- 1Graduate School, Beijing University of Chinese Medicine, Beijing, China
- 2Department of Pediatrics, China-Japan Friendship Hospital, Beijing, China
- 3Center for Evidence-Based Medicine, Capital Institute of Pediatrics, Beijing, China
- 4International Medical Services, China-Japan Friendship Hospital, Beijing, China
Background: Child malnutrition places a major burden on global public health. We aimed to estimate the prevalence of child malnutrition and identify its potential factors among children aged 3–14 years from Beijing and Tangshan.
Methods: We cross-sectionally recruited 18,503 children aged 3–14 years from September 2020 to January 2022, according to a stratified cluster random sampling strategy. Child malnutrition was defined according to the World Health Organization criteria. Data were analyzed by STATA software and R language.
Results: The prevalence of malnutrition among 18,503 children was 10.93%. After multivariable adjustment, seven factors significantly associated with child malnutrition were parental education (adjusted odds ratio, 95% confidence interval, p: 1.52, 1.40 to 1.67, <0.001), family income (1.23, 1.16 to 1.30, <0.001), fast food intake frequency (1.14, 1.06 to 1.21, <0.001), night meals intake frequency (1.09, 1.04 to 1.15, <0.001), eating speed (1.01, 1.01 to 1.02, <0.001), maternal obesity (0.97, 0.95 to 0.99, <0.001), and paternal obesity (0.97, 0.96 to 0.98, <0.001). The seven significant factors had better prediction performance (area under the receiver operating characteristic, 0.956) for child malnutrition.
Conclusion: Approximately 10% of Chinese children aged 3–14 years were in malnutrition status, and seven factors were found to be significant predictors for child malnutrition.
Introduction
Child malnutrition, including stunting, wasting, and being underweight, is featured by inadequate or unbalanced intake of protein energy or nutrients, and it represents a public health issue, deserving special awareness (1). Statistics from the fund of the United Nations Children (UNICEF) and World Health Organization (WHO) show that 22.0% and 6.7% of children younger than 5 years were separately affected by stunting and wasting worldwide (2). In China, the prevalence of underweight and stunting in children younger than 5 years was estimated to be 3.6 and 9.9%, respectively (3), and the prevalence of malnutrition in 7–18-year-old children was 8.6% (4). The detrimental impact of child malnutrition is manifold because it can not only threaten survival, development, and growth of children but also undermine economic growth and national development (5). The causes of child malnutrition are complex, and no consensus has thus far been reached on its risk profiling.
It is widely accepted that besides factors relating to inheritance and diseases, child malnutrition is mainly caused by inadequate nutrient intake. Over the past decades, dietary patterns and modern lifestyles have undergone profound changes, along with the intensification of industrialization and urbanization in China (6), and we are experiencing significant public health challenges (7). Due to inadequate intake of vegetables and fruits, as well as excessive intake of red meat, oil, and salt, nutrition deficiency and nutrition imbalance have aroused extensive concerns (8, 9). There is evidence for the significant influence of parental dieting behavior affected by rhythm of life, family income and education on child dieting behavior, and weight status (10). Hence, identifying potential factors underlying child malnutrition to facilitate the development of personalized intervention and follow-up care is urgently needed. Some factors, such as parental obesity and eating habits, were reported to be associated with child malnutrition (11–13), whereas the majority of these association studies focused on only a few factors and were largely underpowered to provide reliable estimates.
To fill this gap in knowledge, a large cross-sectional survey of children of 3–14 years of age from Beijing and Tangshan estimated the prevalence of child malnutrition and identified factors that are independently associated with the risk of child malnutrition.
Methods
Study design
We conducted two cross-sectional surveys from September 2020 to January 2022. The first survey was performed in Beijing and Tangshan (in Hebei province) from September to December 2020, and the second in January 2022 in Pinggu district, Beijing.
According to the 2022 census data, there are approximately 21.8 million persons in Beijing and 7.7 million persons in Tangshan. There are 16 districts in Beijing and 7 districts in Tangshan. Beijing covers an area of 16,410 km2, and Tangshan covers an area of 13,472 km2. Per capita disposable income was 77,400 RMB in Beijing and 39,600 RMB in Tangshan.
Study participants
The selection process of study participants was reported previously (14, 15). Specifically, in the first survey, study participants were consisted of preschool-aged children attending junior to senior kindergarten classes. We randomly selected 4 out of 16 districts in Beijing and 2 out of 7 districts in Tangshan by using the stratified and cluster sampling methods. In total, 5 kindergartens were selected from each district, and 30 kindergartens were included finally. In the second survey, study participants included children attending primary school or junior high school. We randomly selected 26 schools, including 8 primary schools and 18 junior high schools, in Pinggu district, Beijing. In this study, data were collected from children aged 3–14 years in 30 kindergartens and 26 schools in Beijing and Tangshan.
Data collection
We collected data by self-designed questionnaires. To ensure reliability and validity, both questionnaires were separately distributed in 200 samples before formal circulation, and the reliability coefficient (alpha) was over 0.85.
We integrated the items from both questionnaires, covering four main areas as follows: (i) demographic area: age, sex, nationality, date of birth, height, weight, and food and drug allergy; (ii) fetal and neonatal area: birth body length, gestational age, delivery mode, pregnancy order, delivery order, assisted reproduction, twin birth, infancy feeding, breastfeeding duration, and time of adding solid food; (iii) lifestyle-related area: sitting time, screen time, outdoor activity time, sleep duration, fall asleep time, eating speed, number of dental caries, and weekly intake frequencies of sweet food, night meals, and fast food; (iv) family-related area: parental age, parental weight, parental height, parental education, and family income. Body mass index (BMI) was calculated as weight divided by height squared (kg/m2).
The questionnaires were generated by the “Wenjuanxing” website.1 As an online platform in China, the “Wenjuanxing” can yield a unique QR code for each questionnaire. The QR code can be recognized by smartphones and was sent to the parents or guardians by teachers-in-charge via the “WeChat” social media application. The “Wenjuanxing” platform automatically integrated data from individual questionnaires as an Excel spreadsheet, which can be downloaded for analysis.
Quality control
Before the distribution of questionnaires, healthcare physicians and teachers-in-charge selected in this survey from all schools and kindergartens were trained to be familiar with the survey procedures and each item in the questionnaires. During the survey, healthcare physicians and teachers-in-charge can help parents or guardians of participating children to fill out questionnaires. At the end of the survey, data were downloaded into a Microsoft Office Excel™ spreadsheet from the “Wenjuanxing” platform and were strictly checked by our trained staff. Healthcare physicians and teachers-in-charge were requested to contact the parents or guardians of participating children to resupply or confirm information that was obviously abnormal in the questionnaires. Body weight (to the nearest 0.1 kg) and height (to the nearest 0.1 cm) were measured by healthcare physicians.
Definitions of stunting, underweight, wasting, and malnutrition
Child malnutrition included stunting, underweight, and wasting. In this study, we adopted the WHO criteria to define child malnutrition. Specifically, the 2006 WHO Child Growth Standard (16) defined stunting, underweight, and wasting for children aged 0–5 years (0–60 months), and the 2007 WHO growth reference for school-aged children and adolescents (17) defined stunting and wasting for children aged 5–19 years (61–228 months). The nutritional status of a child was evaluated by the Z-score, which was calculated using the deviation of the value of a child from the median of the reference population, divided by the standard deviation of the reference population. According to the WHO criteria, we defined stunting, underweight, and wasting based on two age stages: (i) children aged 36–60 months: stunting was defined as a height-for-age Z-score (HAZ) less than −2 standard deviations (SDs), underweight as weight-for-age Z-score (WAZ) of less than −2 SDs, and wasting as weight-for-height Z-score (WHZ) less than −2 SDs; (ii) children aged 61–180 months: stunting was defined as a height-for-age Z-score (HAZ) less than −2 standard deviations (SDs) and BMI for age Z-score (BMIZ) less than −2 SDs.
Definitions of other items
Delivery modes included vaginal delivery and cesarean section. Gestational age was recorded in months, and birth between 37 and 42 gestational weeks was regarded as full-term birth. Birth body length (to the nearest 0.1 cm) was reported by the parents or guardians of participating children. Pregnancy order and delivery order meant the times of pregnancy and bearing birth, respectively.
Infancy feeding included pure breastfeeding, partial breastfeeding, and non-breastfeeding. Breastfeeding duration and time of adding solid food were recorded in months. Some lifestyle-related factors, including sitting time, screen time, and outdoor activity time recorded in hours, were calculated as the sum of time spent on workdays × 5 and weekends × 2 divided by 7. In this survey, sitting time included screen time, and screen time referred to the amount of time spent on screen-related behaviors such as watching TV and playing computer games (18). Sleep duration included time for a lunch break. Eating speed was calculated as the average of breakfast, lunch, and dinner. Sweet food refers to food with sweet taste (such as bread cakes and desserts), and fast food is defined as food with high energy and low nutrition, such as hamburger and pizza. Night meal refers to food eaten within 2 h before bedding. We investigated the weekly intake frequency, which was classified as every day, often (3–5 times), occasional (1–2 times), and none, of sweet food, fast food, and night meals.
Parental BMI was calculated from self-reported weight and height. Family education referred to the highest educational level of parents and was categorized as master’s degree or above, bachelor’s degree, and high school degree or below. Family income (RMB per year) was categorized as ≥300,000, 100,000–300,000, and < 100,000.
Statistical analyses
Data were analyzed using STATA software version 16.0 (Stata Corp, College Station, TX, United States) and R programming environment (version 3.5.2). Study power was calculated using PS (Power and Sample Size Calculations) software version 3.0.
We assign values to categorical variables, as shown in Supplementary Table S1. The possible biases arising from different kindergartens and schools were assessed by the intraclass correlation coefficient (ICC). In theory, ICC can be used to quantify observed differences within and between clusters (19).
To reduce the influence of potential bias and confounding, we adopted 1:4 propensity score matching (PSM) between the malnutrition and control groups. Continuous variables are expressed as mean (standard deviation), in the case of no deviation from normal distribution based on the Skewness and Kurtosis tests, and as median (interquartile range). Categorical variables are expressed as count (percent). Between-group comparisons of variables were performed using the t-test, χ2 test, or rank-sum test, where appropriate. The variance inflation factor (VIF) was calculated to assess multiple collinearities.
To identify statistically significant risk factors for child malnutrition, logistic regression analyses were conducted before and after considering age, sex, twins and infancy feeding, birth length, pregnancy order, and delivery order. Effect-size estimates are expressed as odds ratio (OR) and 95% confidence interval (CI).
After adding significant factors associated with child malnutrition to the basic model, prediction performance was appraised from both calibration and discrimination aspects. From the calibration aspect, Akaike information criterion (AIC) and Bayesian information criterion (BIC) were used to observe how closely the prediction probability, by adding significant factors, reflected the actual observed risk and global fit of the modified risk model, and the calibration curves for different models provide another form of visualization. From the discrimination aspect, the receiver operating characteristic (ROC) curve of both models was also presented to observe the discrimination capability of identified significant factors. The net benefits of this addition were also inspected by decision curve analysis (DCA).
Results
Baseline characteristics
In the first survey from September to December 2020, questionnaires were distributed to the parents or guardians of 10,441 children. In the second survey in January 2022, 11,633 questionnaires were sent. We pooled data from both surveys and strictly reviewed the validity of the survey data. Finally, 18,503 questionnaires were deemed eligible for inclusion. In this study, the prevalence of child malnutrition was 10.93% (n = 2022), and stunting, underweight, and wasting were 1.34% (n = 248), 11.22% (n = 563), and 9.77% (n = 1808), respectively.
The baseline characteristics of participating children are shown in Table 1 and Supplementary Table S2. The ICCs for all factors under the study were all relatively low (<0.01), indicating a low probability of clustering within kindergartens or schools and a lower likelihood of differences in surveyed items (Supplementary Table S3).
Identification of potential factors for child malnutrition
Multiple collinearities revealed by variance inflation factor (VIF) indicated that no multiple collinearities existed between different variables (Supplementary Table S4).
As shown in Table 1, baseline variables were selected based on clinical relevance or with a p-value of less than 0.05 on univariate analyses. After adjusting for age, gender, twins and infancy feeding, birth length, pregnancy order, and delivery order, seven factors, namely, family education (OR, 95% CI, p: 1.52, 1.40 to 1.67, <0.001), family income (1.23, 1.16 to 1.30, <0.001), fast food intake frequency (1.14, 1.06 to 1.21, <0.001), night meals intake frequency (1.09, 1.04 to 1.15, <0.001), eating speed (1.01, 1.01 to 1.02, <0.001), maternal BMI (0.97, 0.95 to 0.99, <0.001), and paternal BMI (0.97, 0.96 to 0.98, <0.001) were found to be associated with the significant risk of child malnutrition, as shown in Table 2.
The power to detect significance when estimating the risk for child malnutrition was over 80% for the above comparisons.
Prediction performance assessment
We constructed both the basic model and full models to assess the prediction performance of seven significant factors identified above. The full model included all variables, and the basic model included all variables with the exception of seven significant factors. The prediction performance of both models was assessed from calibration and discrimination aspects, and the difference between the basic model and full model was compared. Significant improvement was observed in the prediction accuracy of the full model relative to that of the basic model (Table 3). In addition, the DCA plot showed that the net benefits gained by adding seven significant factors to the basic model were obvious (Figure 1).
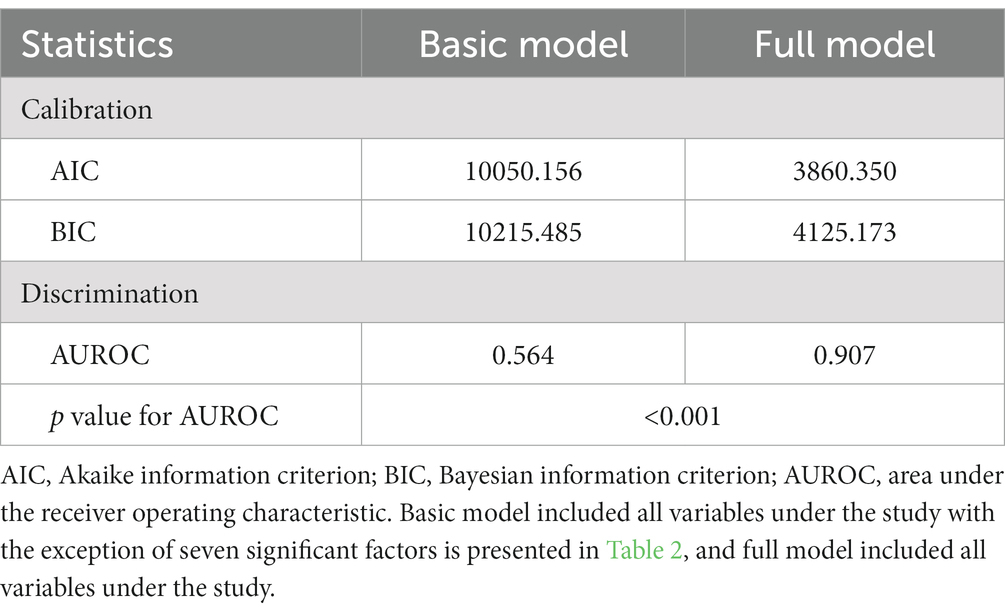
Table 3. Prediction performance before and after adding seven significant factors identified for child malnutrition.
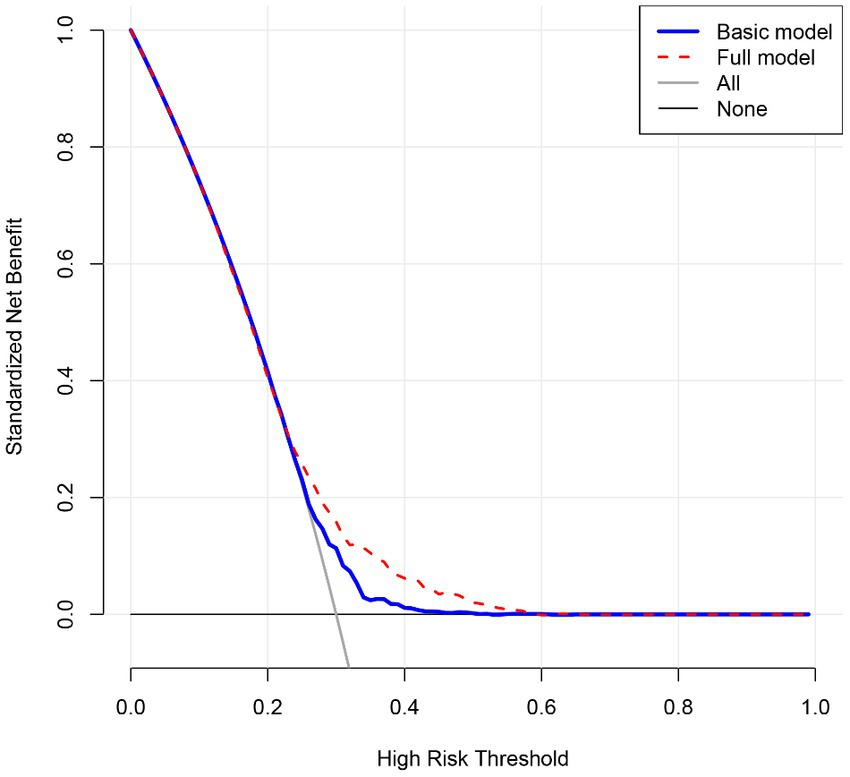
Figure 1. Decision curve analysis on the net benefits gained by adding seven significant factors associated with child malnutrition. Basic model included all variables under study with the exception of seven significant factors is presented in Table 2, and full model included all variables under the study.
The ROC curves are shown in Figure 2, and calibration curves are shown in Supplementary Figure S1. As revealed by the area under the receiver operating characteristic (AUROC) curve, both models differed significantly in discrimination (p < 0.001).
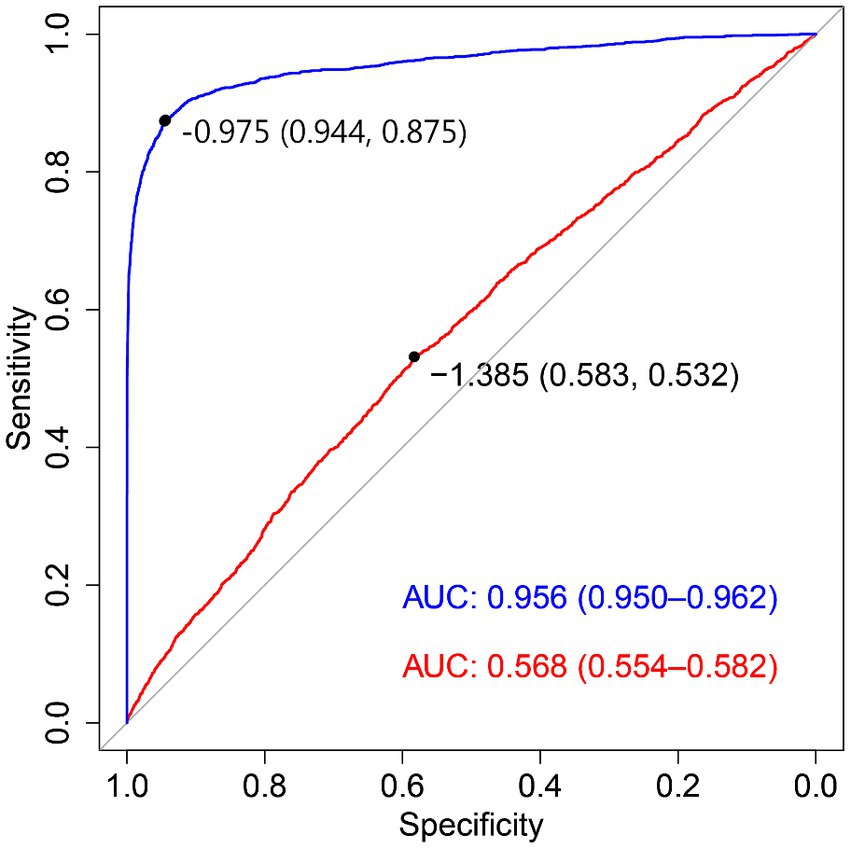
Figure 2. Receiver operating characteristic (ROC) curve for both basic and full models. AUC: area under curve. The red solid line corresponds to basic model, and the blue solid line corresponds to full model. Basic model included all variables with the exception of seven significant factors is presented in Table 2, and full model included all variables under the study.
Discussion
In this study, we aimed to estimate the prevalence of child malnutrition and identify its potential factors among 18,503 Chinese children aged 3–14 years from Beijing and Tangshan. The key finding of this study was that approximately one in ten children suffered from malnutrition in North China. Moreover, parental education, family income, fast food intake frequency, night meals intake frequency, eating speed, and parental obesity were significantly and independently associated with child malnutrition under the WHO criteria. Thus far, to the best of our knowledge, this is the first study that has explored the risk profiling of child malnutrition among Chinese preschool and school-age children.
Recently, the overall detection rate of malnutrition has shown a downward trend (4, 20, 21). As reflected in this study, approximately 10% of Chinese children aged 3–14 years was in malnutrition status (stunting: 1.34%, underweight: 11.22%, and wasting: 9.77%), which was higher than that released in 2019 among Chinese children aged 7–18 years at 8.64% (4). The difference in the prevalence can be attributable to the differences in diagnostic criteria and participant characteristics. In this study, for the sake of extrapolation and comparisons, we adopted the WHO criteria, instead of the China criteria (22). In addition to malnutrition prevalence, we also attempted to identify factors that can predict the significant risk of child malnutrition based on the survey data.
In this study, parental nutrition status (indexed by BMI) was found to be associated with child malnutrition, which was consistent with the results of several previous studies (23, 24). The connection between parental nutrition status and child malnutrition may be due to inherited factors, lifestyle habits, and cultural and social backgrounds. For example, the prevalence of child malnutrition was higher among mothers with lower BMI (25, 26). In light of this significant association, it is of added interest to examine the association of pregnancy BMI, which is not collected in this study, with child malnutrition, as pregnancy BMI can reflect the development of the fetus in utero (27).
The findings of this study underscored the importance of parental socioeconomic status, including education and family income, for child malnutrition. Some studies have reported that high family education and high family income are protective factors against child malnutrition (28, 29). By contrast, our findings indicated that high family education and income were significant risk factors in this study. This conflicting observation is explainable. On one hand, with higher family education and income and heavier work pressure, meal time spent with children becomes fewer, which might serve as a possible reason for child malnutrition. On the other hand, the transition from traditional diets to modern diets is characterized by “high sugar, high oil, and less nutrition” which provides a more convenient choice for parents to please their children, especially in high-income households, while commercial foods available on the market do not always deserve the “healthy halo” (30). Moreover, pocket money given by parents is often used to buy unhealthy snacks (31). Therefore, parents are encouraged to accompany their children to eat and to focus on a healthy and balanced diet for their children.
In addition to inherited and environmental factors, dietary factors were also attributable to the occurrence of malnutrition. In this study, we found that dietary habits such as fast food intake frequency, night meals intake frequency, and eating speed were associated with the occurrence of children’s malnutrition. Generally, fast food, which belongs to ultra-processed foods and is devoid of nutrients, can increase the risk of malnutrition, consistent with the results of Khan et al. (32). In addition, frequent night meals and fast eating speed were associated with child malnutrition, and this association was rarely reported in the literature. There is evidence that late dinner eating was linked to low cortisol concentrations and then low appetite during meals (33, 34), and a lower appetite infecting the speed of eating and alimentation could result in childhood undernutrition. Moreover, slow eating may be a symptom of anorexia, a disorder of nutrient absorption. Nevertheless, we cannot exclude the possible impact of different timelines for measurement on the results of this association study. There is no doubt that such poor dietary habits are considered preliminary, and further large, well-designed studies are needed to confirm or refute this finding.
Limitations
Some limitations should be acknowledged for this study. First, in this study, all participating children were enrolled from Beijing and Tangshan, which are two economically developed cities; hence, extrapolation of our findings to the other areas should be made with caution. Second, data derived from questionnaires filled by parents or caregivers cannot exclude the possibility of recall bias. Third, some socioeconomic data were unavailable for us, such as parental employment. Fourth, the causality of potential risk factors with child malnutrition cannot be addressed due to the cross-sectional design.
Conclusion
To sum up, we found that approximately 10% of Chinese children aged 3–14 years were in malnutrition status, and seven factors, namely, parental education, family income, fast food intake frequency, night meals intake frequency, eating speed, maternal obesity, and parental obesity, were found to be significant predictors for child malnutrition. For practical reasons, our findings provide an opportunity to understand the risk profiles of child malnutrition and address the utility of screening these factors for primary prevention by changing diets and lifestyles, eventually, preventing the occurrence of child malnutrition.
Data availability statement
The datasets presented in this article are not readily available because the data includes identifiable data of minors and privacy. Requests to access the de-identified datasets should be directed to zhangzhixin032@163.com.
Ethics statement
The studies involving humans were approved by The Ethics Committee of China-Japan Friendship Hospital and Beijing University of Chinese Medicine. The studies were conducted in accordance with the local legislation and institutional requirements. Written informed consent for participation in this study was provided by the participants’ legal guardians/next of kin.
Author contributions
XZ, ZhZ, and WN designed the study. XZ, QW, and ZiZ obtained statutory and ethics approvals. QW and ZhZ contributed to data acquisition. ZG, JW, and WN performed the statistical analysis. XZ and QW wrote the first draft. ZhZ and WN are the study guarantors. All authors contributed to the article and approved the submitted version.
Acknowledgments
The authors are grateful to all participating children and their parents or guardians for their cooperation and willingness and kindergarten or school teachers and health physicians for their generous help.
Conflict of interest
The authors declare that the research was conducted in the absence of any commercial or financial relationships that could be construed as a potential conflict of interest.
Publisher’s note
All claims expressed in this article are solely those of the authors and do not necessarily represent those of their affiliated organizations, or those of the publisher, the editors and the reviewers. Any product that may be evaluated in this article, or claim that may be made by its manufacturer, is not guaranteed or endorsed by the publisher.
Supplementary material
The Supplementary material for this article can be found online at: https://www.frontiersin.org/articles/10.3389/fnut.2023.1228799/full#supplementary-material
Footnotes
References
1. Yang, B, Huang, X, Liu, Q, Tang, S, Story, M, Chen, Y, et al. Child nutrition trends over the past two decades and challenges for achieving nutrition SDGs and National Targets in China. Int J Environ Res Public Health. (2020) 17:1129. doi: 10.3390/ijerph17041129
2. Malnutrition, Child: UNICEF & WHO [Available from: https://data.unicef.org/topic/nutrition/malnutrition/.
3. Report on Chinese 0–6 years old child nutrition development (2012) Beijing: Ministry of Health of the People’s Republic of China; (2012) Available at: https://www.chinanutri.cn/yyjkzxpt/yyjkkpzx/xcclk/xinxi/201501/t20150115_109818.html.
4. Liu, JY, Zhong, PL, Ma, N, Shi, D, Chen, L, Dong, YH, et al. Prevalence trend of malnutrition among Chinese Han children and adolescents aged 7-18 years from 2010 to 2019. Zhonghua Yu Fang Yi Xue Za Zhi. (2023) 57:27–35. doi: 10.3760/cma.j.cn112150-20220914-00897
5. de Onis, M, Borghi, E, Arimond, M, Webb, P, Croft, T, Saha, K, et al. Prevalence thresholds for wasting, overweight and stunting in children under 5 years. Public Health Nutr. (2019) 22:175–9. doi: 10.1017/S1368980018002434
6. He, Y, Li, Y, Yang, X, Hemler, EC, Fang, Y, Zhao, L, et al. The dietary transition and its association with cardiometabolic mortality among Chinese adults, 1982-2012: a cross-sectional population-based study. Lancet Diabetes Endocrinol. (2019) 7:540–8. doi: 10.1016/S2213-8587(19)30152-4
7. Chen, P, Wang, D, Shen, H, Yu, L, Gao, Q, Mao, L, et al. Physical activity and health in Chinese children and adolescents: expert consensus statement (2020). Br J Sports Med. (2020) 54:1321–31. doi: 10.1136/bjsports-2020-102261
8. Gao, C, Xu, J, Liu, Y, and Yang, Y. Nutrition policy and healthy China 2030 building. Eur J Clin Nutr. (2021) 75:238–46. doi: 10.1038/s41430-020-00765-6
9. Keats, EC, Rappaport, AI, Shah, S, Oh, C, Jain, R, and Bhutta, ZA. The dietary intake and practices of adolescent girls in low- and middle-income countries: a systematic review. Nutrients. (2018) 10:1978. doi: 10.3390/nu10121978
10. Balantekin, KN. The influence of parental dieting behavior on child dieting behavior and weight status. Curr Obes Rep. (2019) 8:137–44. doi: 10.1007/s13679-019-00338-0
11. Asgari, E, Askari, M, Bellissimo, N, and Azadbakht, L. Association between Ultraprocessed food intake and overweight, obesity, and Malnutrition among children in Tehran, Iran. Int J Clin Pract. (2022) 2022:1–7. doi: 10.1155/2022/8310260
12. Hu, X, Jiang, H, Wang, H, Zhang, B, Zhang, J, Jia, X, et al. Intraindividual double burden of Malnutrition in Chinese children and adolescents aged 6-17 years: evidence from the China health and nutrition survey 2015. Nutrients. (2021) 13:3097. doi: 10.3390/nu13093097
13. Yamborisut, U, Kosulwat, V, Chittchang, U, Wimonpeerapattana, W, and Suthutvoravut, U. Factors associated with dual form of malnutrition in school children in Nakhon Pathom and Bangkok. J Med Assoc Thail. (2006) 89:1012–23.
14. Wang, Q, Yang, M, Pang, B, Xue, M, Zhang, Y, Zhang, Z, et al. Predicting risk of overweight or obesity in Chinese preschool-aged children using artificial intelligence techniques. Endocrine. (2022) 77:63–72. doi: 10.1007/s12020-022-03072-1
15. Xue, M, Wang, Q, Zhang, Y, Pang, B, Yang, M, Deng, X, et al. Factors associated with lower respiratory tract infection among Chinese students aged 6-14 years. Front Pediatr. (2022) 10:911591. doi: 10.3389/fped.2022.911591
16. World Health Organization WHO child growth standards: methods and development. Available from: https://www.who.int/childgrowth/standards/technical_report/en/.
17. de Onis, M, Onyango, AW, Borghi, E, Siyam, A, Nishida, C, and Siekmann, J. Development of a WHO growth reference for school-aged children and adolescents. Bull World Health Organ. (2007) 85:660–7. doi: 10.2471/BLT.07.043497
18. Tremblay, MS, Aubert, S, Barnes, JD, Saunders, TJ, Carson, V, Latimer-Cheung, AE, et al. Sedentary behavior research network (SBRN) – terminology consensus project process and outcome. Int J Behav Nutr Phys Act. (2017) 14:75. doi: 10.1186/s12966-017-0525-8
19. Koo, TK, and Li, MY. A guideline of selecting and reporting Intraclass correlation coefficients for reliability research. J Chiropr Med. (2016) 15:155–63. doi: 10.1016/j.jcm.2016.02.012
20. Levels and trends in child malnutrition: Key Findings of the (2021) Edition of the Joint Child Malnutrition Estimates. Available at: https://data.unicef.org/resources/jme-report-2021.
21. Black, RE, Victora, CG, Walker, SP, Bhutta, ZA, Christian, P, de Onis, M, et al. Maternal and child undernutrition and overweight in low-income and middle-income countries. Lancet. (2013) 382:427–51. doi: 10.1016/S0140-6736(13)60937-X
22. Screeningstandard for malnutrition of school-age children and adolescents. China: NHaFPCPsRo (2014).
23. Li, Z, Kim, R, Vollmer, S, and Subramanian, SV. Factors associated with child stunting, wasting, and underweight in 35 low- and middle-income countries. JAMA Netw Open. (2020) 3:e203386. doi: 10.1001/jamanetworkopen.2020.3386
24. Subramanian, SV, Ackerson, LK, and Smith, GD. Parental BMI and childhood undernutrition in India: an assessment of intrauterine influence. Pediatrics. (2010) 126:e663–71. doi: 10.1542/peds.2010-0222
25. Murhima'Alika, CC, Balolebwami Zigabe, S, Bahati Lufungulo, Y, Mwene-Batu Lyabayungu, P, Garhalangwa Mayeri, D, Gaylord, AN, et al. Nutritional and health status of a cohort of school-age children born to mothers treated for severe acute malnutrition in their childhood in the Democratic Republic of Congo. PLoS One. (2022) 17:e0269527. doi: 10.1371/journal.pone.0269527
26. Prasad, JB, Pezhhan, A, and Patil, SH. Effect of wealth, social inequality, Mother's BMI, and education level on child malnutrition in India. Diabetes Metab Syndr. (2021) 15:102304. doi: 10.1016/j.dsx.2021.102304
27. Amaha, ND, and Woldeamanuel, BT. Maternal factors associated with moderate and severe stunting in Ethiopian children: analysis of some environmental factors based on 2016 demographic health survey. Nutr J. (2021) 20:18. doi: 10.1186/s12937-021-00677-6
28. Singh, S, Srivastava, S, and Upadhyay, AK. Socio-economic inequality in malnutrition among children in India: an analysis of 640 districts from National Family Health Survey (2015-16). Int J Equity Health. (2019) 18:203. doi: 10.1186/s12939-019-1093-0
29. Kang, Y, Aguayo, VM, Campbell, RK, Dzed, L, Joshi, V, Waid, JL, et al. Nutritional status and risk factors for stunting in preschool children in Bhutan. Matern Child Nutr. (2018) 14:e12653. doi: 10.1111/mcn.12653
30. The State of the World’s Children. Children, Food and Nutrition: Growing well in a changing world New York. UNICEF (2019). Available at: https://www.unicef.org/reports/state-of-worlds-children-2019
31. Bridging the gap: engaging adolescents for nutrition, health and sustainable development Rome: Anthrologica and World Food Programme; Available at: https://www.wfp.org/publications/2018-bridging-gap-engaging-adolescents-nutrition-health-and-sustainable-development.
32. Khan, MF, Banerjee, S, Bandyopadhyay, K, Kalaiselvi, S, Akkilgunata, S, Tripathy, J, et al. Role of dietary habits and personal hygiene on nutritional status of school-going adolescents: a cross-sectional study in selected schools located in slum areas of Nagpur City, Maharashtra. Ann Afr Med. (2022) 21:185–92. doi: 10.4103/aam.aam_109_20
33. Sinha, R, Gu, P, Hart, R, and Guarnaccia, JB. Food craving, cortisol and ghrelin responses in modeling highly palatable snack intake in the laboratory. Physiol Behav. (2019) 208:112563. doi: 10.1016/j.physbeh.2019.112563
Keywords: malnutrition, children, prevalence, risk factor, association
Citation: Zhang X, Wang Q, Gao Z, Zhang Z, Wu J, Zhang Z and Niu W (2023) Prevalence of malnutrition and its associated factors among 18,503 Chinese children aged 3–14 years. Front. Nutr. 10:1228799. doi: 10.3389/fnut.2023.1228799
Edited by:
Roberto Fernandes Da Costa, Autonomous University of Chile, ChileReviewed by:
Flavia Prodam, University of Eastern Piedmont, ItalyGilbert Sterling Octavius, University of Pelita Harapan, Indonesia
Copyright © 2023 Zhang, Wang, Gao, Zhang, Wu, Zhang and Niu. This is an open-access article distributed under the terms of the Creative Commons Attribution License (CC BY). The use, distribution or reproduction in other forums is permitted, provided the original author(s) and the copyright owner(s) are credited and that the original publication in this journal is cited, in accordance with accepted academic practice. No use, distribution or reproduction is permitted which does not comply with these terms.
*Correspondence: Zhixin Zhang, zhangzhixin032@163.com; Wenquan Niu, niuwenquan_shcn@163.com; Jing Wu, wujing20221120@163.com