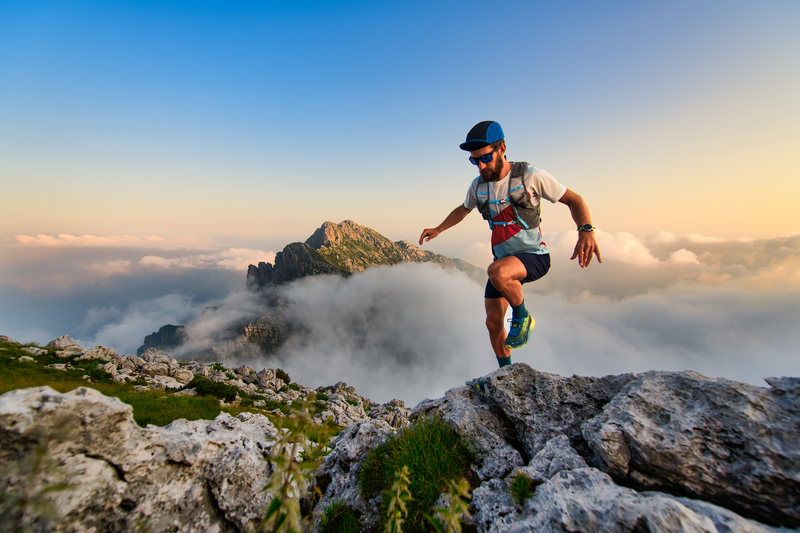
95% of researchers rate our articles as excellent or good
Learn more about the work of our research integrity team to safeguard the quality of each article we publish.
Find out more
ORIGINAL RESEARCH article
Front. Nutr. , 05 January 2023
Sec. Nutritional Epidemiology
Volume 9 - 2022 | https://doi.org/10.3389/fnut.2022.992472
Background: Nutritional management is the cornerstone of gestational diabetes mellitus (GDM) prevention. High quality instead of low quantity of carbohydrate intake has been paying attention in controlling glycemia. Air pollution exposure can be interacted with dietary sourced nutrients, which may modify the associations with GDM. This study aims to explore the associations between overall quality of carbohydrate intake and GDM as well as the modifying effect of prenatal air pollution exposure.
Methods: Carbohydrate quality index (CQI) was calculated was calculated by summing scores of the four components; Land use regression prediction models were used to assess the air pollution exposure levels. GDM definition was based on 75 g glucose tolerance test results. Associations between pre-pregnancy CQI, pre-natal air pollution as well as the modifying effect on GDM were explored based on a birth cohort in China.
Results: A total of 3,183 participants were included, of which 784 (24.63%) were diagnosed with GDM. Higher pre-pregnancy CQI was associated with a lower incidence of GDM [odds ratio (OR) = 0.75, 95% confidence interval (CI): 0.56–0.99, Pfor trend = 0.04], especially for higher fasting blood glucose related GDM (OR = 0.66, 95% CI: 0.47, 0.91). Higher air pollution exposure before and during pregnancy was associated with a greater risk of GDM. Higher exposure to particulate matter with an aerodynamic diameter of < 2.5 μm (P for interaction < 0.01), particulate matter with an aerodynamic diameter of < 10 μm (P for interaction < 0.01), and sulfur dioxide (P for interaction = 0.02) during pregnancy decreased the beneficial effect of high pre-pregnancy CQI on GDM.
Conclusion: CQI related dietary interventions pre-pregnancy to prevent GDM incidence should be considered. Women who are planning to be pregnant should avoid high exposure to air pollution during pregnancy.
Gestational diabetes mellitus (GDM) is defined as chronic hyperglycemia during the gestational period in women without prior diabetes (1). GDM is the most common metabolic disorder during pregnancy; its average prevalence is 17% worldwide, and is higher in Southeast Asia than in North America (2). GDM is associated with elevated risk of long-term complications in mothers and infants, including polyhydramnios, stillbirth, fetal macrosomia, postnatal adiposity, and diabetes (3). Thus, preventing GDM before pregnancy is highly important for the overall health of both mothers and children.
Nutritional management is the cornerstone of GDM prevention (4, 5). An initial study has suggested that higher carbohydrate intake can increase postprandial glycemia in pregnant women (6). The American Dietetic Association recommends a carbohydrate intake of at least 175 g/d during pregnancy to control glycemia and maintain the nutrient supply for the fetus (7, 8). Many women are consuming lower carbohydrate diets (lower than 40% of total energy), even in the preconception period (9) to prevent GDM. However, this diet might be harmful for fetal growth, given the lack of micronutrient intake and the elevated exposure to maternal ketones. Recently, evidence has suggested that higher carbohydrate intake (60–70% of total energy) with high carbohydrate quality [lower glycemic index (GI) or sugars] during pregnancy can also control glycemia (10–12). A study in Australia has indicated that higher fiber intake pre-pregnancy decreases the risk of GDM (13). One carbohydrate source, like as fiber, cannot represent all the complicated effects of carbohydrate intake on GDM. Thus, an indicator representing the overall quality of carbohydrate intake is necessary. One such indicator is the carbohydrate quality index (CQI), which integrates the intake of dietary fiber, the GI, and the intake of whole grain, total grain, liquid, and solid carbohydrate. High CQI was associated with better micronutrient intake adequacy (14), and potential benefits in fetal development as well as GDM prevention. A cohort study has found that higher CQI is associated with a lower prevalence of subclinical atherosclerosis (15).
Air pollution exposure has been another global public health problem related to GDM (16–18). Interactions between air pollution exposure and dietary intake in several diseases including GDM have been reported in previous studies, possibly because both involve similar biological mechanisms, including variations in inflammation, oxidative reaction as well as methylation (19–21). Thus, air pollution exposure may influence the association between pre-pregnancy carbohydrate intake and GDM. We hypothesized that high quality carbohydrate intake pre-pregnancy might decrease the incidence of GDM, and this association might be modified by air pollution exposure levels. Thus, to provide evidence to support developing nutritional strategies for GDM prevention, we aimed to explore the associations between pre-pregnancy CQI and GDM, and the interactions between pre-pregnancy CQI and air pollution exposure before and during pregnancy on GDM.
The present study was conducted in a birth cohort belonging to the Northeast Cohort Study of China (NEC-Biobank). NEC-Biobank is a large, prospective, and dynamic cohort study focusing on typical environmental factors and chronic diseases, and covering a variety of populations including multiple races, professions, maternal-fetal, and children in Northeast China (19, 22). The protocol of the NEC-Biobank was approved by the ethics committee in Shengjing Hospital of China Medical University (no. 2017PS190K). Participants in the birth cohort were enrolled in the first or second trimester, and provided signed informed consent before inclusion. Baseline investigations included a face-to-face questionnaire survey, physical examinations, and biological sample collection started from 1st, August 2018. Follow-up was conducted in the third trimester, delivery period, and postnatal periods at 42 days, 6, and 12 months for mothers, and 12–24 months for children. By the end of August 2021, 4,221 participants were included in the birth cohort. We excluded mothers with the following conditions: history of diabetes or GDM (n = 78), no glucose tolerance test (OGTT) performed during weeks 24–28 of pregnancy (n = 698), no detailed address (n = 207), no age or race information (n = 3), missing data on weight or height (n = 4), unnormal (> 4,000 or < 1,500 kcal/day), or missing data regarding total energy intake per day (n = 48). Finally, a total of 3,183 participants were included in the analyses. The detailed selection process is shown in Figure 1.
Dietary intake in the year before pregnancy was assessed with a validated food frequency questionnaire (FFQ) with 110 food items. Participants were asked to choose their food intake frequencies for each food item from the following seven frequency categories: “2 or 3 times per day,” “1 time per day,” “4–6 times time per week,” “2–3 times per week,” “1 time per week,” “< 1 time per week,” or “almost never.” An ad hoc computer program was developed to calculate the mean daily intake (g/day) of nutrients and energy according to the Chinese Food Composition Tables (23).
The CQI was used to assess the overall carbohydrate intake quality for each participant (14). Components of CQI included the whole grain/total grain ratio, dietary fiber intake (g/day), ratio of solid/total carbohydrate, and total GI. Whole grain was the sum of all types of coarse grains. Total grain referred to whole grain and refined grain products (including rice, steamed bread or steamed twisted rolls, pancakes, noodles, wontons, steamed stuffed buns or dumplings, bread, all types of porridge, and various bean vermicelli or bean starch sheets). Solid carbohydrates were calculated as the total carbohydrate minus liquid carbohydrates. Liquid carbohydrates included the carbohydrates from fruit juice and sugar sweetened beverages. The GI for each food item was calculated with the data from the FFQ combined with the Chinese Food Composition Tables (23). The total GI for each participant was calculated by first multiplying the carbohydrates consumed per food item and its specific GI, then adding the GI contributions across all food items and dividing by the total carbohydrate intake (24).
CQI was calculated by summing scores of the four components as follows: for dietary fiber intake (gram/day), ratio of solid/total carbohydrate, and GI, participants were divided into quartiles. Quartiles in the order of 1–4 were used for dietary fiber intake and the ratio of solid/total carbohydrate scores. Inverse quartiles in the order of 4–1 were used for the GI score. For the ratio of whole grain/total grain, participants who did not consume any whole grains were assigned 1 point, while the other participants were divided into two categories according to the median values, and were assigned 2 or 3 points. The CQI (the sum of the four aforementioned components) ranged from 4 to 15. Participants with higher quality of carbohydrate intake had higher CQI (Table 1). We divided participants into three categories according to three 4-point intervals (4–7 points, 8–11 points, and 12–15 points) of CQI.
Land use regression prediction models were used to assess the air pollution exposure levels to particulate matter with an aerodynamic diameter of < 2.5 μm (PM2.5), particulate matter with an aerodynamic diameter of < 10 μm (PM10), sulfur dioxide (SO2), nitrogen dioxide (NO2), carbon monoxide (CO), and ozone (O3) for each participant before and during pregnancy. Monthly prediction models during 2017–2021 were developing by using monthly averaged air pollution data derived from 78 national monitoring stations in Liaoning province. The detailed development process has been described elsewhere (25). Personal exposure levels to the six aforementioned air pollutants were calculated as follows. First, ArcGIS version 10.5 was used to interpolate monthly spatial distributions of each air pollutants calculated from prediction models. Second, each participant’s detailed address was transformed into latitude and longitude data. Third, the average concentration of each air pollutant was calculated according to the last menstrual period; pre-pregnancy air pollution exposure was the average over the 12 months before the last menstrual period; and air pollution exposure during pregnancy was the average over 6 months (the first and second trimesters) after the last menstrual period. If a participant changed her address in the year before pregnancy, then both addresses and the time spent at each address were recorded, and the final exposures were calculated as the average exposures at both addresses.
The 75 g OGTT during the gestational period of 24–28 weeks was a routine prenatal examination in this study. According to the recommendations of the International Association of Diabetes and Pregnancy Study Groups in 2010 (26), GDM was diagnosed when at least one of the following was met: i, fasting blood glucose level > 5.1 mmol/L; ii, blood glucose level after oral administration of 75 g of glucose for 1 h > 10 mmol/L; iii, blood glucose level after oral administration of 75 g glucose for 2 h > 8.5 mmol/L.
A face to face questionnaire survey was used to collect pre-pregnancy information including the following variables: age, education level, race, family income, occupation, pre-pregnancy smoking, and alcohol consumption (yes or no), number of births, self-reported personal and family history of diabetes or GDM, personal history of other chronic diseases (including cardiac diseases, hypertension, depression, eclampsia, and polycystic ovarian syndrome), and household cooking behavior (almost none, one to two times per week, or more than three times per week). Education level was categorized as high (college graduate or above) or low (high school, middle school, or primary school graduate, or illiterate). Race was categorized as Han ethnicity or others. Occupation was divided into four categories [professional or civil servant, unskilled laborer, no formal occupation, or other (including student, farmer, etc.)]. Parity was categorized as primipara or multipara according to number of births. Comorbidity status was categorized as “no” (no personal history of other chronic diseases) or “yes” (personal history of other chronic diseases including hypertension, depression, anxiety, preeclampsia, etc.). Pre-pregnancy body mass index (BMI, kg/m2) was calculated as weight/(height height). The average metabolic equivalent hours per week (METs-h/week) for the total activities was calculated based on data collected from the Chinese version of the Pregnancy Physical Activity Questionnaire (27).
Participants were divided into three categories according to CQI (4–7 points, 8–11 points, or 12–15 points). Continuous variables are reported as mean values with standard deviations. Categorical variables are presented as counts and percentages. P-values for trends across the three groups of participants were examined with linear regression or logistic regression models. Participants’ characteristics were also described according to GDM status. Variance analysis and chi-squared test were used to examine continuous variables and categorized variables, respectively. Multiple logistic regression was first used to estimate the risk of GDM for each 4-point increase in CQI and was adjusted for age, BMI, race, family income, education level, occupation, family history, comorbidities, parity, smoking, and alcohol consumption, physical activity, daily intake of total energy, protein and fat in the final model. P-values for trends were also calculated. Multiple logistic regression was then used to estimate the risk of GDM for each inter-quartile range (IQR) increase in each air pollutant, and was adjusted for age, BMI, race, family annual income, education level, occupation, family history, comorbidities, parity, smoking, and alcohol consumption, physical activity, and household cooking behavior in the final model. The modifying effect of air pollution on CQI and GDM was examined by adding the interaction terms of each pollutant and CQI categories in the models. For significant interactions, we further assessed the risks of GDM associated with CQI according to the quartiles of each exposure level of air pollutants. For sensitive analysis, multiple linear regression was conducted to examine the linear associations among CQI, air pollution exposure, and glucose levels at fasting, and 1 and 2 h after oral administration of 75 g of glucose. The associations among the four components of CQI and GDM were also examined. SAS version 9.4 for Windows was used to perform all statistical analyses (SAS Institute Inc., Cary, NC, USA). Findings at P < 0.05 were considered significant.
This study included 3,183 participants, 784 (24.63%) of whom were diagnosed with GDM. Participant characteristics according to the three categories of CQI are shown in Table 2. Participants with higher CQI tended to be older (P < 0.0001), and to have higher education levels (P = 0.01), and higher daily intake of total energy (P < 0.0001), protein (P < 0.0001), and fat (P < 0.0001). Participants’ characteristics according to GDM status are shown in Supplementary Table 1. Participants with GDM tended to be older (P < 0.0001), and to have higher BMI (P < 0.0001), CQI (P < 0.01), pre-pregnancy air pollution exposure to PM10 (P = 0.01), and NO2 (P = 0.02), and a family history of diabetes or GDM (P < 0.01) or other comorbidities (P < 0.01).
Table 3 shows the associations between pre-pregnancy CQI and GDM. Higher pre-pregnancy CQI was associated with a lower incidence of GDM (P = 0.04). Compared with the lowest CQI category, participants in the highest category had the lowest risk of GDM (OR = 0.75, 95% CI: 0.56–0.99).
Associations between each IQR increase in air pollutants before and during pregnancy and the risk of GDM are presented in Table 4. Higher air pollution exposure to PM2.5 (OR = 1.28, 95% CI: 1.11, 1.47), PM10 (OR = 1.31, 95% CI: 1.13, 1.51), SO2 (OR = 1.30, 95% CI: 1.13, 1.49), and NO2 (OR = 1.41, 95% CI: 1.20, 1.65) before pregnancy, and air pollution exposure to PM2.5 (OR = 1.27, 95% CI: 1.09, 1.47), PM10 (OR = 1.18, 95% CI: 1.02, 1.36), NO2 (OR = 1.29, 95% CI: 1.11, 1.50), and CO (OR = 1.17, 95% CI: 1.03, 1.33) during pregnancy, were associated with greater risk of GDM. Both higher air pollution exposure to O3 before (OR = 0.87, 95% CI: 0.77, 0.99) and during (OR = 0.73, 95% CI: 0.62, 0.86) pregnancy were associated with greater risk of GDM.
Interaction analysis showed that pre-pregnancy air pollution exposure had no modulatory effects on CQI and GDM. Significant interaction effects between pre-pregnancy carbohydrate quality intake and air pollution exposure to PM2.5 (Pfor interaction < 0.01), PM10 (Pfor interaction < 0.01), SO2 (Pfor interaction = 0.02), and O3 (Pfor interaction = 0.03) during pregnancy were found with GDM. Analysis results stratified by air pollution level during pregnancy (classified into four quantiles) are presented in Figure 2. Higher exposure to PM2.5, PM10, and SO2 during pregnancy decreased the beneficial effect of high CQI pre-pregnancy on GDM.
Figure 2. Modifying effect of air pollution on CQI and GDM. (A) Association between every 4-point CQI increase and GDM according to four quantiles of PM2.5 exposure levels. (B) Association between every 4-point CQI increase and GDM according to four quantiles of PM10 exposure levels. (C) Association between every 4-point CQI increase and GDM according to four quantiles of SO2 exposure levels. (D) Association between every 4-point CQI increase and GDM according to four quantiles of NO2 exposure levels. (E) Association between every 4-point CQI increase and GDM according to four quantiles of CO exposure levels. (F) Association between every 4-point CQI increase and GDM according to four quantiles of O3 exposure levels. P-int, P-value for interaction. Multiple logistic regression models were adjusted for age; BMI, race family income; education level; occupation; family history of diabetes or GDM; comorbidities; parity; smoking and alcohol consumption; physical activity; daily intake of total energy, protein, and fat; and household cooking; Q1, the first quartile; Q2, the second quartile; Q3, the third quartile; Q4, the forth quartile; P-int, P-value for interaction.
As shown in Table 5, higher CQI was associated with only lower blood fasting glucose levels, but not 1 and 2-h glucose levels after oral administration of 75 g of glucose. We further used fasting glucose levels (≥ 5.1 mmol/L) to define GDM (fGDM). The associations between CQI in three categories and fGDM are shown in Supplementary Table 2, which indicate the risk of fGDM decreased as the CQI increased. Separate associations between scores of the four components of CQI and GDM are shown in Supplementary Table 3. Higher whole grain intake was significantly associated with lower risk of GDM (OR = 0.68, 95% CI: 0.52, 0.88; P for trend < 0.01). Association between air pollution exposure and blood glucose levels are shown in Supplementary Tables 4-1, 4-2. Every IQR increase in PM2.5, NO2, CO, and O3 during pregnancy was significantly associated with the fasting blood glucose level (PM2.5: β = 0.05, 95%CI: 0.01, 0.09; NO2: β = 0.07, 95%CI: 0.03, 0.11; CO: β = 0.04, 95%CI: 0.01, 0.08; O3: β = −0.11, 95%CI: −0.15, −0.07). We further analyzed the interaction effects between air pollution exposure during pregnancy and CQI on fGDM. The interaction P-values were 0.02, 0.03, < 0.01, 0.06, 0.02, and 0.01 for PM2.5 × CQI, PM10 × CQI, SO2 × CQI, NO2 × CQI, CO × CQI, and O3 × CQI, respectively.
Our findings indicated that higher pre-pregnancy CQI decreased the risk of GDM, particularly fasting blood glucose. Higher air pollution exposure to PM2.5, PM10, and SO2 during pregnancy decreased the protective effect of high carbohydrate intake quality on GDM. Higher O3 exposure during pregnancy strengthened the protective effect of high carbohydrate intake quality on GDM.
Previous studies have gradually recognized that merely decreasing the total quantity of carbohydrate intake can have a series of adverse effects on the health and development of mothers and children, instead of controlling current blood glucose levels (8, 9, 28). Increasing attention is being paid to the quality or nutritional source of carbohydrate intake (29, 30). A recent systematic review has indicated that higher quality carbohydrate intake during pregnancy can control blood glucose levels in GDM patients (31). An intervention experiment has indicated that oat and buckwheat compounds decrease the fasting glucose levels in diabetic rats (32). A clinical trial has reported that carbohydrate counting and adherence to the DASH diet decreases fasting blood glucose levels in GDM with metformin therapy (30). Most interventional studies have focused on dietary intake during pregnancy and GDM control (30, 33). Only limited studies have explored components of carbohydrate intake and the risk of GDM development. A study in the Seremban cohort has reported that glycemic index and glycemic load in dietary intake before pregnancy are not associated with GDM (34). A cohort study from Australia has reported that higher intake of cereal pre-pregnancy increases the risk of GDM, whereas higher intake of dietary fiber, fruits, and fruit juices decreases the risk of GDM (13). However, previous studies have usually focused on only one or two components of carbohydrate rather than overall intake quality. In this study, we took the total quality of carbohydrate intake before pregnancy into account and found a protective effect of higher pre-pregnancy CQI on GDM, particularly that related to fasting glucose levels, which is of high importance for dietary modification among women preparing for pregnancy. Regarding the components of CQI, we found that a higher ratio of whole grain intake pre-pregnancy significantly decreased the risk of GDM, in agreement with findings from a previous study (13).
Air pollution exposure before and during pregnancy has been a major environmental risk factor for GDM. The association between air pollution exposure before pregnancy and during pregnancy and GDM found in this study was in accordance with the results of previous studies (19, 35).
To our knowledge, no study has reported the interaction effect of CQI and air pollution on GDM. Studies since 2008 have focused mainly on the modifying effects of dietary or nutritional supplements on the association between air pollution exposure and chronic diseases (36). A birth cohort study in Spain has found that lower intake of vegetables and vitamin D, as well as a lack of breastfeeding, strengthen the adverse effects of NO2 exposure on infant mental development (37). A cohort study in the United States has found that a higher Mediterranean diet score is associated with lower rates of cardiovascular disease risk due to long-term PM2.5 exposure (20). Similar modification effects of vegetables, fruits, and Mediterranean diet intake have also been found on O3 exposure and lung function (36). Studies have also indicated that fish oil or other oil supplements mitigate the effects of inflammation in cardiovascular outcomes caused by air pollution exposure (38–40).
In this study, we examined the modifying effect of air pollution exposure on CQI and GDM, because dietary intervention is the fundamental strategy for the prevention and control of GDM. The elevated inflammatory response and oxidative stress due to higher exposure to air pollutants are associated with the development of GDM (41, 42). Higher CQI intake, with higher dietary fiber, lower glycemic index, and higher whole grain, has been found to be associated with lower GDM risk, through elevating plasma alkylresorcinols (43), slowing postprandial insulin responses (44), and decreasing oxidative stress (45). Thus, the modifying effect of air pollution on CQI and GDM might be due to that higher CQI decreased while air pollution of PM2.5, PM10 and SO2 increased the oxidative stress (46, 47), particulate matter induced inflammation and resulting oxidative stress has been proved to be a major pathway for the development of GDM. The finding that O3 exposure strengthened the interaction between CQI and GDM was unexpected, because O3 is a strong oxidant and can lead to insulin resistance by inducing oxidative stress. Possible reasons for this finding might be that pre-pregnancy O3 exposure could cause fetal loss before the GDM diagnosis is made (48) or that higher O3 exposure is correlated with lower exposure to all other pollutants (49) (Supplementary Table 5).
This is the first study to explore the associations between pre-pregnancy CQI and GDM, as well as the modulatory effects of air pollution, in a birth cohort. This study has several limitations. First, the participants in our birth cohort mainly came from urban areas and had high education levels. The CQI in this sample was categorized into three levels because of the moderate variability in dietary intake, and the results of this study do not represent people in rural areas. Second, although the FFQ was collected by trained reviewers, we could not rule out the possibility of dietary intake recall bias before pregnancy. Third, except for household cooking behavior, we did not consider other indoor air pollution, thus potentially resulting in biases in precision air pollution exposure. Fourth, although we adjusted for many covariates, residual and unmeasured factors might have contributed to the observed associations between CQI and GDM. Fifth, longitudinal statistical and analytical results cannot be involved in this study, future longitudinal researches should be conducted to provide more strengthened evidences between CQI, air pollution and GDM.
High quality carbohydrate intake pre-pregnancy is associated with a decreased risk of GDM. A CQI related dietary intervention pre-pregnancy to prevent GDM incidence should be considered. However, the modification effects of air pollution during pregnancy cannot be ignored, and women who are preparing to be pregnant should avoid high exposure to PM2.5, PM10, and SO2 during pregnancy.
The original contributions presented in this study are included in the article/Supplementary material, further inquiries can be directed to the corresponding author.
The studies involving human participants were reviewed and approved by the Ethics Committee in Shengjing Hospital of China Medical University. The patients/participants provided their written informed consent to participate in this study.
HZ and YX analyzed the cohort data and were responsibility for the integrity of the data and the accuracy of the data analysis. HZ was a major contributor in writing the manuscript. QC and XZ reviewed and revised the manuscript. YZ designed the study process. All authors read and approved the final manuscript.
This work was supported by the National Natural Science Foundation of China (no. 82103791), the Natural Science Foundation of Liaoning Province (no. 2020MS172), and the 345 Talent Project of Shengjing Hospital of China Medical University (no. M0294).
We sincerely thank for all the participants and filed worker in NEC-biobank Study. We thank International Science Editing (http://www.internationalscienceediting.com) for editing this manuscript.
The authors declare that the research was conducted in the absence of any commercial or financial relationships that could be construed as a potential conflict of interest.
All claims expressed in this article are solely those of the authors and do not necessarily represent those of their affiliated organizations, or those of the publisher, the editors and the reviewers. Any product that may be evaluated in this article, or claim that may be made by its manufacturer, is not guaranteed or endorsed by the publisher.
The Supplementary Material for this article can be found online at: https://www.frontiersin.org/articles/10.3389/fnut.2022.992472/full#supplementary-material
1. Plows JF, Stanley JL, Baker PN, Reynolds CM, Vickers MH. The pathophysiology of gestational diabetes mellitus. Int J Mol Sci. (2018) 19:3342. doi: 10.3390/ijms19113342
2. Rahnemaei FA, Pakzad R, Amirian A, Pakzad I, Abdi F. Effect of gestational diabetes mellitus on lipid profile: a systematic review and meta-analysis. Open Med. (2022) 17:70–86. doi: 10.1515/med-2021-0408
3. McIntyre HD, Catalano P, Zhang C, Desoye G, Mathiesen ER, Damm P. Gestational diabetes mellitus. Nat Rev Dis Primers. (2019) 5:47. doi: 10.1038/s41572-019-0098-8
4. Buchanan TA, Xiang AH, Page KA. Gestational diabetes mellitus: risks and management during and after pregnancy. Nat Rev Endocrinol. (2012) 8:639–49. doi: 10.1038/nrendo.2012.96
5. Cui Y, Liao M, Xu A, Chen G, Liu J, Yu X, et al. Association of maternal pre-pregnancy dietary intake with adverse maternal and neonatal outcomes: a systematic review and meta-analysis of prospective studies. Crit Rev Food Sci Nutr. (2021) [Online ahead of print]. doi: 10.1080/10408398.2021.1989658
6. Jovanovic-Peterson L, Peterson CM. Dietary manipulation as a primary treatment strategy for pregnancies complicated by diabetes. J Am Coll Nutr. (1990) 9:320–5. doi: 10.1080/07315724.1990.10720387
7. Trumbo P, Schlicker S, Yates AA, Poos M. Food and Nutrition Board of the Institute of Medicine, The National Academies. Dietary reference intakes for energy, carbohydrate, fiber, fat, fatty acids, cholesterol, protein and amino acids. J Am Diet Assoc. (2002) 102:1621–30. doi: 10.1016/S0002-8223(02)90346-9
8. Sweeting A, Mijatovic J, Brinkworth GD, Markovic TP, Ross GP, Miller JB, et al. The carbohydrate threshold in pregnancy and gestational diabetes: how low can we go? Nutrients. (2021) 13:2599. doi: 10.3390/nu13082599
9. Desrosiers TA, Siega-Riz AM, Mosley BS, Meyer RE. National birth defects prevention study. Low carbohydrate diets may increase risk of neural tube defects. Birth Defects Res. (2018) 110:901–9. doi: 10.1002/bdr2.1198
10. Hernandez TL, Van Pelt RE, Anderson MA, Daniels LJ, West NA, Donahoo WT, et al. A higher-complex carbohydrate diet in gestational diabetes mellitus achieves glucose targets and lowers postprandial lipids: a randomized crossover study. Diabetes Care. (2014) 37:1254–62. doi: 10.2337/dc13-2411
11. Asemi Z, Samimi M, Tabassi Z, Esmaillzadeh A. The effect of DASH diet on pregnancy outcomes in gestational diabetes: a randomized controlled clinical trial. Eur J Clin Nutr. (2014) 68:490–5. doi: 10.1038/ejcn.2013.296
12. Hernandez TL, Van Pelt RE, Anderson MA, Reece MS, Reynolds RM, de la Houssaye BA, et al. Women with gestational diabetes mellitus randomized to a higher-complex carbohydrate/low-fat diet manifest lower adipose tissue insulin resistance, inflammation, glucose, and free fatty acids: a pilot study. Diabetes Care. (2016) 39:39–42. doi: 10.2337/dc15-0515
13. Looman M, Schoenaker D, Soedamah-Muthu SS, Geelen A, Feskens EJM, Mishra GD. Pre-pregnancy dietary carbohydrate quantity and quality, and risk of developing gestational diabetes: the Australian Longitudinal Study on Women’s Health. Br J Nutr. (2018) 120:435–44. doi: 10.1017/S0007114518001277
14. Zazpe I, Sánchez-Taínta A, Santiago S, de la Fuente-Arrillaga C, Bes-Rastrollo M, Martínez JA, et al. Association between dietary carbohydrate intake quality and micronutrient intake adequacy in a Mediterranean cohort: the SUN (Seguimiento Universidad de Navarra) Project. Br J Nutr. (2014) 111:2000–9. doi: 10.1017/S0007114513004364
15. Munoz-Cabrejas A, Laclaustra M, Guallar-Castillon P, Casasnovas JA, Jarauta E, Sandoval-Insausti H, et al. High-quality intake of carbohydrates is associated with lower prevalence of subclinical atherosclerosis in femoral arteries: The AWHS study. Clin Nutr. (2021) 40:3883–9. doi: 10.1016/j.clnu.2021.04.049
16. Hu Q, Wang D, Yue D, Xu C, Hu B, Cheng P, et al. Association of ambient particle pollution with gestational diabetes mellitus and fasting blood glucose levels in pregnant women from two Chinese birth cohorts. Sci Total Environ. (2021) 762:143176. doi: 10.1016/j.scitotenv.2020.143176
17. Sorensen M, Poulsen AH, Hvidtfeldt UA, Frohn LM, Ketzel M, Christensen JH. Exposure to source-specific air pollution and risk for type 2 diabetes: a nationwide study covering Denmark. Int J Epidemiol. (2022) 51:1219–29. doi: 10.1093/ije/dyac040
18. Venter ZS, Aunan K, Chowdhury S, Lelieveld J. Air pollution declines during COVID-19 lockdowns mitigate the global health burden. Environ Res. (2021) 192:110403. doi: 10.1016/j.envres.2020.110403
19. Hehua Z, Yang X, Qing C, Shanyan G, Yuhong Z. Dietary patterns and associations between air pollution and gestational diabetes mellitus. Environ Int. (2021) 147:106347. doi: 10.1016/j.envint.2020.106347
20. Lim CC, Hayes RB, Ahn J, Shao Y, Silverman DT, Jones RR, et al. Mediterranean diet and the association between air pollution and cardiovascular disease mortality risk. Circulation. (2019) 139:1766–75. doi: 10.1161/CIRCULATIONAHA.118.035742
21. Romieu I, Barraza-Villarreal A, Escamilla-Nunez C, Texcalac-Sangrador JL, Hernandez-Cadena L, Díaz-Sánchez D, et al. Dietary intake, lung function and airway inflammation in Mexico City school children exposed to air pollutants. Respir Res. (2009) 10:122. doi: 10.1186/1465-9921-10-122
22. Zhang H, Xia Y, Cao L, Chang Q, Zhao Y. Associations between long term exposures to outdoor air pollution and indoor solid fuel use and depression in China. J Environ Manage. (2022) 302(Pt A):113982. doi: 10.1016/j.jenvman.2021.113982
23. Yang Y. China Food Composition (Standard Edition)M. Beijing: Peking University Medical Press (2018).
24. Finley CE, Barlow CE, Halton TL, Haskell WL. Glycemic index, glycemic load, and prevalence of the metabolic syndrome in the cooper center longitudinal study. J Am Diet Assoc. (2010) 110:1820–9. doi: 10.1016/j.jada.2010.09.016
25. Zhang H, Zhao Y. Land use regression for spatial distribution of urban particulate matter (PM10) and sulfur dioxide (SO2) in a heavily polluted city in Northeast China. Environ Monit Assess. (2019) 191:712. doi: 10.1007/s10661-019-7905-2
26. Metzger BE, Gabbe SG, Persson B, Buchanan TA, Catalano PA, Damm P, et al. International association of diabetes and pregnancy study groups recommendations on the diagnosis and classification of hyperglycemia in pregnancy. Diabetes Care. (2010) 33:676–82. doi: 10.2337/dc10-0719
27. Xiang M, Konishi M, Hu H, Takahashi M, Fan W, Nishimaki M, et al. Reliability and validity of a chinese-translated version of a pregnancy physical activity questionnaire. Matern Child Health J. (2016) 20:1940–7. doi: 10.1007/s10995-016-2008-y
28. Seo Y, Kim EM, Choi JS, Park CY. Using a mobile-based nutritional intervention application improves glycemic control but reduces the intake of some nutrients in patients with gestational diabetes mellitus: a case series study. Clin Nutr Res. (2020) 9:73–9. doi: 10.7762/cnr.2020.9.1.73
29. Mustad VA, Huynh DTT, López-Pedrosa JM, Campoy C, Rueda R. The role of dietary carbohydrates in gestational diabetes. Nutrients. (2020) 12:385. doi: 10.3390/nu12020385
30. Allehdan S, Basha A, Hyassat D, Nabhan M, Qasrawi H, Tayyem R. Effectiveness of carbohydrate counting and Dietary Approach to Stop Hypertension dietary intervention on managing Gestational Diabetes Mellitus among pregnant women who used metformin: a randomized controlled clinical trial. Clin Nutr. (2022) 41:384–95. doi: 10.1016/j.clnu.2021.11.039
31. Farabi SS, Hernandez TL. Low-carbohydrate diets for gestational diabetes. Nutrients. (2019) 11:1737. doi: 10.3390/nu11081737
32. Liu S, Yin X, Hou C, Liu X, Ma H, Zhang X, et al. As a staple food substitute, oat and buckwheat compound has health-promoting effects for diabetic rats. Front Nutr. (2021) 8:762277. doi: 10.3389/fnut.2021.762277
33. Cui M, Li X, Yang C, Wang L, Lu L, Zhao S, et al. Effect of carbohydrate-restricted dietary pattern on insulin treatment rate, lipid metabolism and nutritional status in pregnant women with gestational diabetes in Beijing, China. Nutrients. (2022) 14:359. doi: 10.3390/nu14020359
34. Hasbullah FY, Mohd Yusof BN, Shariff ZM, Rejali Z, Yong HY, Mitri J. Factors associated with dietary glycemic index and glycemic load in pregnant women and risk for gestational diabetes mellitus. Int J Food Sci Nutr. (2020) 71:516–24. doi: 10.1080/09637486.2019.1686752
35. Hu CY, Gao X, Fang Y, Jiang W, Huang K, Hua XG, et al. Human epidemiological evidence about the association between air pollution exposure and gestational diabetes mellitus: systematic review and meta-analysis. Environ Res. (2020) 180:108843. doi: 10.1016/j.envres.2019.108843
36. Romieu I, Castro-Giner F, Kunzli N, Sunyer J. Air pollution, oxidative stress and dietary supplementation: a review. Eur Respir J. (2008) 31:179–97. doi: 10.1183/09031936.00128106
37. Guxens M, Aguilera I, Ballester F, Estarlich M, Fernández-Somoano A, Lertxundi A, et al. Prenatal exposure to residential air pollution and infant mental development: modulation by antioxidants and detoxification factors. Environ Health Perspect. (2012) 120:144–9. doi: 10.1289/ehp.1103469
38. Tong H, Rappold AG, Diaz-Sanchez D, Steck SE, Berntsen J, Cascio WE, et al. Omega-3 fatty acid supplementation appears to attenuate particulate air pollution-induced cardiac effects and lipid changes in healthy middle-aged adults. Environ Health Perspect. (2012) 120:952–7. doi: 10.1289/ehp.1104472
39. Lin Z, Chen R, Jiang Y, Xia Y, Niu Y, Wang C, et al. Cardiovascular benefits of fish-oil supplementation against fine particulate air pollution in China. J Am Coll Cardiol. (2019) 73:2076–85. doi: 10.1016/j.jacc.2018.12.093
40. Tong H, Rappold AG, Caughey M, Hinderliter AL, Bassett M, Montilla T, et al. Dietary supplementation with olive oil or fish oil and vascular effects of concentrated ambient particulate matter exposure in human volunteers. Environ Health Perspect. (2015) 123:1173–9. doi: 10.1289/ehp.1408988
41. Münzel T, Sørensen M, Gori T, Schmidt FP, Rao X, Brook FR, et al. Environmental stressors and cardio-metabolic disease: part II-mechanistic insights. Eur Heart J. (2017) 38:557–64. doi: 10.1093/eurheartj/ehw294
42. Zhang H, Wang Q, He S, Wu K, Ren M, Dong H, et al. Ambient air pollution and gestational diabetes mellitus: a review of evidence from biological mechanisms to population epidemiology. Sci Total Environ. (2020) 719:137349. doi: 10.1016/j.scitotenv.2020.137349
43. Tryggvadottir EA, Halldorsson TI, Landberg R, Hrolfsdottir L, Birgisdottir BE, Magnusdottir OK, et al. Higher alkylresorcinol concentrations, a consequence of whole-grain intake, are inversely associated with gestational diabetes mellitus in Iceland. J Nutr. (2021) 151:1159–66. doi: 10.1093/jn/nxaa449
44. Zhang X, Gong Y, Della Corte K, Yu D, Xue H, Shan S, et al. Relevance of dietary glycemic index, glycemic load and fiber intake before and during pregnancy for the risk of gestational diabetes mellitus and maternal glucose homeostasis. Clin Nutr. (2021) 40:2791–9. doi: 10.1016/j.clnu.2021.03.041
45. Chatzakis C, Sotiriadis A, Tsakmaki E, Papagianni M, Paltoglou G, Dinas K, et al. The effect of dietary supplements on oxidative stress in pregnant women with gestational diabetes mellitus: a network meta-analysis. Nutrients. (2021) 13:2284. doi: 10.3390/nu13072284
46. Lappas M, Hiden U, Desoye G, Froehlich J, Hauguel-de Mouzon S, Jawerbaum A. The role of oxidative stress in the pathophysiology of gestational diabetes mellitus. Antioxid Redox Signal. (2011) 15:3061–100. doi: 10.1089/ars.2010.3765
47. Chen X, Scholl TO. Oxidative stress: changes in pregnancy and with gestational diabetes mellitus. Curr Diab Rep. (2005) 5:282–8. doi: 10.1007/s11892-005-0024-1
48. Padula AM, Yang W, Lurmann FW, Balmes J, Hammond SK, Shaw GM, et al. Prenatal exposure to air pollution, maternal diabetes and preterm birth. Environ Res. (2019) 170:160–7. doi: 10.1016/j.envres.2018.12.031
Keywords: nutrition, air pollution, gestational diabetes mellitus, interaction, birth cohort
Citation: Zhang H, Xia Y, Zhang X, Chang Q and Zhao Y (2023) Carbohydrate intake quality and gestational diabetes mellitus, and the modifying effect of air pollution. Front. Nutr. 9:992472. doi: 10.3389/fnut.2022.992472
Received: 12 July 2022; Accepted: 15 December 2022;
Published: 05 January 2023.
Edited by:
Stefan Kabisch, Charité – Universitätsmedizin Berlin, GermanyReviewed by:
Daniela Kretzer, Federal University of Rio Grande do Sul, BrazilCopyright © 2023 Zhang, Xia, Zhang, Chang and Zhao. This is an open-access article distributed under the terms of the Creative Commons Attribution License (CC BY). The use, distribution or reproduction in other forums is permitted, provided the original author(s) and the copyright owner(s) are credited and that the original publication in this journal is cited, in accordance with accepted academic practice. No use, distribution or reproduction is permitted which does not comply with these terms.
*Correspondence: Yuhong Zhao, ✉ eWh6aGFvc2pfaG9zcGl0YWxAMTI2LmNvbQ==, ✉ emhhb3loQHNqLWhvc3BpdGFsLm9yZw==
†These authors have contributed equally to this work
Disclaimer: All claims expressed in this article are solely those of the authors and do not necessarily represent those of their affiliated organizations, or those of the publisher, the editors and the reviewers. Any product that may be evaluated in this article or claim that may be made by its manufacturer is not guaranteed or endorsed by the publisher.
Research integrity at Frontiers
Learn more about the work of our research integrity team to safeguard the quality of each article we publish.