- 1Department of Community Health, Guangzhou Center for Disease Control and Prevention, Guangzhou, China
- 2Department of Epidemiology, School of Public Health, Sun Yat-sen University, Guangzhou, China
- 3School of Public Health, Guangdong Pharmaceutical University, Guangzhou, China
- 4Guangzhou Yuexiu District Center for Disease Control and Prevention, Guangzhou, China
- 5School of Public Health and Women’s Hospital, Zhejiang University School of Medicine, Hangzhou, China
- 6Baiyun Street Community Health Service Center, Guangzhou, China
- 7Nancun Town Community Health Service Center, Guangzhou, China
- 8Guangzhou Haizhu District Center for Disease Control and Prevention, Guangzhou, China
- 9Department of Geriatrics, Guangdong Provincial People’s Hospital, Institute of Geriatrics, Guangdong Academy of Medical Science, Guangzhou, China
- 10School of Public Health and Emergency Management, Southern University of Science and Technology, Shenzhen, China
- 11Department of Cardiology, Guangdong Provincial People’s Hospital, Guangdong Cardiovascular Institute, Guangdong Academy of Medical Science, Guangzhou, China
Background: This study aimed to investigate the associations between multiple glycolipid biomarkers and the risk of obstructive sleep apnea (OSA).
Methods: Participants (10,286) aged from 35 to 74 years old were included in this cross-sectional study from the baseline survey of the Guangzhou Heart Study. OSA was ascertained using both Berlin Questionnaire and STOP-BANG Questionnaire. Fasting blood samples were collected from each participant; fasting blood glucose (FBG) and serum concentrations of high-density lipoprotein cholesterol (HDL-CH), low-density lipoprotein cholesterol (LDL-CH), total cholesterol (TC), and triglyceride (TG) were determined. Odds ratio (OR) with 95% confidence interval (CI) was calculated using the multivariate logistic regression model after adjustment for covariates.
Results: Of the participants included, 15.56% were categorized into the pre-OSA group, and 8.22% into the OSA group. When comparing the highest with the lowest quartiles, HDL-HC was associated with a 22% (OR: 0.78, 95% CI: 0.65–0.94) and 41% (OR: 0.59, 95% CI: 0.45–0.78) reduced risk of pre-OSA and OSA, triglyceride was associated with a 32% (OR 1.32, 95% CI 1.08–1.60) and a 56% (OR 1.56, 95% CI 1.18–2.07) increased risk of pre-OSA and OSA, and FBG was associated with a 1.37-fold (95% CI 1.13–1.67) risk of pre-OSA and 1.38-fold (95% CI 1.03–1.85) risk of OSA. A significant exposure-response trend was observed for HDL-HC, TG, and FBG with both OSA and Pre-OSA (all p < 0.05). No significant association of LDL-CH and TC with the risk of both pre-OSA and OSA was observed.
Conclusion: The findings suggest that serum HDL-CH was inversely associated with OSA risk, while elevated serum TG and FBG could increase the risk of OSA. Healthy glycolipid metabolism warrants more attention in the field of OSA prevention.
Introduction
Obstructive sleep apnea (OSA) is a common sleep disorder characterized by recurrent episodes of upper airway obstruction and hypopnea during sleep [1]. It is an adverse health condition that has become increasingly prevalent worldwide [2]. The overall number of affected adults has reached approximately 1 billion [3]. In the United States, the estimated prevalence of OSA ranged from 7 to 12% among men aged 30–49 years old and was even higher as the age went up [4]. China was considered to have the largest number of individuals with OSA among 16 countries [3]. OSA was well recognized as an independent risk factor for multifactorial consequences including cardiovascular diseases, cognitive impairment, [5, 6].
Previous studies have shown that OSA is associated with multiple risk factors, including a low level of physical activity, obesity, and metabolic syndrome [7, 8]. OSA can be both a sleep disorder and a heterogeneous metabolic disorder [9]. Glycolipid biomarkers are considerable screening tools in many chronic diseases [10, 11], the level of which is also considered to be independently associated with the risk of OSA. Pathogenic pathways of the association are consist of activation of the sympathetic nervous system, changes in hypothalamic–pituitary–adrenal axis activity, and formation of reactive oxygen species, etc. [12, 13]. Previous studies have shown that individuals with OSA had a higher prevalence of elevated total cholesterol (TC) and triacylglycerol (TG) [14], and a lower level of high-density lipoprotein cholesterol (HDL-CH) [15]. Dyslipidemia and diabetes, two types of diseases resulting from the dysfunction of glycolipids, were also observed to be associated with OSA in several reports [8, 16, 17]. However, no conclusive results have been found in research on the associations between glycolipid biomarkers and OSA up till now. In a multiethnic study among American people, lower HDL-CH was associated with a higher apnea-hypopnea index (AHI), the key metric used to define OSA severity [18]. In contrast, a remarkable association with AHI was observed in low-dense lipoprotein cholesterol (LDL-CH) but not in HDL-CH among Chinese people [19]. Besides, data from the Heart Institute in America did not present a significant association between HDL-CH and OSA [20].
Therefore, this study aimed to assess the association between glycolipid biomarkers and the risk of OSA using data from the Guangzhou Heart Study (GZHS).
Method
Setting and subjects
Participants in this cross-sectional study were recruited from baseline survey of the Guangzhou Heart Study (GZHS), which recruited 12,013 individuals aged 35 years old and above using a randomized multistage cluster sampling between July 2015 and August 2017. Detailed information of the cohort has been reported elsewhere [7, 21–23]. The inclusion criteria for this study were: (1) Guangzhou permanent residents, (2) aged 35 years old and above; (3) having lived in the selected communities for at least 6 months before being involved into the study. Those who were aged 75 years old and above, were pregnant or lactating women, were non-permanent residents in Guangzhou, had mental or cognitive disorders including dementia, disturbance of understanding and deaf-mutters, had mobility difficulties including high paraplegia, and had any cancer history, were excluded.
Finally, a total of 10,826 individuals from the GZHS baseline survey were involved for further analysis. This study was approved by the Ethical Review Committee for Biomedical Research, School of Public Health, Sun Yat-sen University, and Ethics Committee of Guangzhou Centre for Disease Control and Prevention. It was conducted in accordance with the Declaration of Helsinki. Written informed consent was obtained from all participants.
Ascertainment of OSA
Obstructive sleep apnea was ascertained by Berlin Questionnaire (BQ) and STOP-BANG Questionnaire (SBQ), which are both widely used as screening tools for identifying OSA. The Chinese versions of both questionnaires had been proved to have superior predictive validity and reliability [24–27]. Berlin Questionnaire contains ten questions in three categories: snoring and cessation of breathing (category 1), symptoms of excessive daytime sleepiness (category 2), body mass index (BMI), and hypertension (category 3). High risk in category 1 and category 2 was defined by persistent symptoms (> 3–4 times/week) in at least two questions of each category, and high risk in category 3 was defined by the history of hypertension or BMI higher than 30 kg/m2. When two or more categories were considered positive, it indicated a high risk for OSA, otherwise a low-risk for OSA [28]. The SBQ included eight questions with dichotomous (yes/no) answers. The questions referred to snoring, fatigue, observed apnea, high blood pressure (or treatment for it), body mass index (BMI > 35 kg/m2), age (> 50 years old), neck circumference (> 40 cm), and gender (male). For each question, answering “yes” scores 1 and a “no” response scores 0. Subjects scoring three or more were considered to be at a high risk of OSA, otherwise were as at a low risk of OSA [24]. Then, participants who were assessed as having a high risk of OSA by both BQ and SBQ were classified into the OSA group, those who were assessed as having a lower risk of OSA by both BQ and SBQ were classified into the non-OSA group, and the remain participants were classified in the pre-OSA group.
Measurements of glycolipid biomarkers
Fasting blood samples were collected in the morning from each participant at the baseline survey and then detected within 4 h after collection in a qualified third-party medical laboratory (Guangzhou KingMed Diagnostics Group Co., Ltd). Fasting blood glucose (FBG) and serum lipids, including high-density lipoprotein cholesterol (HDL-CH), low-density lipoprotein cholesterol (LDC-CH), total cholesterol (TC), and triglyceride, were detected. 5% samples were randomly selected for parallel double-sample detection, and the results showed that the detection results were reliable.
Measurements of covariates
A face-to-face interview approach and a medical examination were adopted to collect information. A structured questionnaire was used to collect each participant’s social-demographic characteristics and lifestyle factors, including age, gender, marital status (married or others), educational status (primary school and lower, junior high school, senior high school, college and above), work intensity (light, moderate, vigorous, retirement), active smoking (never, occasional, frequent), passive smoking (yes or no), alcohol drinking (yes or no), fresh vegetable and fruit intake (< once/day or ≥ once/day). Leisure-time physical activity (LTPA) was assessed by a modified Global Physical Activity Questionnaire and the total volume of LTPA was calculated according to the method we reported [7, 22]. Personal history of chronic diseases, including hypertension, dyslipidemia, diabetes, chronic obstructive pulmonary disease (COPD), and cardiovascular diseases (CVDs) was collected. Hypertension was confirmed if the participant reported having physician-diagnosed hypertension, and/or had a mean systolic blood pressure (SBP) ≥ 140 mmHg, and/or mean diastolic blood pressure (DBP) ≥ 90 mmHg, and/or was on anti-hypertensive drugs [29]. A participant would be defined as dyslipidemia if he/she self-reported dyslipidemia diagnosed by a physician, or had serum cholesterol ≥ 5.2 mmol/l, LDC-CH ≥ 3.4 mmol/l, or TG ≥ 1.7 mmol/l [30]. A participant who had fasting plasma glucose ≥ 7.0 mmol/l and/or HbA1c ≥ 6.5%, and/or self-reported physician-diagnosed diabetes, and/or on diabetes treatment would be defined as having diabetes [31]. Height and weight were measured to calculate body mass index (BMI). Waist circumference and hip circumference were measured to calculate the waist-hip ratio (WHR). In addition, individual exposure to PM2.5 was assessed by 4-year average PM2.5 concentration from 2014 to 2017 within a 1,000 m circular buffer of each participant’s residential address. We obtained daily average PM2.5 data from 142 monitoring stations within or around Guangzhou City by the inverse distance weighting interpolation method [32].
Statistical analysis
The Kolmogorov–Smirnov test was used to test for normality. Continuous variables that were normally distributed are presented as mean and standard deviation (SD), otherwise were presented as median and interquartile range (IQR). Distributions of categorical variables were presented as frequencies and percentages. The distribution of demographic and socioeconomic characteristics, history of chronic diseases, and glycolipid biomarkers were described. Comparison of characteristics among the non-OSA group, the pre-OSA group, and the OSA group, was conducted by one-way analysis of variance (ANOVA) for continuous variable and Chi-square tests for categorical variable. Each glycolipid biomarker was transformed into a categorical variable by using quartile methods. Unadjusted and adjusted odds ratios (ORs) with 95% confidence intervals (CIs) were estimated by using multivariate logistic regression models to display the association between glycolipid biomarkers and the risk of OSA and pre-OSA. The linear exposure-response relationship was examined by putting the median of each quartile of exposure as a continuous variable in the model. Sensitivity analysis was carried out by excluding participants with COPD and CVDs. The associations between dyslipidemia and diabetes and risk of the OSA and pre-OSA were also assessed among overall participants and participants without COPD and CVDs, as dyslipidemia and diabetes were diseases resulting from dysfunction of glycolipids. All analyses were performed using the R software (version 3.6.1). All tests were two-sided and a p-value of less than 0.05 was considered to be statistically significant.
Results
Of the 10,826 participants included, 15.56% were categorized into the pre-OSA group, and 8.22% into the OSA group (Table 1). The proportion of males in the OSA group was 76.18% and was the highest among the three groups. Compared with participants without OSA, those with OSA were older and more likely to smoke frequently and drink alcohol, had higher levels of BMI [26.68 (3.98) kg/m2] and the waist-hip ratio [0.93 (0.06)], had a lower volume of LTPA [36.84 (34.77) MET-hours/week] and had a higher prevalence of dyslipidemia (77.19%) and diabetes (12.13%). The mean concentration of HDL-CH significantly decreased from 1.54 (0.43) mmol/L in the non-OSA group to 1.44 (0.41) mmol/L in the pre-OSA group and 1.39 (SD: 0.41) mmol/L in the OSA group. In contrast, the mean levels of LDL-CH, Triglyceride, and FBG increased in sequence from the non-OSA group, pre-OSA group, and OSA group (all p < 0.05).
The associations between glycolipid biomarkers and the risk of pre-OSA and OSA were presented in Table 2. Every 1 mmol/l increment of HDL-CH was associated with a decreased risk of both pre-OSA (OR: 0.82, 95% CI: 0.70–0.96) and OSA (OR: 0.63, 95% CI: 0.50–0.79) after adjustment for covariates. Compared with subjects within the lowest quartile of HDL-CH, the adjusted OR of pre-OSA and OSA in subjects within the highest quartile (>1.76 mmol/l) was 0.78 (95% CI: 0.65–0.94) and 0.59 (95% CI, 0.45–0.78), respectively; and a significant exposure-response trend was observed for both pre-OSA and OSA (both p < 0.05).
Conversely, when comparing the highest with the lowest quartile, triglyceride was associated with a 32% (OR: 1.32, 95% CI: 1.08–1.60) and a 41% (OR: 1.59, 95% CI: 1.18–2.07) increased risk of pre-OSA and OSA, separately; FBG was associated with a 37% (OR: 1.37, 95% CI: 1.13–1.67) and a 38% (OR: 1.38, 95% CI: 1.03–1.85) increased risk of pre-OSA and OSA, separately; significant exposure-response trends were also observed for pre-OSA and OSA (all p < 0.05). However, every 1 mmol/l increment of Triglyceride or FBG was associated with a slightly increased risk of pre-OSA and OSA, despite that the associations were nonsignificant. No significant association of hemoglobin, LDL-CH, or cholesterol with the risk of both pre-OSA and OSA was observed.
Besides, subjects with dyslipidemia had a higher risk of pre-OSA (OR 1.19, 95% CI 1.06–1.34) and OSA (OR 1.54, 95% CI 1.31–1.81) in the crude model. However, the association disappeared after adjusting for covariates (Table 3). Similarly, there was no significant association between diabetes and the risk of pre-OSA (OR 1.08, 95% CI 0.87–1.25) or OSA (OR 1.29, 95% CI 0.96–1.74) after adjustment for covariates.
The sensitivity analysis was conducted by excluding participants with COPD and CVDs, and similar results were obtained between five glycolipid biomarkers and risk of Pre-OSA and OSA (Table 4), and between dyslipidemia and diabetes and risk of Pre-OSA and OSA (Table 5).
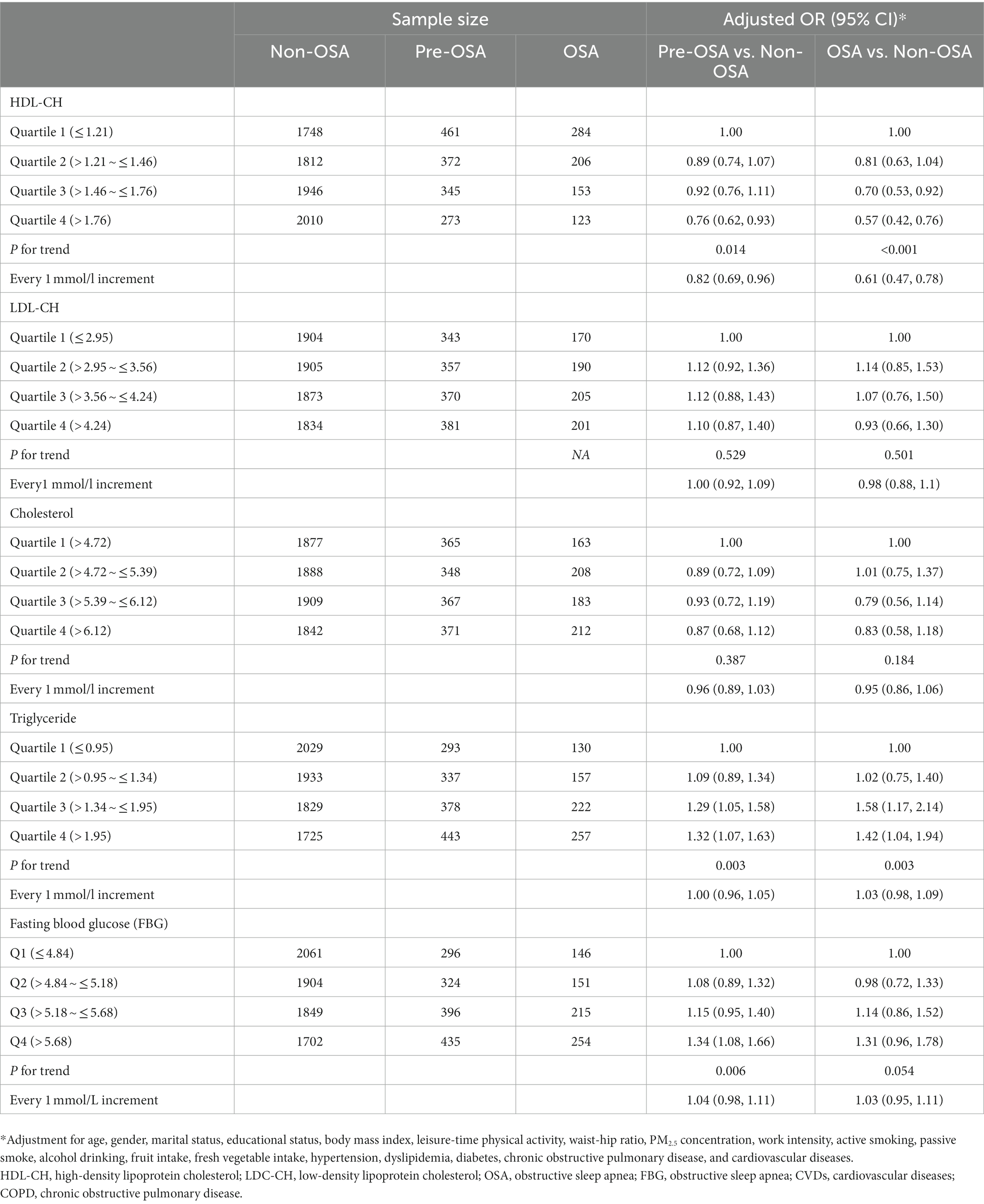
Table 4. Association between glycolipid biomarkers and OSA risk after excluding participants with COPD and CVDs.
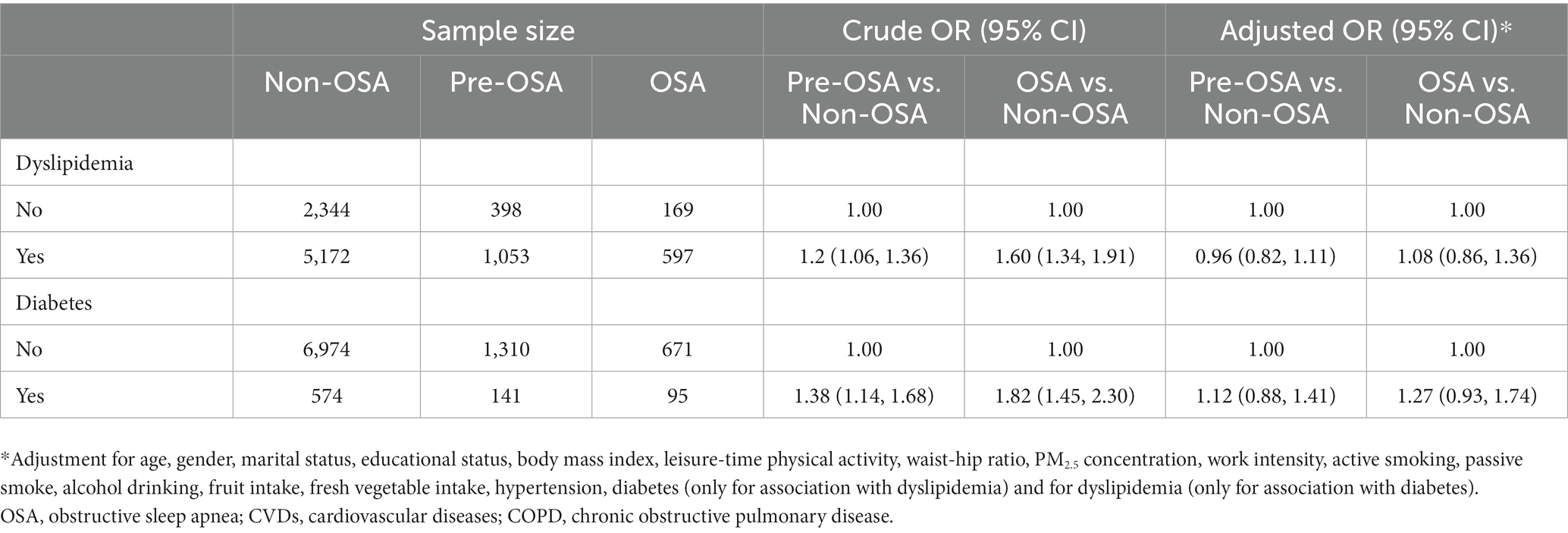
Table 5. Association between dyslipidemia and diabetes and risk of pre-OSA and OSA after excluding participants with COPD and CVDs.
Discussion
To our best knowledge, this is the first study to assess the association between glycolipid biomarkers and the risk of OSA identified by the combination of the Berlin Questionnaire and STOP-BANG Questionnaire in China. Given the difficulty of OSA diagnosis by polysomnography in a large-scale population [33], the use of double questionnaires could improve the specificity of OSA. This present study found that a higher level of HDL-CH was associated with a decreased risk of both pre-OSA and OSA, while triglyceride and FBG were positively associated with the risk of both pre-OSA and OSA. No significant association of LDL-CH, cholesterol, dyslipidemia, or diabetes with the risk of both pre-OSA and OSA was observed.
Metabolic abnormalities could increase the chance of upper airway collapsibility [8, 34]. Previous studies used serum lipid parameters to predict the risk of OSA, such as glucose [35], TG [9], and HDL-CH [14]. Novel composite parameters of glycolipids such as lipid accumulation product (LAP) also showed strong associations with OSA [36]. Some clinical OSA cohorts reported a positive association between TG and AHI [9, 37]. Moreover, a twin study analyzed the heritability of the relationship between OSA and hypertriglyceridemia and found that common genetic factors significantly determined the relationship between indices of chronic intermittent hypoxia and serum TG levels [38]. A genome-wide association study on the United Kingdom Biobank also indicated that genetically increased TG levels have independent causal effects on the risk of sleep apnea without the confounding effects of obesity [39]. Consistently, our study found similar results that triglyceride and fasting blood glucose were positively associated with the risk of both OSA and pre-OSA, whereas HDL-CH was negatively associated with the risk of both OSA and pre-OSA, further suggesting that regardless of the number of OSA-related symptoms or severity of OSA, glycolipid biomarkers may help determine risk and should be controlled during daily life. In this study, we took both BMI and WHR into consideration to exclude the confounding effect of peripheral and abdominal adiposity, which indicated that elevated level of HDL and TG was independently associated with OSA risk. Compared with peripheral obesity, abdominal obesity has a greater effect on upper airway function [40, 41].
In adult individuals, a large number of cross-sectional studies have shown independent associations between fasting levels of TC and the severity of OSA, particularly the frequency of intermittent hypoxic events [34]. Evidence from a Chinese large-scale cross-sectional study showed that, of the various components in serum lipid, only LDL-CH was independently associated with OSA [42]. However, we did not find a significant association of TC or LDL-CH with OSA risk. Furthermore, the results from our study were opposite to results from the ELSA-Brazil cohort study that drew the conclusion that OSA was independently associated with total cholesterol but not with HDL levels [37]. The conflicts may be connected with the reason that the possible association of TC and LDL-CH with OSA can be covered by comorbidities of OSA, such as hypertension and multiple cardiovascular diseases, due to their shared risk factors [34, 43]. Moreover, evidence showed that oxidized LDL (oxLDL) was associated with OSA. OxLDL is no longer recognized by cellular receptors with consequent inflammation and plaque formation on the internal surfaces of blood vessels [44]. This could be due to the fact that LDL particles are not removed by the liver and peripheral cells due to the depletion of LDL receptor-related protein-1 (LRP-1) in OSA [45]. In addition, emerging randomized trials for patients with OSA found that plasma levels of lipid biomarkers were reversed by CPAP treatment, which suggests causality [34]. Taking these elements together, we found that pre-OSA and OSA were associated with HDL and TG levels, but not with dyslipidemia. The possible explanation may be that even a minor change of blood lipid can initiate the pathologic development of OSA, and this change does not necessarily depend on the presence of specific symptoms, whereas dyslipidemia is widely believed to be closely associated with OSA [8, 9].
Noticeably, we did not find any association of diabetes with pre-OSA risk and OSA risk. Nevertheless, we found that the highest quantile of blood glucose (> 5.68 mmol/l) was linked with an increased risk of pre-OSA and OSA. Although diabetes was commonly assessed by fasting blood glucose higher than ≥7.0 mmol/l, our findings suggested that even a lower level of FBG could lead to a higher risk of pre-OSA and OSA. OSA is commonly considered as frequent comorbidity in patients with type 2 diabetes, and cardinal features of OSA, including intermittent hypoxemia and sleep fragmentation, have been linked to abnormal glucose metabolism in laboratory-based experiments [46]. The relationship between OSA and type 2 diabetes may be bidirectional in nature given that diabetic neuropathy can affect the central control of respiration and upper airway neural reflexes, promoting sleep-disordered breathing [46]. Early attention to individual blood glucose levels may have significant preventive implications for reducing OSA prevalence.
Our study has some strengths. First, we conjunctively used the Berlin Questionnaire and the STOP-BANG Questionnaire, two widely used questionnaires with high validity and reliability, to identify OSA statuses, which enhanced the screening specificity. Second, we studied the association between several glycolipid biomarkers and the risk of pre-OSA and OSA among a representative population in Guangdong, which attenuated selection bias. Third, we took a large number of confounding factors into account, including PM2.5 exposure, individual lifestyles, and abdominal adiposity indicator, which could eliminate confounders to a large degree and reveal the independent associations between those indicators and OSA. Finally, sensitivity analyses yielded similar results, demonstrating the robustness of our findings.
However, there are also some limitations. First, we used questionnaires to define OSA rather than overnight polysomnography, for it was difficult to implement in a large-scale population study. Nevertheless, the questionnaires used in this study showed high validity and reliability in predicting OSA [26, 27] and were commonly implemented in previous studies [26, 27, 47]. Second, the nature of a cross-sectional study could not support causality inference. Since OSA often coexists with various chronic diseases such as hyperlipidemia, diabetes, and cardiovascular disease, or acts as an intermediate link in the occurrence and development of these diseases, it is difficult to clarify the mechanism of glucose and lipid metabolism and OSA. Further studies with longitudinal design are needed to confirm this relationship.
Conclusion
The findings suggest that the level of HDL-CH was inversely associated with OSA risk, while elevated serum triglyceride and FBG could increase the risk of OSA. Healthy glycolipid metabolism warrants more attention in the field of OSA prevention, and more reports from rigorous longitudinal studies are expected.
Data availability statement
The raw data supporting the conclusions of this article will be made available by the authors, without undue reservation.
Ethics statement
The studies involving human participants were reviewed and approved by the Ethical Review Committee for Biomedical Research, School of Public Health, Sun Yat-sen University, and Ethics Committee of Guangzhou Centre for Disease Control and Prevention. The patients/participants provided their written informed consent to participate in this study.
Author contributions
XL and HD conceived and designed the study. HD, HZ, MZ, GS, JC, XW, SR, JH, and XL collected the data. MZ and HZ analyzed the data. MZ, XD, and WS drafted the manuscript. XL, HZ, HD, JH, and WZ reviewed and edited the manuscript. All authors contributed to the article and approved the submitted version.
Funding
This work was supported by the Guangdong Basic and Applied Basic Research Foundation (No. 2022A1515010686), the Medical Science and Technology Research Foundation of Guangdong Province (No. A2023408), the Guangdong Provincial Key R&D Program (No.2019B020230004) and the National Key R&D Program of China (No.2018YFC1312502). The founder had no role in the design, analysis, or writing of this manuscript.
Acknowledgments
The authors are grateful to epidemiologists, nurses, and doctors in Guangdong Provincial People’s Hospital, Guangzhou Center for Disease Control and Prevention, and community healthcare centers for data collection, and appreciate all study subjects for their participation.
Conflict of interest
The authors declare that the research was conducted in the absence of any commercial or financial relationships that could be construed as a potential conflict of interest.
Publisher’s note
All claims expressed in this article are solely those of the authors and do not necessarily represent those of their affiliated organizations, or those of the publisher, the editors and the reviewers. Any product that may be evaluated in this article, or claim that may be made by its manufacturer, is not guaranteed or endorsed by the publisher.
References
1.Jordan, AS, McSharry, DG, and Malhotra, A. Adult obstructive sleep apnoea. Lancet. (2014) 383:736–47. doi: 10.1016/S0140-6736(13)60734-5
2.Punjabi, NM. The epidemiology of adult obstructive sleep apnea. Proc Am Thorac Soc. (2008) 5:136–43. doi: 10.1513/pats.200709-155MG
3.Benjafield, AV, Ayas, NT, Eastwood, PR, Heinzer, R, Ip, MSM, Morrell, MJ, et al. Estimation of the global prevalence and burden of obstructive sleep apnoea: a literature-based analysis. Lancet Respir Med. (2019) 7:687–98. doi: 10.1016/S2213-2600(19)30198-5
4.Peppard, PE, Young, T, Barnet, JH, Palta, M, Hagen, EW, and Hla, KM. Increased prevalence of sleep-disordered breathing in adults. Am J Epidemiol. (2013) 177:1006–14. doi: 10.1093/aje/kws342
5.Arnaud, C, Bochaton, T, Pépin, JL, and Belaidi, E. Obstructive sleep apnoea and cardiovascular consequences: pathophysiological mechanisms. Arch Cardiovasc Dis. (2020) 113:350–8. doi: 10.1016/j.acvd.2020.01.003
6.Andrade, AG, Bubu, OM, Varga, AW, and Osorio, RS. The relationship between obstructive sleep apnea and Alzheimer's disease. J Alzheimers Dis. (2018) 64:S255–s270. doi: 10.3233/JAD-179936
7.Duan, X, Zheng, M, He, S, Lao, L, Huang, J, Zhao, W, et al. Association between physical activity and risk of obstructive sleep apnea. Sleep Breath. (2021) 25:1925–34. doi: 10.1007/s11325-021-02318-y
8.Castaneda, A, Jauregui-Maldonado, E, Ratnani, I, Varon, J, and Surani, S. Correlation between metabolic syndrome and sleep apnea. World J Diabetes. (2018) 9:66–71. doi: 10.4239/wjd.v9.i4.66
9.Kim, DH, Kim, B, Han, K, and Kim, SW. The relationship between metabolic syndrome and obstructive sleep apnea syndrome: a nationwide population-based study. Sci Rep. (2021) 11:8751. doi: 10.1038/s41598-021-88233-4
10.Dehelean, L, Sarbu, M, Petrut, A, and Zamfir, AD. Trends in glycolipid biomarker discovery in neurodegenerative disorders by mass spectrometry. Adv Exp Med Biol. (2019) 1140:703–29. doi: 10.1007/978-3-030-15950-4_42
11.Li, G, Li, L, Joo, EJ, Son, JW, Kim, YJ, Kang, JK, et al. Glycosaminoglycans and glycolipids as potential biomarkers in lung cancer. Glycoconj J. (2017) 34:661–9. doi: 10.1007/s10719-017-9790-7
12.Almendros, I, and García-Río, F. Sleep apnoea, insulin resistance and diabetes: the first step is in the fat. Eur Respir J. (2017) 49:1700179. doi: 10.1183/13993003.00179-2017
13.Meszaros, M, and Bikov, A. Obstructive sleep Apnoea and lipid metabolism: the summary of evidence and future perspectives in the pathophysiology of OSA-associated Dyslipidaemia. Biomedicine. (2022) 10:2754. doi: 10.3390/biomedicines10112754
14.Karadeniz, Y, Onat, A, Akbaş, T, Şimşek, B, Yüksel, H, and Can, G. Determinants of obstructive sleep apnea syndrome: pro-inflammatory state and dysfunction of high-density lipoprotein. Nutrition. (2017) 43-44:54–60. doi: 10.1016/j.nut.2017.06.021
15.Sertogullarindan, B, Komuroglu, AU, Ucler, R, Gunbatar, H, Sunnetcioglu, A, and Cokluk, E. Betatrophin association with serum triglyceride levels in obstructive sleep apnea patients. Ann Thorac Med. (2019) 14:63–8. doi: 10.4103/atm.ATM_52_18
16.Patel, SR. Obstructive sleep apnea. Ann Intern Med. (2019) 171:Itc81–Itc96. doi: 10.7326/AITC201912030
17.Drager, LF, Santos, RB, Silva, WA, Parise, BK, Giatti, S, Aielo, AN, et al. OSA, short sleep duration, and their interactions with sleepiness and Cardiometabolic risk factors in adults: the ELSA-Brasil study. Chest. (2019) 155:1190–8. doi: 10.1016/j.chest.2018.12.003
19.Zhao, X, Li, X, Xu, H, Qian, Y, Fang, F, Yi, H, et al. Relationships between cardiometabolic disorders and obstructive sleep apnea: implications for cardiovascular disease risk. J Clin Hypertens (Greenwich). (2019) 21:280–90. doi: 10.1111/jch.13473
20.Drager, LF, Lopes, HF, Maki-Nunes, C, Trombetta, IC, Toschi-Dias, E, Alves, MJNN, et al. The impact of obstructive sleep apnea on metabolic and inflammatory markers in consecutive patients with metabolic syndrome. PLoS One. (2010) 5:e12065. doi: 10.1371/journal.pone.0012065
21.Deng, H, Guo, P, Zheng, M, Huang, J, Xue, Y, Zhan, X, et al. Epidemiological characteristics of atrial fibrillation in southern China: results from the Guangzhou heart study. Sci Rep. (2018) 8:17829. doi: 10.1038/s41598-018-35928-w
22.Duan, X, Huang, J, Zheng, M, Zhao, W, Lao, L, Li, H, et al. Association of healthy lifestyle with risk of obstructive sleep apnea: a cross-sectional study. BMC Pulm Med. (2022) 22:33. doi: 10.1186/s12890-021-01818-7
23.Duan, X, Zheng, M, Zhao, W, Huang, J, Lao, L, Li, H, et al. Associations of depression, anxiety, and life events with the risk of obstructive sleep apnea evaluated by Berlin questionnaire. Front Med. (2022) 9:9. doi: 10.3389/fmed.2022.799792
24.Chung, F, Abdullah, HR, and Liao, P. STOP-Bang questionnaire: a practical approach to screen for obstructive sleep apnea. Chest. (2016) 149:631–8. doi: 10.1378/chest.15-0903
25.Chiu, HY, Chen, PY, Chuang, LP, Chen, NH, Tu, YK, Hsieh, YJ, et al. Diagnostic accuracy of the Berlin questionnaire, STOP-BANG, STOP, and Epworth sleepiness scale in detecting obstructive sleep apnea: a bivariate meta-analysis. Sleep Med Rev. (2017) 36:57–70. doi: 10.1016/j.smrv.2016.10.004
26.Hu, YY, Yu, Y, Wang, ZB, Liu, C, Cui, YH, and Xiao, WM. Reliability and validity of simplified Chinese STOP-BANG questionnaire in diagnosing and screening obstructive sleep apnea hypopnea syndrome. Curr Med Sci. (2019) 39:127–33. doi: 10.1007/s11596-019-2010-x
27.Ha, SC, Lee, DLY, Abdullah, VJ, and van Hasselt, CA. Evaluation and validation of four translated Chinese questionnaires for obstructive sleep apnea patients in Hong Kong. Sleep Breath. (2014) 18:715–21. doi: 10.1007/s11325-013-0889-1
28.Tan, A, Yin, JD, Tan, LW, van Dam, R, Cheung, YY, and Lee, CH. Using the Berlin questionnaire to predict obstructive sleep apnea in the general population. J. Clin. Sleep Med. (2017) 13:427–32. doi: 10.5664/jcsm.6496
29.National High Blood Pressure Education, P. The Seventh Report of the Joint National Committee on Prevention, Detection, Evaluation, and Treatment of High Blood Pressure. Bethesda (MD): National Heart, Lung, and Blood Institute (US) (2004).
30.Joint Committee Issued Chinese Guideline for the Management of Dyslipidemia in Adults. [2016 Chinese guideline for the management of dyslipidemia in adults]. Zhonghua Xin Xue Guan Bing Za Zhi. (2016) 44:833–853. doi: 10.3760/cma.j.issn.0253-3758.2016.10.005
31.American Diabetes Association. Standards of medical care in diabetes–2010. Diabetes Care. (2010) 33:S11–61. doi: 10.2337/dc10-S011
32.Xu, R, Tian, Q, Lu, W, Yang, Z, Ye, Y, Li, Y, et al. Association of short-term exposure to air pollution with recurrent ischemic cerebrovascular events in older adults. Int J Hyg Environ Health. (2022) 240:113925. doi: 10.1016/j.ijheh.2022.113925
33.Barros, D, and García-Río, F. Obstructive sleep apnea and dyslipidemia: from animal models to clinical evidence. Sleep. (2019) 42:zsy236. doi: 10.1093/sleep/zsy236
34.Gileles-Hillel, A, Kheirandish-Gozal, L, and Gozal, D. Biological plausibility linking sleep apnoea and metabolic dysfunction. Nat Rev Endocrinol. (2016) 12:290–8. doi: 10.1038/nrendo.2016.22
35.Xu, H, Zhao, X, Shi, Y, Li, X, Qian, Y, Zou, J, et al. Development and validation of a simple-to-use clinical nomogram for predicting obstructive sleep apnea. BMC Pulm Med. (2019) 19:18. doi: 10.1186/s12890-019-0782-1
36.Bikov, A, Frent, S, Reisz, D, Negru, A, Gaita, L, Breban Schwarzkopf, D, et al. Comparison of composite lipid indices in patients with obstructive sleep Apnoea. Nat Sci Sleep. (2022) 14:1333–40. doi: 10.2147/NSS.S361318
37.Silva, WA, Almeida-Pititto, B, Santos, RB, Aielo, AN, Giatti, S, Parise, BK, et al. Obstructive sleep apnea is associated with lower adiponectin and higher cholesterol levels independently of traditional factors and other sleep disorders in middle-aged adults: the ELSA-Brasil cohort. Sleep Breath. (2021) 25:1935–44. doi: 10.1007/s11325-021-02290-7
38.Meszaros, M, Tarnoki, AD, Tarnoki, DL, Kovacs, DT, Forgo, B, Lee, J, et al. Obstructive sleep apnea and hypertriglyceridaemia share common genetic background: results of a twin study. J Sleep Res. (2020) 29:e12979. doi: 10.1111/jsr.12979
39.Tang, H, Zhou, Q, Zheng, F, Wu, T, Tang, YD, and Jiang, J. The causal effects of lipid profiles on sleep apnea. Front Nutr. (2022) 9:9. doi: 10.3389/fnut.2022.910690
40.Schwab, RJ, Pasirstein, M, Pierson, R, Mackley, A, Hachadoorian, R, Arens, R, et al. Identification of upper airway anatomic risk factors for obstructive sleep apnea with volumetric magnetic resonance imaging. Am J Respir Crit Care Med. (2003) 168:522–30. doi: 10.1164/rccm.200208-866OC
41.Bikov, A, Losonczy, G, and Kunos, L. Role of lung volume and airway inflammation in obstructive sleep apnea. Respir Investig. (2017) 55:326–33. doi: 10.1016/j.resinv.2017.08.009
42.The Shanghai Sleep Health Study Research GroupXu, H, Guan, J, Yi, H, Zou, J, Meng, L, et al. Elevated low-density lipoprotein cholesterol is independently associated with obstructive sleep apnea: evidence from a large-scale cross-sectional study. Sleep Breath. (2016) 20:627–34. doi: 10.1007/s11325-015-1262-3
43.Drager, LF, McEvoy, R, Barbe, F, Lorenzi-Filho, G, and Redline, S, INCOSACT Initiative (International Collaboration of Sleep Apnea Cardiovascular Trialists). Sleep apnea and cardiovascular disease: lessons from recent trials and need for team science. Circulation. (2017) 136:1840–50. doi: 10.1161/CIRCULATIONAHA.117.029400
44.Feres, MC, Fonseca, FAH, Cintra, FD, Mello-Fujita, L, de Souza, AL, de Martino, MC, et al. An assessment of oxidized LDL in the lipid profiles of patients with obstructive sleep apnea and its association with both hypertension and dyslipidemia, and the impact of treatment with CPAP. Atherosclerosis. (2015) 241:342–9. doi: 10.1016/j.atherosclerosis.2015.05.008
45.Meszaros, M, Kunos, L, Tarnoki, AD, Tarnoki, DL, Lazar, Z, and Bikov, A. The role of soluble low-density lipoprotein receptor-related Protein-1 in obstructive sleep Apnoea. J Clin Med. (2021) 10:1494. doi: 10.3390/jcm10071494
46.Reutrakul, S, and Mokhlesi, B. Obstructive sleep apnea and diabetes: a state of the art review. Chest. (2017) 152:1070–86. doi: 10.1016/j.chest.2017.05.009
Keywords: lipid, blood glucose, obstructive sleep apnea, risk factor, biomarker
Citation: Zheng M, Duan X, Zhou H, Sun W, Sun G, Chen J, Wu X, Rong S, Huang J, Zhao W, Deng H and Liu X (2023) Association between glycolipids and risk of obstructive sleep apnea: A population-based study. Front. Nutr. 10:974801. doi: 10.3389/fnut.2023.974801
Edited by:
Huajun Xu, Shanghai Jiao Tong University, ChinaReviewed by:
Babak Amra, Isfahan University of Medical Sciences, IranChenyang Li, Shanghai Jiao Tong University, China
András Bikov, The University of Manchester, United Kingdom
Copyright © 2023 Zheng, Duan, Zhou, Sun, Sun, Chen, Wu, Rong, Huang, Zhao, Deng and Liu. This is an open-access article distributed under the terms of the Creative Commons Attribution License (CC BY). The use, distribution or reproduction in other forums is permitted, provided the original author(s) and the copyright owner(s) are credited and that the original publication in this journal is cited, in accordance with accepted academic practice. No use, distribution or reproduction is permitted which does not comply with these terms.
*Correspondence: Hai Deng, doctordh@hotmail.com; Xudong Liu, xdliu.cn@hotmail.com; Wengjing Zhao, zhaowj@sustech.edu.cn
†These authors have contributed equally to this work