- 1Department of Environmental Health and Occupational Medicine, School of Public Health, Guilin Medical University, Guilin, Guangxi, China
- 2The Guangxi Key Laboratory of Environmental Exposomics and Entire Lifecycle Heath, Guilin, Guangxi, China
- 3Department of Environmental and Occupational Health, School of Public Health, Guangxi Medical University, Nanning, Guangxi, China
Objective: The objective of this study was to investigate the relationship between dietary patterns and cognitive function in older adults (≥60 years old).
Methods: Food intake was quantitatively assessed by the Food Frequency Questionnaire (FFQ), and cognitive function was assessed by the Chinese version of the Simple Mental State Examination Scale (MMSE). Four major dietary patterns were identified by the factor analysis (FA) method. The relationship between dietary patterns and cognitive function was evaluated by logistic regression.
Results: A total of 884 participants were included in the study. Four dietary patterns (vegetable and mushroom, oil and salt, seafood and alcohol, and oil tea dietary patterns) were extracted. In the total population, Model III results showed that the fourth quartile of dietary pattern factor scores for the vegetable and mushroom pattern was 0.399 and 7.056. The vegetable and mushroom dietary pattern may be a protective factor for cognitive function, with p-value = 0.033, OR (95% CI): 0.578 (0.348, 0.951) in Model III (adjusted for covariates: sex, ethnic, marital, agricultural activities, smoking, drinking, hypertension, diabetes, dyslipidemia, BMI, and dietary fiber). In the ethnic stratification analysis, the scores of dietary pattern factors of the vegetable and mushroom among the Yao participants were 0.333 and 5.064. The Vegetable and mushroom diet pattern may be a protective factor for cognitive function, p-value = 0.012, OR (95% CI): 0.415 (0.206, 0.815).
Conclusion: The fourth quartile of the vegetable and mushroom dietary pattern scores showed dose-dependent and a strong correlation with cognitive function. Currently, increasing vegetable and mushroom intake may be one of the effective ways to prevent and mitigate cognitive decline. It is recommended to increase the dietary intake of vegetables and mushroom foods.
1. Introduction
As global lifespan increases, there is a concern about diseases that significantly impact older adults, including those that cause chronic ailments, impact physical functioning, and lead to cognitive decline. Cognitive decline can have multiple causes, with Alzheimer's disease being the most common, with more than 46 million people worldwide suffering from dementia, which is impacted strongly (1, 2). It is expected that the number of Alzheimer's disease cases will increase to 131.5 million by 2050, and the aging population in China is constantly increasing. In 2020, the prevalence of cognitive decline and dementia in elderly individuals aged 60 years and above in China was 15.54% and 6.04%, respectively (3–5). Areas of cognitive dysfunction, such as progressive memory loss and language decline, are progressively impairing the ability of older adults to live independently.
The implementation of healthy dietary patterns is a potential preventive strategy for declining cognitive function and can mitigate the cognitive decline that occurs with age (6). The effects of single nutrients or foods on cognitive function have been well documented. For example, high intakes of fish, omega-3 polyunsaturated fatty acids, and high linoleic acid may reduce cognitive decline (7–9). Nutrients such as monounsaturated fatty acids (MUFAs), polyunsaturated fatty acids (PUFAs), and antioxidants (e.g., beta-carotene and vitamin C) can protect the nervous system and mitigate cognitive decline (10–12).
However, it has been found that one's entire diet should be considered and that there are synergistic effects between the nutrients consumed (stronger than their single components), meaning that dietary patterns may have a more significant impact on cognitive function (13, 14). In recent years, the focus has shifted from investigating specific nutrients or foods to generalizing dietary patterns, and some dietary patterns have become representative of the theoretical basis that the whole diet reduces cognitive decline, such as the Mediterranean, Dietary Approaches to Stop Hypertension (DASH), Mediterranean-DASH Intervention for Neurodegenerative Delay (MIND), low-fat and plant-based, traditional food, and Westernized and seafood-rich dietary patterns (15–19). The findings on dietary patterns and cognitive function vary, with plant-based dietary patterns and Mediterranean dietary patterns alleviating the decline in cognitive impairment (17, 20). These results offer hope for dietary patterns to prevent cognitive impairment. However, the greatest limitation of theoretically guided dietary patterns is that they do not provide complete access to the full information of the dietary matrix and do not reflect the true picture of dietary intake, especially for specific populations in particular regions that cannot strictly adhere to typical dietary patterns. For example, the Mediterranean dietary model does not make use of the accumulated knowledge on the relationship between dietary intake of nutrients and neurodegeneration and only considers nine food groups (13).
The most commonly used method to explore dietary patterns is factor analysis (FA) using a data-driven approach. This method is not limited by theory but reflects dietary intake in a specific population. For example, adherence to a “grain” pattern may be a risk factor for cognitive function and a “plant” pattern may be a protective factor for cognitive function (21). Gongcheng County is the “hometown of longevity” in China, and oil tea is a daily beverage for people in southwest Guangxi, among which Gongcheng oil tea (22) is the most famous. According to the analysis of China's National Food hygiene standard method, the main components of oil tea are green tea, ginger, and oil (mainly peanut oil), and its main nutritional components include tea polyphenols, gingerol, total fat, and dietary fiber. As a specialty drink of the Yao people, Chinese medicine considers oil tea to be a compound preparation of traditional Chinese medicine, which has the functions of strengthening the spleen and eliminating food, treating the common cold and loosening the bowels, relieving symptoms of diarrhea, and stopping diarrhea (22). Ginger (23) and tea polyphenols (24) have been shown to have neuroprotective effects. We found that the dietary structure of the local elderly is relatively stable and less affected by external influences. However, there are few studies on the relationship between the dietary patterns of oil tea drinkers and cognitive function. Therefore, we further investigated the relationship between dietary patterns and cognitive function in Gongcheng County to provide an epidemiological basis for the prevention of cognitive impairment in local older adults.
2. Participants and methods
2.1. Participants
The study site was Gongcheng Yao Autonomous County, Guilin, Guangxi, China. According to the cross-sectional sampling survey, the sample size calculation formula is as follows:
where N is the sample size, Z is the statistic, α = 0.05, Z = 1.96, p is the expected prevalence, q = 1-p, and d is the allowable error (d = 0.1p). The prevalence of cognitive dysfunction in rural Chinese older adults was 42.9% (25). The estimated sample size for this study was 589, with an additional 10–15% of the sample. The number of people surveyed in this cross section was N = 1,246. Each participant completed the MMSE and FFQ questionnaire and underwent a physical examination and laboratory tests.
Inclusion criteria were as follows: older adults who (a) resided in the study area; (b) were over 60 years of age; (c) had blood sample, height, weight, and questionnaire information; and (d) had a reasonable energy intake of 500–6000 kcal (26). Finally, the participants were propensity score matched (PSM) (1:3) by age and years of education. A total of 884 adults aged 60 years and older were included in this study (2018–2019). The study included men (n = 370) and women (n = 514).
This research was conducted in accordance with the guidelines set forth in the Declaration of Helsinki, and all procedures involving human participants were approved by the Ethics Committee of Guilin Medical College. Written informed consent was obtained from each participant (No. 20180702-3).
2.2. Assessment of cognitive function
The MMSE questionnaire has high reliability and validity for the assessment of cognitive function. The questionnaire has the advantage of being easily applied and implemented in a short period. To assess the relationship between dietary patterns and the risk of cognitive dysfunction, MMSE scores have been classified according to the level of literacy: illiterate group ≤17, primary group ≤20, secondary and higher group ≤24 as the cutoff point, regarded as the cognitive impairment group (CI), compared to the non-CI group, and the rest are classified as the non-cognitive impairment (non-CI) group (27, 28).
2.3. FA and identification of dietary patterns
The main food intake during the previous year was determined by reference to the food frequency questionnaire (FFQ), which included the frequency and quantity of food consumed (29). The intake of each group (g/day) was then calculated by adding the intake of the member's food. Daily nutrient intake was calculated by multiplying the frequency of consumption of each component of each food by the nutrient content of that particular component. In this study, foods were combined into different food groups according to their nutritional content and use. To extract dietary patterns, we divided 109 foods into 14 food groups with reference to similar nutrients: cereals and potatoes, vegetables, fruits, soybeans and nuts, poultry, gut, aquaculture, egg, milk, mushroom, alcohol, oil, salt, and oil tea. Dietary patterns were generated by utilizing the factor analysis method (FA) for 14 predefined food groups. Factor analysis is a nutritional epidemiological method. By distinguishing one or more dietary factors, we found that certain types of food tend (or not) to be ingested in the same sitting.
Feature values of >1 and scree plots were used to determine the number of dietary patterns. Furthermore, by using orthogonal transformations of rotation factors, the results were easier to interpret. Food groups with absolute factor loadings of >0.3 were significant in the dietary pattern because the food items in these food groups appeared to be strongly associated with the identified factors. Factor scores were calculated by weighting each participant's intake of the 14 food groups by factor loadings. We named dietary patterns based on food groups with factor loadings of >0.6.
2.4. Definition of covariates
Diabetes was defined as fasting blood glucose (FBG) of ≥7.0 mmol/L or a regimen of glucose-lowering medications (30). Hypertension was defined as systolic blood pressure (SBP) of ≥140 mmHg and/or diastolic blood pressure (DBP) of ≥90 mmHg or a regimen of antihypertensive medication (31) (for this study, hypertension includes having had hypertension and not knowing that you have hypertension but having hypertension on your test.). Dyslipidemia was defined as total cholesterol (TC) of ≥6.22 mmol/L, triacylglycerol (TG) of ≥ 2.26 mmol/L, low-density lipoprotein cholesterol (LDL-C) of ≥4.14 mmol/L as high LDL-hemorrhage, and high-density lipoprotein cholesterol (HDL-C) of <1.04 mmol/L as hypo-HDL-C (32). The following demographic information was collected through questionnaires: age (60–69, 70–79, and ≥80 years), sex (male or female), ethnicity (Han, Yao, or other), marital status (unmarried, married, or other), education (illiterate [uneducated], primary education [educated for 0–6 years], and higher education [educated for more than 6 years]), agricultural activities [involved in agriculture (plowing, planting, and weeding)], and BMI (body mass index). Participants who were retired, homemakers, unemployed for more than 1 year, or physically disabled were considered nonworking individuals, unlike those involved in physical activities in agriculture. Smoking was defined as currently smoking at least one cigarette per day. Alcohol consumption was defined as drinking at least 50 g of alcohol or more once a month (33).
2.5. Statistical methods
The chi-square test and Student's t-test were applied for continuous and categorical variables, respectively, and the Wilcoxon rank-sum test was used for comparisons between groups of nonnormally distributed information. The energy and nutrient contents of food were obtained from the Chinese Food Composition Table (2009 version) (34). Nutrients were corrected using the residual method. Variance inflation factors (VIF < 10) (35) were employed to avoid multicollinearity between nutrients. The ranges of the included nutrients are as follows: SFAs, 229.324; MUFAs, 1159.459; PUFAs, 220.699; energy, 336.380; protein, 13.121; fat, 3052.548; carbohydrates, 81.488; and dietary fiber, 2.182. Case matching and controls by age and educational years were performed using the propensity score matching method. Four dietary patterns were extracted using the factor analysis (FA) method with a factor loading matrix criterion of 0.3. Food groups that exceeded the criterion of 0.6 showed extreme correlation with their corresponding dietary patterns and were then named according to those patterns. Unconditional regression of binary logistic regression was used to fit a continuous intake of 14 food groups to the cognitive function model, and linear trend analysis was implemented. Simultaneous restricted cubic spline (RCS) plots were used to represent the relationship between continuous dietary pattern scores and cognitive function. The lowest quartile (first quartile) was defined as the reference group in each model. The results were expressed as weighted OR (95% CI). After adjusting for potential confounders, unconditional binary logistic regression was applied to analyze the relationship between dietary patterns and cognitive function and to perform between-group trend analysis stratified by ethnicity. All analyses were performed in R (4.1.3), and two-tailed p-values of <0.05 were considered statistically significant. The authors can be contacted for the source code should there be any questions about the analysis.
3. Result
3.1. Participant characteristics
The propensity score matching (PSM) results were as follows: non-CI (non-cognitive impairment) (N = 663) and CI (cognitive impairment group, compared to the non-CI group.) (N = 221). The jitter scatter plot and the histogram of the propensity value distribution show good results (Supplementary Figure 1).
Statistically significant differences occurred between the sex (P-values = 0.001), hypertension (P-values = 0.049), MMSE scores, orientation, memory, attention calculation, language skills, and visuospatial skills (P-values < 0.001) of the non-CI and CI groups, as shown in Table 1. Different ethnic groups had statistically significant variations in sociodemographic characteristics, including marital status (P-values = 0.009) and agricultural activities (P-values = 0.009), as shown in Table 2.
Significant differences were observed in the dietary pattern scores of SFAs (P-values = 0.040), protein (P-values = 0.010), carbohydrate (P-values = 0.027), dietary fiber (P-values = 0.025), fruit (P-values = 0.008), soybeans and nuts (P-values = 0.037), poultry (P-values = 0.030), aquaculture (P-values = 0.005), eggs (P-values < 0.001), mushrooms (P-values < 0.001), and vegetables and mushrooms (P-values = 0.001) of the non-CI and CI groups, as shown in Table 3. For the non-CI and CI groups, dietary pattern scores for PUFAs (P-values = 0.008), soybeans and nuts (P P-values = 0.002), oil tea (P P-values = 0.020), oil and salt (P-values = 0.042), and seafood and alcohol (P-values = 0.049) significantly varied among different ethnic groups, as shown in Table 4.
3.2. Dietary patterns
Kaiser–Meyer–Olkin (KMO) factor adequacy is equal to 0.81, Bartlett's test of sphericity is less than 0.001, and the cumulative variance explained is 44.64%, all of which meet the conditions for the use of factor analysis. The left side of the Scree plots shows the characteristic roots, and the slope for 4–5 factors becomes flatter, with the first four factors already covering most of the information, as shown in Supplementary Figure 2. Thus, four can be chosen as the number of factors. The dietary patterns were named according to the factor loadings of the 14 food groups in the dietary patterns (vegetables and mushrooms, oil, and salt, seafood and alcohol, and oil tea), as shown in Table 5. The first factor, which was defined by a high intake of vegetables, mushrooms, fruits, cereal potatoes, soybean nuts, gut, egg, and milk, was labeled “vegetables and mushroom dietary pattern.” The second factor, which was defined by a high intake of oil, salt, poultry, and soybean nuts, was labeled the “oil and salt dietary pattern.” The third factor, which was defined by a high intake of aquaculture, alcohol, poultry, and fruits, was labeled “seafood and alcohol dietary pattern.” The fourth factor, which was defined by a high intake of oil tea, milk, and gut, was labeled the “oil tea dietary pattern.”
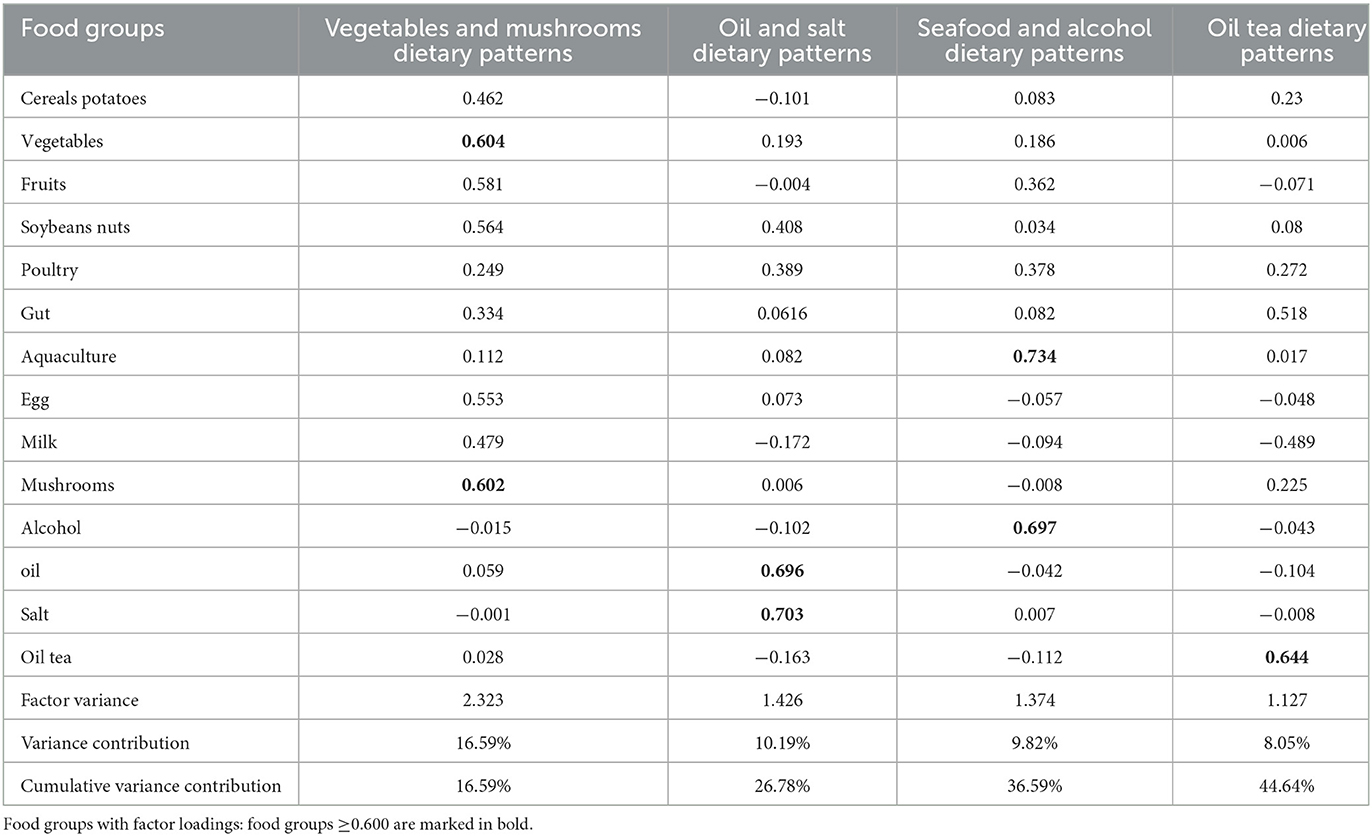
Table 5. Factor loadings of four principal components of 14 food groups extracted from principal component analysis (PCA) of eating frequency data of 884 adults aged 60 years or older.
3.3. Linear trend of 14 food groups and cognitive function scores
There was a nonlinear relationship between consumption and cognitive function values in the cereal and potato, fruit, and poultry food groups, with P-values of p = 0.009, p = 0.01, and p = 0.024, respectively (Supplementary Figure 3-1). There was also a non-linear relationship between dietary intake and cognitive function in the aquaculture, egg, milk, and mushroom food groups, with P-values of p = 0.001, p < 0.001, p = 0.037, and p = 0.045, respectively (Supplementary Figure 3-2).
3.4. Linear trend of factor scores of dietary patterns and cognitive function
We used restricted cubic spline (RCS) to flexibly model and visualize the relationship between dietary pattern scores and cognitive function. The seafood and alcohol dietary pattern showed a non-linear relationship with cognitive function, with a trend consisting of an initial decline, a brief increase, and a final flattening off, P-values = 0.003 (Supplementary Figure 4).
3.5. Association of dietary patterns with the risk of cognitive function
In Model I, after adjusting for sex and ethnicity, the score of the vegetable and mushroom dietary pattern factor was in the fourth quartile (0.399, 7.056); the vegetable and mushroom dietary pattern may be a protective factor for cognitive function, with an OR (95% CI) of 0.484 (0.302, 0.769). Other dietary patterns were not associated with cognitive function. In Model II, after adjusting for sex, ethnicity, marital status, agricultural activities, smoking, alcohol consumption, hypertension, diabetes, dyslipidemia, and BMI, when the score of the vegetable and mushroom dietary pattern factor was in the fourth quartile (0.399 and 7.056), it was found that the vegetable and mushroom dietary pattern may be a protective factor of cognitive function, with an OR (95% CI) of 0.517 (0.320, 0.830). Other dietary patterns were not associated with cognitive function. In Model III, after adjusting for sex, ethnicity, marital status, agricultural activities, smoking, alcohol consumption, hypertension, diabetes, dyslipidemia, BMI, and dietary fiber, when the score of the vegetable and mushroom dietary pattern factor was in the fourth quartile (0.399, 7.056), it was found that the vegetable and mushroom dietary pattern may be a protective factor of cognitive function, with an OR (95% CI) of 0.578 (0.348, 0.951). Other dietary patterns were not associated with cognitive function. A linear trend was noted between the quartiles of the dietary scores of the vegetable and mushroom (P-values = 0.017) and oil tea (P-values = 0.042) dietary patterns, as shown in Table 6.
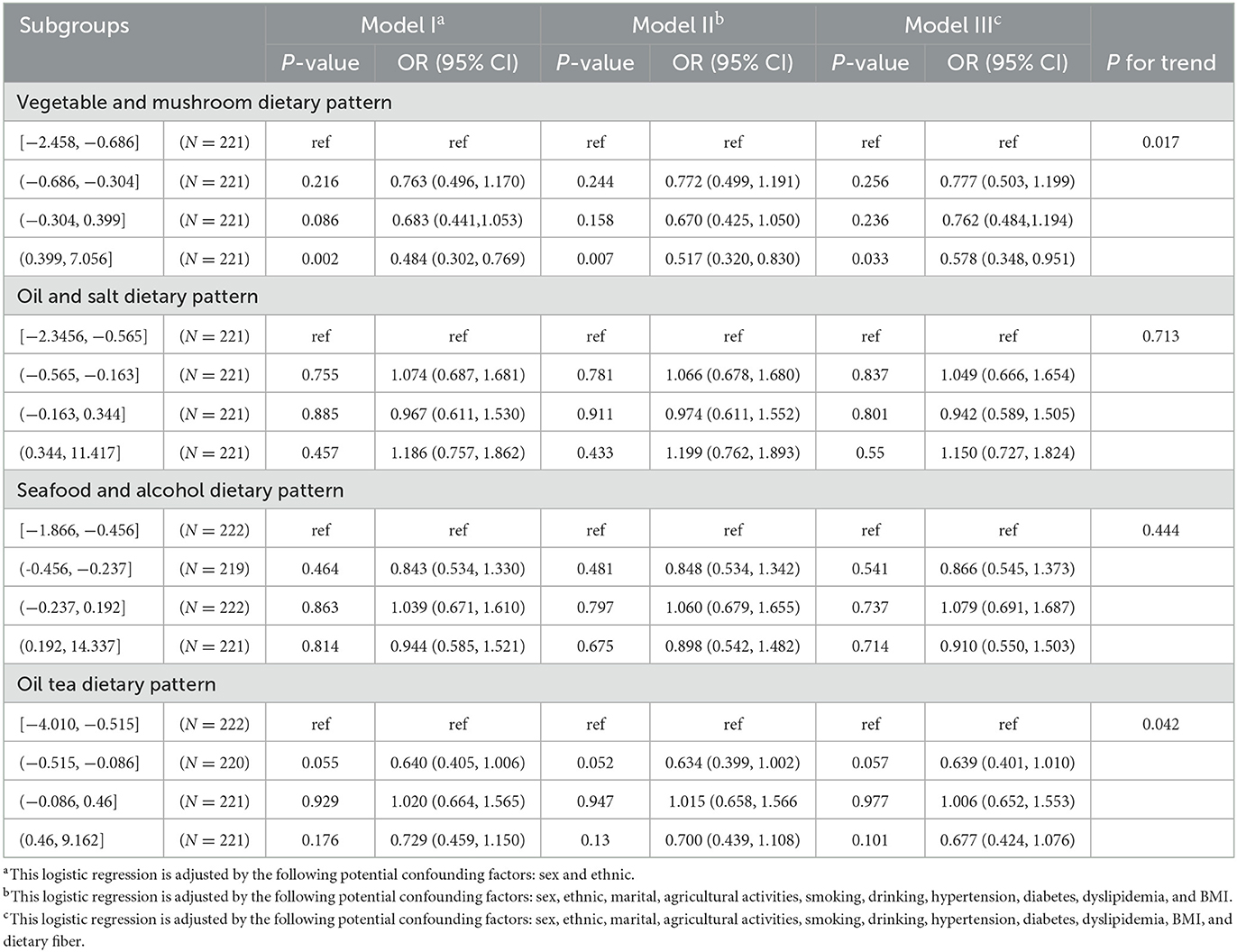
Table 6. Different potential confounders entered the logistic regression model and participants adhered to the relationship between different dietary patterns and cognitive functioning.
4. Discussion
This research was a case–control study based on a cross-sectional survey (N = 884). In a study of elderly people in Gongcheng County, female participants had a higher prevalence of cognitive impairment than other rural Chinese populations. There is substantial variation when considering the beneficial effects of diet in studies of the association of diet and cognitive impairment with age, and one potential reason for this variation in dietary pattern-cognitive function association may be sex (36, 37). Female participants should give greater attention to dietary intake. Our study points to a higher rate of cognitive impairment in hypertensive patients than in participants without cognitive impairment. Previous studies have suggested that hypertension is a risk factor for cognitive decline (38), and this association is mediated through microvascular brain damage (39). Appropriate blood pressure management may help alleviate cognitive decline in participants.
Four dietary patterns (vegetables and mushroom, oil and salt, seafood and alcohol, and oil tea) were extracted. The vegetable and mushroom dietary pattern (a) showed significant outcomes when the dietary pattern scores were at the fourth quartile after different levels of adjustment for potential confounders and (b) may be a protective factor for cognitive function. Among the Yao respondents, the vegetable and mushroom dietary pattern showed strong correlations with cognitive health when the dietary pattern scores were at the fourth quartile and after adjusting for different degrees of potential confounding factors. It is suggested that vegetable and mushroom dietary pattern may be a protective factor for cognitive function. After reviewing the previous literature, we made even more surprising findings. The Mediterranean dietary pattern includes various vegetables and mushrooms. Strict adherence to the Mediterranean diet (MD) may result in better cognitive status (40, 41). In a cross-sectional and longitudinal study of older adults in Taiwan, a dietary pattern high in phytonutrient-rich plant foods (fruits, whole grains, nuts/seeds, and vegetables) was associated with better cognitive function in older adults (42). A large epidemiological study in rural Shanxi, China, reported that two dietary patterns, the MVF (mushroom, vegetable, and fruit) and the MS (meat and soybean products) patterns, were predominant in the area, with greater adherence to the former being a possible protective factor for cognitive function (43). In the Chinese Longitudinal Healthy Longevity Survey (CLHLS), which assesses cognitive function using the Chinese version of the MMSE, a lower intake of fresh fruits and vegetables was significantly associated with a higher risk of cognitive impairment (44). Dietary intake patterns dominated by vegetables and mushrooms appear to have a protective effect on cognitive function, an outcome that is consistent with our findings. The likely reason for this protective effect is that antioxidants in the brain protect brain tissue from damage caused by free radicals, and eating more antioxidant-rich vegetables and fruits is strongly linked to the mitigation of damage in Alzheimer's patients (45). More prospective studies are needed to explore the correlation between diet and cognitive function and to determine the underlying molecular mechanisms. However, other studies indicate that cognitive function is not associated with the vegetable and mushroom dietary pattern. As discussed, the Mediterranean dietary pattern is known to include an abundance of vegetables and mushrooms. A New Zealand cohort study of older adults reported no significant associations between the Mediterranean dietary pattern and cognitive function or components of cognitive function (16). A study in Taiwan noted that a) in adults aged 65–74 years, a Western diet was detrimental to the maintenance of cognitive function, and b) in adults aged ≥75 years, the dietary pattern may not be correlated with cognitive function (46). The reason for those outcomes may be as follows: first, the Taiwan study adjusted for lifestyle factors and ADL, but no adjustment was made for nutrients, BMI, common diseases (including hypertension, diabetes, and dyslipidemia), and the covariate adjustment effect on the relationship between dietary pattern and cognitive function. Second, the healthy diet pattern involved a small sample size, thereby weakening the research strength. Finally, obvious differences in eating habits occur between rural and urban populations.
Oil and salt were not correlated with cognitive function. This finding is inconsistent with previous studies, which suggest that the oil and salt dietary pattern may be a risk factor for cognitive function (47). Dietary salt promotes neurovascular and cognitive dysfunction through gut-initiated TH17 participants (48), and high salt intake is associated with an increased risk of hypertension (49). Hypertension is an important risk factor for cognitive impairment (38), resulting in a small sample size that may lead to reduced statistical power for oil and salt dietary patterns to correlate with cognitive function.
The seafood and alcohol dietary pattern may not be associated with cognitive function. Previous reports suggest that the dietary patterns of older adult French people may be beneficial for cognitive function (fish for males and fruits and vegetables for females) (50). However, alcohol was not a protective factor for cognitive function (51). The complexity of this pattern could explain, to some extent, this inconsistent finding. Furthermore, the reason for the lack of association between the seafood and alcohol dietary pattern and cognitive function may be due to reverse causality. People with cognitive impairment may be advised to change their dietary habits and food choices. In conclusion, these possibilities cannot be ruled out in our analysis.
Oil tea dietary patterns were not associated with decreased cognitive function. Previous studies have reported that high doses of oil tea intake may be associated with a low risk of abnormal HDL cholesterol (29). This study explored the relationship between oil tea intake and blood lipids by grouping oil tea intake doses into more detailed quartiles. Recent studies have suggested that the weekly dietary intake of oil tea may be associated with diabetes under different dietary patterns (22). It may be implied that more detailed investigations should be conducted to explore the relationship between oil tea dietary patterns and cognitive function.
4.1. Limitations
This study also has some limitations that must be carefully considered. First, this study is a cross-sectional study, consisting mainly of older Chinese adults, and therefore, it is not possible to establish a clear chronological order or to exclude the possibility of reverse causality and the existence of Neyman bias. Second, the dietary intake assessment was not performed before the cognitive function measures, and we cannot infer the existence of the resulting causality. Third, the dependence of the FFQ method on memory or cognitive performance in this study did not allow for a precise estimation of nutrient intake. Fourth, we controlled for the effects of nutrient dietary intake in the model using the residual method and VIF. Fifth, we were unable to adjust for genetically related lipoprotein E status, but we controlled for a wide range of health-related factors as potential confounders. Sixth, because we conducted a large cross-sectional epidemiological survey, the MMSE questionnaire is not clinically adequate to accurately classify cognitive impairment, and our data do not adequately support studies with a detailed classification of patients with cognitive impairment. The MMSE questionnaire can only be used to initially assess the status of cognitive function. Therefore, to obtain more precise findings, longer follow-up and larger prospective studies are necessary to explore the relationship between dietary patterns and cognitive function.
5. Conclusion
In this population-based study, the vegetable and mushroom dietary pattern showed a strong correlation with cognitive function, and this association was dose-dependent. Therefore, the vegetable and mushroom dietary pattern is the safest choice at this time. Finally, the vegetable and mushroom dietary pattern may be a protective factor for cognitive function, suggesting that more vegetables and mushroom foods should be consumed in the diet to prevent cognitive impairment.
Data availability statement
The original contributions presented in the study are included in the article/Supplementary material, further inquiries can be directed to the corresponding authors.
Ethics statement
The studies involving human participants were reviewed and approved by the Ethics Committee of Guilin Medical College. The patients/participants provided their written informed consent to participate in this study.
Author contributions
RG and ZZ had full access to all of the data in the study, took responsibility for the integrity of the data, and the accuracy of the data analysis. RG drafted the manuscript and performed the statistical analysis. WP, JC, TL, KH, SX, and XT critically revised the manuscript for important intellectual content. YL obtained funding. YL and JQ supervised the study. All authors contributed to the article and approved the submitted version.
Funding
This study was supported by the National Natural Science Foundation of China (Grant No. 81960583), Guangxi Graduate Education Innovation Project (Grant/Award No. GYYK2021001), and Guangxi Science and Technology Major Special Project (Grant No. GKAA22096026).
Acknowledgments
The authors' deepest gratitude goes to the editors and reviewers for their careful work and thoughtful comments that have helped improve this study substantially. We first thank the Longevity Group of Guilin Medical University and, second, the Guangxi Key Laboratory of Environmental Exposomics and Entire Lifecycle Heath of Guilin Medical University. This study was previously submitted to the preprint platform (doi: 10.21203/rs.3.rs-944450/v2).
Conflict of interest
The authors declare that the research was conducted in the absence of any commercial or financial relationships that could be construed as a potential conflict of interest.
Publisher's note
All claims expressed in this article are solely those of the authors and do not necessarily represent those of their affiliated organizations, or those of the publisher, the editors and the reviewers. Any product that may be evaluated in this article, or claim that may be made by its manufacturer, is not guaranteed or endorsed by the publisher.
Supplementary material
The Supplementary Material for this article can be found online at: https://www.frontiersin.org/articles/10.3389/fnut.2023.1093456/full#supplementary-material
References
1. Cheung BH, Ho IC, Chan RS, Sea MM, Woo J. Current evidence on dietary pattern and cognitive function. Adv Food Nutr Res. (2014) 71:137–63. doi: 10.1016/B978-0-12-800270-4.00004-3
2. Ma W, Hagan KA, Heianza Y, Sun Q, Rimm EB, Qi L, et al. Adult height, dietary patterns, and healthy aging. Am J Clin Nutr. (2017) 106:589–96. doi: 10.3945/ajcn.116.147256
3. Jia L, Du Y, Chu L, Zhang Z, Li F, Lyu D, et al. Prevalence, risk factors, and management of dementia and mild cognitive impairment in adults aged 60 years or older in china: a cross-sectional study. Lancet Public Health. (2020) 5:e661–71. doi: 10.1016/S2468-2667(20)30185-7
4. Pang SJ, Jia SS, Man QQ, Song S, Li YQ, Song PK, et al. Dietary cholesterol in the elderly chinese population: an analysis of cnhs 2010-2012. Nutrients. (2017) 9. doi: 10.3390./nu9090934
5. Prince M. World Alzheimer Report 2015: The Global Impact of Dementia. King's College London, London, United Kingdom. (2015). Available online at: https://www.alzint.org/resource/world-alzheimer-report-2015/
6. Feart C, Samieri C, Barberger-Gateau P. Mediterranean diet and cognitive function in older adults. Curr Opin Clin Nutr Metab Care. (2010) 13:14–8. doi: 10.1097/MCO.0b013e3283331fe4
7. Kulzow N, Witte AV, Kerti L, Grittner U, Schuchardt JP, Hahn A, et al. Impact of omega-3 fatty acid supplementation on memory functions in healthy older adults. J Alzheimers Dis. (2016) 51:713–25. doi: 10.3233/JAD-150886
8. Mocchegiani E, Bertoni-Freddari C, Marcellini F, Malavolta M. Brain, aging, and neurodegeneration: role of zinc ion availability. Prog Neurobiol. (2005) 75:367–90. doi: 10.1016/j.pneurobio.04005
9. Morris MC, Evans DA, Tangney CC, Bienias JL, Wilson RS. Fish consumption and cognitive decline with age in a large community study. Arch Neurol. (2005) 62:1849–53. doi: 10.1001/archneur.62.12.noc50161
10. Beydoun MA, Fanelli-Kuczmarski MT, Kitner-Triolo MH, Beydoun HA, Kaufman JS, Mason MA, et al. Dietary antioxidant intake and its association with cognitive function in an ethnically diverse sample of us adults. Psychosom Med. (2015) 77:68–82. doi: 10.1097/PSY.0000000000000129
11. MacDonald-Wicks L, McEvoy M, Magennis E, Schofield PW, Patterson AJ, Zacharia K, et al. Dietary long-chain fatty acids and cognitive performance in older australian adults. Nutrients. (2019) 11:711. doi: 10.3390./nu11040711
12. Reay JL, Smith MA, Riby LM. B vitamins and cognitive performance in older adults: review. ISRN Nutr. (2013) 2013:650983. doi: 10.5402/2013/650983
13. Gu Y, Nieves JW, Stern Y, Luchsinger JA, Scarmeas N. Food combination and alzheimer disease risk: a protective diet. Arch Neurol. (2010) 67:699–706. doi: 10.1001/archneurol.2010.84
14. Wang CJ, Yang TF, Wang GS, Zhao YY, Yang LJ, Bi BN, et al. Association between dietary patterns and depressive symptoms among middle-aged adults in china in 2016–2017. Psychiatry Res. (2018) 260:123–9. doi: 10.1016/j.psychres.11052
15. Chlebowski RT, Rapp S, Aragaki AK, Pan K, Neuhouser ML, Snetselaar LG, et al. Low-fat dietary pattern and global cognitive function: exploratory analyses of the women's health initiative (whi) randomized dietary modification trial. Eclinicalmedicine. (2020) 18:100240. doi: 10.1016/j.eclinm.2019.100240
16. Mumme KD, Conlon CA, von Hurst PR, Jones B, Haskell-Ramsay CF, de Seymour JV, et al. Dietary patterns and cognitive function in older new zealand adults: the reach study. Eur J Nutr. (2022) 61:1943–56. doi: 10.1007/s00394-021-02775-x
17. Nishi SK, Babio N, Gomez-Martinez C, Martinez-Gonzalez MA, Ros E, Corella D, et al. Mediterranean, dash, and mind dietary patterns and cognitive function: the 2-year longitudinal changes in an older spanish cohort. Front Aging Neurosci. (2021) 13:782067. doi: 10.3389/fnagi.2021.782067
18. Okubo H, Inagaki H, Gondo Y, Kamide K, Ikebe K, Masui Y, et al. Association between dietary patterns and cognitive function among 70-year-old japanese elderly: a cross-sectional analysis of the sonic study. Nutr J. (2017) 16:56. doi: 10.1186/s12937-017-0273-2
19. Yu FN, Hu NQ, Huang XL, Shi YX, Zhao HZ, Cheng HY, et al. Dietary patterns derived by factor analysis are associated with cognitive function among a middle-aged and elder chinese population. Psychiatry Res. (2018) 269:640–5. doi: 10.1016/j.psychres.09004
20. Zhu A, Yuan C, Pretty J, Ji JS. Plant-based dietary patterns and cognitive function: a prospective cohort analysis of elderly individuals in china (2008–2018). Brain Behav. (2022) 12:e2670. doi: 10.1002/brb3.2670
21. Huang Q, Jiang H, Zhang J, Jia X, Huang F, Wang H, et al. Dietary patterns are associated with multi-dimensional cognitive functions among adults aged 55 and older in china. Front Nutr. (2022) 9:806871. doi: 10.3389/fnut.2022.806871
22. Liu Q, Cai J, Qin J, Zhang J, Xu X, Liu S, et al. Association between oil tea intake and the risk of type 2 diabetes in adults: a cross-sectional study in gongcheng, guangxi, china. Asia Pac J Clin Nutr. (2021) 30:487–96. doi: 10.6133/apjcn.202109_30(3).0015
23. Lim S, Moon M, Oh H, Kim HG, Kim SY, Oh MS, et al. Ginger improves cognitive function via ngf-induced erk/creb activation in the hippocampus of the mouse. J Nutr Biochem. (2014) 25:1058–65. doi: 10.1016/j.jnutbio.05009
24. Ni L, Zhao M, Hu Z, Yang K, Zhao X, Niu H, et al. Neural mechanism of shentai tea polyphenols on cognitive improvements for individuals with subjective cognitive decline: a functional near-infrared spectroscopy study. J Alzheimers Dis. (2021) 82:1137–45. doi: 10.3233/JAD-210469
25. Wang J, Xiao LD, Wang K, Luo Y, Li X. Cognitive impairment and associated factors in rural elderly in north china. J Alzheimers Dis. (2020) 77:1241–53. doi: 10.3233/JAD-200404
26. Kim KY, Yun JM. Association between diets and mild cognitive impairment in adults aged 50 years or older. Nutr Res Pract. (2018) 12:415–25. doi: 10.4162/nrp.125.415
27. Golden JMAP. Sensitivity of the mini-mental state exam to neuropsychological impairment in the elderly. Arch Clin Neuropsychol. (2000) 8:743–4. doi: 10.1016/S0887-6177(00)80175-4
28. Petersen RC. Mild cognitive impairment. Continuum. (2016) 22:404–18. doi: 10.1212/CON.0000000000000313
29. Cai J, Liu S, Li Y, Liu Q, Xu M, Mo C, et al. Effects of oil tea on obesity and dyslipidemia: a cross-sectional study in china. Diabetes Metab Syndr Obes. (2021) 14:3173–85. doi: 10.2147/DMSO.S312280
30. Li-Nong JI, Ju-Ming LU, Zhu DL, Endocrinology DO. Chinese expert recommendation on basal insulin treatment in adult type 2 diabetes mellitus. Chinese J Diabetes. (2017) 25:2–9. doi: 10.3969/j.issn.1006-6187.2017.01.002
31. Revision JCFG. 2018 chinese guidelines for prevention and treatment of hypertension-a report of the revision committee of chinese guidelines for prevention and treatment of hypertension. J Geriatr Cardiol 16. (2019) 182–241. doi: 10.11909/j.issn.1671-5411.03014
32. Revision JCFG. 2016 chinese guidelines for the management of dyslipidemia in adults. J Geriatr Cardiol. (2018) 15:1–29. doi: 10.11909/j.issn.1671-5411.01011
33. Cai J, Li Y, Liu S, Liu Q, Min X, Zhang J, et al. Associations between multiple heavy metals exposure and glycated hemoglobin in a chinese population. Chemosphere. (2022) 287:132159. doi: 10.1016/j.chemosphere.2021.132159
34. Prevention NIFN. Chinese Food Composition Chart. Beijing: Beijing University Medical Press (2009).
35. Kassambara A. Machine Learning Essentials: Practical Guide in r: STHDA. STHDA (2017). Available online at: http://www.sthda.com
36. Baker AH, Wardle J. Sex differences in fruit and vegetable intake in older adults. Appetite. (2003) 40:269–75. doi: 10.1016/s0195-6663(03)00014-x
37. D'Amico D, Parrott MD, Greenwood CE, Ferland G, Gaudreau P, Belleville S, et al. Sex differences in the relationship between dietary pattern adherence and cognitive function among older adults: findings from the nuage study. Nutr J. (2020) 19:58. doi: 10.1186/s12937-020-00575-3
38. Breteler MM, Claus JJ, Grobbee DE, Hofman A. Cardiovascular disease and distribution of cognitive function in elderly people: the rotterdam study. Bmj. (1994) 308:1604–8. doi: 10.1136/bmj.308.6944.1604
39. Hajjar I, Quach L, Yang F, Chaves PH, Newman AB, Mukamal K, et al. Hypertension, white matter hyperintensities, and concurrent impairments in mobility, cognition, and mood: the cardiovascular health study. Circulation. (2011) 123:858–65. doi: 10.1161/CIRCULATIONAHA.110.978114
40. De Amicis R, Leone A, Foppiani A, Osio D, Lewandowski L, Giustizieri V, et al. Mediterranean diet and cognitive status in free-living elderly: a cross-sectional study in northern italy. J Am Coll Nutr. (2018) 37:494–500. doi: 10.1080/07320181442263
41. Trichopoulou A, Kyrozis A, Rossi M, Katsoulis M, Trichopoulos D, La Vecchia C, et al. Mediterranean diet and cognitive decline over time in an elderly mediterranean population. Eur J Nutr. (2015) 54:1311–21. doi: 10.1007/s00394-014-0811-z
42. Chuang SY, Lo YL, Wu SY, Wang PN, Pan WH. Dietary patterns and foods associated with cognitive function in taiwanese older adults: the cross-sectional and longitudinal studies. J Am Med Dir Assoc. (2018) 20:544–50. doi: 10.1016/j.jamda.10017
43. Yin Z, Chen J, Zhang J, Ren Z, Dong K, Kraus VB, et al. Dietary patterns associated with cognitive function among the older people in underdeveloped regions: finding from the ncdfac study. Nutrients. (2018) 10:464. doi: 10.3390./nu10040464
44. Zhang Q, Wu Y, Han T, Liu E. Changes in cognitive function and risk factors for cognitive impairment of the elderly in china: 2005–2014. Int J Environ Res Public Health. (2019) 16. doi: 10.3390./ijerph16162847
45. Engelhart MJ, Geerlings MI, Ruitenberg A, van Swieten JC, Hofman A, Witteman JC, et al. Dietary intake of antioxidants and risk of alzheimer disease. Jama. (2002) 287:3223–9. doi: 10.1001/jama.287.24.3223
46. Tsai HJ. Dietary patterns and cognitive decline in taiwanese aged 65 years and older. Int J Geriatr Psychiatry. (2015) 30:523–30. doi: 10.1002/gps.4176
47. Qin YF, Guo YF, Ruan Y, Sun SY, Huang ZZ, Wu F, et al. Cross-sectional study of association between dietary pattern and cognitive performance in people aged 50 and above years in shanghai. Zhonghua Liu Xing Bing Xue Za Zhi. (2022) 43:674–80. doi: 10.3760/cma.j.cn112338-20210929-00758
48. Faraco G, Brea D, Garcia-Bonilla L, Wang G, Racchumi G, Chang H, et al. Dietary salt promotes neurovascular and cognitive dysfunction through a gut-initiated th17 response. Nat Neurosci. (2018) 21:240–9. doi: 10.1038/s41593-017-0059-z
49. Zheng PF, Shu L, Zhang XY, Si CJ, Yu XL, Gao W, et al. Association between dietary patterns and the risk of hypertension among chinese: a cross-sectional study. Nutrients. (2016) 8:239. doi: 10.3390/nu8040239
50. Samieri C, Jutand MA, Feart C, Capuron L, Letenneur L, Barberger-Gateau P, et al. Dietary patterns derived by hybrid clustering method in older people: association with cognition, mood, and self-rated health. J Am Diet Assoc. (2008) 108:1461–71. doi: 10.1016/j.jada.06437
Keywords: cognitive function, dietary patterns, older adults, vegetables, mushrooms
Citation: Gou R, Qin J, Pang W, Cai J, Luo T, He K, Xiao S, Tang X, Zhang Z and Li Y (2023) Correlation between dietary patterns and cognitive function in older Chinese adults: A representative cross-sectional study. Front. Nutr. 10:1093456. doi: 10.3389/fnut.2023.1093456
Received: 09 November 2022; Accepted: 13 March 2023;
Published: 04 April 2023.
Edited by:
Annamaria Anita Livia Colao, University of Naples Federico II, ItalyReviewed by:
Gianluca Rizzo, Independent Researcher, Messina, ItalyXu Gao, Peking University, China
Copyright © 2023 Gou, Qin, Pang, Cai, Luo, He, Xiao, Tang, Zhang and Li. This is an open-access article distributed under the terms of the Creative Commons Attribution License (CC BY). The use, distribution or reproduction in other forums is permitted, provided the original author(s) and the copyright owner(s) are credited and that the original publication in this journal is cited, in accordance with accepted academic practice. No use, distribution or reproduction is permitted which does not comply with these terms.
*Correspondence: Zhiyong Zhang, cnBhenpAMTYzLmNvbQ==; You Li, bGl5b3UxMjEzMDBAMTYzLmNvbQ==
†These authors have contributed equally to this work and share first authorship