1038/s41581-021-00430-6
13. Zhang, Y, Chen, Y, and Ma, L. Depression and cardiovascular disease in elderly: current understanding. J Clin Neurosci. (2018) 47:1–5. doi: 10.1016/j.jocn.2017.09.022
14. de Bekker, A, Geerlings, MI, Uitewaal-Poslawsky, IE, and de Man-van Ginkel, JM. Depression in stroke survivors: ten-year follow-up. Determinants of the natural course of depressive symptoms in stroke survivors in the Netherlands: the smart-Medea study. J Stroke Cerebrovasc Dis. (2022) 31:106272. doi: 10.1016/j.jstrokecerebrovasdis.2021.106272
15. Rost, NS, Meschia, JF, Gottesman, R, Wruck, L, Helmer, K, Greenberg, SM, et al. Cognitive impairment and dementia after stroke: design and rationale for the Discovery study. Stroke. (2021) 52:e499–516. doi: 10.1161/STROKEAHA.120.031611
16. Alagiakrishnan, K, Mah, D, Ahmed, A, and Ezekowitz, J. Cognitive decline in heart failure. Heart Fail Rev. (2016) 21:661–73. doi: 10.1007/s10741-016-9568-1
17. Sbolli, M, Fiuzat, M, Cani, D, and O'Connor, CM. Depression and heart failure: the lonely comorbidity. Eur J Heart Fail. (2020) 22:2007–17. doi: 10.1002/ejhf.1865
18. Wang, R, Wang, W, Hu, P, Zhang, R, Dong, X, and Zhang, D. Association of Dietary Vitamin D Intake, serum 25(OH)D(3), 25(OH)D(2) with cognitive performance in the elderly. Nutrients. (2021) 13:3089. doi: 10.3390/nu13093089
19. Han, S, Gao, Y, and Gan, D. The combined associations of depression and cognitive impairment with functional disability and mortality in older adults: a population-based study from the Nhanes 2011-2014. Front Aging Neurosci. (2023) 15:1121190. doi: 10.3389/fnagi.2023.1121190
20. Yang, J, Deng, Y, Yan, H, Li, B, Wang, Z, Liao, J, et al. Association between grip strength and cognitive function in us older adults of Nhanes 2011-2014. J Alzheimers Dis. (2022) 89:427–36. doi: 10.3233/JAD-215454
21. Maestro, MA, Molnar, F, and Carlberg, C. Vitamin D and its synthetic analogs. J Med Chem. (2019) 62:6854–75. doi: 10.1021/acs.jmedchem.9b00208
22. Brandi, ML, and Minisola, S. Calcidiol [25(OH)D3]: from diagnostic marker to Therapeutical agent. Curr Med Res Opin. (2013) 29:1565–72. doi: 10.1185/03007995.2013.838549
23. Tay, SH, Ho, CS, Ho, RC, and Mak, A. 25-Hydroxyvitamin D3 deficiency independently predicts cognitive impairment in patients with systemic lupus erythematosus. PLoS One. (2015) 10:e0144149. doi: 10.1371/journal.pone.0144149
24. Jin, H, Jung, S, and Won, S. Missforest with feature selection using binary particle swarm optimization improves the imputation accuracy of continuous data. Genes Genomics. (2022) 44:651–8. doi: 10.1007/s13258-022-01247-8
25. Matsuo, LH, Confortin, SC, Ceolin, G, Soar, C, Xavier, AJ, D'Orsi, E, et al. Association between lower serum vitamin D (25-Hydroxy-cholecalciferol) concentrations and cognitive impairment in older adults: data from a Populational-based cohort study in a middle-income country. Public Health Nutr. (2022) 25:2507–16. doi: 10.1017/S1368980021004407
26. Ates Bulut, E, Soysal, P, Yavuz, I, Kocyigit, SE, and Isik, AT. Effect of vitamin D on cognitive functions in older adults: 24-week follow-up study. Am J Alzheimers Dis Other Dement. (2019) 34:112–7. doi: 10.1177/1533317518822274
27. Olsson, E, Byberg, L, Karlstrom, B, Cederholm, T, Melhus, H, Sjogren, P, et al. Vitamin D is not associated with incident dementia or cognitive impairment: an 18-Y follow-up study in community-living old men. Am J Clin Nutr. (2017) 105:936–43. doi: 10.3945/ajcn.116.141531
28. Kang, JH, Vyas, CM, Okereke, OI, Ogata, S, Albert, M, Lee, IM, et al. Effect of vitamin D on cognitive decline: results from two ancillary studies of the vital randomized trial. Sci Rep. (2021) 11:23253. doi: 10.1038/s41598-021-02485-8
29. Sultan, S, Taimuri, U, Basnan, SA, Ai-Orabi, WK, Awadallah, A, Almowald, F, et al. Low vitamin D and its association with cognitive impairment and dementia. J Aging Res. (2020) 2020:6097820–10. doi: 10.1155/2020/6097820
30. Pan, Q, Shen, X, Li, H, Zhu, B, Chen, D, and Pan, J. Depression score mediate the association between a body shape index and infertility in overweight and obesity females, Nhanes 2013-2018. BMC Womens Health. (2023) 23:471. doi: 10.1186/s12905-023-02622-7
31. Zhou, W, Sun, L, Zeng, L, and Wan, L. Mediation of the association between sleep disorders and cardiovascular disease by depressive symptoms: an analysis of the National Health and nutrition examination survey (Nhanes) 2017-2020. Prev Med Rep. (2023) 33:102183. doi: 10.1016/j.pmedr.2023.102183
32. Reese, H, Sinharoy, SS, and Clasen, T. Using structural equation modelling to untangle sanitation, water and hygiene pathways for intervention improvements in height-for-age in children <5 years old. Int J Epidemiol. (2019) 48:1992–2000. doi: 10.1093/ije/dyz202
33. Bell, BA, Onwuegbuzie, AJ, Ferron, JM, Jiao, QG, Hibbard, ST, and Kromrey, JD. Use of design effects and sample weights in complex health survey data: a review of published articles using data from 3 commonly used adolescent health surveys. Am J Public Health. (2012) 102:1399–405. doi: 10.2105/AJPH.2011.300398
34. Park, M, Katon, WJ, and Wolf, FM. Depression and risk of mortality in individuals with diabetes: a Meta-analysis and systematic review. Gen Hosp Psychiatry. (2013) 35:217–25. doi: 10.1016/j.genhosppsych.2013.01.006
35. Holt, RI, de Groot, M, and Golden, SH. Diabetes and depression. Curr Diab Rep. (2014) 14:491. doi: 10.1007/s11892-014-0491-3
Keywords: mediation analysis, 25-hydroxyvitamin D3, depression, cognitive function, structural equation modeling
Citation: Shu C, Zheng C, Du X and Luo D (2024) Exploring the role of vitamin D in cognitive function: mediation by depression with diabetes modulation in older U.S. adults, a NHANES weighted analysis. Front. Nutr. 11:1356071. doi: 10.3389/fnut.2024.1356071
Edited by:
Paula Goolkasian, University of North Carolina at Charlotte, United StatesReviewed by:
Alessandro Cuomo, University of Siena, ItalyWenming Zhao, First Affiliated Hospital of Anhui Medical University, China
Copyright © 2024 Shu, Zheng, Du and Luo. This is an open-access article distributed under the terms of the Creative Commons Attribution License (CC BY). The use, distribution or reproduction in other forums is permitted, provided the original author(s) and the copyright owner(s) are credited and that the original publication in this journal is cited, in accordance with accepted academic practice. No use, distribution or reproduction is permitted which does not comply with these terms.
*Correspondence: Da Luo, nkchangshu@163.com